Abstract
Educational institutions training engineers and technical experts operate in a dynamic environment to which they are bound to respond by developing new organizational forms for the adaptation to new conditions. The rapid development of information technology, economic situation, and hardly-predictable events like the waves of the COVID-19 pandemic and the restrictions of people's mobility pose new problems for the directors of educational institutions. The pursuit for a flexible management structure that would satisfy the current requirements brings about the necessity for a formal description of universities and their environments based on the systemic approach. The purpose of this research was to test a model based on a fuzzy cognitive map and assess the possibility of change scenario development in the education system. The authors demonstrate that this approach can conveniently describe the elements of the education system and its environment, as well as the communications and links between them. The authors obtained and analyzed the modeling results showing the possibility of using event scenarios for the facilitation of strategic decisions concerning the management of engineering education at a technical university.
Keywords: COVID-19, digital education, engineering education, modeling
Introduction
The development of the digital education environment is a global development trend (Digital economy and society statistics - enterprises - Statistics Explained, 2021) provoking changes in the education sector. Educational institutions providing training programs in IT play a special role in this process. The economic cycles of the global economy, instability of export prices, demographic processes, border closures due to the COVID-19 pandemic (Coronavirus disease (COVID-19) travel advice, 2021; Vagaeva et al., 2021), and the modifications of the plans concerning international studies are among the causes of instabilities in the operation of universities.
Therefore, the management structure of a technical university must adapt to current requirements and aim for greater flexibility, efficiency, and effectiveness. This may include new communications with the environment, new management systems at universities, and new resource distribution methods. The studies of flexible university structures within the changing social and economic environment have long been classified as a discipline of its own, known as the university adaptation theory (Sporn, 1999). New organizational forms are introduced that support the trends for the expansion of entrepreneurial universities, life-long learning, and quick adjustment of the education system according to the market requirements. In all of the world’s countries, university environments have national specifics grounded in the nation's role in global labor specialization and local traditions. This primarily pertains to external factors: legislative and political, economic, demographic, social, cultural, globalization, and technological. We should also mention internal factors like university missions, their goals, corporate culture, leadership problems, institutional environment, education quality, education costs, efficiency, and availability of learning resources. Each of these factors may be deemed significant.
Figure 1 shows a simplified static model of relations between an educational institution training engineers and the environment according to the authors’ perceptions. Since the weight of the factors and the degree of their impact shift, it is necessary to describe the dynamics of university behaviors as those of an open system adjusting to a new steady state:
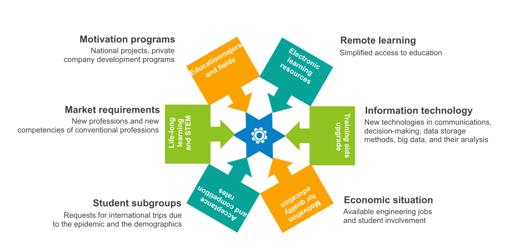
Problem Statement
The authors aimed at complementing the conventional systemic analysis with the tools for development scenario modeling and forecasting for a higher education establishment. The formal description of the elements of a university learning system, as well as links and communications between them and their environment, could help make strategic management decisions that help the institution survive and develop. For the problem in question, it is also interesting to verify the adequacy of the model and the possibility of its application.
Research Questions
The erratic impacts of external factors on the training of engineering competencies are manifested in the conflicts between people’s ambitions to study at the world’s best universities and the travel restrictions caused by the coronavirus pandemic, as well as between the government efforts to develop IT education and economic stagnation or recession, etc. We believe that situation development forecasts are fuzzy problems whose solutions can be sets of scenarios. If we look at a system of work elements and the key components of the environment, the most significant factors impacting the acquisition of digital economy competencies will include the economic situation, the actual level of education support, demographics, and acts of God.
Purpose of the Study
In this research work, we pursue to construct a model reflecting the process of student acquisition of competencies required to work in a digital learning environment (Efremkina, 2018; Pashchenko et al., 2016), featuring various motivation or demotivation options for engineering students. It is interesting to analyze the scenarios for the events occurring in the education system due to external factors to form a development strategy for an educational institution.
Research Methods
We used the fuzzy cognitive map (FCM) (Kosko, 1993) as a modeling tool fit for the research purposes and aligned with the systemic approach in problem-solving. Control system elements are the FCM concept nodes (factors) shown as directed graph nodes. These concept nodes are characterized by numerical levels and connected with each other with directed graph arcs, whose weights represent the intensity of the causal link. Exogenic concepts that do not depend on other concepts belong to the state of the environment and other factors beyond the impact of the object modeled.
FCM is used as a modeling tool in various sectors including scientific experiments (Stylios & Peter, 1999), environmental systems (Devisscher et al., 2016), digital learning systems for computer programing (Chrysafiadi & Virvou, 2013), research institute management (Banerjee, 2009), political studies (Neocleous et al., 2016), medical diagnostic (Papageorgiou et al., 2009), terrorism studies (Osoba & Kosko, 2017), and accident prevention for industrial facilities (Micheev et al., 2018). Moreover, the increasing flow of research works resulted in the emergence of open information repositories and FCM libraries for various sectors like (Know-Why.Net, 2019).
The use of FCM in education is less common (Cole & Persichitte, 1999; Pressley & McCormick, 1995). Nevertheless, some researchers achieved good results in this area. Pressley and McCormick presented FCMs describing students' knowledge construction. Jason R. Cole, Kay A. Persichitte described new application methods for modeling results and specialized approaches to the construction of FCMs for the education sector. The first of the research areas involved the identification of the missing meta-knowledge of students and the identification of the implicit links between the subjects studied. The anticipated result was the improvement of the education system through the elimination of gaps starting from matriculation. In the second research work, the authors suggested the building procedures for the map, concept set, and links between them as exemplified by a remote learning system.
The authors of this article aimed to construct a model version describing the state of a university’s training system against the background of rapidly changing IT competencies and the presence of an adverse environment. Complementing the set of applications of the FCM tools with university operation models is useful for the forecasting of the effects of changes in adaptive higher education institutions.
To build fuzzy cognitive maps, we involved experts who were tasked with the identification of the set of concepts defining the domain area and the formal description of links between them. The use of cognitive maps allowed for a natural unification of the knowledge of several experts for a more adequate description of the domain area. Each of the experts selected their own set of concepts characterizing the set domain area and the links between them. The cognitive maps of each of the experts were incorporated into one final map accounting for all of the expert opinions. This incorporation procedure for the expert knowledge is not provided in this article but its results are shown in Figure 2 as a fuzzy cognitive map.
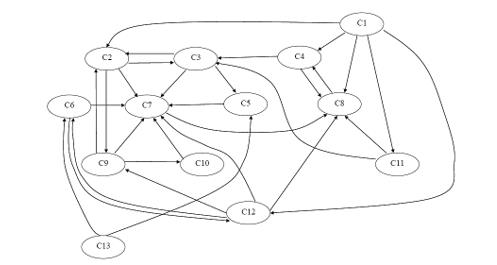
The research is based on expert polling, building a fuzzy cognitive map, transient process simulation for the education system, and analyzing change scenarios.
After the expert polling, we proposed the following list of concepts:
С1 – production decline in the region;
С2 – academic achievement level;
С3 – workforce capacity of the educational institution;
С4 – state of partner companies;
С5 – level of methodological support;
С6 – the quality of enrollee training;
С7 – the quality of learning;
С8 – graduate competence levels;
С9 – extra-budgetary funds;
С10 – the quality of laboratory facilities;
С11 – labor migration of graduates;
С12 – number of students on a contractual basis;
С13 – number of potential enrollees.
Assume that concept levels are integers from 0 to 100, and arc weights are integers from -100 to 100. Arc weights representing the links between concepts are shown in Table 1. Weight values in the table are set “by default”. Concept levels are set at 50 “by default”.
The concepts included in the model are characterized by their initial level that can change during the system operation and by the relations with other concepts with various link intensities. Besides, the model features exogenic concepts (C1, C13) that lack causes to change within the modeled system but reflect the objective causes existing in the environment of a higher education institution. In particular, the number of potential enrollees (C13) may be associated with the country's demographics and with the effects of the end of the pandemic and the recovery of international travel for studying. In the model suggested, we assume that the remaining concepts are the causes that can be changed within the education system.
Modeling was carried out using the method implemented by Guillermo Ochoa de Aspuru (2017) in his Java application:
k is the iteration number;
is the level of factor i between 0 and 100;
is the result of factor j impacting factor i;
is the direction of the impact that can be -1 or 1;
is the intensity of the causal link ranging from 0 to 100.
Findings
The experiment included the assessment of the education system at the average concept and link states and various extreme states of external concepts. Besides, we assessed specifically the intensity of the link between actions taken by the government to fight back the epidemic and improve the demographics and the state of the learning system within a higher education institution.
The list of factors was developed in the main dialog window of the program (Figure 3), and link intensity (effect) values and the initial values of factor levels were entered in the editor window (Figure 4). The illustration shows an example of a positive link entry between the effect concept C5 and cause concept C3 at an intensity of 70.
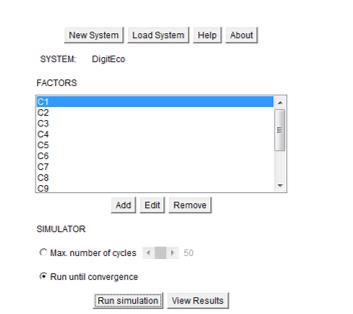
The iteration process launched to shift the map into a new steady-state was stopped using the zero adjustment norm between the vectors of factor state in consecutive iterations (run until convergence). The simulation finishing state is shown in Figure 5. The results of the research using the cognitive map were obtained in iteration processes with convergence reached in all cases. By default, concept levels and link intensities (in absolute magnitude) were equal to 50.
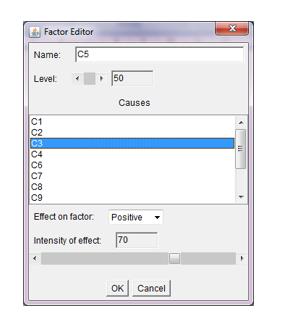
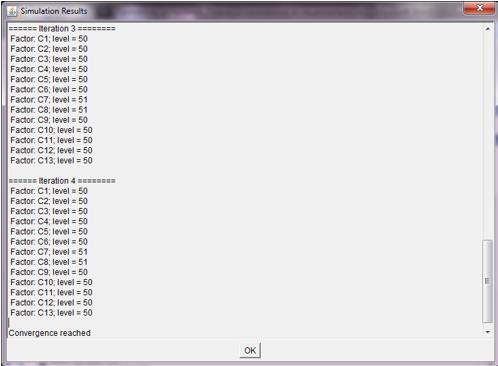
The steady-states of the concepts as a result of simulation are shown in Table 2. The columns correspond to experiment numbers, and the number of iterations is denoted as K.
Below we briefly recap the results according to experiment numbers.
- The average values of concept levels and link intensities have been selected. The simulation result in almost no changes and finished quickly.
- Government actions have good results, and the university has well-trained enrollees (С13), the concept level was set at 100. The majority of dependent concepts took high values that are favorable for the educational institution. Contractual training (С12) takes up the minimum. The labor migration (С11) of the graduates from the region, as well as concepts С9 and С10, remain at some medium level.
- C13 differs from Scenario 2 by a low level (20). In this scenario, a low number of enrollees is expected due to the ongoing COVID-19 pandemic and (or) due to the historically formed demographics. The result is the opposite of Scenario 2: previously high concept levels changed to low, and contractual education (С12) played a crucial role due to the inflow of less trained enrollees.
- The experiment showed no production drop C1, although this representation was simplified and adjusted for both the stagnation and economic growth. The majority of dependent concepts took high values that are favorable for the educational institution. The labor migration (C11) of graduates was reduced to zero.
- The conditions are the same as in Experiment 2, yet the impact intensity of concept С13 on С5 is increased to 100. The overall result is positive, like in Experiment 2.
- The experiment corresponds to the zero implementation of concept C13 and zero impact intensity. The states of dependent concepts remained at the medium level.
- Experiments 7-10 reflect a full factor experiment relative to concepts C1 and C13. Here we reviewed all 4 combinations of extreme levels of independent concepts. Experiment 7 presents a stress test for the production drop C1 at the maximum (100) and concept C13 responsible for student subgroups at zero. The effects include the highest increase in labor migration C11 and the reduction of other concepts.
- The simulation of an optimistic scenario: production drop С1 is at 0, and the number of potential enrollees C13 is at 100. Other concepts are highly developed, apart from labor migration.
- The simulation of the erratic impact of external factors: production drop against a sufficient number of enrollees. As a result, the high quality of enrollee training coexists with the high labor migration of graduates and low levels of other concepts.
- The situation is the opposite of Experiment 9. The quality of enrollee training is reduced, as well as the acquisition of competencies by the graduates. Other concepts, apart from labor migration, are at high or medium levels.
- Low enrollee training quality C6 (30) resulted in the maximizing of contractual learning C12 and the reduction of some important concepts responsible for the quality of learning.
- This option differs from Experiment 1 because the impact intensity of concept C13 for enrollee training level C6 is zero. The simulation result in almost no changes and finished quickly.
- This option differs from Experiment 12 because the impact intensity of concept C13 for enrollee training level C6 was set at 100. No changes occurred as well.
- The simulation of a 100-strong production drop if the initial concept levels were high (80) except contractual education C12 (50). The levels of the majority of the dependent concept dropped.
- Experiments 15-17 were designed to identify the influence of enrollee training level C6 when all other concepts are at medium levels.
Enrollee training level C6 equals 20. The maximum level of contractual education C12 is achieved. The majority of concepts are normalized against low values, including C6.
- When C6 equals 50, Experiment 1 is repeated, and no changes occur.
- When С6 equals 80, the majority of the concepts crucial for the education systems grow.
Conclusion
Experiments 1-4, 7-10 are interesting to test the model adequacy, and we found no contradictions with the basic representations of the domain area. The comparison of Experiments 5 and 6 shows that the impact of C13 on the system is significant in the model suggested. The greatest graduate competence levels C8 were obtained provided that there were enough enrollees С13 (Experiments 2, 5, 8). The demand for contractual education does not disappear even in the most favorable scenarios (Experiment 8). The low quality of enrollees’ previous training in this model is not compensated by the links to other concepts (Experiment 11). The comparison of Experiments 12 and 13 showed that the favorable situation with enrollees C13 in this system cannot produce the desired positive outcome unless it is complemented with positive changes in the economy, the emergence of the demand for knowledge, and enrollee motivation. Experiment 14 shows that the system degrades during economic downturns. The degradation of the system represented by the drop in the high initial levels of the majority of concepts occurs during a prolonged economic recession (within the simulation interval). Experiments 15-17 show that the level of enrollee training C6 impacts the system rather than depends on it, which is one of the improvement areas for the fuzzy cognitive map structure. If we take graduate employment implicitly characterized by concept C11 as a key criterion for the efficiency of a higher education institution, the value of production drop С1 (Experiments 4, 8, 10) becomes crucial. If we focus on graduate training quality C8, it can be achieved when a good selection of potential enrollees С13 is available. In any case, the construction of transient process scenarios and the assessment of the future state of system elements may provide additional information for the strategic management of a higher education institution and promotes the increased survivability of the organization.
References
Aspuru, G. O. (2017). Fuzzy Cognitive Maps. Retrieved on 07 June 2022, from http://www.ochoadeaspuru.com/fuzcogmap/index.php
Banerjee, G. (2009). Fuzzy Cognitive Maps for Identifying Critical Path in Strategic Domains. Defence Science Journal, 59(2), 152-161. DOI:
Chrysafiadi, K., & Virvou, M. (2013). A knowledge representation approach using fuzzy cognitive maps for better navigation support in an adaptive learning system. Springerplus, 2(1), 81. DOI:
Cole, J. R., & Persichitte, K. A. (1999). Fuzzy cognitive mapping: Applications in education. International Journal of Intelligent Systems, 15(1), 1-25. DOI: 10.1002/(SICI)1098-111X(200001)15:1%3C1::AID-INT1%3E3.0.CO;2-V
Coronavirus disease (COVID-19) travel advice. (2021). Retrieved on 03 June 2022, from https://www.who.int/emergencies/diseases/novel-coronavirus-2019/travel-advice
Devisscher, T., Boyd, E., & Malhi, Y. (2016). Anticipating future risk in social-ecological systems using fuzzy cognitive mapping: thecase of wildfire in the Chiquitania. Ecology and Society, 21(4), 18. DOI:
Digital economy and society statistics - enterprises - Statistics Explained. (2021). Retrieved on 03 June, 2022 from https://ec.europa.eu/eurostat/statistics-explained/index.php
Efremkina, I. N. (2018). Issledovanie dinamiki kar'ernyh orientacij, uchebnoj motivacii, ih vzaimosvyazi u studentov-bakalavrov kak usloviya formirovaniya gotovnosti k professional'noj mobil'nosti [The study of the dynamics of career orientations, educational motivation, their interrelations among bachelor students as the conditions for forming readiness for professional mobility]. Perspektivy Nauki i Obrazovania, 32(2), 175-180. https://psejournal.files.wordpress.com/2018/07/1802pno.pdf
Know-Why.Net (2019). We See the Interconnections. International platform for sharing models from different fields. http://www.know-why.net
Kosko, B. (1993). Fuzzy Thinking: The New Science of Fuzzy Logic. Hyperion Press; First Edition. https://www.amazon.com/Fuzzy-Thinking-New-Science-Logic/dp/B00BG2MYR8
Micheev, M. Yu., Prokofiev, O. V., & Savochkin, A. E. (2018). Fuzzy Cognitive Map of Pre-Emergency Prediction. Fuzzy technologies in the industry - FTI 2018 Proceedings of the II International Scientific and Practical Conference, CEUR Workshop Proceedings, 2258, 502-509. http://ceur-ws.org/Vol-2258/paper59.pdf
Neocleous, C., Schizas, C., & Yenethlis, C. (2016). Fuzzy cognitive models in studying political dynamics: The case of the Cyprus problem. https://www.researchgate.net/publication/228808958_Fuzzy_cognitive_models_in_studying_political_dynamics_The_case_of_the_Cyprus_problem
Osoba, O. A., & Kosko, B. (2017). Fuzzy cognitive maps of public support for insurgency and terrorism. Journal of Defense Modeling and Simulation: Applications, Methodology, Technology, 14(1), 17-32. DOI: 10.1177%2F1548512916680779
Papageorgiou, E. I., Papandrianos, N., Karagianni, G., Kyriazopoulos, G., & Sfyras, D. (2009). Fuzzy Cognitive Map Based Approach for Assessing Pulmonary Infections. Lecture Notes in Computer Science book series, 5722, 109-118. https://link.springer.com/chapter/
Pashchenko, D. V., Sinev, M., Trokoz, D., Sineva, M., Tokarev, A., & Dubravin, A. (2016). Construction features of procedural simulator for objective control expert systems management devices of rocket and space technology ground infrastructure objects. MATEC Web of Conferences, 44, 01010. DOI:
Pressley, M., & McCormick, C. (1995). Advanced educational psychology for educators, researchers, and policymakers. HarperCollins College Publishers. https://www.worldcat.org/title/advanced-educational-psychology-for-educators-researchers-and-policymakers/oclc/30738902
Sporn, B. (1999). Higher Education Policy Book 54. Jessica Kingsley Publishers.
Stylios, C. D., & Peter, P. (1999). Proceedings of the 7th Mediterranean Conference on Control and Automation (MED99), 2251-2261.
Vagaeva, O. A., Liksina, E. V, Galimullina, N. M., Sergeeva, S. V., & Lyusev, V. N. (2021). Distance Learning—Challenges and New Opportunities Under COVID-19 Pandemic. Smart Innovation, Systems and Technologies, 227, 1081-1091. DOI:
Copyright information
This work is licensed under a Creative Commons Attribution-NonCommercial-NoDerivatives 4.0 International License.
About this article
Publication Date
29 August 2022
Article Doi
eBook ISBN
978-1-80296-126-3
Publisher
European Publisher
Volume
127
Print ISBN (optional)
-
Edition Number
1st Edition
Pages
1-496
Subjects
Economics, social trends, sustainability, modern society, behavioural sciences, education
Cite this article as:
Prokofiev, O. V., & Savochkin, A. E. (2022). Development Scenarios And Modeling For System Of Engineering Education At Universities. In I. Kovalev, & A. Voroshilova (Eds.), Economic and Social Trends for Sustainability of Modern Society (ICEST-III 2022), vol 127. European Proceedings of Social and Behavioural Sciences (pp. 121-131). European Publisher. https://doi.org/10.15405/epsbs.2022.08.14