Abstract
The Colombo Metropolitan Region is the economic powerhouse of the whole country, with vigorous commercial and industrial activities. Over the past few decades, its low-lying areas have been undergoing drastic changes. All factors relating to this have been considered by studying three sectorial models and an overall model. In the sectorial models, several variables
Keywords: Geo-StatisticGIShousingsettlementsuburbanurban planning
Introduction
It is acknowledged by town and city planners that the utilization and conversion of land are primarily influenced by the factors of time, space and human interactions. For example, it can be seen that civil works such as concrete highways, asphalt roads, factories, industrial sites and housing developments are densely scattered across environmentally sensitive low-lying lands including marshes and paddy fields that have been filled in. Colombo and its suburbs have now become heavily built-up areas with a preponderance of individual housing units occupying practically all the low-lying areas (Samaratunga & O'Hare, 2013). As mentioned earlier, this has been an on-going process that has eventually resulted in the takeover of every available plot in the low-lying areas and its gradual transformation into a stable homestead. The conversion of land occurs very slowly at first but the process gains momentum later on until every vacant plot of land is acquired and occupied. This uncontrolled growth ultimately leads to urban chaos on several fronts (Dayaratne, 2010).
Classification of Houses in Low-lying Areas
Classification of houses in low-lying areas can be a difficult task. Especially, the demarcation of the land plot areas can only be done by using public participatory practices in the field. In this research, setting up land plots with GIS database was found to be very time consuming. This is the reason most research has been done using cell or grid based analysis rather than digitized land plot analysis. The geo-spatial database together with the household information was used to establish whether houses were stable or non-stable.
Problem Statement
Changes to low-lying areas that occurred over the period under review can be made visible as hot spots across the entire area covered by the CMR using spatial techniques. One of the tasks of the study was to find out, “Where are the low-lying areas that are still being threatened by the emergence of new households?” Therefore, a comprehensive spatial database was prepared to show how the low-lying areas have changed in the recent past due to the emergence of a large number of housing units. This had to be accomplished using the satellite information system and GIS software after carefully geo-referencing the locations of households. By having this type of information system, anyone could see where the low-lying areas have been gradually encroached upon and captured over time. But this high-tech satellite based GIS system can represent only pixel based information (Samat, 2007), which by itself is insufficient for statistical analysis. Variations among the housing units and the effect of human interactions are also crucial factors and this information can only be collected by conducting door to door surveys. But combining socio-economic and human behavioural information together with geo-spatial data can prove to be a difficult exercise.
Research Questions
Hence, this study has addressed mainly four aspects (one task and three objectives) of unauthorized conversion of land as stated in the research questions and objectives. In addition to the questions posed in this study, the reliability of the model is examined by using a separate dataset in the same area as the validation model. Furthermore, this study suggested that it is necessary to reiterate the procedure once in every seven years to see the updated conversion situation.
With respect to evolution of housing units emerging over a period of time, which are the most affected areas?
What are the conditions that prevail in the households in low-lying areas?
Identify the important human influences and geo-spatial conditions involved in the conversion of low-lying areas?
What is the rate at which the land is being transformed?
Purpose of the Study
The phenomenon of individual households arising in the low-lying localities of a metropolis is not unique to Sri Lanka but common to nearly all tropical countries (Makunde, 2016). Despite the presence of various administrative arms of the government to supervise and regulate all on-going land reclamation and building work, these agencies have not proved effective at preventing the proliferation of unauthorised households in the suburbs. This problem is particularly acute in developing countries, and the situation in the Colombo Metropolitan Region is a good example. The changes take place slowly at the beginning but gradually accelerate and after the passage of some years end up causing a myriad problems in the built-up areas. Despite the importance of the issue and the need to address it urgently, no proper study has been conducted on the negative impact caused by private housing units in the low-lying areas. The effects of this phenomenon and the consequences on both the natural environment and the urban environment have not been studied and analysed adequately. The purpose of this study is to focus on and assess the changes that occur in low-lying areas during the conversion process with special emphasis being placed on the following three aspects:
Socio-economic
Behavioural
Geo-spatial
In addition to above, overall conditions are also tested through modelling techniques to identify the most influential variables. Hence, the following contribution to knowledge and to society has been made by this study.
Research Methods
Dependent variable of entire logistic model is the housing type and that is categorically coded as ‘0 = non-stable house’ and ‘1 = stable house’. Sample size is 294 householders living in 185 stable houses and 109 non-stable houses as of May, 2012 in the low-lying areas of Kerawalapitiya GN division, Wattala, which obtained the highest ranking as the fastest developing area. The model is built up of 19 independent variables. It is divided into three categories covering socio-economic variables, behaviour variables, and geo-spatial variables (Liao & Wei, 2014). Finally, all three sub-models were tested together to identify the most influential variables within the overall model.
i) Socio-economic variables (Model 1) 8 independent variables
ii) Behaviour variables (Model 2) 7 independent variables
iii) Geo-spatial variables (Model 3) 5 independent variables
iv) Overall model variables (Model 4) 19 independent variables
‘Family savings’ was used as one of the behaviour variables at the group level, but it was excluded from the overall model because of multicollinearity with the variable ‘family income’ at the overall level (Smith & Sasaki, 1979; Zainodin, Noraini, & Yap, 2011). Combinations of household income, family savings and family expenditure have high VIF value when they are tested in the collinearity statistic test. If multicollinearity is high, the better solution is to remove the relevant independent variable from the model (Chong & Jun, 2005).
Model Building
The process of selecting subsets out of a larger number of variables is called model building. An important objective of model building in logistic regression is prediction (Lee & Koval, 1997). In respect of low-lying areas, transformation of the land due to emergence of households and the variables responsible for same has been modelled. Houses act as convertors of low-lying areas and so ‘House’ is the dependent variable. In a particular housing plot, the conversion process will depend upon several factors but housing condition will be the main indicator of how this particular housing plot will act in the near future in the context of conversion. Therefore, the dependent variable has only two aspects: first, how stable houses will act or have acted in the context of conversion, and secondly, how non-stable houses will act or have acted in the context of conversion of their particular land plots. Thus, this model can be represented in terms of a multivariate logistic regression equation as given below: (1)
Where p is the probability that the dependent variable (Y) is 1, p (1-p) is the odds ratio, X is the intercept, and Y are coefficients that measure the contribution of independent factors B to the variations in Y.
To appropriately interpret the meaning of Eq. (1), it is necessary to use the coefficients as a power to the natural log (e), which represents the odds (or likelihood) ratio. Table
Findings
One of the major objectives of the research was to quantify the influence of factors that were primarily due to human intervention. In the case of low-lying land conversion, three aspects of human interaction together with their overall effect have been discussed. In addition, results for all models were linked to actual locations on terrestrial maps using GIS techniques.
According to the summarized results presented in Table
Another important aspect of the overall model is that three of the behaviour variables, three geo-spatial variables, and two socio-economic variables proved highly significant. Hence, behaviour variables and geo-spatial variables have proved to be a strong part of the model, and that is very much in agreement with the research objectives and second hypothesis of the study. Upon analysing the sectorial model, the behaviour model shows excellent performance as five variables out of the seven independent variables prove significant as seen in the results of Table
According to Table
Family Income is also well supportive of the model but its strength (odds ratio) is not very high in comparison to the behaviour variables. PPP has an odds ratio of 8.009 and TSA has odds ratio of 5.438 in the overall model but family income has only 1.182 odds ratio. Geo-spatial variables GWS, PPG and RWR are significant in both stages. RWR is also a highly significant variable that has a negative relationship with stable house. If rain water remains stagnant in a particular land plot for several days, then that household is not likely to become a home garden. This is borne out by the result that shows it has an odds ratio of less than 1. Hence, if a house has fewer days of rain water remaining in its particular land plot then that house is more likely to undergo conversion into a stable homestead. Job skill is a significant variable in the overall model, though it is not significant at group level; this result illustrates the importance of overall model testing.
Variations among the Land Plots
Third objective of the research is to quantify the variations in conversion using models. Hence, probability maps of the models in Figure
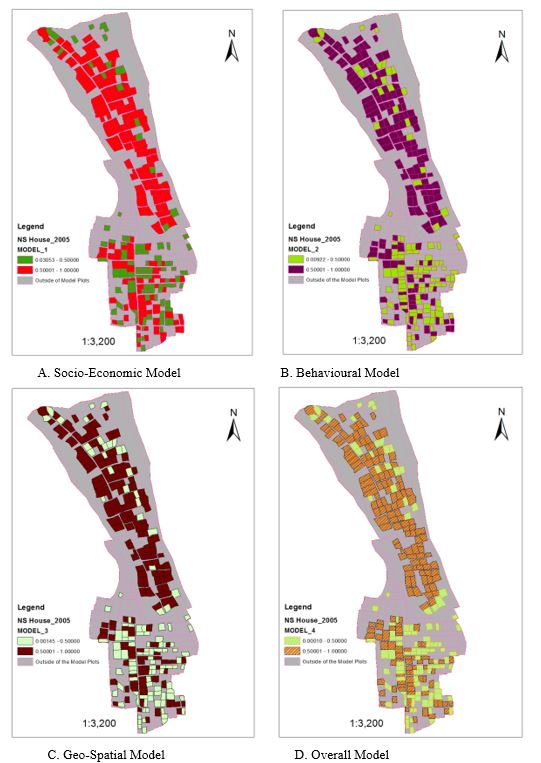
Discussion
To measure the factors behind low-lying land conversion in future studies, it would be helpful to know the accuracy of this model. It is clear this study has improved the spatial logistic model accuracy to 92.2% for the overall model. It can be seen that the accuracy levels of similar studies conducted earlier, for both model and validation have been somewhat below the model accuracy obtained in this study. Therefore, the high accuracy attained by this model can contribute much to future studies in the same study area or other study areas facing similar land conversion issues. By applying the same methods followed in this study, specifically by building a more comprehensive database and by adopting a more analytical approach, very reliable results can be obtained. Therefore, it can be said that this study could make a solid contribution towards solving many urban development and environment related problems caused by mass migration of the populace from rural to urban areas.
Conclusion
In the sectorial models, the important variables
Spatial logistic model results have been linked with the spatial database of GIS map so that it indicates the areas converted by establishment of households as well as the non-converted areas. Hence, this classification has been done based on spatial logistic model results. Urban Planning authorities can distinguish between the converted and non-converted areas and even the assessment values of the individual land plots. Therefore, this model can be used as a geo-spatial management system for low lands under threat in other places as well. Thus, the contribution made by this study will be very useful for local government bodies and environmental authorities when they are planning for sustainable development of their urban low lands (Table
In this study, all influential factors have been identified and grouped into three key sectors, based on the relevant literature review; the variables in the key categories were then investigated in detail. In the past, several studies devoted to land conversion in low-lying areas have addressed only socio-economic factors. But as a result of the sectorial level analysis conducted by this study, it has been proven that geo-spatial factors (89.5%) and behaviour factors (86.7%) are more influential than the socio-economic factors (81.3%). Therefore, it is now clear that geo-spatial factors and behaviour factors carry at least as much weight as socio-economic factors. Compared to previous studies that did not address all of the above factors in the conversion of low-lying areas, the additional sectorial information that has been included in this study will prove very useful in managing conversion of land and related activities and contribute substantially to future urban planning in low-lying areas.
Acknowledgments
Authors acknowledge the School of Humanities, Universiti Sains Malaysia for the waiver of registration fees for INCoH 2017.
References
- Arbuckle, J. L. (2010). IBM SPSS Amos 19 user’s guide. Crawfordville. FL:Amos Development Corporation .
- Chong, I. G., & Jun, C. H. (2005). Performance of some variable selection methods when multicollinearity is present. Chemometrics and Intelligent Laboratory Systems, 78(1), 103-112. https://dx.doi:10.1016/j.chemolab.2004.12.011
- Dayaratne, R. (2010). Moderating urbanization and managing growth: How can Colombo prevent the emerging chaos? Working paper//World Institute for Development Economics Research. Retrieved from: http://hdl.handle.net/10419/54061
- Dong, J. J., Tung, Y. H., Chen, C. C., Liao, J. J., & Pan, Y. W. (2011). Logistic regression model for predicting the failure probability of a landslide dam. Engineering Geology, 117(1), 52-61. https://dx.doi:
- Lee, K. I., & Koval, J. J. (1997) Determination of the best significance level in forward stepwise logistic regression. Communications in Statistics-Simulation and Computation 26, 559-575.
- Liao, F. H., & Wei, Y. D. (2014) Modeling determinants of urban growth in Dongguan, China: a spatial logistic approach. Stochastic environmental research and risk assessment 28, 801-816. https://doi:
- Makunde, G. (2016) Challenges in urban development control and housing provision: a case of Epworth, Chitungwiza and Harare, Zimbabwe. International Journal of Technology and Management 1, 1-12.
- Samat, N. (2007). Integrating GIS and celular automata spatial model in evaluating urban growth: prospects and challenges. Universiti Teknologi Malaysia, Faculty of Built Environment.
- Samaratunga, T. C., & O'Hare, D. (2013) ‘Sahaspura’: the first high-rise housing project for low-income people in Colombo, Sri Lanka. Australian Planner, 51(3), 230-231.
- Smith, K. W., & Sasaki, M. S. (1979). Decreasing Multicollinearity A Method for Models with Multiplicative Functions. Sociological Methods & Research, 8(1), 35-56.
- Vasconcelos, D. M. J .P, Silva, S., Tome, M., Alvim, M., & Pereira, J. M. C. (2001). Spatial prediction of fire ignition probabilities: comparing logistic regression and neural networks. Photogrammetric Engineering and Remote Sensing, 67(1), 73-81.
- Xie, C., Huang, B., Claramunt, C., & Chandramouli, C. (2005). Spatial logistic regression and gis to model rural-urban land conversion. Paper presented at the Proceedings of PROCESSUS Second International Colloquium on the Behavioural Foundations of Integrated Land-use and Transportation Models: Frameworks, Models and Applications.
- Zainodin, H. J., Noraini, A., & Yap, S.J. (2011). An alternative multicollinearity approach in solving multiple regression problem. Trends in Applied Sciences Research, 6(11), 1241-1255.
Copyright information
This work is licensed under a Creative Commons Attribution-NonCommercial-NoDerivatives 4.0 International License.
About this article
Publication Date
23 September 2019
Article Doi
eBook ISBN
978-1-80296-067-9
Publisher
Future Academy
Volume
68
Print ISBN (optional)
-
Edition Number
1st Edition
Pages
1-806
Subjects
Sociolinguistics, linguistics, literary theory, political science, political theory
Cite this article as:
Hemakumara*, G., & Rainis, R. (2019). Models Comparison To Assess Emerging Of Low Income Housing In Colombo Suburbs. In N. S. Mat Akhir, J. Sulong, M. A. Wan Harun, S. Muhammad, A. L. Wei Lin, N. F. Low Abdullah, & M. Pourya Asl (Eds.), Role(s) and Relevance of Humanities for Sustainable Development, vol 68. European Proceedings of Social and Behavioural Sciences (pp. 543-553). Future Academy. https://doi.org/10.15405/epsbs.2019.09.60