Abstract
The regional domestic development tourism in Russia is critical today due to ongoing geopolitical, economic, and epidemiological events. Using the existing tourism potential, diversifying the regional economies through the development of tourism and related industries are the near future tasks for regional politics. It is necessary to systematize the national tourism development factors in the regions and analyze their impact on tourist flows, which is the purpose of this work. The authors study the existing scientific background in the field of research on the factors for national tourism development in the regions, identify and systematize the factors of national tourism development, and propose a system of indicators for domestic tourism analysis. As a result of the study, regression models of factors affecting tourist flows in Russian regions were developed. The paper uses statistical analysis methods. The information base of the study is statistical sample in the regional context of the Federal Statistics Service of Russia, data from portals devoted to the tourism development in the Russian Federation, data from the rating agency "Expert" on the investment attractiveness of Russian regions. As a result, a multidimensional grouping was carried out by the analytical hierarchy method; four models developed for the entire sample and three groups of regions. The specific factors influencing the development of regional tourism in Russia in various regions are highlighted. Recommendations for the development of regional tourism are formulated based on the developed models.
Keywords:
Introduction
According to Rostourism estimates, the Russian tourism industry has suffered losses of about 1.5 trillion rubles due to the pandemic; this is one of the most affected industries. It is important to note that such a sharp decline could be a chance for national tourism development in our country if the Government manages to develop effective mechanisms to support the industry and redirect tourist flows into the country. This requires serious, careful work to identify the potential and domestic tourism development factors in the regions. Many countries recovering from the coronavirus epidemic are currently developing tools to support domestic tourism (for example, subsidizing travel tickets to domestic tourist destinations - the experience of Japan and Kazakhstan). The purpose of this article is to investigate the factors of national tourism development in the regions of Russia and to build a correlation-regression model describing the influence of these factors on tourist flows in the regions. The development of tourism in many potentially ready-made regions will diversify regional economies, improve their image and attractiveness, including investment, and it would stop the "flight" of capital and human resources from the regions. Therefore, in our opinion, this topic is extremely relevant today.
Despite the great interest of scientists in the problems of tourism development in Russia and abroad, the factors of tourism development, in our opinion, remain unexplored. Kolpakidi (2015) explores institutional mechanisms (programs to support small businesses in tourism) on the example of the Irkutsk region. Adashova (2016) studies the creative potential of young people as a factor of tourism development because they are actively involved in volunteer activities and help create certain tourist brands. Kuzmina and Pegushina (2019) conducted a comprehensive analysis of the factors of the development of environmental and ethnographic tourism in the Republic of Crimea. Kirillova and Chernukha (2017) analyze the state of tourism in Russia and the Republic of Bashkiria, and the authors revealed a tendency to increase national and inbound tourism flows both at the macro level and the regional level. These researchers identify infrastructure, institutional, resource, environmental, and image factors that hinder tourism development. Fidorenko (2016) gives a more complex classification when analyzing the tourism development factors in rural regions. The author identifies two factors: first-order factors (static, natural-climatic, and cultural-historical) and second-order factors (institutional, economic, personnel, socio-demographic). Many researchers study the impact of major sporting events on the regional economy in the economic literature. Scandizzo and Pierleoni (2018) examines the impact of the Olympic Games on the economy of the regions, including aspects of sustainable economic development. Swart et al. (2018) explores the experience of holding the 2014 World Cup in Brazil. The authors propose a model for tourism development, which allows assessing the likelihood of repeated visits to Rio de Janeiro by football fans. The model includes such factors of tourist satisfaction as information support, the image of the territory, and criminal risks. The authors conclude that careful marketing creation and promotion of the territory's brand are the most critical factors. Meurer and Lins (2018) also explore the impact of the 2014 World Cup and the 2016 Olympic Games on Brazil's tourism and economic development. They revealed an increase in tourist inflows of about 50% during the World Cup for two months, and 28% during the month during the Olympics, although they note that these effects are short-term. Gil-Alana et al. (2019) analyze the impact of the London Olympic Games, Brazil World Cup, and Rio Olympic Games on the regional economic growth. Castanho et al. (2020) investigate factors attracting tourists in the Azores. These are primarily hotels (54.7%) and nature (51.9%); culture (15%) and service quality (17.9%) became the least influential factors. Scandizzo and Pierleoni (2018) study the influence of the Olympic Games on the regional economy, including sustainable economic development aspects. Silova et al. (2020) study the impact the FIFA World Cup held in 11 Russian regions in 2018 on regional economies. Zhang et al. (2020) show the importance of measures to support tourism by the municipal Government using the example of Taiwan entrepreneurs engaged in the tourism sector. Liu et al. (2020) analyzed the problem of tourism regulation on China's example and revealed the institutional factors of tourism development. The authors highlighted five critical aspects of institutional regulation: truthful informing tourists, regulating the rules, supporting tourists, and fulfilling contracts with them, processing reviews left by tourists, feedback. Thus, researchers devote their works to individual regions or individual areas when considering tourism development problems, but there is no comprehensive analysis of the regional tourism development factors Chen et al. (2020) researched different aspects of China’s tourism development including geography, publicity, environmental protection and others. Rosato et al. (2021) study the problem of sustainable development and tourism influence, different business models were analized.
Problem Statement
Today the search for domestic economic development factors that can improve the quality of people’s lives and increase economic well-being, develop and diversify regional economies, as well as reduce the impact of external sanctions and the consequences of the 2020 pandemic, which led to severe crisis phenomena in many sectors of the economy, becomes essential. The tourism industry was severely affected in 2020, but at the same time, as the results of the tourism season of 2020 showed, the southern regions of Russia experienced a real boom in domestic tourism. The potential of domestic tourism in many regions of Russia is enormous but underestimated. For national tourism clusters, today there is a window of opportunity that should be used for advanced development, to strengthen and develop domestic tourism. That will attract additional investment in the regions, increase the standard of living of people in the regions, solve many structural economic problems. The Government considers the promotion of tourism to be an important measure of economic development at the regional level. This requires a scientific understanding of the factors that lead to changes in tourist flows, the construction of relevant analytical models, a scientifically based forecast of specific measures implementation aimed at the development of domestic tourism. The creation of a quality institutional environment as a formal institutional framework in which participants in relevant economic processes can act as conveniently as possible and the development of informal institutions associated with sustainable changes in culture and business practices should also be an essential element of regional economic policy.
Research Questions
The study raised and resolved questions: what factors affect the national tourism development in Russia, what the nature of these factors influence is, and how similar these factors are for all Russian regions. The authors analyze the following factors as initial test factors:
1. The readiness of regional residents to travel outside the region (indirectly characterizes the reluctance to relax in the region);
2. Investment attractiveness of the region (indirectly characterizes the degree of economic development);
3. Degree of tourism development in the region as assessed by relevant institutions
4. Average per capita income (indirectly characterizing economic well-being in the region);
5. The cost of arriving in the region's capital from Moscow (the higher, the less attractive it is for tourists).
Purpose of the Study
The purpose of the article is to analyze the domestic tourism development factors in the Russian regions, using regression analysis methods and systematized data on tourist flows and development of Russian regions in 2018.
Research Methods
The authors used statistical data collection methods, cluster analysis methods, and regression analysis methods. The logic of the study is described in five stages:
1. Forming a sample for analysis: collecting data on the number of tourists who visited each region and the main factors that can affect this indicator. The clue indicators are the number of residents leaving the region on tourist trips to Russia and abroad, the average per capita income in the region, rating of tourist attractiveness of the region, rating of investment attractiveness of the region, cost of a ticket from the regional capital to Moscow. Indicators reflecting tourist flows are given in relative form by dividing by the number of inhabitants;
2. Clearing the sample of incomplete data (missing data) and emissions (outliers) - regions in which the values of the indicators under consideration do not fit into the logic of all-Russian trends (such as Moscow, Krasnodar Territory);
3. Multidimensional grouping by cluster analysis, identification of groups of regions homogeneous in terms of selected indicators;
4. Developing and evaluation of linear regression model parameters for the whole sample and each group, comparison of results, identification of excess and harmful variables;
5. Calculation of refined models for each group, comparison, and interpretation of results.
The study is based on statistical data provided by the Federal Statistics Service of the Russian Federation on the number of tourists who visited the regions of Russia in 2018 (the resulting indicator). In the study, the authors used the following indicators: the population of the region, average per capita income in the region; these indicators are provided by the Federal Statistics Service of the Russian Federation. The authors also use the rating of tourist attractiveness of the region, calculated by the Center for Information Communications "Rating" and the magazine "Rest in Russia." This rating takes into account such components as the level of the hotel business and infrastructure development; the significance and profitability of the tourism industry in the region's economy; the region’s popularity among tourists; the region’s popularity among foreigners; tourism uniqueness; crime rate; interest in the region on the Internet; promotion of the region's tourism potential in the information space. For estimation of the regional economic development, we used the rating of investment attractiveness provided by rating agency ExpertRA. Also, the authors use the number of tourists from among the population of the region who went on tours in Russia and on foreign tours (the data provided by Federal Statistics Service); ticket price (mainly avia) from the capital of the region to Moscow (the data is provided by Aeroflot company’s site).
Findings
Data design
The calculations were made using open source software Wessa.net (Wessa, P., (2017), Agglomerative Nesting (v1.0.5) in Free Statistics Software (v1.2.1), Office for Research Development and Education, URL https://www.wessa.net/rwasp_agglomerativehierarchicalclustering.wasp/) . At this stage, data were collected for analysis, cleared of incomplete data, and emissions. As a result, 66 out of 85 Russian regions were suitable for analysis. Some regions did not provide complete data; other regions were excluded as outliers. As a result, a table was formed, including one explained variable (Y) and six explaining variables (X1... X6). A fragment of the table for regional data from the Central Federal District is given in Table
Hierarchical clustering
On the next stage, the authors compute the agglomerative nesting (hierarchical clustering) of a multivariate dataset, as proposed by Kaufman and Rousseeuw. At each level, the two nearest clusters are merged to form the next cluster using the Euclidean metric. This procedure computes the 'agglomerative coefficient,' which can be interpreted as the amount of clustering structure that has been found. Results of calculations presented on the figure
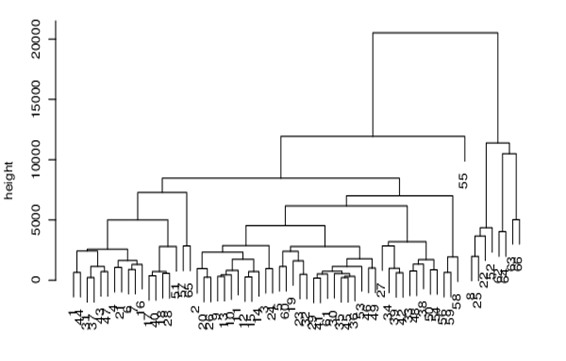
After this procedure, three types of regions with similar values of tourism development factors are defined; the results are presented in table
The first group of regions is relatively close to Moscow, except for a few (Arkhangelsk, Amur regions), as evidenced by the relatively moderate price of a flight to the capital. The highest tourist attractiveness ratings distinguish the first group of regions, but their investment attractiveness is more than twice lower than that of the second group. We can say that these are the regions with the highest tourist potential. The second group of regions has the highest share of tourists relative to the population - almost 90%. The second group included regions from a variety of federal districts, but mainly from Central Russia. The price of air tickets to Moscow in this group is minimal. Nevertheless, this group has the lowest index of tourist attractiveness
The development of business tourism characterizes the second group's regions since they have the highest rating of investment attractiveness. These are the leading regions in the field of tourism, but it is necessary to develop the tourist image. The regions of the third group are quite far from the center, except for the Moscow region. This explains the highest price of air tickets. At the same time, these regions have high tourist attractiveness (due to unique attractions), but moderate investment attractiveness. In the third type of regions, in our opinion, it is necessary to develop infrastructure, make the region more accessible for extensive tourism. In the next step, linear regression models are constructed for each group of regions and the entire sample using the least-squares method.
Source models evaluation
The following formulae (1) to (4) show the calculated regression models for the entire sample of regions and each of the selected three groups, respectively. The description of variables X1 - X6 corresponds to Table
(1)
(2)
(3) (4)
Normalized R-squared for basic model is 0.173, for group models – 0.375, 0.220, 0.694 respectively. This means that the distribution of regions into three groups made it possible to form qualitatively homogeneous groups. The influence of factors on regional tourism development has its special character in each. As can be seen from the given values of t-statistics for coefficients, different factors have a statistically significant influence in different groups of regions. In the next step, we exclude the variables which were not statistically significant from the models for the groups of regions.
Models enhancements
For the first group of regions, X1, X2, X5 are recognized as statistically significant factors. The number of tourists in these regions was significantly influenced by the share of tourists traveling from the region in Russia (X1) and abroad (X2), as well as the size of the average per capita income of the residents of the region (X5). A model built on three significant factors showed that X1 and X5 have a positive effect, and X2 - a negative:
(5)
Normalized R-squared is 0.467, and F-statistics is 5.96. This means that the model is high-quality and corresponds well to the source data.
The number of tourists in the regions of the second group was significantly influenced by the share of tourists traveling from the region to Russia (X1), as well as the investment attractiveness of the region (X4) and the ticket price from the regional capital to Moscow (X6). A model built on three significant factors showed that X1 and X4 have a positive effect, and X6 - a negative:
(6)
Normalized R-squared is 0.293, and F-statistics is 5.84. This means that the model is high-quality and corresponds well to the source data. However, of all the resulting models, this one requires further improvement, including through the search for outliers. However, of all the final models, it is this one that requires further improvement, including through the search for outliers.
The number of tourists in the regions of the third group was significantly influenced by the national tourist rating of the region (X3), the investment attractiveness of the region (X4) and the average per capita income (X5). The model built on three significant factors showed that X3 and X5 have a positive effect, and X4 – negative. The number of tourists in the regions of the third group was significantly influenced by the national tourist rating of the region (X3), the investment attractiveness of the region (X4) and the value of per capita income (X5). The model built on three significant factors showed that X3 and X5 have a positive impact, and X4 - a negative one:
(7)
Normalized R-squared is 0.877, and F-statistics is 15.74. This means that the resulting model is high-quality and corresponds well to the source data. This model has the highest quality of all.
Conclusion
The study allows to distinguish three types of regions by the level of tourism development. The first type of region is with the most significant tourist potential. Tourist flows here are significantly influenced by average per capita income. These are regions with high tourist attractiveness, but economic development here is moderate. Therefore, tourism development in these regions can solve issues of economic growth. The regions in the second group are leaders in the field of tourism. The investment attractiveness of the region and the cost of flight have a significant influence on tourist flows. In these regions, a policy should be pursued to create and promote tourism brands. In the regions of the third group, the national tourist rating and incomes of the population have a significant impact on touristic flows. These are regions located far enough from the center, so the unique tourist places determine the presence of tourist flows. Interestingly, in these regions, investment attractiveness harms the size of tourist flows. The presented results of the analysis can be used to develop measures to stimulate tourism in different regions.
References
- Adashova, T. A. (2016). Developing the creative potential of young people as a factor in the effective development of Russian tourism. Russian regions: looking to the future, 3(1), 43-51.
- Castanho, R. A., Couto, G., Pimentel, P., Carvalho, C. B., & Sousa, Á. (2020). Territorial management and governance, regional public policies and their relationship with tourism. A case study of the Azores Autonomous Region. Sustainability (Switzerland), 12(15), 6059.
- Chen, A., Ng, Y., Zhang, E., & Tian, M. (2020). Research on Regional Tourism Development. In: Dictionary of Geotourism. Singapore: Springer. DOI:
- Fidorenko, Y. I. (2016). Factors of rural tourism development in the Russian Federation. Socio-economic phenomena and processes, 11(1), 100-107.
- Gil-Alana, L. A., dos Santos Figueiredo, O. H., & Wanke, P. (2019). Structural breaks in Brazilian tourism revenues: Unveiling the impact of exchange rates and sports mega-events. Tourism Management, 74, 207-211.
- Kirillova, S. A., & Chernukha, D. S. (2017). Development of global, national and regional tourism: state, trends, forecasts. Online journal Science, 9(6), 82.
- Kolpakidi, D. V. (2015). The development of the institutional environment as a factor in improving the management of the development of entrepreneurial structures in the field of tourism. Economics and management, 12(122), 29-34.
- Kuzmina, O. M., & Pegushina, A. A. (2019). Identification and systematization of factors of development and attractiveness of the Republic of Crimea in the context of the development of ecological and ethnographic tourism. Service in Russia and abroad, 5(87), 110-124.
- Liu, Y., Yao, Y., & Fan, D. X. F. (2020). Evaluating Tourism Market Regulation from Tourists’ Perspective: Scale Development and Validation. Journal of Travel Research, 59(6), 975-992.
- Meurer, R., & Lins, H. N. (2018). The effects of the 2014 World Cup and the 2016 Olympic Games on Brazilian international travel receipts. Tourism economics, 24(4), 486-491.
- Rosato, P. F., Caputo, A., Valente, D., & Pizzi, S. (2021). 2030 Agenda and sustainable business models in tourism: A bibliometric analysis Ecological Indicators, 121, 106978 DOI:
- Scandizzo, P. L., & Pierleoni, M. R. (2018). Assessing the Olympic Games: the economic impact and beyond. Journal of economic surveys, 32(3), 649-682.
- Silova, E., Pletnev, D., Seifi, A., Motaghi, S., & Ahmadi, S. (2020). GDP, investments, and touristic flows in Russian regions –hosts of major sporting events. Economic and Social Development. 50th International Scientific Conference on Economic and Social Development. Book of Proceedings, 209-218.
- Swart, K., George, R., & Cassar, J. (2018). The 2014 FIFA World Cup (TM): Tourists' satisfaction levels and likelihood of repeat visitation to Rio de Janeiro. Journal of destination marketing & management, 8, 102-113.
- Zhang, Y., Chan, J. H., Ji, Z., Lane, B., & Qi, X. (2020). The influence of community factors on local entrepreneurs’ support for tourism. Current Issues in Tourism, 23(14), 1758-1772.
Copyright information
This work is licensed under a Creative Commons Attribution-NonCommercial-NoDerivatives 4.0 International License.
About this article
Publication Date
16 April 2021
Article Doi
eBook ISBN
978-1-80296-104-1
Publisher
European Publisher
Volume
105
Print ISBN (optional)
-
Edition Number
1st Edition
Pages
1-1250
Subjects
Sustainable Development, Socio-Economic Systems, Competitiveness, Economy of Region, Human Development
Cite this article as:
Pletnev, D., Silova, E., Seifi, A., & Mottaghi, S. (2021). Regional Tourism Development In Russia. In E. Popov, V. Barkhatov, V. D. Pham, & D. Pletnev (Eds.), Competitiveness and the Development of Socio-Economic Systems, vol 105. European Proceedings of Social and Behavioural Sciences (pp. 999-1009). European Publisher. https://doi.org/10.15405/epsbs.2021.04.106