Abstract
The use of autonomous vehicles in megalopolises is of particular interest. However, the optimization of the transport system is complicated by the increase in the costs of resources that ensure transportation. This article investigates the challenges of using self-driving vehicles in a modern economy and explores the advantages of autonomous cars for the passenger transport. In particular, this paper indicates the problems of using new technologies on the basis of Hyundai Sonata cars as well as their benefits and future possibilities. The article touches upon the MatrixNet machine learning method used by Yandex to render users with various services. As a result of the analysis of economic indicators and return on investment, this research assumes the foreseeable outweighing benefits of autonomous cars in the supply of transport services to the public relative to the cars with the taxi driver. These benefits might exist provided respective legal and regulatory framework. Finally, this article puts an emphasis on the reduction in threats to economic security in the transport sector in case of the use of self-driving vehicles.
Keywords: Digital economy, national economy, self-driving cars, autonomous vehicles
Introduction
The near future of the Russian economy depends on its technological development. In a new reality, the socio-economic needs of society dictate the necessity to introduce radical technological models of research and development. In the pursuit of high growth rates, it is essential to promote competitive industries of the economy with the use the intelligent technologies. To this end, special attention is devoted to the digitalization of the transport industry in general, as well as the creation of an intelligent transport system, in particular.
It is evident that modern transport might provide a higher level of services through the implementation of innovative digital technologies, which will require changes in the business model of transport organizations. In this regard, the use of autonomous vehicles in megalopolises is of particular interest. However, the optimization of the transport system is complicated by the increase in the costs of resources that ensure transportation.
Problem Statement
The technical and economic characteristics of autonomous vehicles are investigated all over the world (Hörl et al., 2018). The countries such as the USA, Germany, France, and the United Kingdom start moving towards optimization of the traffic and improvement of the corresponding rules (Hoyle, 2016). Specifically, self-driving cars and electro buses are already tested on some roads of the European Union (Stanley, 2013). This, in turn, begets disruptive innovation in the transport sector which irrevocably changes the role of the car as a subject of ownership, expressed via the rights to own, use and dispose.
Research Questions
Evidently, both rights to use and own a vehicle in the pursuit of gaining income as well as receiving various benefits from its exploitation become more realistic for those previously lacking opportunities to use the car for health reasons or due to inability to spend time in the traffic jams (Collie et al., 2017). In light of the above, such disruptive innovation has brought a change to the right of disposal of the vehicle. On the one hand, owners of the several cars will be able to rent out some of them to generate more income, thereby growing the demand and increasing the capacity of the fleet. On the other hand, the expansion of the car fleet will exert a negative influence on the environment, leading to negative externalities.
A significant reduction in the size of the daily utilized fleet is only possible through the use of autonomous cars, as confirmed by the multi-agent modelling of traffic flows. Therewith, an income increases of the car owner as well as the increase in the number of trips undertaken by the self-driving vehicles will be accompanied by the reduction in fleet. At the same time, the advantages of autonomous cars over the existing vehicles will be expressed not only through the more efficient transport system but also via an improvement in the situation on the roads, not to mention of an increase in the income level of private car owners. An increase in the unemployment rate, the problem of parking, obsolescence of cars, and the restriction of private rights to use them can be attributed to the costs of introducing self-driving cars.
Purpose of the Study
The goal of this research is to determine and analyse the benefits from using self-driving taxis in the conditions of digital economy.
Research Methods
Foreign and domestic scholars and practitioners study the dynamics of traffic and carrying capacities by means of micro- and macro modeling of traffic flows. It is well known fact that the widespread deployment of self-driving cars will increase the distance travelled by these vehicles by 8%. Over the past few years, this observation has given a rise to a great deal of research interest in the problem of introducing autonomous cars. One in particular stands out – the work of van den Berg and Verhoef (2016) investigates a dynamic model that defines the relationship between an increase in the traffic capacity of the road network and the changes in user time based on the example of unmanned vehicles in the United Stated and the Netherlands. The analysis of this model allows to substantiate the acceptable size of subsidies for unmanned vehicles. Subsequently, in their work Llorca et al. (2017) analyzed the dynamics for changing the loads of road network of the Munich agglomeration based on the methods of simulation modeling MATSim. The conclusion was drawn about the direct relationship between the average distance and travel time.
A report by the Organization for Economic Cooperation and Development [OECD] (OECD, 2015) confirms the effectiveness of car sharing (versus personal car use) by analyzing two factors: car usage time (on average, in hours) and utilization rate (person /car). The data presented in the report lead us to the conclusion that a car-sharing is 5-6 times more efficient than a personal car. The carrying capacity is influenced by such parameters as quality of the roads, technical parameters of transport means and driver experience. However, the walking distance of a potential passenger to the nearest vehicle makes car-sharing services unattractive and restrains the demand for them.
As for the impact of unmanned vehicles on the future of a city, it can be assessed based on a number of factors which affect the urban environment, residents, and the social structure.
. The substantive parameter for an evaluation of the transport factor is the number of cars on the street per unit of time, which equally depends on both the capacity of the fleet and the load of the road network. In case of latent demand and the absence of factors constraining the demand for unmanned vehicles, there would be an increase in their number proportional to the growth of the road network. With the reduction in the fleet, there should be a decrease in the number of cars on the city streets per unit of time. These conclusions are drawn based on the Lewis-Mogridge position, according to which the freer the roads, the more often residents tend to use their own cars. In order to assess the traffic situation and the time spent on overcoming it, certain parameters concerning the traffic network load and the traffic flow density were adopted. The evaluation of the need for parking lots includes only qualitative changes in their structure, volume and location.
. The assessment of the costs and damage from road accidents was made based on the data on the CASCO and OSAGO insurances. It is assumed that the ratio of accidents with various degrees of damage and fatalities is constant through time whereas their total number is reduced. The cost of the trip was calculated on the basis of fixed (buying a car, taking into account depreciation period, insurance, parking) and variable (gasoline, maintenance) costs.
The areas vacated from parking lots are planned to be used exclusively for landscaping to improve the environmental situation. Along with it, it is expected to reduce the emission of harmful substances into the atmosphere.
The reduction in the need for taxi drivers will lead to a drop in employment. In addition, it is essential to control the transport activities of unmanned vehicles, develop measures in case of the critical road transport situations and take decisions in case of violations of transport activities.
Findings
Yandex is a web-search engine and the IT Company which provides various products and services in Russia, Common Wealth of Independent states and Turkey. The mechanism of the Yandex services is based on the use of machine learning and prioritization in the search for the necessary information. Moreover, it is used in the demonstration of the preferred service for the consumer as well as in the use of various computer programs. These programs allow translating oral and written texts from one language to another. The machine learning method "MatrixNet", used by Yandex since 2009, is the brainchild of this company. All Yandex services contribute to a better quality of life for their consumers. Yandex allows to buy any current and durable goods without leaving your home, “visit” any online theater production, every corner of the globe, not to mention receive all sorts of services, including getting a taxi, without ordering it in advance while waiting outside.
The idea of unmanned vehicle dates back to the 30s of the XX century. It is associated with the name of General Motors. Despite skeptical attitude of engineers, their brilliant ideas subsequently prompted the technological development of unmanned vehicles and engineering artificial intelligence. Indeed, already in the 50th of the last century, the implementation of these ideas was reflected in the testing of the first "smart" car Firebird II by General Motors. A secret behind car operation was not only in its latest braking system but also in its magnetic fuses designed to interact with an electric cable running under the "smart" road. Nevertheless, the test autopilot was still far from being “smart” in spite of the fact that self-driving cars were controlled by a system regulating the speed on the highway and avoiding emergencies.
The modern self-driving car incorporates all beneficial sides of test unmanned vehicles. In other words, self-driving vehicle is the car that moves without a driver. This car operates through its control unit. In essence, the vehicle is controlled by the special software - the brain of the car, whereas the sensors are the eyes of the car: LIDAR’s (high-precision laser radars), radars, cameras and GPS, to name but a few. The information gathered by sensors is classified according to the level of security for various objects: pedestrians, mobile vehicles, animals, and so forth. Subsequently, the data stream is analyzed by neural networks and special software.
All in all, Yandex unmanned vehicles are getting smarter every day due to the digitalization of the economy, the development of intelligent technologies and the creation of artificial intelligence (Figure 01).
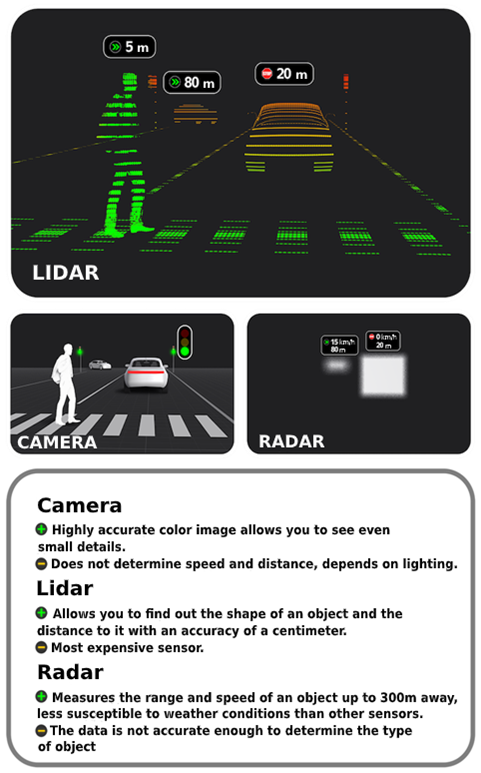
The analysis of the image presented above (Figure 01) allows to assess the potential capabilities of an unmanned vehicle on the road. Particularly, it provides insight into how different traffic participants - cars and pedestrians - behave on the road, thereby suggesting the self-driving car whether to move forward, turn, stop, or other actions. Provided the aforementioned characteristics of the self-driving car, the autonomous vehicle should instantly stop in order to avoid an accident. For instance, when a pedestrian crosses the road on a red light, the self-driving car would read this information from the devices and stop instantly until the obstacles are removed from the road. Thus, the “sense organs” and “vision” of Yandex unmanned vehicles allow to expand the vision from objects of the road network, pedestrians to any other possible obstacles on the road by scanning and recognizing them. In case the unmanned vehicle is not able to bypass an obstacle, the car stops and continues to move only after this obstacle is eliminated. As for the sensors of an unmanned vehicle, they collect information about objects that surround the car, allowing the software to process it. Meantime, the laser radar creates a 3D map of the space around the car, which enables to calculate the distance to objects. This information is gathered from both LIDAR’s and radars, which have more accurate technical capabilities for object recognition.
The unit located on the roof of the autopilot contains 3 LIDAR’s, 5 cameras, GPS and GSM mobile communication antennas, and an antenna for the GNSS satellite navigation system. There are 4 radars in the front of the car, but it is possible to have additional radars. Due to such combination of data obtained from various devices the unmanned vehicle plots the safest trajectory, which instils confidence among the consumers of the services of this type of transport. The safe movement of the autonomous car might also be due to its computer vision and special sensors designed for respective control. At the same time, using the HD-map, the car can determine the most accurate location of the necessary objects, i.e. coordinates of their location on the map. Based on the obtained data, the artificial intelligence of the car decides on the course of travel.
As intelligent technologies and the digitalization of the economy improve, the cost of manufacturing the components of an unmanned module should fall, whereas the supply would grow. Consequently, the price per module will also decrease (at present, the costs of its production are comparable to the price of the machine itself). Meaning that the modules in the near future will be more affordable for the consumer.
The third-generation Yandex unmanned vehicle has seven LIDAR’s, four of which are on the roof and three in the front. In addition, it has six cameras mounted on the roof and six radars: two in the rear, four in the front (two of them are located on sides) (Figure 02).
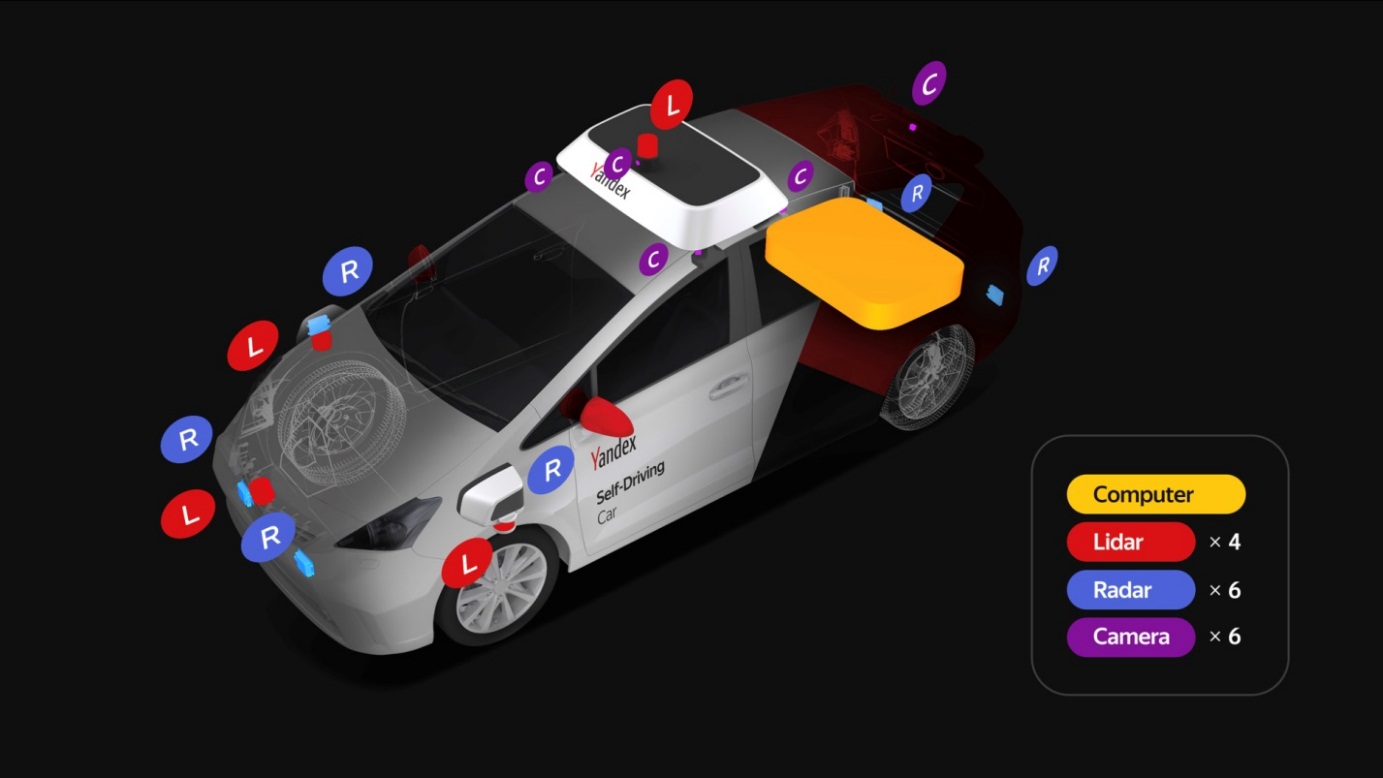
Currently, a fourth-generation unmanned vehicle has been created (Figure 03). Relative to the third-generation unmanned vehicle, the main characteristics of the newest type are presented below as follows:
- it has more cameras. Having added 3 additional cameras with different focal lengths, the newest model has nine cameras in total;
- it has more updated sensors that perfectly read the road picture due to the convenient location;
- the radars have been moved to the roof of the vehicle, which allows the software to distinguish more vehicles around it;
- LIDAR’s on the front fenders now allow to see pedestrians, cars and other objects on the side, thereby expanding the side view;
- it has several NVidia GTX graphics cards, with refined cooling system by Mobis engineers.
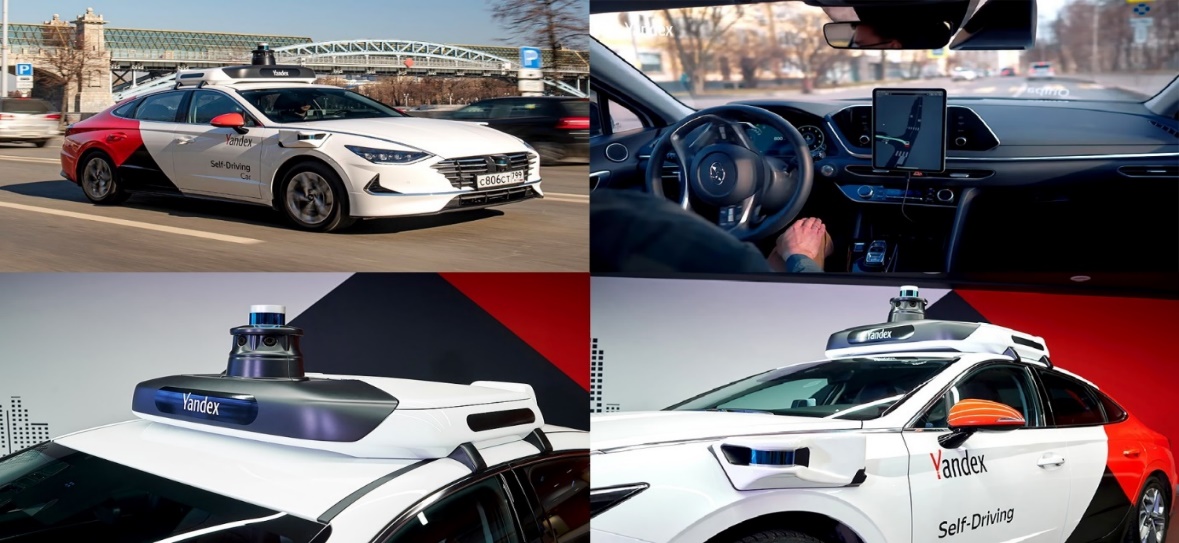
Since unmanned taxi is an expensive pleasure, we will compare it with the taxi of the “Comfort” class - Hyundai Genesis G70. This car has similar characteristics with Hyundai Sonata. We will consider it based on the example of Yandex Taxi. The costs per саг are presented in Table 01.
The starting price of the autonomous car Hyundai Sonata is lower than the price of the taxi Hyundai Genesis G70 by 7%. Taking into account the annual Casco insurance, such difference in the beginning price equals to 9.2%. At the same time, annual Casco insurance costs are lower by 15.8% for unmanned vehicle relative to the taxi of the «Comfort» class. Meanwhile, the starting price of a self-driving taxi includes the cost of equipment to begin work as well as the costs associated with turning it into a self-driving taxi. The costs of equipment to begin work and transformation costs associated with autonomous cars are equal to 65.2%, whereas such costs are not incurred by the taxi of the «Comfort» class.
Since Yandex does not hire drivers, but provides a platform for placing orders, we will proceed from the moment when a hypothetical driver rents a car in the application “Yandex. Drive" and places orders in “Yandex. Taxi" (Table 02).
Let’s assume that the driver's income from orders execution is 15 thousand rubles per day for a 12-hour shift. In calculating profits from the operation of an unmanned vehicle, we will assume that the price of trips and the mileage are similar to those of a taxi of the "Comfort" class. Since “Yandex” is the owner of its unmanned taxi and its software, we need to take into account the costs of car washing and gasoline and subtract them from the final amount of income in order to calculate the profit per day. As a result, “Yandex” gets 15.0 · 0, 25 = 3750 rubles per shift. The above calculation is based on the presumption that the share of income constitutes 25% of the driver's income per shift. If the driver rents out the car from “Yandex. Drive”, “Yandex” will receive 4500 rubles.
Let's calculate the payback period for each type of taxi. To do this, we multiply the weekly profit by the number of weeks in the year (52 weeks) and subtract the annual costs, namely: insurance, car maintenance and a new set of tires (Table 03).
Based on the calculations presented in the Table 03, we can draw the conclusion that the payback period of the unmanned vehicle Hyundai Sonata is 1.17 times lower than payback period of the taxi of the “Comfort” class Hyundai Genesis G70.
Conclusion
The first ride of the autonomous car "Yandex" was performed on June 22, 2018 from Moscow to Kazan. The unmanned vehicle traveled 780 km during 11 hours, following the traffic rules. In the same year, Skolkovo innovation Center has opened an experimental platform for trips by unmanned public transport, equipped with all of the essentials needed to scale up towards the real conditions on public roads.
With the advent of autonomous cars used for passenger transport, the following tasks might be solved in the economy:
- reduction of transportation costs;
- price reduction of the taxi services;
- coverage of more passengers with the taxi services;
- efficient transport management;
- reduction in the number of accidents on highways;
- reduction of harmful emissions and contribution to environmental sustainability.
Should the width of the road space decline in the future, the efficient use of oil and lubricants will increase, thereby contributing to the cost’s minimization.
Given the reason for the large number of crashes and accidents caused by the road transport, reducing threats to economic security in the transport sector is of major importance. Therefore, it is essential to note that with the advent of unmanned vehicles the number of deaths and injuries in the car accidents should significantly decrease.
References
Collie, B., Rose, J., Choraria, R., & Wegscheider, A. (2017). The Reimagined Car. Shared, Autonomous, and Electric. Boston Consulting Group.
Hörl, S., Ciari, F., & Axhausen, K. (2018). Recent perspectives on the impact of autonomous vehicles IVT Working Paper 10XX. Zurich, ETH Zurich. Institute for Transport Planning and Systems IVT.
Hoyle, A. (2016). Apple and Google reportedly buying land for autonomous car facilities. https://www.cnet.com/roadshow/news/apple-and-google-reportedly-buying-land-for-autonomous-car-facilities
Llorca, C., Moreno, A., & Moeckel, R. (2017). Effects of Shared Autonomous Vehicles on the Level of Service in the Greater Munich Metropolitan Area. Paper presented at the International Conference on Intelligent Transport Systems in Theory and Practice mobil TUM 2017 4–5 July. Munich, Germany. https://www.msm.bgu.tum.de/fileadmin/w00bvh/www/publications/ moeckel)
OECD. (2015). https://stats.oecd.org/
Stanley, M. (2013). Autonomous Cars: Self-Driving the New Auto Industry Paradigm. Morgan Stanley.
Van den Berg, V., & Verhoef, E. (2016). Autonomous cars and dynamic bottleneck congestion: The effects on capacity, value of time and preference heterogeneity. Transportation Research. Part B: Methodological, 94, 43–60.
Copyright information
This work is licensed under a Creative Commons Attribution-NonCommercial-NoDerivatives 4.0 International License.
About this article
Publication Date
06 December 2021
Article Doi
eBook ISBN
978-1-80296-118-8
Publisher
European Publisher
Volume
119
Print ISBN (optional)
-
Edition Number
1st Edition
Pages
1-819
Subjects
Uncertainty, global challenges, digital transformation, cognitive science
Cite this article as:
Belova, М. А., Kuntsman, М. V., & Sultygova, А. А. (2021). The Economic Benefits Of Implementation Self-Driving Taxis In A Digital Economy. In E. Bakshutova, V. Dobrova, & Y. Lopukhova (Eds.), Humanity in the Era of Uncertainty, vol 119. European Proceedings of Social and Behavioural Sciences (pp. 810-819). European Publisher. https://doi.org/10.15405/epsbs.2021.12.02.101