Abstract
The article examines the influence of the technological changes on the labor market formation and employment in new forms. It is shown that the employment structure will develop under the increasing influence in market demand for highly qualified workers and wide diffusion of employment alternative forms. Market demand for highly skilled workers in the digital age will grow for several reasons. First, digital technologies can be expected to create a significant number of new jobs in big data analytics, training and management of artificial intelligence, intelligent computing technologies and software development, training, maintenance and management of intelligent robots. Secondly, there is always a new technologies diffusion indirect effect when new jobs are created in related industries. Jobs in these emerging industries will require deep and versatile math, engineering knowledge, job skills that can only be gained through successful graduate and postgraduate studies. Conversely, digital technologies create new opportunities for finding and organizing work for a potential employee, which has already formed a significant market for employment alternative forms. In this regard, the authors aimed to create a mathematical model of jobs technological substitution in the digital economy and to assess the labor force distribution across three forms of employment (full employment, contingent employed and employed on electronic platforms) in economy digital transformation context. For the first time, the authors proposed mathematical models of labor force distribution for three forms of employment, probability distribution curves of labor force technological replacement depending on employment form in context of economy digital transformation.
Keywords:
Introduction
In the last two centuries, economic development is connected with science and education progress. Their close connection provided the production material sectors, and at later development stages and the service sector, with new technologies and materials, it was possible to achieve rapid transformations in the economic and social spheres. At the same time, the relationship between technological progress and employment has always been complex and ambiguous. At the company and firm level, new technologies tended to result in job losses. At the level of individual sectors and the national economy as a whole, this negative effect was offset by institutional factors (such as government intervention or shifts in spatial distribution) or market mechanisms (internal labor migration, the creation of new firms). The economic literature contains quite many works describing the mechanisms of interaction between new technologies and employment (Arntz et al., 2016; Autor & Salomons, 2018; Bessen, 2018; Graetz & Michaels, 2018).
It is to be expected that the impact of new technologies on the employment system will only increase with the transition to the digital economy. The milestone in the digital economy emergence is considered to be 2007, when the mobile Internet started working, which developed so rapidly that by 2017 it occupied almost half of all Internet traffic in the world. During this period, breakthroughs have occurred in key digital technologies - cloud computing, big data analytics, artificial intelligence (A.I.), the Internet of things (IoT), blockchain and A.I. robotics, as well as intelligent computing technologies. The world started talking about the onset of the 4th industrial revolution and the digital economy formation (Schwab, 2016). Today, in a number of developed and avant-garde developing countries of the world, the digital economy foundations have already been laid, which has now become one of the economic growth main driving forces. The digital economy growth rate in the leading countries (USA, Japan, U.K., China, etc.) over the past 5 years averaged 5-7% per year (Huaten, 2019). Thus, the digital economy is already helping to increase labor productivity in production, creating new markets and new points of economy growth, significantly changing the structure of the labor market itself. Therefore, it is quite natural to expect an increase in the the digital economy knowledge intensity as a whole, which implies an increased demand for highly qualified specialists from the STEM sector (Science, Technology, Engineering, Mathematics).
At the same time, the global digital infrastructure formation has created new opportunities for creating a new type of employee-employer relationship. This has found its expression in more developed alternative employment forms, such as: contractors, external part-time and independent workers, employees hired on electronic platforms. At present, all these employment forms are defined as "contingent labor force", which is formed by two main groups: "temporary employment" and "part-time employment". According to OECD estimates, in 2018, the average for the organization, the first indicator was at the level of 11.7% of those with permanent contracts. However, in countries such as Croatia, Korea, the Netherlands, Portugal, Poland, Spain, Chile and Colombia, the figure ranged from 20 to 28.8 percent (OECD, 2020a). As for the second indicator, in 2018 its average value was 16.5% of all employed, but there were also strong deviations from this value: Germany, Great Britain, Japan, Australia, Switzerland and the Netherlands had this indicator ranging from 22 up to 37.3 percent (OECD, 2020b). If we consider the geographically broader regions, then, according to experts, the “contingent labor force” in the first quarter of 2018 in Europe, the Middle East and Africa was about 15.25% of the total labor force, or 68.7 million. people, in the USA their number was about 23.6 million people in 2015. or 16% of the total labor force (Katz & Krueger, 2016; ManpowerGroup, 2018). The Sharing Economy is becoming an important component of the emerging digital economy. The technological basis of the latter is digital platforms, which have opened up new opportunities, both for doing business and for the employees themselves (Degryse, 2016; Valenduc & Vendramin, 2016). Therefore, one of the main changes in the labor market over the past decade has been the emergence of online electronic job search platforms. Thus, clusters of digital technologies are able to radically change the already established labor markets, which can significantly affect the growth rate of the world economy as a whole. In this regard, the purpose of our study is to model and assess the distribution of the labor force in three forms of employment (full employment, contingent employed and employed on electronic platforms) in the context of the digital transformation of the economy.
Problem Statement
An early and pioneering study, published in 2003, noted that mid-level manufacturing and clerical professions are characterized by a high intensity of rule-based procedures that can be characterized as “routine tasks” and which can be relatively easily replaced by computer programs. (Autor et al., 2003). Rapid advances in information and communications technology, beginning in the early 1980s, have accelerated the automation of such routine tasks, enabling the start of the replacement process in many mid-range activities such as accounting, clerical work and batch production. As a result, the relative general economic demand for ordinary middle-level occupations has dropped significantly. At the same time, a significant layer of non-standard manual cognitive tasks remained in the economy, which are difficult to replace with machines or programs (for example, driving a car or cleaning an office). These trends in the labor market, caused by technological factors, occurred against the backdrop of a significantly increased educational level of those employed in the economy. For example, in the American economy, the those employed share in the economy with a secondary education in 1985 amounted to 73.9% against 24.5% in 1940 and subsequently increased to 89.8% in 2018 (U.S. Department of Education, National Center for Education Statistics (2019). table
Research Questions
Market demand for highly skilled workers in the digital age will grow for a number of reasons. First, digital technologies can be expected to create a significant number of new jobs in areas such as big data analytics, training and management of artificial intelligence, development of intelligent computing technologies and software, training, maintenance and management of intelligent robots. Secondly, there is always an indirect effect of the diffusion of new technologies, when new jobs are created in related industries. Jobs in these emerging industries will require the deep and versatile math and engineering knowledge and job skills that can only be gained through successful graduate and postgraduate studies. Today, for example, the high-tech sector in the United States employs about 2.9 million people (1.9% of all employed), in Germany, this sector employs about 2.4% of the workforce. As the McKinsey Global Institute predicts, an increase in spending on technology will create by 2030, within the global economy, a demand for 20-46 million additional, mostly highly skilled, workers, half of which will be in countries such as China, Germany, India, the Netherlands and the United States. At the same time, it is expected that by the same time about 30% of the total working time fund will be automated (Manyika et al., 2017). The U.S. Department of Labor predicts that by 2024 new jobs will be created for the following STEM jobs: ICT (+76%), Mathematics (+7%), Science (+6%), Engineering (+11%) (Bureau of Labor Statistics, U.S. Department of Labor (2015). EMPLOYMENT PROJECTIONS — 2014-24. Retrieved from www.bls.gov/emp/ep_table_102.htm) .
The world today is experiencing a new stage in the labor market evolution, caused by the transition to a high-tech and knowledge-based economy. Currently, knowledge-intensive industries are divided into two subgroups: based on advanced technologies (Leading-edge technology), when internal expenditures on R&D from the cost of sales are at least 9%; and high technologies (High-level technology), when internal expenditures on R&D from the cost of sales are at least 3% (Gehrke et al., 2012). It should be noted that almost all technologies that form the new, digital economy are advanced. And this is primarily due to the fact that these technologies are associated with the knowledge generation based on investments in human capital (Gehrke et al., 2012). Therefore, one should expect an outstripping growth in spending on science and education. It is also necessary to take into account the fact that the R&D sector uses “rare factors of production” - highly qualified workers, researchers and scientists. Consequently, the growing knowledge-intensiveness of the digital economy also creates an increased demand for highly qualified specialists from the STEM sector (Danish Technological Institute, 2015).
Thus, technological development significantly affects the labor market state and its structure, and in the digital transformation context, a wide diffusion of new technologies, and, consequently, the replacement of living labor by machines, should be expected in the intermediate skilled labor segment.
Purpose of the Study
This paper sets two goals:
- to propose a mathematical model for jobs technological replacement in the digital economy;
- to evaluate, based on the model, the labor force distribution in three employment forms (full employment, contingent employed and employed on electronic platforms) in the economy digital transformation context.
Research Methods
Today, the digital economy share in developed countries is approximately 5-10%. It is estimated that the digital transformation of the entire economy will take approximately 10-12 years (Huaten, 2019; Schwab, 2016). Consequently, already in the 2030s, in most countries of the world, the digital economy will function on a significant scale, and the main trend in the development of industry will be the transition to a fully automated digital industry 4.0.
The initial assumptions when building the model are that human capital is formed based on received income and can be described using the formula (Jones & Vollrath, 2013):
where
Another assumption of the study is that the distribution of the labor force in traditional employment obeys the normal law (Monsik & Skrynnikov, 2012, p. 127-128). Denoting the distribution of the relative labor force by skill level
(where
To describe the jobs technological replacement process in the digital economy, let us denote
Assuming the medium-skilled workers active replacement with I.M. and a gradual transition to the highly-skilled workers replacement, let us take the workers replacement probability in the lower segment of the average skill close to one and the replacing researchers probability is close to 0 .
Assuming the logistic law of the substitution process, we obtain the following distribution curve describing the labor force technological substitution probabilities in the current decade:
Where the parameter is found from the condition .
Further, the effective labor force employed distribution in the economy after the digital transformation completion in the 2030s can be written as:
The effective workers shares of low (
Formulas (1-6) form a complex for analyzing the labor force distribution to describe digital transformation impact on labor market.
Findings
As a basis for verifying the proposed model, we use data from the U.S. Federal Bureau of Labor Statistics for 2017 (U.S. Department of Labor, Bureau of Labor Statistics (2018, June). Table 3. Employed contingent and noncontingent workers by school enrollment and educational attainment, May 2017 [Data file]. Retrieved from https://www.bls.gov/news.release/conemp.t03.htm) , (U.S. Department of Labor, Bureau of Labor Statistics (2018, June). Table 7. Employed workers with alternative and traditional work arrangements by school enrollment and educational attainment, May 2017 [Data file]. Retrieved from https://www.bls.gov/news.release/conemp.t07.htm) , (U.S. Department of Labor, Bureau of Labor Statistics (2018, September). Table 3. Electronically mediated workers by selected characteristics, in thousands, May 2017 [Data file]. Retrieved from https://www.bls.gov/cps/electronically-mediated-employment.htm) .
The workers' shares distribution of various categories by education is presented in table
According to the testing the hypothesis of a normal distribution results (with a significance level of
Similarly, the normal distribution hypothesis is confirmed for Electronically mediated workers - the observed Pearson statistics was 4.36, which is less than the critical, the distribution parameters are and .
At the same time, distribution is not normal for contingent workers and workers with alternative working conditions according to the Pearson agreement criterion. However, additional verification showed that a lognormal distribution law is suitable for these employment types, since the share logarithms are normally distributed.
Figure
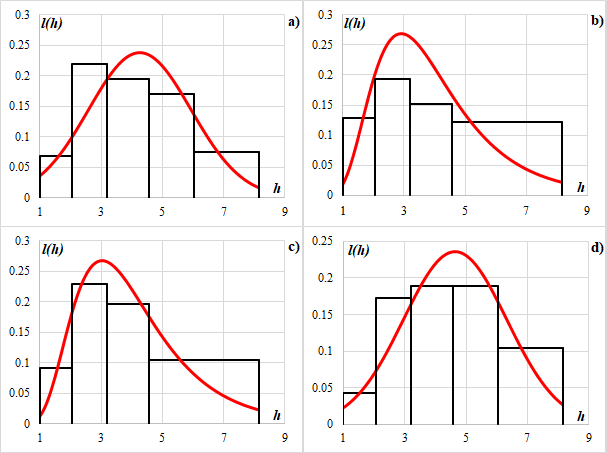
Based on the obtained theoretical distributions, taking into account the functional transformation given by formula (4), using formula (6), we calculated the change in the employed effective labor shares in the U.S. economy as a result of digital transformation (Table
According to calculations using formula (4), digital transformation will lead to 51% of workforce replacement of medium and high qualifications with employment traditional type. Taking into account the distributions characteristics - the contingent labor force average human capital according to formula (1) is 3.83, and for workers with alternative working conditions it is 3.85 - according to calculations using the model, the most likely scenario is in which 19.5% of replaced labor resources will be forced to abandon traditional employment in favor of other types. Thus, if according to U.S. Federal Bureau of Labor Statistics in 2017, 87.1% of workers with traditional employment were observed in the employed structure, then as a result of digital transformation, this share will decrease to 67.6%. In this scenario, replacing labor process with intelligent machines will lead to an increase in electronically mediated workers number by 26%.
Conclusion
The 2020s will see a steady technological shift in labor demand towards high qualifications. According to our estimates, in the 2030s in U.S. economy the highly skilled workers share with a master's degree and above will be 26.5% of employed in labor force economy, against the current 17.2%. Also, in the course of digital transformation, there will be a significant polarization of labor into highly- and low-skilled, with a sharp reduction in jobs of average qualifications - the workers share with average qualifications will decrease to 49.2%, from the current 72.4%.
As a result of workforce replacement with I.M., the employed on electronic platforms share will grow by 26%, and contingent workers and workers with alternative working conditions will account for about third of all employed in labor market.
Acknowledgments
This article was prepared as part of the RFBR grant No. 20-010-00279 “An integrated system for assessing and forecasting the labor market at the stage of transition to a digital economy in developed and developing countries.”
References
- Acemoglu, D. (2009). Introduction to Modern Economic Growth. Princeton: Princeton University Press.
- Acemoglu, D., & Autor, D. H. (2011). Skills, Tasks and Technologies: Implications for Employment and Earnings. Handbook of Labor Economics, 4B(12), 1043-1171.
- Arntz, M., Gregory, T., & Zierahn, U. (2016). The Risk of Automation for Jobs in OECD Countries: A Comparative Analysis. OECD Social, Employment and Migration Working Papers, 189. https://www.oecd-ilibrary.org/social-issues-migration-health/the-risk-of-automation-for-jobs-in-oecd-countries_5jlz9h56dvq7-en
- Autor, D. H., Levy, F., & Murnane, R. (2003). Тhe Skill Content of Recent Technological Change: An Empirical Exploration. Quarterly Journal of Economics, 118(4), 1279-1333. DOI:
- Autor, D. H., & Dorn, D. (2013). The Growth of Low-Skill Service Jobs and the Polarization of the U.S. Labor Market. The American Economic Review, 103(5), 1553-1597. DOI:
- Autor, D. H., & Salomons, A. (2018). Is Automation Labor Share-Displacing? Productivity Growth, Employment, and the Labor Share. NBER Working Paper, 24871. http://www.nber.org/papers/w24871.pdf
- Bergson-Shilcock, A. (2017). Foundational Skills in the Service Sector. Washington, DC: NATIONALSKILLSCOALITION. https://static1.squarespace.com/static/55a158b4e4b0796a90f7c371/t/58955943e6f2e17b27f98df2/1486182731254/2.3+NSC+foundational+skills_v7.pdf
- Bessen, J. E. (2018). Automation and Jobs: When Technology Boosts Employment. Boston Univ. School of Law, Law and Economics Research paper, 17-09. https://scholarship.law.bu.edu/cgi/viewcontent.cgi?article=1809&context=faculty_scholarship
- Danish Technological Institute (2015). Does the E.U. need more STEM graduates? https://op.europa.eu/en/publication-detail/-/publication/60500ed6-cbd5-11e5-a4b5-01aa75ed71a1
- Degryse, C. (2016). Digitalisation of the Economy and its Impact on Labour Markets. https://www.researchgate.net/publication/297392058_Digitalisation_of_the_Economy_and_its_I mpact_on_Labour_Markets
- Gehrke, B., Frietsch, R., Neuhäusler, P., Rammer, C., & Leidmann, M. (2012). Re-Definition of Research-Intensive Industries and Goods. https://www.econstor.eu/bitstream/10419/156589/1/StuDIS_2013-08e.pdf
- Graetz, G., & Michaels, G. (2018). Robots at Work. Review of Economics and Statistics, 100(5), 753-768. DOI:
- Huaten, M. (2019). Tsifrovaya transformatsiya Kitaya [The digital transformation of China]. Moscow: Alpina.
- Jones, Ch. I., & Vollrath, D. (2013). Introduction to Economic Growth. London: W. W. Norton & Company.
- Katz, L. F., & Krueger, A. B. (2016). The Rise and Nature of Alternative Work Arrangements in the United States, 1995-2015. NBER Working Paper, 22667. https://www.nber.org/papers/w22667
- Kochhar, R., & Barroso, A. (2020). Young workers likely to be hard hit as COVID-19 strikes a blow to restaurants and other service sector jobs. https://www.pewresearch.org/fact-tank/2020/03/27/young-workers-likely-to-be-hard-hit-as-covid-19-strikes-a-blow-to-restaurants-and-other-service-sector-jobs/
- Manyika, J., Chui, M., Miremadi, M., Bughin, J., George, K., Willmott, P., & Dewhurst, M. (2017). A Future that Works: Automation, Employment, and Productivity. New York: McKinsey Global Institute.
- Monsik, V. B., & Skrynnikov, A. A. (2012). Veroyatnost' i statistika [Probability and statistics]. Moscow: BINOM.
- ManpowerGroup (2018). Quarterly Market Report European Contingent Workforce Q1. https://insights.manpowergroupsolutions.com/wp-content/uploads/2018/03/Q1-2018-European-QMR_201803.pdf
- OECD (2020a). Temporary employment (indicator). DOI:
- OECD (2020b). Part-time employment rate (indicator). DOI:
- Schwab, K. (2016). The Fourth Industrial Revolution. New York: Crown Business.
- Schwab, K., & Davis, N. (2018). Shaping the Future of the Fourth Industrial Revolution: a Guide to Building a Better World. USA: Crown Publishing Group.
- Siu, H., & Jaimovich, N. (2012). Jobless Recoveries and the Disappearance of Routine Occupations. NBER Working Paper No. 18334. https://www.nber.org/papers/w18334.pdf
- Valenduc, G., & Vendramin, P. (2016). Work in the digital economy: sorting the old from the new. http://ftu-namur.org/fichiers/Work_in_the_digital_economy-ETUI2016-3-EN.pdf
Copyright information
This work is licensed under a Creative Commons Attribution-NonCommercial-NoDerivatives 4.0 International License.
About this article
Publication Date
16 April 2021
Article Doi
eBook ISBN
978-1-80296-104-1
Publisher
European Publisher
Volume
105
Print ISBN (optional)
-
Edition Number
1st Edition
Pages
1-1250
Subjects
Sustainable Development, Socio-Economic Systems, Competitiveness, Economy of Region, Human Development
Cite this article as:
Akaev, A., Sarygulov, A., Petryakov, A., & Podgornaya, V. (2021). Technological Development And Employment Structure In Context Of Economy Digital Transformation. In E. Popov, V. Barkhatov, V. D. Pham, & D. Pletnev (Eds.), Competitiveness and the Development of Socio-Economic Systems, vol 105. European Proceedings of Social and Behavioural Sciences (pp. 57-66). European Publisher. https://doi.org/10.15405/epsbs.2021.04.7