Abstract
Creating and developing purchase intention in social media is an important strategic goal for businesses. Marketers, in particular, feel the need to invest in consumer-brand engagement (CBE) in social media marketing more than ever before as social media platforms are available for consumers to socialize and interact with each other. However, current research in marketing provides little guidance to marketers how brand pages in Facebook can be leveraged to engage customers and create purchase intention for the brands. Toward filling this gap, the present research examines the customer’s brand engagement-purchase intention link with the mediating role of customer’s information motivation in social media. Using data from 298 surveys of brand pages are analysed in partial-least squares (PLS) structural equation modelling (SEM). The research fills a gap in the literature through its analysis of the mediating role of information motivation of consumers in the relationship between customer-brand engagement and purchase intention in social media. The specific strategies, and the implications of this research for theory and practise are discussed.
Keywords: Customer-Brand engagementPurchase intentionInformation motivationSocial media marketing
Introduction
As social media networking has expanded dramatically in the past decade, emphasis in social media marketing among marketing practitioners and scholars has grown rapidly worldwide. Companies become more active in including social networks such as Facebook, as part of their marketing communications, thus they have turned their interest to questions regarding the customers’ purchase intention: Can social media marketing activities create customers’ purchase intention.
Researchers have explored some benefits of social media marketing activities. They describe
Customer-brand engagement (CBE) is a recent concept in the marketing literature growing in the domain of relationship marketing. CBE has been gained the importance in the social media environment, and there is an interest in how brands can leverage new media and online social communities on platforms such as Facebook, Twitter or YouTube to engage and collaborate with customers due to social media’s multi-dimensional, two-way peer-to-peer communication properties (Yan, 2011; Jahn and Kunz, 2012; Brodie et al, 2013; Hutter et al, 2013).
There has been significant growth in the adoption and use of brand pages found on the Facebook social networking platform by consumers to communicate with their favorite brands and with one another. In this sense, consumers are becoming pivotal authors of brand stories through the easy sharing of brand experiences due to the triad of communication arising from new dynamic networks among consumers and brands formed through social media (Gensler et al, 2013).
The construct of purchase intention (PI) as indicator of customer loyalty has always interest from both academics and practitioners. This is because customer loyalty is an important asset in today’s intensely competitive environment (Keller, 2001; Brodie et al, 2011).
In recent years, there has been greater emphasis on CBE in branding and relationship marketing, which has been argued to act as a vehicle for enhancing consumer relationship. CE can be defined as a psychological state reflecting customers’ interactive, co-creative experiences with a brand, which highlights the active role of consumer (Brodie et al, 2011).
Foundations of the study
Brand Engagement
Brand engagement (BE) can be expressed as a composite of experiential and social dimensions (Gambetti et al., 2012; Verleye, Gemmel, and Rangarajan, 2014). Customer-Brand engagement (CBE) can be defined as “the level of an individual customer’s motivational, brand-related and context-dependent state of mind characterised by specific levels of cognitive, emotional and behavioural activity in direct brand interactions” (Hollebeek, 2011, p. 790). This research concentrates on the “social dimension” of CBE (Gambetti et al., 2012, p. 681).
Facebook permits consumers to interact with brands, and thus with others who share the same brand preferences (Hollenbeck and Zinkhan, 2006). On Facebook, self-identity is created through consumers’ definitions of themselves, and how they attach to others within a network (Schau and Gilly, 2003). Consumers who select “like” for a brand may do so to allow that brand to express their ideal or actual selves (Ahuvia, 2005; Trusov et al., 2010). We draw on Sprott et al. (2009), who considered the role of brand engagement in the self-concept, as consumers’ propensity to include brands as part of how they view themselves. On Facebook, consumers engaging with brands, state they “like” them to build part of their online self-expression (Lipsman et al., 2012; Trusov et al., 2009).
Information Motivation
Motivation can be defined as goal-directed arousal (Park and Mittal 1985). In the current context, the goal is restricted to the processing of brand information. Thus, motivation is defined as consumers' desire or readiness to process brand information in an ad. That view is consistent with recent definitions such as readiness (Burnkrant 1976; Burnkrant and Sawyer 1983; Moorman 1990), willingness (Roberts and Maccoby 1973), interest (Celsi and Olson 1988), and desire (Petty and Cacioppo 1986) to process infor-mation in a persuasive communications context. Though slightly different, those terms each suggest heightened arousal to process external stimuli such as brand in-formation. High motivation implies that consumers are willing to allocate processing resources to brand information in an ad.
Purchase Intention
Venkatraman and Ramanujam (1986) offer a two-dimensional classification scheme for evaluation of performance. On the one hand, they differentiate financial and operational indicators, and on the other hand, they distinguish between primary and secondary source of information (Falk, 1997). While financial measures are related to accounting measures and economic performance (e.g. profit, sales), operational measures are related to operational success factors that might lead to financial performance like customer satisfaction, quality, market share or new product development (Hirschman and Holbrook 1982; Venkatraman, and Ramanujam, 1986). From the point of the view of the source of information, data for primary measures is collected from organization while data for secondary measures are collected from external or derivative databases. Another classification distinguishes between objective and subjective measures. Objective measures refer to performance indicators impartially quantified. They are usually financial indicators obtained directly from organizations through secondary sources. On the other hand subjective measures refer to the judgmental assessment of internal or external respondents. They usually cover both financial and operational/commercial indicators.
Conceptual Model and Hypotheses Development
The conceptual model to study the effects of the mediation role of information motivation in the relationship between consumers’ brand engagement and purchase intention is presented Fig.
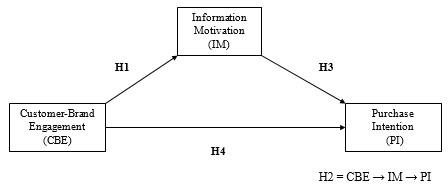
H1. Customer brand engagement (CBE) is positively associated with purchase intentions (PI).
H2. The relationship between information motivation (IM) and purchase intentions (PI) is mediated by information motivation
H3. Information motivation (IM) is positively associated with purchase intention.
H4. Customer brand engagement (CBE) is positively associated with information motivation (IM).
Method
Sample and data collection
The sampling frame of the research consists of students from one of the well-known university in Istanbul, Turkey. Undergraduate students completed the paper and pen survey in the class. Students were representative of young population that is active on Facebook. All respondents had Facebook profiles. A total of three-hundred and eight students participated in the study. After removing invalid or incomplete responses, 297 valid responses were obtained for the final analysis. The average age of students was 19.8 years and the sample had 42.2% females.
Measures
All the constructs in the study were measured by five-point multi-item scales (“1” = strongly disagree and “5” = strongly agree) which were either adapted extant literature and modified to suit the study context (see Table
Facebook is commonly used by students. popular among students. Facebook has been seen as a digital public arena where young consumers can attach virtually, and its popularity among this age companion in part mirrors younger consumers’ motivations to gain interest from others (Bowley, 2006). Furthermore, past studies of Facebook users employed student populations (e.g. Hunt et al., 2012; Lewis et al., 2008; Patterson, 2011). Further, student samples have importance for the constructs of the study. To inspire responses of the students, extra grade was offered as an incentive. Sample population is 308. As the literature takes into account “likes” as a manifest variable of brand management on social networks (Chauhan and Pillai, 2013; Hoffman and Fodor, 2010; Malthora et al., 2013). Participants were required to determine a brand they “liked” on Facebook. Brands “liked” were in the following categories; fashion brands 20%, sports wear 15%, cosmetics 15 %, electronics 10%, other web sites 40%.
The participants were asked to report their average daily Facebook usage in hour/minutes. Also, a pre-test of the questionnaire was administered to 30 students that they could understand the statements correctly. Based on their feedback, minor modifications were made to finalize the survey.
Information motivations were measured by three items adapted from Ko et al. (2009) and Cousins and Menguc (2006). Customer-brand engagements were measured by six items adapted from Keller (2011). Purchase intentions were measured by seven items adapted from Cronin et.al. (2000), Ha and Janda (2012).
Model estimation and results
A structural equation modeling (SEM) with partial least square (PLS) approach was used to test the proposed model and the hypotheses. The PLS was selected for the following reasons: (a) the focus of this study is to predict the purchase intentions, and (b) the study uses latent variables' scores in further mediation analysis (Hair, Hult, Ringle, & Sarstedt, 2016). PLS analysis was conducted using the SmartPLS 3.2.4 software (Ringle, Wende, & Becker, 2015). The mediation analysis was carried out using in SmartPLS.
Since this study is based on self-reported data, the potential issue of common method variance (CMV) was analyzed (Podsakoff, MacKenzie, Lee, & Podsakoff, 2003). As a preemptive approach, to reduce the likelihood of CMV students were assured of anonymity and confidentiality (Chang, Witteloostuijn, & Eden, 2010). A post hoc Harman's singlefactor test revealed that the first factor accounts only for 22% of the variance. Further, marker-variable technique was performed for CMV validity checks and results indicated that the difference between the original and CMV-adjusted correlations were very small (≤0.06) for all the relevant constructs (Lindell & Whitney, 2001). Hence, it can be concluded that CMV does not seriously distort the results and predictions in this study.
Assessment of the measurement model
An evaluation of the measurement model was undertaken by assessing internal consistency (composite reliability), item-indicator reliability, convergent validity, and discriminant validity. All the outer loadings of the constructs were statistically significant, and the composite reliability (CR) values were above the recommended value of 0.7 (Table
The discriminant validity was confirmed by examining the cross loadings and through the Fornell-Larcker criteria. Each indicator's loading was checked, and none loaded higher on any construct other than on its own. The square root of AVE for each construct was higher than the inter-construct correlations (Table
In Table
Assessment of the structural model
The structural model was estimated using the bias-corrected and accelerated (Efron, 1987) bootstrapping procedure with 5000 resamples. The predictors were checked for multicollinearity using VIF values for each construct, which were lower than the recommended value of 5 (Hair et al., 2016). The R2 value of 21% (p < 0.001) was obtained for predicting the information motivation and R2 value of 56% (p < 0.001) purchase intentions in the model. The blindfolding procedure was performed (omission distance = 7) to obtain cross-validated redundancy measures for each dependent construct. The resulting positive Q2 value for information motivation (0.118) and Q2 value for purchase intention (0.375) indicates that the model has predictive relevance.
We followed the recommendations from Henseler et al. (2014) to assess the overall model fit by using standardized root mean square residual (SRMR) as an index for model validation. Generally, values below 0.08 are considered favorable (Hu & Bentler, 1999). The model estimation with PLS reveals a SRMR value of 0.067 and the estimation with PLSc indicates a SRMR value of 0.038.
The PLS analysis results (Table
The results shown in Fig.
The explanatory power of PI increases substantially (21.8%), to reach 55.6%, which highlights the significant influence that IM has on the PI.
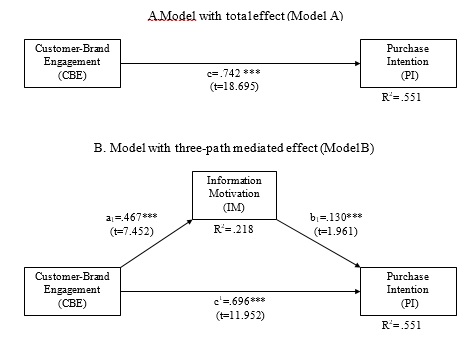
All the direct effects in Fig. 1B are significant. The percentile bootstrap at a 95% confidence interval also has this outcome (Table
Mediation analysis
Tests on the mediation hypotheses (H2) use an application of the analytical approach that Hayes, Preacher, and Myers (2011) describe. Fig.
The application of bootstrapping permits for testing of the mediation hypotheses (Preacher, & Hayes, 2008). This study's 5000 resamples generate 95% confidence intervals (percentile) for the mediator. As Fig. 1A and Table
Results
In this study, partial least squares (PLS) was used to analyse the data and test the hypotheses model, by employing SmartPLS software to analyse the measurement and structural models. PLS facilitates the analysis of complex models with many manifest and latent variables (Henseler, Ringle, and Sinkovics, 2009). In addition, PLS deploys a component-based approach which lowers the requirement for sample size and residual distributions (Gefen, Straub, & Boudreau, 2000). PLS also does not demand stringent assumptions about the manifest and latent variables' distributions, which is best for testing data with skewed or non-normal distributions, or the inter-related observations (Falk & Miller, 1992).
Table
Table
Conclusion and Discussions
Theoretical Implications
Academically, this study builds on social media literature by empirically examining antecedents and outcomes of purchase intention (Smith, Dinev, and Xu 2011). Although the popularity of social media and the fact that marketing practitioners are turning to social media as necessary platforms, little academic research has been performed to help marketing practitioners understand the best practices for creating customers’ purchase intention. The results offer some guidance for engaging consumers through carefully designed message and information content and message cues to foster and generate purchase intention.
Managerial Implications
Marketing practitioners must meet consumers’ motivations for information and engagement to heighten relationships, which then create purchase intention. That is, when marketers develop social media pages that allow consumers to feel entertained and informed by connected with brands, friends and improve customer equity.
Limitations and further research
In this research, liking and commenting behaviors of participants were used as part of consumer engagement with brands on Facebook. However, the recently introduced “share” function, which gives the users the opportunity to share posts on their timeline has also become quite popular among consumers. In fact, now whenever there is a new post on Facebook, there are three buttons below each post for consumers to choose from: like, comment and share. Future studies nd test the relationships between personality traits, modes of interaction and sharing in the way we tested our conceptual model. The profile of our respondents fits the overall profile of heavy social media users. However, given the increasing use of social media by different age cohorts, future studies may want to test should extend our conceptual model by including “share” in addition to liking and commenting behaviors our model with respondents from different age groups to see if the results will hold. Finally, while we agree with Sashi (2012) that there is a need for more studies on the nature of consumer engagement in social media, it is also necessary to understand the effects of heavily discussed engagement behaviors on brands’ financial performance. For example, in this study, we investigated liking and commenting behaviors as examples of consumer engagement and presented a model
That included the factors that affect those two types of behaviors. However, it would be critical and useful for brands to understand if such behavior results in higher likelihood of purchase of the same brand by the consumers who liked or commented on the brand’s Facebook page.
This study contributes to the proposed theory and provides imperative implications for practice, but several limitations exist, thus suggesting direction for further research. First, the data came from a single country. As such, the findings are restricted to a specific national context. These differences affect the generalizability of the empirical results to other countries. One of the major limitations of this study is that data was collected only from Facebook in social media world. Future research should take into account to analyze the relationship between the study constructs (CBE, IM, and PI) in different social media platforms such as Twitter, Instagram, Youtube etc. This study identifies quantity and creditability as one outcome construct; future studies may consider the other dimensions of customer loyalty. Furthermore, future studies should consider other dimensions of customer-brand engagement as an antecedent variable. Since in this study social dimension of brand engagement was used. Overall, we believe that despite its limitations, this research is an important step in understanding the motives and factors affecting consumers’ Facebook behavior and customer-brand engagement, information motivation and purchase intention in social media, and it offers useful insights for practitioners intending to use Facebook as part of their marketing strategy.
References
- Ahuvia, A. C. (2005). Beyond the extended self: Loved objects and consumers' identity narratives. Journal of consumer research, 32(1), 171-184.
- Berthon, P. R., Pitt, L. F., Plangger, K., & Shapiro, D. (2012). Marketing meets Web 2.0, social media, and creative consumers: Implications for international marketing strategy. Business horizons, 55(3), 261-271.
- Brodie, R.J., Hollebeek, L.D., Juric, B. and Ilic, A. (2011) Customer engagement: Conceptual domain, fundamental propositions, and implications for research. Journal of Service Research 14(3): 252–271.
- Brodie, R.J., Ilic, A., Juric, B. and Hollebeek, L. (2013) Consumer engagement in a virtual brand community: An exploratory analysis. Journal of Business Research 66(1): 105–114.
- Burnkrant, R. E. (1976). A motivational model of information processing intensity. Journal of Consumer Research, 3(1), 21-30.
- Burnkrant, R. E., & Sawyer, A. G. (1983). Effects of involvement and message content on information-processing intensity. Information processing research in advertising, 12(2), 46-64.
- Celsi, Richard L. and Jerry Olson (1988), The Role of In-volvement in Attention and Comprehension Processes, Journal of Consumer Research, 15 (September), 210-24.
- Chang, S. J., Van Witteloostuijn, A., & Eden, L. (2010). From the editors: Common method variance in international business research. Journal of International Business Studies, 41(2), 178-184.
- Cousins, P. D., & Menguc, B. (2006). The implications of socialization and integration in supply chain management. Journal of operations management, 24(5), 604-620.
- Cronin, J. J., Brady, M. K., & Hult, G. T. M. (2000). Assessing the effects of quality, value, and customer satisfaction on consumer behavioral intentions in service environments. Journal of Retailing , 76 (2), 193–218
- Efron, B. (1987). Better bootstrap confidence intervals. Journal of the American statistical Association, 82(397), 171-185.
- Falk, R. F., & Miller, N. B. (1992). A primer for soft modeling. University of Akron Press.
- Falk, P. (1997). "The scopic regimes of shopping", in Falk, P. and Campbell, C. (Eds.), The Shopping Experience, Saga Publications, London, pp. 177–185.
- Gambetti, R. C., Graffigna, G., & Biraghi, S. (2012). The grounded theory approach to consumer-brand engagement. International Journal of Market Research, 54(5), 659-687.
- Gefen, D., Straub, D. W., & Boudreau, M. -C. (2000). Structural equation modeling and regression: Guidelines for research practice. Communications of the Association for Information Systems, 4, 1–79.
- Gensler, S., Völckner, F., Liu-Thompkins, Y., & Wiertz, C. (2013). Managing brands in the social media environment. Journal of Interactive Marketing, 27(4), 242-256.
- Ha, H.-Y., Janda, S., 2012. Predicting consumer intentions to purchase energy-efficient products. J. Consum. Mark. 29 (7), 461–469.
- Ha, H.-Y., Janda, S., 2012. Predicting consumer intentions to purchase energy-efficient products. J. Consum. Mark. 29 (7), 461–469..
- Hair Jr, J. F., Hult, G. T. M., Ringle, C., & Sarstedt, M. (2016). A primer on partial least squares structural equation modeling (PLS-SEM). Sage Publications.
- Hair, J.F., Hult, G.T.M., Ringle, C.M., Sarstedt, M., (2016). A Primer on Partial Least Squares Structural Equation Modeling (PLS-SEM). Sage, Thousand Oaks.
- Hayes, A. F., Preacher, K. J., & Myers, T. A. (2011). Mediation and the estimation of indirect effects in political communication research. Sourcebook for political communication research: Methods, measures, and analytical techniques, 23, 434-465.
- Henseler, J., Ringle, C. M., & Sinkovics, R. R. (2009). The use of partial least squares path modelling in international marketing. In New challenges to international marketing (pp. 277-319). Emerald Group Publishing Limited.
- Henseler, J., Dijkstra, T. K., Sarstedt, M., Ringle, C. M., Diamantopoulos, A., Straub, D. W., ... & Calantone, R. J. (2014). Common beliefs and reality about PLS: Comments on Rönkkö and Evermann (2013). Organizational Research Methods, 17(2), 182-209.Hu & Bentler, 1999).
- Henseler, J., Ringle, C. M., & Sarstedt, M. (2015). A new criterion for assessing discriminant validity in variance-based structural equation modeling. Journal of the Academy of Marketing Science, 43(1), 115–135.
- Hirschman, E.C. and Holbrook, M.B. (1982), “Hedonic consumption: emerging concepts, methods and propositions”, Journal of Marketing, Vol. 46, pp. 92-101.
- Hoffman, D. L., & Fodor, M. (2010). Can you measure the ROI of your social media marketing?. MIT Sloan Management Review, 52(1), 41.
- Hollebeek, L. (2011). Exploring customer brand engagement: definition and themes. Journal of strategic Marketing, 19(7), 555-573.
- Hollenbeck, C. R., & Zinkhan, G. M. (2006). Consumer activism on the internet: The role of anti-brand communities. ACR North American Advances.
- Hulland, J. (1999). Use of partial least squares (PLS) in strategic management research: A review of four recent studies. Strategic management journal, 195-204.Henseler, J., Ringle, C. M., & Sinkovics, R. R. (2009). The use of partial least squares path modeling in international marketing. In New challenges to international marketing (pp. 277-319). Emerald Group Publishing Limited.
- Hunt, A., Lucas, S., Spielmann, J., & Cantrell, T. S. (2012). Are-Evaluation Oflate Pennsylvanian Bromalites From The Kinney Brickquarrylagerstätte, Newmexico, Usa. Vertebrate Coprolites: Bulletin 57, 57, 185.
- Hutter, K., Hautz, J., Dennhardt, S. and Fuller, J. (2013) The impact of user interactions in social media on brand awareness and purchase intention: The case of MINI on Facebook. Journal of Product & Brand Management 5/6(22): 342–351.
- Jahn, B. and Kunz, W. (2012) How to transform consumers into fans of your brand. Journal of Service Management 23(3): 344–361.
- Keller, K. L., Parameswaran, M. G., & Jacob, I. (2011). Strategic brand management: Building, measuring, and managing brand equity. Pearson Education India.
- Keller, K.L. (2001), “Building customer-based brand equity: a blueprint for creating strong brands”, working paper series, Marketing Science Institute, Cambridge, MA.
- Ko, H., Cho, C.H. and Roberts, M.S. (2009), “Internet uses and grafications: a structural equation model of interactive advertising”, Journal of Advertising, Vol.34 No. 2, pp.57-70.
- Lindell, M. K., & Whitney, D. J. (2001). Accounting for common method variance in cross-sectional research designs. Journal of applied psychology, 86(1), 114.
- Moorman, C. (1990). The effects of stimulus and consumer characteristics on the utilization of nutrition information. Journal of Consumer Research, 17(3), 362-374.
- Park, C. Whang and Mittal, Banwari. (1985). "A Theory of Involve-ment in Consumer Behavior: Problems and Issues," in Re-search in Consumer Behavior, Vol. 1, Jagdish N. Sheth, ed. Greenwich, CT: JAI Press, Inc., 201-31.
- Patterson, T. E. (2011). Out of Order: An incisive and boldly original critique of the news media's domination of Ameri. Vintage.Podsakoff, MacKenzie, Lee, & Podsakoff, 2003).
- Petty, Richard E. and John T. Cacioppo (1986), Communication and Persua-sion: Central and Peripheral Routes to Attitude Change. New York: Springer-Verlag.
- Preacher, K. J., & Hayes, A. F. (2008). Asymptotic and resampling strategies for assessing and comparing indirect effects in multiple mediator models. Behavior Research Methods, 40, 879–891.
- Ringle, C. M., Wende, S., & Will, A. (2005). SmartPLS 2.0 (beta). Hamburg, Germany: University of Hamburg.
- Roberts, Donald F. and Nathan Maccoby (1973), Information Processing and Persuasion: Counterarguing Behavior," in New Models for Mass Communication Research, Peter Clarke, ed. Beverly Hills, CA: Sage Publications, Inc., 269- 307.
- Schau, J.H., & Gilly, M. C. (2003). We are what we post? Self-presentation in personal web space. Journal of consumer research, 30(3), 385-404.
- Shrout, P. E., & Bolger, N. (2002). Mediation in experimental and nonexperimental studies: New procedures and recommendations. Psychological methods, 7(4), 422-445.
- Smith, H. J., Dinev, T., & Xu, H. (2011). Information privacy research: an interdisciplinary review. MIS quarterly, 35(4), 989-1016.
- Sprott, D., Czellar, S., & Spangenberg, E. (2009). The importance of a general measure of brand engagement on market behavior: Development and validation of a scale. Journal of Marketing Research, 46(1), 92-104.
- Srivastava, R. K., Shervani, T. A., & Fahey, L. (1998). Market-based assets and shareholder value: a framework for analysis. The Journal of Marketing, 2–18.
- Trusov, M., Bodapati, A. and Bucklin, R. (2010), “Determining influential users in internet social networks”, Journal of Marketing, Vol. 47 No. 4, pp. 643-660.
- Trusov, M., Bucklin, R. and Pauwels, K. (2009), “Effects of word-of-mouth versus traditional marketing: findings from an internet social networking site”, Journal of Marketing, Vol. 73 No. 5, pp. 90-102.
- Verleye, K., Gemmel, P. and Rangarajan, D. (2014) Managing engagement behaviors in a network of customers and stakeholders evidence from the nursing home sector. Journal of Service Research 17(1): 68–84.
- Yan, J. (2011) Social media in branding: Fulfilling a need. Journal of Brand Management 18(9): 688–696.
Copyright information
This work is licensed under a Creative Commons Attribution-NonCommercial-NoDerivatives 4.0 International License.
About this article
Publication Date
20 December 2017
Article Doi
eBook ISBN
978-1-80296-033-4
Publisher
Future Academy
Volume
34
Print ISBN (optional)
--
Edition Number
1st Edition
Pages
1-442
Subjects
Business, business studies, innovation
Cite this article as:
Şahin, A., & Şahin, A. (2017). Reflection of Customer-Brand Engagement on Purchase Intention in Social Media. In M. Özşahin (Ed.), Strategic Management of Corporate Sustainability, Social Responsibility and Innovativeness, vol 34. European Proceedings of Social and Behavioural Sciences (pp. 133-145). Future Academy. https://doi.org/10.15405/epsbs.2017.12.02.12