Effects of Self-Regulation and Cooperation on Technology Acceptance and Teachers’ E-Teaching Competence
Abstract
Based on The Unified Theory of Acceptance and Use of Technology (UTAUT2) model as well as on recently published research findings on the role of self-regulation and collegial cooperation in fostering e-teaching competence, the aim of this quantitative questionnaire study (n = 258) was to examine the influence of two main determinants of self-directed learning - the disposition to self-regulated and to cooperative action - on teachers’ intention to use digital media in the classroom. For this, the mediating role of three technology acceptance factors of the UTAUT model was taken into consideration and analyzed using structural equation modelling (SEM) in order to identify personal conditions conducive to the development of e-teaching skills within a framework of work-integrated learning processes. The results of the SEM path analyzes show a positive effect of metacognitive aspects of self-regulation as well as of teachers’ collegial cooperation on their willingness to adapt to the requirements of digitalization through the use of digital media in education.
Keywords: Collegial cooperation, E-teaching, self-regulation, UTAUT
Introduction
In view of the rapidly developing of the Information communication technologies (ICT) and the related digitalization of education, bringing the professional standards of competence in teaching into line with the digital transformation of society requires the inclusion of both - media-specific and non-specific - competences in the teaching competency profile (European Parlament, 2022). The latter are currently discussed under the terms "21st-century skills" and concern both cognitive areas, such as critical thinking, as well as non-cognitive areas, including the ability to self-regulation and cooperation. These are considered to play an important role for the successful use of ICT in teaching and therefore to contribute significantly to the development of teachers’ technological-pedagogical expertise (McGrath et al., 2011).
Self-directed learning (SDL) in work-integrated settings
Facing the digitalization in education teachers need to develop their professional competency in an action-oriented manner based mainly on the management of complex requirements in new, non-standardized teaching situations. The acquisition and development of e-teaching competence become in this context a work-integrated, self-regulated, informal learning process that goes beyond the framework of formal professional development training. The maine characteristic of this process is providing a learning and competence-promoting working arrangement in which self-learning architectures can emerge (Dehnbostel, 2019). These are learning settings based on self-directed learning (SDL) with the following characteristics: skill orientation through problem-solving after confrontation with complex challenges, cooperation through expertise sharing, reflection and evaluation of the learning experience (Müller, 2021; Zimmerman & Lebeau, 2000). Thus, in the digitalization era, self-regulation and collegial support are considered to be essential features of work-integrated learning.
The concept of self-regulation and its contribution to SDL
One of the main characteristics of SDL is that it brings with it specific requests to the learner, involving a range of personal dispositions, which allow for the proper recognition and use of decision-making and action freedom in the learning process (Dyrna, 2021). These dispositions are associated with self-regulation that empowers the individual to regulate and control all mental processes involved in learning (Sembill et al., 2007). Theoretical concepts of self-regulation derived from empirical research so far allow its definition as an internal process, whereby the learner plans, implements and evaluates his own learning. This process encompasses metacognition as a way to predict, monitor, control and evaluate cognitive processes as well as motivation, which mainly refers to the individual's ability to self-motivate for learning success (e.g. Hong & O'Neil, 2001; Zimmerman, 2001). The ability to make plans and monitor their own actions (i.e. metacognition) as well as the ability to maintain effort during learning (i.e. motivation) are main characteristics of self-regulated learners. There are several recent studies indicating the importance of self-regulation in e-teaching, showing the importance of personal dispositions like perceived ease of use and self-confidence in the handling of digital tools for the development of e-teaching skills (Lucas et al., 2021) and a positive influence of self-efficacy in basic ICT and of online collaboration on the use of digital tools in schools (Hatlevik, 2016). A recent study by Chen and Jang (2019), in which the influence of self-regulation on teachers‘ pedagogical-technological competency was investigated, indicated that metacognitive components of self-regulation correlated higher with content knowledge and pedagogical content knowledge but lower with technological knowledge and technological-pedagogical-content expertise. These leaves open the question of whether and which components of self-regulation are relevant for the acquisition of technology-related teaching skills.
The role of collegial cooperation in SDL
Collaborative learning experiences that enable group members to interact together and learn with and from each other are mainly involved in the good functioning of SDL in work-integrated learning settings (Schrode et al., 2021). Recent studies showed that group cooperation improves problem-solving and self-directed learning abilities (Kim & Kim, 2019; Wang et al., 2021). With regard to the influence of the teachers’ collegial cooperation on the use of ICT in the classroom, the findings so far point to the fact that teamwork can bring up further development of competence in e-teaching. By working together in a team, teachers can better cope with digitalization-related professional challenges through an increased ability to act and solve problems (Drossel et al., 2017). In order to bring more evidence for the role of the group as a critical source for modelling and scaffolding of the process by which teachers can better direct their own learning in the field of e-education, further research is necessary in this area.
Technology acceptance as a precondition for developing competence in e-teaching
Understanding what influences teachers’ intention to engage in e-teaching is necessary for any attempt to improve their pedagogical-technological skills. Therefore, personal dispositions like self-regulation and the ability to engage in collegial cooperation should be considered in the effort to identify those factors that influence technology acceptance in the classroom.
Technology Acceptance Models are used to measure the behavioural intention of continued use of ICT in several domains, including education. The Unified Theory of Acceptance and Use of Technology (UTAUT) is an integrated theoretical model to explain the acceptance to use ICT (Venkatesh et al., 2003) by extending the Technology Acceptance Model (TAM) proposed by Davis (1989). Similar to the TAM, UTAUT is based on the basic assumption that personal expectations regarding the usefulness (i.e. performance expectancy) and the ease of use (i.e. effort expectancy) of a system have a direct influence on someone's intention to use it. Additional to these, in the UTAUT model the social influence, meaning the degree to which the use of technology is believed to be relevant as a result of social interactions with important others, also directly influences the behavioural intention. The model explains up to 70% of the variance in technology use behavior and therefore represents a robust and reliable instrument that can be used to gain a deeper understanding in the field of technology acceptance (Vekantesh, 2021). Previous studies have shown that UTAUT can be used to predict learners’ behaviour intention in online courses (e.g. Chen & Hwang, 2019; Hsu, 2012). However, there are very few studies in which the UTAUT model has been used to measure teachers’ technology acceptance. Tappe (2019) examined UTAUT factors that influence the teachers‘ intention to use ICT in the classroom. The results showed that the behavioral intention is mainly dependent on a positive attitude towards technology and an increased self-efficacy with regard to digital tools. Therefore, there is a need for follow-up research to better identify those relevant conditions for the technology use in the classroom.
Problem Statement
In order for teachers to be able to meet the digital transformation challenges in education, they have to permanently adapt their teaching methods by taking into consideration the didactically use of ICT. They do this best by properly using technology in their classroom and learning from experience, i.e. in a self-directed work-integrated learning context. In view of the current state of research on SDL and e-teaching, there is a need to better understand the relationship between individual differences concerning self-regulation and collegial cooperation, as SDL components, and technology acceptance. This understanding is necessary to identify individual mechanisms that positively influence teachers‘ pedagogical-technological expertise in a SDL setting, which should be taken into account when designing teacher training programs.
Research Questions
The present study is intended to make an empirical contribution to determining the effects of self-regulation and collegial cooperation on technology acceptance and teachers’ willingness to use ICT in the classroom. It is assumed that the intention to integrate technology into teaching depends on its acceptance (Tappe, 2019), which in turn is influenced by the self-assessed individual expression of self-regulation (Chen & Hwang, 2019) and the degree of appreciation of collegial cooperation with regard to its relevance for one's teaching (Drossel et al., 2017).
The main questions of the investigation are:
Is there a relation between teachers’ self-regulation of and their intention to use ICT in the classroom?
Is there a relation between teachers’ cooperation and their intention to use ICT in the classroom?
Do the UTAUT acceptance factors (performance expectancy, effort expectancy, and social influence) have an effect on the relation between self-regulation, respectively collegial cooperation, and the intention to use ICT in the classroom?
Based on the study of Chen and Hwang (2019), the Hong and O’Neil’s theory is considered in the present study to differentiate between the metacognitive and motivational dimension of self-regulation, whereas collegial cooperation is regarded as an one-dimensional factor (Rothland, 2009). Regarding the technology acceptance, Venkatesh et al.’s UTAUT model is used including the four dimensions of performance expectancy, effort expectancy, social influence and behavioural intention. It is assumed that the behavioural intention for technology use is influenced by self-regulation and collegial cooperation, and these effects are mediated by the expectancy and the social influence. Accordingly, the hypotheses for this study are given as follows:
H1: Teachers’ metacognition positively relates to the behavioral intention to use digital media in the classroom.
H2: Teachers’ motivation positively relates to the intention to use digital media in the classroom.
H3: Teachers’ metacognition positively relates to performance expectancy.
H4: Teachers’ metacognition positively relates to effort expectancy.
H5: Teachers’ metacognition positively relates to social influence.
H6: Teachers’ motivation positively relates to performance expectancy.
H7: Teachers’ motivation positively relates to effort expectancy.
H8: Teachers’ motivation positively relates to social influence.
H9: Teachers’ cooperation positively relates to the intention to use digital media in the classroom.
H10: Teachers’ cooperation positively relates to performance expectancy.
H11: Teachers’ cooperation positively relates to effort expectancy.
H12: Teachers’ cooperation positively relates to social influence.
H13: Teachers’ performance expectancy positively relates to the behavioral intention to use digital tools in the classroom.
H14: Teachers’ effort expectancy positively relates to the behavioral intention to use digital media for educational purposes in the classroom.
H15: Teachers’ social influence positively relates to the behavioral intention to use digital media for educational purposes in the classroom.
The hypothesized models are illustrated in Figure 1 and 2, which illustrates the structural relations between the components of self-regulation, respectively the collegial cooperation and the UTAUT.
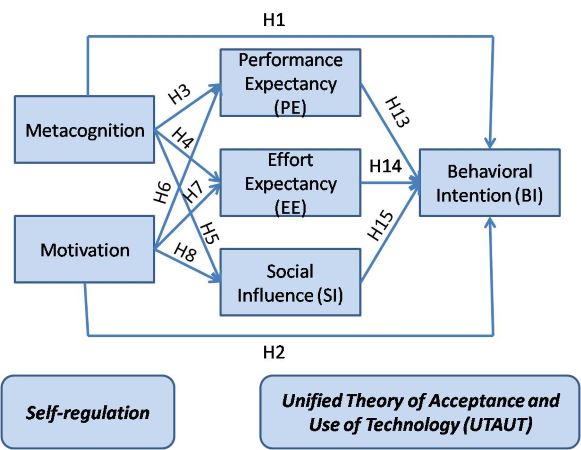
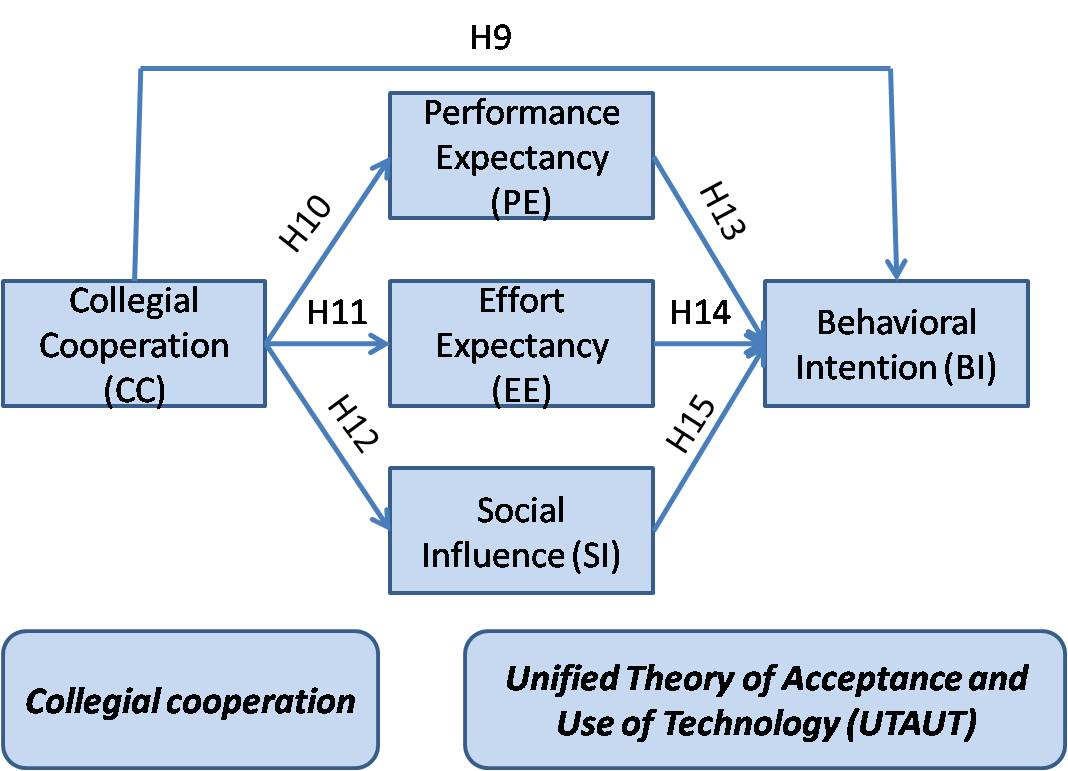
Purpose of the Study
The purpose of this study is to investigate the influence of self-regulation and collegial cooperation in a work-integrated learning setting on teachers‘ technology acceptance and their willingness to use digital tools in the classroom. The study emphasizes the personal factors in self-directed learning, meaning that it focuses more on individual dispositions in order to explore the relationships between self-regulation as well as collegial cooperation and technology acceptance. Taking these variables into consideration plays an important role for the development of training offers that build up e-competency in teaching. Therefore, the design of this study aimes to contribute towards a better understanding of the way they relate to each other.
Research Methods
Measuring tools
The measuring tool was a questionnaire from prior studies which was revised to consider the work-integrated, self-directed and cooperative learning situation in the present study. It contained self-reported questions organized in four sections. First, demographic related data on teachers’ background information regarding gender, age, teaching position, teachers’ professional development level and digital experience were collected. Second, the teachers’ self-regulation in terms of metacognition and motivation were measured using a short version of the original Short Self-Regulation Questionnaire (SSRQ) with 22 items und five constructs: goal attainment (GA), mindfulness (MF), adjustment (AD), proactivness (PA) and goal setting (CS) (Chen & Lin, 2018). For this, the items were translated from English to German and renumbered; the translation was verified by two bilingual volunteers and the translated questionnaire was pilot tested on a sample of 36 students of the Pedagogical Highschool in Sibiu, Romania. Third, the collegial cooperation was assessed using a 10-item scale from a standardized German questionnaire for measuring teachers’ social-communicative competences (Rothland, 2009). Finally, the teachers’ performance expectancy, effort expectancy, social influence and behavioural intention were measured with a validated German translation of the UTAUT (Harborth & Pape, 2018). A five-point Likert scale ranging from “strongly disagree” to “strongly agree” was used to measure all of the items, with exception of those for the demographic data.
Target group
The target group of this study were teachers aged between 18 and 65 from all public schools with German teaching in Romania. The invitation to participate in the study was made by the Romanian Center for Teacher Training in German Language (www.zfl.ro). The data collection took place over two weeks using an online survey tool. All the study participants participated voluntary and were assured of the confidentiality of their information. A total of 416 questionnaires were submitted. After the removal of all questionnaires with more than 5 missing values, 258 data sets remained, which were included in the descriptive statistical evaluation. The sample is described in Table 1, showing good sample representativeness. The large difference between male and female responders can be attributed to the significantly higher proportion of female teachers in Romanian schools (INSSE, 2020).
GA = goal attainment, MI = mindfulness, GS = goal setting, CC = collegial cooperation; PE = performance expectancy, EE = effort expectancy, SI = social influence; BI = behavioral intention.
Data analysis
SPSS (version 27) was used for the descriptive statistics, item analysis, reliability and validity analysis. The Structural Equation Modelling (SEM) with AMOS (version 25) was applied to analyse the goodness of fit of the research model by measuring direct and indirect effects between the investigated variables in multivariate mediation setting.
In the preliminary data analysis, there was a filtering of the outliers on the basis of the Mahalanobis distance, with a 228 (88.4%) remaining total number of data sets. Because of the non-normal distribution, the data was subjected to bootstrapping using the Bca method with a number of 5000 bootstrapping samples and 95% confidence intervals.
The reliability of the scales was proved by calculating the Cronbach’s alpha to check for their internal consistency. Construct validity, which refers to the degree to which multiple items measure the same construct, was assessed based on the item-total correlation. A confirmatory factor analysis (CFA) using SEM was performed to test whether the data fit the hypothesized measurement models. The chi-square test and other goodness-of-fit indices (i.e. CFI, RMSEA) were used to evaluate the adequacy of the hypothesized models.
A path analysis using SEM was performed to assess the pattern of relations among the model variables as formulated in the 15 hypotheses. For this, the assumed covariance between the variables were presented as path diagrams to show the causal effects of the independent variables on the mediators and the dependent variable.
Findings
CFA analyses for self-regulation and collegial cooperation
The first step in data analysing, was to conduct a CFA for the research models measuring the self-regulation and the collegial cooperation. Given the existing construct validation for the German translation of the UTAUT scales by Harborth and Pape (2018), only Cronbach’s α was measured in this case, the value of 0.85 showing a good internal consistency.
The validation strategy related to the number of latent variables (constructs), the assignment of the measurement variables to the constructs, and the relationships between the constructs. In order to confirm the construct validity, the factor loadings should be significant and exceed 0.5, and the average variance extracted (AVE) by each construct should also exceed 0.5. The overall fitness of the models was evaluated by the following indicators: the chi-square and Bollen-Stine test, the comparative fit index (CFI) and the root-mean-square error of approximation (RMSEA). For a good model fit, the chi-square values normalized by degrees of freedom (chi-square/degrees of freedom) should not exceed 3, CFI should exceed 0.9, and RMSEA should not exceed 0.08. Furthermore, the Bollen-Stine test should not be significant.
The initial CFA showed that the measurement models for both constructs were a poor fit. With regard to the self-regulation scale, this was due to the high correlations between three of the five self-regulation constructs (r > 0.90) in the model: goal achievement (GA), adjustment (AD) and proactivness (PA) and also to the fact that the goal setting/GS construct contained only two items. Therefore, an exploratory factor analysis (EFA) was performed in order to better uncover the underlying construct structure. After EFA analysis the 5-dimensional structure of the self-regulation scale was reduced to three dimensions, respectively goal achievement (GA), mindfulness (MF) and goal setting (GS). As shown in Table 2, all three constructs had a strong internal reliability and no item was excluded because of a low item-total correlation (< 0.30).
For the collegial cooperation scale, the Cronbach’s α for all 10 items was less than 0.7 because of the items 5 and 10 whose item-total correlation was below 0.3. After excluding both items, Cronbach’s α improved to 0.88 and the remaining 8 items showed an item-total correlation above 0.7. The AVE was 0.50.
The CFA for the new estimated models for self-regulation and collegial cooperation showed the following results: the ratio of chi-square to degrees of freedom was 1.65, respectively 1.97, CFI = 0.95, respectively 0.99, and RMSEA = 0.05, respectively 0.06. These data indicate an acceptable model fit in both cases, showing good construct validity of the measuring scales.
Path analyses
Two structural equation models (SEM) of the hypothesized relationships between self-regulation or collegial cooperation and technology acceptance were tested using path analysis. The overall fit indices for both research models were acceptable as the results fell within the commonly accepted values (see Table 3).
The SEM path analysis results for testing each hypothesis are presented in Figure 3. The results for the self-regulation model indicate that, regarding to metacognition, Goal attainment has a significant influence on performance expectancy, effort expectancy, and social influence (path coefficient = 0.52, 0.54, 0.35, respectively). Although, there were no significant effects for Goal setting, these findings support the hypotheses H3, H4, and H5, showing that metacognitive aspects of self-regulation have a positive influence on the adaptation of teaching behaviour to the requirements of digitalization. However, the motivational factor Mindfulness has no significant impact on any technology acceptance factors, which can be referred to the fact that similar to Goal setting all the items measuring Mindfulness were reversed. This can trigger a potential different understanding of the reversed items compared to the regular ones.
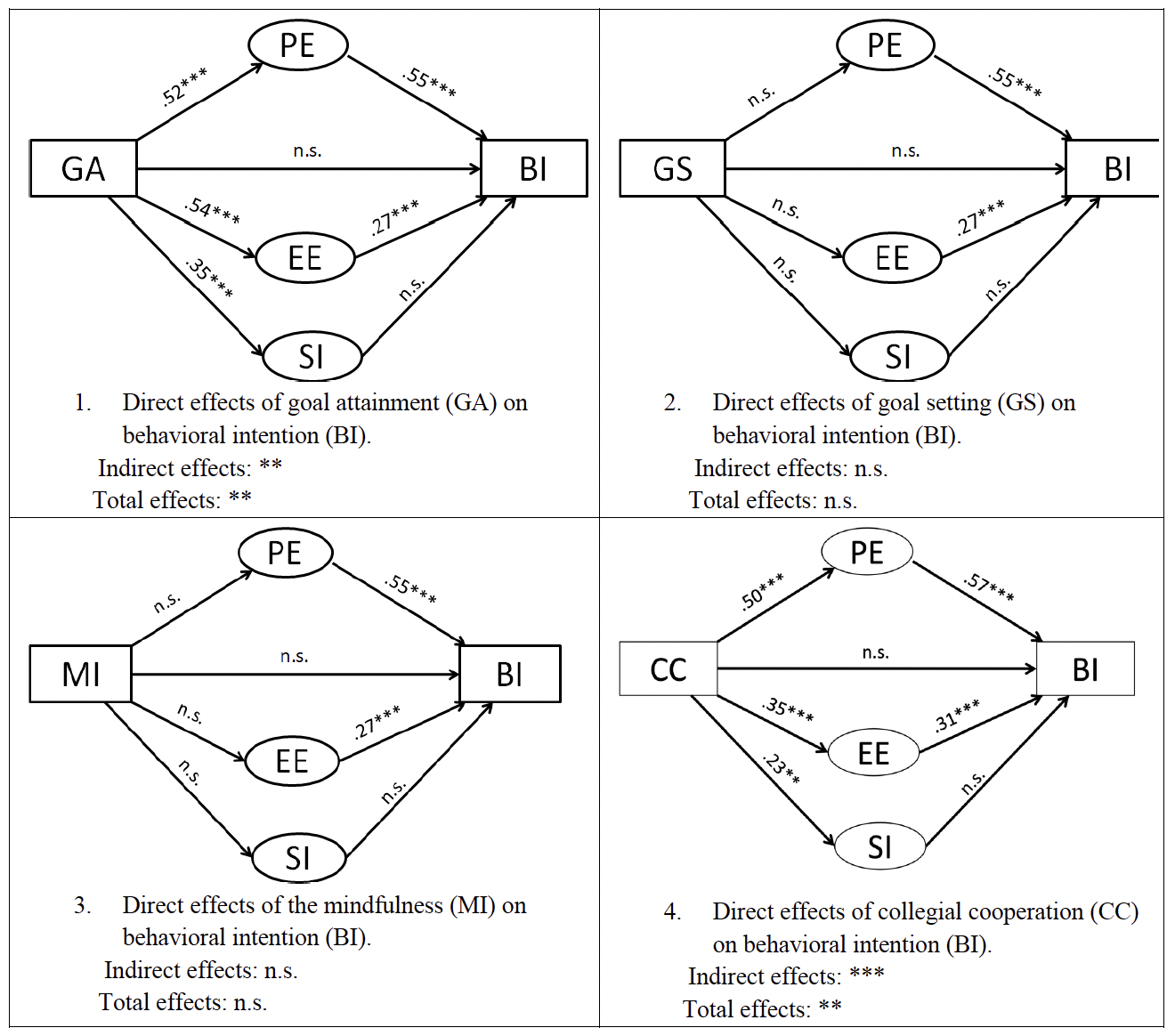
The results for Collegial cooperation indicate a significant effect on performance expectancy, effort expectancy, social influence (path coefficient = 0.50, 0.35, 0.23, respectively) verifying hypotheses H10, H11, and H12. This indicates a positive relation between a highly appreciated professional exchange o the basis of collegial cooperation and teachers’ expectancy towards the use of ICT in the classroom.
Both Performance expectancy and Effort expectancy are significantly associated with behavioural intention in both models (path coefficient = 0.55/0.37, 0.57/0.31 respectively), confirming hypotheses H13 and H14. However, Social influence has a non-significant impact on behavioural intention. This means that the degree to which a teacher believes that using ICT in the classroom is useful for the achievement of the teaching goals and is easy to manage will significantly influence the degree to which he or she intends to use technology in the future for didactic purposes.
In summary, Goal attainment as part of the metacognitive self-regulation positively relates to performance expectancy, effort expectancy and social influence and show indirect effects on behavioural intention through performance expectancy and effort expectancy, which are strong mediators in this relation. The same was shown for collegial cooperation. However, there were no significant direct positive effects of the metacognitive or motivational self-regulation factors as well as the collegial cooperation and social influence on the behavioural intention. This may be due to the limitations of this study regarding cultural and design aspects of the scales used.
Conclusions
This study was designed to identify those internal factors that support self-directed, work-integrated learning processes for building competence in e-teaching. By this, this work contributes to the literature on the determinants of teacher’s behavioural intentions to use ICT in the classroom. We assumed that self-regulated and cooperative teachers are more reliant on their ability to learn, mastering their tasks accordingly to their learning goals (Zimmerman, 2001), also being able to effectively share good practices in an informal, on-the-job learning setting (Drossel et al., 2017). By this, they will have positive expectations with regard to the use of technology in learning and a better adaptation to the digitalization in education (Chen & Hwang, 2019).
Based on the study results, there is a mediated influence of metacognitive factors of self-regulation on the behavioural intention to use ICT in the classroom. Therefore, a high ability to monitor one's progress towards goal achievement and to search for appropriate problem solutions on its own initiative strenghtens the willingness to learn and teach with ICT by rising the technology acceptance. The same result pattern has been shown for the collegial cooperation. Teacher cooperation can thus be regarded as an important variable of influence in relation to use of innovative technology-based teaching strategies.
Consequently, if we want to promote the teachers’ ability to use ICT for didactic purposes, in order to increase their competence in e-teaching, we should take into account personal dispositions for self-regulated and cooperative action which influence the expectations regarding the usefulness and operability of a digital system to be integrated in the classroom and by this, the behavioural intention to use it. Therefore, teachers’ professionalization in the area of pedagogical-technological expertise requires the designing of learning programs that take into account complex interrelated effects, some of them yet to be clarified. Future studies should consider the dependency of the self-regulation construct on cultural dimensions and may examine the effect of different cooperation levels on the development of e-competence in teaching.
References
Chen, P. Y., & Hwang, G. J. (2019). An empirical examination of the effect of self-regulation and the Unified Theory of Acceptance and Use of Technology (UTAUT) factors on the online learning behavioral intention of college students. Asia Pacific Journal of Education, 39(1), 79-95.
Chen, Y-H., & Jang, S.-J. (2019). Exploring the Relationship Between Self-Regulation and TPACK of Taiwanese Secondary In-Service Teachers. Journal of Educational Computing Research, 57(4), 978-1002. https://doi.org/10.1177%2F0735633118769442
Chen, Y-H., & Lin, Y. J. (2018). Validation of the Short Self-Regulation Questionnaire for Taiwanese College Students (TSSRQ). Frontiers in Psychology. 9, 259.
Davis, F. (1989). Perceived usefulness, perceived ease of use, and user acceptance of information technology. MIS Quarterly, 13(3), 475-487. https://www.jstor.org/stable/249008
Dehnbostel, P. (2019). Betriebliche Lernorte, Lernräume und Selbstlernarchitekturen in der digitalisierten Arbeitswelt. [Company learning locations, learning spaces and self-learning architectures in digitized work settings]. Magazin erwachsenenbildung.at, 35/36. Wien.
Drossel, K., Eickelmann, B., & Gerick, J. (2017). Predictors of teachers’ use of ICT in school – the relevance of school characteristics, teachers’ attitudes and teacher collaboration. Education and Information Technologies, 22(2), 551–573.
Dyrna, J. (2021). Selbstgesteuertes Lernen – Begriffsbestimmung und Operationalisierung. In J. Dyrna, J. Riedel, S., Schulze-Achatz, & T., Köhler (Eds.), Selbstgesteuertes Lernen in der beruflichen Weiterbildung. Ein Handbuch für Theorie und Praxis (pp. 65-79). Waxmann.
European Parlament. (2022). Digital Agenda for Europe. https://www.europarl.europa.eu/ftu/pdf/en/FTU_2.4.3.pdf
Harborth, D., & Pape, S. (2018). German Translation of the Unified Theory of Acceptance and Use of Technology 2 (UTAUT2) Questionnaire (March 23, 2018). https://ssrn.com/abstract=3147708
Hatlevik, E. O. (2016). Examining the Relationship between Teachers’ Self-Efficacy, their Digital Competence, Strategies to Evaluate Information, and use of ICT at School. Scandinavian Journal of Educational Research, 61(5), 555-567.
Hong, E., & O'Neil Jr, H. F. (2001). Construct validation of a trait self‐regulation model. International journal of psychology, 36(3), 186-194.
Hsu, H. H. (2012). The acceptance of Moodle: An empirical study based on UTAUT. Creative Education, 3, 44. DOI:
INSSE. (2020). Sistemul educational in Romania. https://insse.ro/cms/sites/default/files/field/publicatii/sistemul_educational_in_romania_2018_2019_0.pdf
Kim, M. G., & Kim, H. W. (2019). The Effects of Jigsaw Cooperation Learning on Communication Ability, Problem Solving Ability, Critical Thinking Disposition, Self-directed Learning Ability and Cooperation of Nursing Students. The Journal of Korean Academic Society of Nursing Education, 25(4), 508-516.
Lucas, M., Bem-Haja, P., Siddiq, F., Moreira, A., & Redecker, C. (2021). The relation between in-service teachers' digital competence and personal and contextual factors: What matters most? Computers & Education. 160, 104052.
McGrath, J., Karabas, G., & Willis, J. (2011). From TPACK concept to TPACK practice: An analysis of the suitability and usefulness of the concept as a guide in the real world of teacher development. International Journal of Technology in Teaching and Learning, 7(1).
Müller, H. (2021). Selbstgesteuertes Lernen und Fähigkeiten – ein symbiotisches Konzeptpaar [Self-directed learning and skills - a symbiotic pair of concepts]. In J. Dyrna, J. Riedel, S., Schulze-Achatz, & T., Köhler (Eds.), Selbstgesteuertes Lernen in der beruflichen Weiterbildung. Ein Handbuch für Theorie und Praxis (pp. 332-345). Waxmann.
Rothland, M. (2009). Kooperation und kollegiale Unterstützung im Lehrerberuf [Cooperation and collegial support in teaching]. Lehrerbildung auf dem Prüfstand, 2009, 2(2), 282-303.
Schrode, N., Horn, K., & Burger, B. (2021). Eine Didaktik für selbstorganisiertes Lernen: Erwachsene(n)lernen in Selbstlernarchitekturen [Didactics for self-organized learning: Adults learn in self-learning architectures]. In J. Dyrna, J. Riedel, S., Schulze-Achatz, & T., Köhler (Eds.), Selbstgesteuertes Lernen in der beruflichen Weiterbildung. Ein Handbuch für Theorie und Praxis (pp. 144-162). Waxmann.
Sembill, D., Wuttke, E., Seifried, J., Egloffstein, M., & Rausch, A. (2007). Selbstorganisiertes Lernen in der beruflichen Bildung – Abgrenzungen, Befunde und Konsequenzen [Self-organized learning in vocational training - delimitations, findings and consequences]. Berufs- und Wirtschaftspädagogik, 13, 1–33. http://nbn-resolving.de/urn:nbn:de:bsz:352-opus-68217
Tappe, E.-H. (2019). Predictors of intention for the didactic use of digital media in teaching - conversion of the Unified Theory of Acceptance and Use of Technology (UTAUT) into a school examination setting. In T. Knaus (Ed.), Forschungswerkstatt Medienpädagogik III (pp. 999-1027). Munich: copaed.
Vekantesh, V. (2021). Adoption and use of AI tools: a research agenda grounded in UTAUT. Annals of Operations Research, 308(1), 641-652.
Venkatesh, V., Morris, M. G., Davis, G. B., & Davis, F. D. (2003). User acceptance of information technology: Toward a unified view. MIS Quarterly, 27(3), 425-478.
Wang, Y., Ma, J., Gu, Y., Wang, J., Chen, C., Zhang, Y., & Wang, R. (2021). How does group cooperation help improve self-directed learning ability in nursing students? Nurse Education Today, 98, 104750.
Zimmerman, B. J. (2001). Theories of Self-Regulated Learning and Academic Achievement: An Overview and Analysis. In B. J. Zimmerman, & D. H. Schunk (Eds.), Self-Regulated Learning and Academic Achievement: Theoretical Perspectives (pp. 1-65). Lawrence Erlbaum Associates.
Zimmerman, B. J., & Lebeau, R. B. (2000). A commentary on self-directed learning. In D. H. Evenson, & C. E. Hmelo (Eds.), Problem-based learning: A research perspective on learning interactions (pp 299-313). Routledge.
Copyright information
This work is licensed under a Creative Commons Attribution-NonCommercial-NoDerivatives 4.0 International License.
About this article
Publication Date
10 April 2023
Article Doi
eBook ISBN
978-1-80296-961-0
Publisher
European Publisher
Volume
5
Print ISBN (optional)
-
Edition Number
1st Edition
Pages
1-1463
Subjects
Education sciences, teacher education, curriculum development, educational policies and management
Cite this article as:
Zoppelt, D., Hermann, A., Crețulescu, R., Wild, R., & Elsholz, U. (2023). Effects of Self-Regulation and Cooperation on Technology Acceptance and Teachers’ E-Teaching Competence. In E. Soare, & C. Langa (Eds.), Education Facing Contemporary World Issues - EDU WORLD 2022, vol 5. European Proceedings of Educational Sciences (pp. 334-346). European Publisher. https://doi.org/10.15405/epes.23045.35