Abstract
Keywords: Dynamic relationship, nonrenewable energy, renewable energy, electricity generation, production function, Malaysia
Introduction
One of the key concerns being researched by numerous scholars throughout the world is energy sustainability. It refers to a type of energy that may be constantly used and meets the current need for energy without endangering its life or causing it to become depleted. However, given that it doesn't affect the environment, it should be increasingly emphasized (Bekhet & Harun, 2017; Chen & Lin, 2020; Du et al., 2021; Kardooni et al., 2018; Zafar et al., 2018). Thus, energy components are highlighted to stress on this issue. It is categorized as nonrenewable and renewable energy. Energy's importance in the process of a nation's development and industrialisation, as well as in boosting economic activity, is widely acknowledged. Furthermore, the primary driver of energy demand for economic growth is the usage of electricity. It shows that consuming energy is now more commonly viewed as a fundamental right than as a societal necessity (Oh et al., 2018). Given the rising worldwide need for power, the trend is anticipated to continue in the coming years.
However, in March 2020, world faced COVID-19 pandemic and affected many lives globally. The global COVID-19 epidemic has had an impact on the economy, including in Malaysia. It demonstrated that the GDP performance for 2020 decreased by 5.6% compared to a positive rise of 4.3% in 2019, which was impacted by the deterioration of all economic sectors including energy industries (Jiang et al., 2021). In markets where strong confinement is used, the demand for electricity has decreased by about 15% in nations under partial and total lockdown (IEA, 2021). Thus, Figure 1 shows the growth rate of the Malaysian GDP from 1980 to 2020 at 5.9 percent, which has been steadily rising over time. The favourable trends of Malaysia's energy generation (EG) and electricity consumption (EC) are still stabilising and indicated at 7.5 percent and 7.8 percent, respectively, despite the economic activities dealing with the COVID-19 pandemic problem (see Figure 1). It shows that the continuing rise in electricity demand still made it necessary to consider alternative methods of generating electricity.
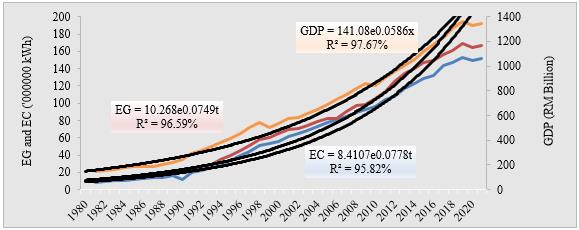
Additionally, Malaysia's primary source of electricity generation is nonrenewable energy, which results in significant air pollution and greenhouse gas (GHG) emissions. The primary sources of emissions are transportation —vehicles— and waste—municipal solid waste that is disposed of in landfills; and energy—electricity use. As a result, Malaysia aspires to achieve zero net GHG emissions by the year 2050 with the following strategy planning (MGTC, 2022): 1) Increasing human capability; 2) promoting green research; 3) enhancing communities through green interactions; 4) facilitating green partnerships; and 5) laying forth a green road map. The government's commitment for environmental preservation offers the opportunity to use renewable energy for efficient electricity generation in Malaysia.
Problem statement
Utilizing renewable energy sources to their full potential for Malaysia's electricity generation, therefore, the government wants to increase labour productivity and generate more possibilities for highly qualified people. As a result, by 2025, Green Technology Master Plan (MGTC) seeks to partner with 2,000 green industry businesses that will create 10,000 new jobs. Additionally, the Ministry of Environment and Water (KASA) hopes to create over 200,000 green jobs by 2030 (New Straits Times, 2017). The International Labour Organization (ILO) defines a "green job" as work that supports environmental preservation and protection, whether it be in a traditional industry like manufacturing or construction, or in a green industry like energy efficiency and renewable energy.
In the fourth quarter of 2020, the unemployment rate increased by 4.8% as a result of the COVID-19 pandemic's effects on economic activity. Additionally, more persons now meet the criteria for underemployment than in 2019. Due to the indirect effects of this scenario, the gross national income (GNI) per capita decreased from RM45,212 in 2019 to RM42,531 in 2020. This scenario has an effect on discretionary income, indicating a slowdown. In the interim, the government's movement restrictions caused domestic consumer spending to decline (DOSM, 2021).
Additionally, innovation investment is the most crucial method for promoting renewable energy for power generation in Malaysia as it enters a new phase of industrialization. These investments in innovation boost the efficiency of capital in the accomplishment of its particular duties. They relate to new technological knowledge that develops over time. Then, through distinctive goods and services that meet customer needs, innovation investments can generate new revenue streams for the electrical sector. The adoption of technical change is focused with identifying the most economically viable energy sources and provides greater potential for economies of scale because Malaysia has been in the new industrialization and structural change stage. In order to better understand the dynamic long-term link between electricity generation and its factors in Malaysia, this study will evaluate that relationship.
Research questions
Accordingly, this study highlighted the research question as follow: Is there a dynamic long-term relationship between electricity generation performance and its factors in Malaysia?
Purpose of the study
The goal of this study is to examine the dynamic long-term relationships between electricity generation performance and its factors in Malaysia in order to respond to the aforementioned question. To accomplish the goal of this study, the design of this paper is as follows: a literature review in Section 2, research methodologies in Section 3, findings in Section 4, conclusions, and policy implications in Section 5.
Literature Review
The subject of sustainable energy and environmental protection has recently been the focus of substantial economic and energy production studies. The dynamic relationship between nonrenewable and renewable energy and Malaysia's electricity generation performance is one area of energy that has drawn a lot of attention. A brief comparison of the findings across different nations and historical periods is provided in order to better grasp the review of prior studies. The literature has also thoroughly investigated the study on the relationship between energy use and economic growth (Chandran et al., 2010; Jamil & Ahmad, 2010; Hamdi et al., 2014; Karanfil & Li, 2015; Khatun & Ahamad, 2015; Lorde et al., 2010; Rafindadi & Ozturk, 2017; Wolde-Rufael, 2014).
Furthermore, most of the previous studies employed the autoregressive distributed lag (ARDL) model to examine the dynamic long-run relationship between electricity consumption or electricity generation and economic growth in various countries (Atems & Hotaling, 2018; Bekhet & Harun, 2017; Chen & Fang, 2018; Dogan, 2015; Dogan, 2016; Hamdi et al., 2014; Lean & Smyth, 2010; Lorde et al., 2010; Shahbaz et al., 2014; Islam et al., 2013; Sekantsi & Thamae, 2016; Shahbaz et al., 2017; Shahbaz & Lean, 2012). Their discussion validated this relationship between economic growth and electricity consumption/generation. At the same time, some of them applied other determinants such as capital, labor, nonrenewable and renewable energy, financial development, export, and price to highlight the role of these variables in their studies. Yet, the nonrenewable and renewable energy variables receive little attention, especially in terms of the dynamic relationship between electricity generation and economic growth (Harun & Bekhet, 2020). In Italy and Tunisia, the study applied nonrenewable and renewable energy as the determinants; however, these variables are added to analyze their role in environmental pollution (Bekhet & Othman, 2018; Bento & Moutinho, 2016; Chien, 2022; Destek & Aslan, 2017; Jebli & Youssef, 2015; Romano et al., 2017; Sharmin et al., 2022). On the other hand, a study in the Baltic region applied both of these variables as the determinants of electricity consumption (Furuoka, 2017).
As a result, this study differs from earlier studies for a number of reasons. First, as a proxy for electricity, this study emphasises electricity generation rather than electricity consumption (Altintas & Kum, 2013; Dogan, 2015; Ghosh, 2009; Lean & Smyth, 2010; Sarker & Alam, 2010; Yoo & Kim, 2006). In accordance with the most recent state-of-the-art research, this study argues that GDP should be a factor of production function in the same manner that capital and labour are (Bekhet & Harun, 2017; Harun & Bekhet, 2020), and electricity generation should be a dependent variable. Second, several recent studies discussed this energy and classified it by sources (renewable and nonrenewable energy consumption) in the AC-D production function in order to highlight energy consumption as one of the significant factors of production (Begum et al., 2015; Dogan, 2015, 2016; Dogan & Ozturk, 2017; Hamdi et al., 2014; Harun & Bekhet, 2020; Jebli & Youssef, 2015; Omri et al., 2015; Shahbaz et al., 2015). Examining and separating each source's impact on economic activity is the goal (Apergis & Payne, 2010, 2013; Kahia et al., 2017).
In addition, research has been done on the potential for solar energy in Vietnam and Iran (Roy et al., 2022), as well as on wind and solar energy (Mostafaeipour et al., 2022).
Therefore, this study adds to the debate about the dynamic long-run relationship between nonrenewable and renewable energy on the performance of electricity generation, with an emphasis on Malaysia. To accomplish the goal of this study and to give more chance for drawing policy implications that are directly relevant to Malaysia, the augmented production function is specifically employed.
Research Methodology
This study examines the dynamic long-term relationships in order to assess the factors that affect the generation of electricity in Malaysia. In addition to its operations, the electrical sector must increasingly adapt to changing environmental conditions as the world's weather patterns and climate continue to change. Because time series data can offer a better study of the factors influencing Malaysia's power generation, time series data of these variables have been gathered for this purpose. Data from the Energy Commission of Malaysia (ST), World Bank, Department of Statistic, Malaysia (DOSM), and World in Data have therefore been gathered for 39 years between 1982 and 2020. Table 1 provides a summary of the information's sources and data.
Accordingly, it is applied to perform time series by using natural log-linear functional form of Augmented Cobb-Douglas production function as in Equation (1).
(1)
Where ln means for the natural logarithm of each of the following: technology (A), capital (K), labour (L), GDP (Y), nonrenewable energy (NE), and renewable energy (RE). The production elasticities of these variables are shown by the notation φjs (j=K, L, Y, NE, and RE). Additionally, it displays the positive or negative sign of production determinants that indicate the favourable or unfavourable impact on EG, εt denotes the stochastic error components that are considered to be regularly distributed together with white noise (Bento & Moutinho, 2016), and t stands for the annual time-series data.
The F-Bound test is thereafter used to evaluate the dynamic long-run relationship between the variables. Compared to the other conventional procedures, it provides a lot of benefits (Boutabba, 2014; Matar & Bekhet, 2015). First off, it enables a mixture of I(0) and I(1) variables in the result of the stationary test. However, the F-Bounds test cannot be used with I(2) (Sufyanullah et al., 2022). Second, with small samples, 30 ≤ t ≤ 80, it works better. Thirdly, it enables multiple optimal lags for the variables. Fourthly, the ARDL model addresses the endogeneity issues with the Engle-Granger technique, assuming that all the variables are endogenous (Al-mulali et al., 2015). As a result, Equation (2) might be used to evaluate the dynamic long-run relationship.
(2)
Where φ0 is a constant value, φ1 elasticity of EGt-1 and φis (i = 2,3,4,5 and 6) are elasticity of Kt-1, Lt-1, Yt-1, NEt-1 and REt-1, respectively. Then, the null and alternative hypotheses are established as follows. H0: φi=0 (no dynamic long-run relationship exists) and H1: φi≠0 (the dynamic long-run relationship exists). The F-statistic of Pesaran et al. (2001) is used to analyse the presence of dynamic long-run links among the variables regardless of whether the variables are stationary at I(0) or I(1), therefore, this joint significance of the coefficients of lagged variables is examined. In order to do this, Pesaran et al. (2001) estimated two asymptotic critical values: one when the variables are supposed to be lower critical bounds, I(0), and another when the variables are assumed to be upper critical bounds, I(1).
If the computed F-statistic is greater than I(1), the null hypothesis of no cointegration is rejected. In other words, there is proof of a significant dynamic long-run relationship. On the other hand, if the calculated F-statistic is lower than I(0), the null hypothesis of no cointegration is not successfully rejected. However, if the I(0) < F-statistic < I(1), the result is uncertain or cannot be accurately concluded. As a result, the outcome in this instance could be related to previous studies that determined whether dynamic long-run relationships existed or not. Alternatively, certain studies have suggested that the error correction term derived from the normalised cointegrating equation will be a useful way to establish dynamic long-run relationships (Boutabba, 2014; Ivy-Yap & Bekhet, 2016; Matar & Bekhet, 2015).
Findings
Descriptive statistics
Descriptive statistics were calculated and are shown in Table 2 in order to assess the normality of the EG, K, L, GDP, NE, and RE data. Since all data values fall within the permitted range of the data, the mean value of each of the six variables demonstrates that none of the data for any of these variables contains an outlier. The standard deviation for each variable also shows that none of the data for any variable exhibits excessive or unacceptable volatility. As a result, the data's mean value and allowable variance serve as evidence that all of the variables' data are sufficient. Skewness's result, which demonstrates that all variables are larger than -1 but less than +1, establishes the validity of the present data is normal.
The statistics of kurtosis, which should fall within the range of 1-3 for a normal data, have further supported the normality of the current time series data. It demonstrates that the kurtosis value does not exceed 3. It is further demonstrated that the existing data is typical in this way. Finally, the likelihood of Jarque-Bera is examined to see whether the data are normally distributed. The Jarque-Bera test is a goodness of fit test used in statistics to examine if sample data show the skewness and kurtosis of a normal distribution. It is demonstrated by various descriptive statistics that all of the study's variables are normal and in good enough shape to be examined further.
Unit root analysis
Augmented Dickey Fuller (ADF) and Phillips Perron (PP) unit root tests have been used on series results to evaluate the stationarity of variables. It is clear that all variables were stationary at the first discrepancy between the two tests' results (see Table 3).
Source: Output of the Eviews Package Version 12.0.
As a result, the stationarity of the variables is established, and the F-Bound test requirement that the series be I(0) or I(1) is satisfied. The appropriate lag order is chosen to be employed in the F-Bound test with respect to the lag order after the stationarity of the data has been verified. Different lag order estimating methods, including as LR, FPE, AIC, SIC, and HQ, have been utilized for this purpose. However, Akaike information criterion (AIC) has been used to determine the proper lag order since it offers more reliable and consistent information and results than other criteria. The result shows the appropriate of lag order is 3 (see Table 4).
Source: Output of the Eviews Package version 12.0.
Dynamic long-run relationship
In this current data, the F-Bound test is applied to identify the dynamic long-run relationship between the variables. It is determined to reject the null hypothesis and draw the conclusion that there is a substantial dynamic long-run relationship in the given data because the F-statistics value is 10.04, which is unquestionably bigger than 5.26, i.e., the upper bound value (see Table 5).
Source: Output of the Eviews Package version 12.0.
Accordingly, the elasticity is examined with their significance and t-statistics of all production determinants towards the Malaysia electricity generation performance. Thus, Table 6 highlights the result of elasticity of determinants towards the electricity generation in the long-run. It shows that L has a negative and elastic long-run elasticity on EG at 10% significance value. It means that one percent increase in L is significantly associated with the decrease of 2.26% in EG. However, GDP has shown a positive and elastic long-run elasticity on EG by 1.79% at 5% significance level. While, the elasticity of K, NE and RE is not significant on EG in the long run. Nevertheless, there is a positive inelastic for K, NE and RE in the long-run towards Malaysia electricity generation performance. Additionally, the outcome of the diagnostic analysis shows that the error term has a normal distribution and is unaffected by serial correlation or heteroscedasticity issues. A model's functional form is clearly defined. These outcomes have demonstrated the validity of the long-run findings. Additionally, it can be demonstrated that the model is comparatively stable over time (see Figure 2).
statistics = 3713 (0.00); Jarque-Bera = 1.44 (0.49); ARCH = 1.89 (0.18); Serial correlation = 7.31 (0.29)
Ramsey RESET test = 2.07 (0.16)
Notes: * and ** as defined in Table 3
Source: Output of the Eviews Package version 12.0.
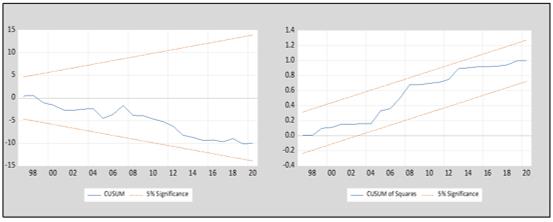
Conclusions and Policy Implications
This study analyzes the dynamic long-run relationship among the variables (EG, K, L, GDP, NE, RE) in Malaysia by collecting time series data of 39 years from 1982 to 2020. The secondary data collected from databases of ST, World Bank, DOSM and World in Data. The F-Bound test is applied to analyze the dynamic relationship between the variables. Also, the elasticity of each variables is examined towards the electricity generation. The result confirms that the distribution of data variables is normal. Furthermore, electricity generation, capital, labour, GDP, nonrenewable and renewable energy stationary at I(1) at 5% significance level. Then, the F-Bound test is performed to analyzed the dynamic long-run elasticity, due to the unique order of integration and small sample (T=39). Also, the suitable lag selection is 3 to determine the long-run elasticity towards the Malaysia electricity generation performance.
The result indicate that labour has negative elastic in the long-run towards electricity generation performance in Malaysia. It shows that the usage of technology is developed due to the less usage on the labour for generating elasticity in Malaysia. Nowadays, Malaysia is the country that developed with the technology change, particularly in manufacturing and electricity sector. In light of past experiences, the greatest difficulties lie in ensuring that technology advancement benefits everyone, especially workers. The ideal outcome of technological development would be higher pay, more job opportunities for graduates, and less reliance on foreign labour (IR4.0). It's crucial to remember that not all technological advancement involves digital devices. Whether labour and technology serve as substitutes or complements in the production process determines how much technological development affects the workforce. Additionally, the sort of economic sector is relevant (New Straits Times, 2017).
On the other hand, the GDP has positive elastic in the long-run elasticity towards electricity generation in Malaysia. It proven that the GDP growth is depends on the performance of economic activities i.e., electricity sector. Nowadays, in order to generate electricity, TNB need other resources i.e. technology advancement to support supplying the energy sources in the electricity generation. Furthermore, Malaysia's Gross Domestic Product (GDP) growth and electricity demand, both continue to increase. It highlights that electrical power in Malaysia is human-dependent energy-in system (Ali et al., 2020; Ali et al., 2022). The study clearly indicates that capital, nonrenewable and renewable energy are less important factors towards electricity generation in Malaysia.
Yet, renewable energy is very less usage for electricity generation in Malaysia. Due to the less awareness from Malaysia community, will delaying the usage of this alternative energy in order to protect out environment. Nonetheless, the renewable energy become one of potential production determinants to compete with the nonrenewable energy i.e., coal. Coal is major source that contribute for generating electricity. In pledges made at the COP26 climate summit, more than 40 nations have agreed to abandon coal. It is important to embrace the financial and environmental advantages of creating a clean energy future.
Acknowledgments
This documented work was funded by Yayasan Cancellor UNITEN (YCU 2022) under project code: 202210007YCU.
References
Afonso, T. L., Marques, A. C., Fuinhas, J. A., & Saldanha, E. M. M. (2018). Interactions between electricity generation sources and economic activity in two Nord Pool systems. Evidence from Estonia and Sweden. Applied Economics, 50(29), 3115-3127.
Akber, M. Z., Thaheem, M. J., & Arshad, H. (2017). Life cycle sustainability assessment of electricity generation in Pakistan: Policy regime for a sustainable energy mix. Energy Policy, 111, 111-126.
Al-mulali, U., Weng-Wai, C., Sheau-Ting, L., & Mohammed, A. H. (2015). Investigating the environmental Kuznets curve (EKC) hypothesis by utilizing the ecological footprint as an indicator of environmental degradation. Ecological Indicators, 48, 315-323.
Ali, S. S. S., Razman, M. R., & Awang, A. (2020). The Nexus of Population, Gdp Growth, Electricity Generation, Electricity Consumption and Carbon Emissions Output In Malaysia. International Journal of Energy Economics and Policy, 10(3), 84-89.
Ali, U., Guo, Q., Kartal, M. T., Nurgazina, Z., Khan, Z. A., & Sharif, A. (2022). The impact of renewable and non-renewable energy consumption on carbon emission intensity in China: Fresh evidence from novel dynamic ARDL simulations. Journal of Environmental Management, 320, 115782.
Altintas, H., & Kum, M. (2013). Multivariate Granger Causality between Electricity Generation, Exports, Prices and Economic Growth in Turkey. International Journal of Energy Economics and Policy, 3, 41-51. https://dergipark.org.tr/en/pub/ijeeep/issue/31907/350762
Apergis, N., & Payne, J. E. (2010). Renewable energy consumption and economic growth: evidence from a panel of OECD countries. Energy Policy, 38(1), 656-660.
Apergis, N., & Payne, J. E. (2012). Renewable and non-renewable energy consumption-growth nexus: Evidence from a panel error correction model. Energy Economics, 34(3), 733-738.
Apergis, N., & Payne, J. E. (2013). Another look at the electricity consumption-growth nexus in South America. Energy Sources, Part B: Economics, Planning, and Policy, 8(2), 171-178.
Atems, B., & Hotaling, C. (2018). The effect of renewable and nonrenewable electricity generation on economic growth. Energy Policy, 112, 111-118.
Begum, R. A., Sohag, K., Abdullah, S. M. S., & Jaafar, M. (2015). CO2 emissions, energy consumption, economic and population growth in Malaysia. Renewable and Sustainable Energy Reviews, 41, 594-601.
Bekhet, H. A., & Harun, N. H. (2012). Analyzing Elasticities and the Relationship Among Construction Production determinants in Malaysia. Asian Forum on Business Education (AFBE 2012): Proceeding, Malaysia-Selangor, UNITEN (pp. 418-429).
Bekhet, H. A., & Harun, N. H. (2017). Elasticity and causality among electricity generation from renewable energy and its determinants in Malaysia. International Journal of Energy Economics and Policy, 7(2), 202-216. https://dergipark.org.tr/en/pub/ijeeep/issue/31921/351197?publisher=http-www-cag-edu-tr-ilhan-ozturk
Bekhet, H. A., & Othman, N. S. (2018). The role of renewable energy to validate dynamic interaction between CO2 emissions and GDP toward sustainable development in Malaysia. Energy Economics, 72, 47-61.
Bélaïd, F., & Youssef, M. (2017). Environmental degradation, renewable and non-renewable electricity consumption, and economic growth: Assessing the evidence from Algeria. Energy Policy, 102, 277-287.
Bento, J. P. C., & Moutinho, V. (2016). CO2 emissions, non-renewable and renewable electricity production, economic growth, and international trade in Italy. Renewable and Sustainable Energy Reviews, 55, 142-155.
Boutabba, M. A. (2014). The impact of financial development, income, energy and trade on carbon emissions: Evidence from the Indian economy. Economic Modelling, 40, 33-41.
Chandran, V. G. R., Sharma, S., & Madhavan, K. (2010). Electricity consumption–growth nexus: the case of Malaysia. Energy Policy, 38(1), 606-612.
Chen, X., & Lin, B. (2020). Assessment of eco-efficiency change considering energy and environment: A study of China’s non-ferrous metals industry. Journal of Cleaner Production, 277, 123388.
Chen, Y., & Fang, Z. (2018). Industrial electricity consumption, human capital investment and economic growth in Chinese cities. Economic Modelling, 69, 205-219.
Chien, F. (2022). How renewable energy and non-renewable energy affect environmental excellence in N-11 economies? Renewable Energy, 196, 526-534.
Destek, M. A., & Aslan, A. (2017). Renewable and non-renewable energy consumption and economic growth in emerging economies: Evidence from bootstrap panel causality. Renewable Energy, 111, 757-763.
Dogan, E. (2015). The relationship between economic growth and electricity consumption from renewable and non-renewable sources: A study of Turkey. Renewable and Sustainable Energy Reviews, 52, 534-546.
Dogan, E. (2016). Analyzing the linkage between renewable and non-renewable energy consumption and economic growth by considering structural break in time-series data. Renewable Energy, 99, 1126-1136.
Dogan, E., & Ozturk, I. (2017). The influence of renewable and non-renewable energy consumption and real income on CO2 emissions in the USA: evidence from structural break tests. Environmental Science and Pollution Research, 24(11), 10846-10854.
Du, W., Li, M., Wang, Y., Ma, X., Hu, C., Zhang, Y., & Zhang, Z. (2021). Dynamic energy efficiency characteristics analysis of a distributed solar photovoltaic direct-drive solar cold storage. Building and Environment, 206, 108324.
Energy Commission, Malaysia (ST). (2022). Malaysia Energy Information Hub (MEIH). Retrieved on 2022, August 4, from https://www.st.gov.my/
Furuoka, F. (2017). Renewable Electricity Consumption and Economic Development: New Findings from the Baltic Countries. Renewable and Sustainable Energy Reviews, 71, 450-463.
Ghosh, S. (2009). Electricity supply, employment and real GDP in India: evidence from cointegration and Granger-causality tests. Energy Policy, 37(8), 2926-2929.
Hamdi, H., Sbia, R., & Shahbaz, M. (2014). The nexus between electricity consumption and economic growth in Bahrain. Economic Modelling, 38, 227-237.
Harun, N. H. B., & Bekhet, H. A. (2020). Elasticity of Non-renewable and Renewable Energy towards Electricity Generation for Sustainable Energy in Malaysia. Global Business & Management Research, 12(4), 176-188. http://www.gbmrjournal.com/vol12no4.htm
Industrial Revolution (IR4.0). Official Bulletin 4th Edition. Retrieved on 8 September, 2022, from https://www.mbot.org.my/MBOT/files/13/136c4e63-8f2f-4d98-9951-69c96316ca46.pdf
Islam, F., Shahbaz, M., Ahmed, A. U., & Alam, M. M. (2013). Financial development and energy consumption nexus in Malaysia: a multivariate time series analysis. Economic Modelling, 30, 435-441.
Ivy-Yap, L. L., & Bekhet, H. A. (2016). Modelling the causal linkages among residential electricity consumption, gross domestic product, price of electricity, price of electric appliances, population and foreign direct investment in Malaysia. International Journal of Energy Technology and Policy, 12(1), 41-59.
Jamil, F., & Ahmad, E. (2010). The relationship between electricity consumption, electricity prices and GDP in Pakistan. Energy Policy, 38(10), 6016-6025.
Jebli, M. B., & Youssef, S. B. (2015). The environmental Kuznets curve, economic growth, renewable and non-renewable energy, and trade in Tunisia. Renewable and Sustainable Energy Reviews, 47, 173-185.
Jiang, A., Cao, Y., Sohail, M. T., Majeed, M. T., & Sohail, S. (2021). Management of green economy in China and India: dynamics of poverty and policy drivers. Environmental Science and Pollution Research, 28(39), 55526-55534.
Kahia, M., Aïssa, M. S. B., & Lanouar, C. (2017). Renewable and non-renewable energy use-economic growth nexus: The case of MENA Net Oil Importing Countries. Renewable and Sustainable Energy Reviews, 71, 127-140.
Kahouli, B. (2017). The short and long run causality relationship among economic growth, energy consumption and financial development: Evidence from South Mediterranean Countries (SMCs). Energy Economics, 68, 19-30.
Karanfil, F., & Li, Y. (2015). Electricity consumption and economic growth: exploring panel-specific differences. Energy Policy, 82, 264-277.
Kardooni, R., Yusoff, S. B., Kari, F. B., & Moeenizadeh, L. (2018). Public opinion on renewable energy technologies and climate change in Peninsular Malaysia. Renewable Energy, 116, 659-668.
Khatun, F., & Ahamad, M. (2015). Foreign direct investment in the energy and power sector in Bangladesh: Implications for economic growth. Renewable and Sustainable Energy Reviews, 52, 1369-1377.
Lean, H. H., & Smyth, R. (2010). Multivariate Granger Causality between Electricity Generation, Exports, Prices and GDP in Malaysia. Energy, 35(9), 3640-3648.
Lorde, T., Waithe, K., & Francis, B. (2010). The importance of electrical energy for economic growth in Barbados. Energy Economics, 32(6), 1411-1420.
Malaysian Green Technology and Climate Change Corporation (MGTC). (2022). Green Jobs Portal. Retrieved on 2022, August 19 from https://www.mgtc.gov.my/what-we-do/green-academy/green-jobs-portal/
Matar, A., & Bekhet, H. A. (2015). Causal Interaction among Electricity Consumption, Financial Development, Exports and Economic Growth in Jordan: Dynamic Simultaneous Equation Models. International Journal of Energy Economics and Policy, 5(4), 955-967. https://dergipark.org.tr/en/pub/ijeeep/issue/31915/351025?publisher=http-www-cag-edu-tr-ilhan-ozturkelectricity
Mostafaeipour, A., Bidokhti, A., Fakhrzad, M. B., Sadegheih, A., & Mehrjerdi, Y. Z. (2022). A new model for the use of renewable electricity to reduce carbon dioxide emissions. Energy, 238, 121602.
New Straits Times. (2017, October 12). GTMP aims for RM180b revenue, 200,000 jobs by 2030, https://www.nst.com.my/business/2017/10/290270/gtmp-aims-rm180b-revenue-200000-jobs-2030
Oh, T. H., Hasanuzzaman, M., Selvaraj, J., Teo, S. C., & Chua, S. C. (2018). Energy policy and alternative energy in Malaysia: Issues and challenges for sustainable growth–An update. Renewable and Sustainable Energy Reviews, 81(2), 3021-3031.
Omri, A., Mabrouk, N. B., & Sassi-Tmar, A. (2015). Modeling the causal linkages between nuclear energy, renewable energy and economic growth in developed and developing countries. Renewable and Sustainable Energy Reviews, 42, 1012-1022.
Our World in Data. (2022). Nonrenewable Energy and Renewable Energy Data. Retrieved at https://ourworldindata.org/renewable-energy
Pesaran, M. H., Shin, Y., & Smith, R. J. (2001). Bounds testing approaches to the analysis of level relationships. Journal of Applied Econometrics, 16(3), 289-326.
Praene, J. P., Radanielina, M. H., Rakotoson, V. R., Andriamamonjy, A. L., Sinama, F., Morau, D., & Rakotondramiarana, H. T. (2017). Electricity generation from renewables in Madagascar: Opportunities and projections. Renewable and Sustainable Energy Reviews, 76, 1066-1079.
Rafindadi, A. A., & Ozturk, I. (2016). Effects of financial development, economic growth and trade on electricity consumption: Evidence from post-Fukushima Japan. Renewable and Sustainable Energy Reviews, 54, 1073-1084.
Rafindadi, A. A., & Ozturk, I. (2017). Impacts of renewable energy consumption on the German economic growth: Evidence from combined cointegration test. Renewable and Sustainable Energy Reviews, 75, 1130-1141.
Raza, S. A., Shahbaz, M., & Nguyen, D. K. (2015). Energy conservation policies, growth and trade performance: Evidence of feedback hypothesis in Pakistan. Energy Policy, 80, 1-10.
Robalino-López, A., Mena-Nieto, Á., García-Ramos, J. E., & Golpe, A. A. (2015). Studying the relationship between economic growth, CO2 emissions, and the environmental Kuznets curve in Venezuela (1980–2025). Renewable and Sustainable Energy Reviews, 41, 602-614.
Romano, A. A., Scandurra, G., Carfora, A., & Fodor, M. (2017). Renewable investments: The impact of green policies in developing and developed countries. Renewable and Sustainable Energy Reviews, 68, 738-747.
Roy, S., Lam, Y. F., Hossain, M. U., & Chan, J. C. L. (2022). Comprehensive evaluation of electricity generation and emission reduction potential in the power sector using renewable alternatives in Vietnam. Renewable and Sustainable Energy Reviews, 157, 112009.
Sarker, M. A. R., & Alam, K. (2010). Nexus between electricity generation and economic growth in Bangladesh. Asian Social Science, 6(12), 16-22.
Sekantsi, L. P., & Thamae, R. I. (2016). Electricity consumption and economic growth in Lesotho. Energy Sources, Part B: Economics, Planning, and Policy, 11(10), 969-973.
Shahbaz, M., & Lean, H. H. (2012). Does financial development increase energy consumption? The role of industrialization and urbanization in Tunisia. Energy Policy, 40, 473-479.
Shahbaz, M., Arouri, M., & Teulon, F. (2014). Short-and long-run relationships between natural gas consumption and economic growth: Evidence from Pakistan. Economic Modelling, 41, 219-226.
Shahbaz, M., Benkraiem, R., Miloudi, A., & Lahiani, A. (2017). Production function with electricity consumption and policy implications in Portugal. Energy Policy, 110, 588-599.
Shahbaz, M., Loganathan, N., Zeshan, M., & Zaman, K. (2015). Does renewable energy consumption add in economic growth? An application of auto-regressive distributed lag model in Pakistan. Renewable and Sustainable Energy Reviews, 44, 576-585.
Shahbaz, M., Zeshan, M., & Afza, T. (2012). Is energy consumption effective to spur economic growth in Pakistan? New evidence from bounds test to level relationships and Granger causality tests. Economic Modelling, 29(6), 2310-2319.
Sharmin, M., Dey, S. R., Ahmed, F., & Tareque, M. (2022). An analysis of energy, environment and economic growth (EEE) nexus: a 2SLS approach. OPEC Energy Review, 46(2), 228-249.
Sufyanullah, K., Ahmad, K. A., & Ali, M. A. S. (2022). Does emission of carbon dioxide is impacted by urbanization? An empirical study of urbanization, energy consumption, economic growth and carbon emissions-Using ARDL bound testing approach. Energy Policy, 164, 112908.
Wolde-Rufael, Y. (2014). Electricity consumption and economic growth in transition countries: A revisit using bootstrap panel Granger causality analysis. Energy Economics, 44, 325-330.
World Bank (2022). Gross Fixed Capital Formation (% of GDP). Retrieved at https://data.worldbank.org/indicator/NE.GDI.FTOT.ZS
Yoo, S. H., & Kim, Y. (2006). Electricity generation and economic growth in Indonesia. Energy, 31(14), 2890-2899.
Zafar, U., Rashid, T. U., Khosa, A. A., Khalil, M. S., & Rahid, M. (2018). An overview of implemented renewable energy policy of Pakistan. Renewable and Sustainable Energy Reviews, 82, 654-665.
Copyright information
This work is licensed under a Creative Commons Attribution-NonCommercial-NoDerivatives 4.0 International License.
About this article
Publication Date
18 August 2023
Article Doi
eBook ISBN
978-1-80296-963-4
Publisher
European Publisher
Volume
1
Print ISBN (optional)
-
Edition Number
1st Edition
Pages
1-1050
Subjects
Multi-disciplinary, Accounting, Finance, Economics, Business Management, Marketing, Entrepreneurship, Social Studies
Cite this article as:
Harun, N. H., & Md. Hashim, S. L. (2023). Dynamic Relationship Between Nonrenewable, Renewable Energy and Electricity Generation in Malaysia. In A. H. Jaaffar, S. Buniamin, N. R. A. Rahman, N. S. Othman, N. Mohammad, S. Kasavan, N. E. A. B. Mohamad, Z. M. Saad, F. A. Ghani, & N. I. N. Redzuan (Eds.), Accelerating Transformation towards Sustainable and Resilient Business: Lessons Learned from the COVID-19 Crisis, vol 1. European Proceedings of Finance and Economics (pp. 353-366). European Publisher. https://doi.org/10.15405/epfe.23081.31