Abstract
The article analyzes the problems of managing a loan portfolio of a commercial bank and identifying potentially troubled debt as the most significant part of assets. Modern trends in the banking industry, which are associated with a decrease of marginality, oblige banks to look for ways to improve the efficiency of their activities Among the two areas that are significant factors in increasing the efficiency of current business models of banks, it is advisable to highlight work with a credit portfolio and modern technologies. The authors systematize the basic principles of managing the credit portfolio of corporate borrowers, which are based on proactive diagnostics. The analysis is based on a fundamental analysis of the external and internal parameters of the borrower, depending on its industry affiliation. The main idea is to use key elements of the enterprise's operating cash flow and sensor data from the equipment (IoT), that is involved in the production process. The modelling of the normal state of the enterprise, risk assessment of the level of technological facilities, financial risks (identifying cash shortages, failure of a CF for Debt Service) is assumed due to the analysis of the retrospective dynamics and structure of costs (specific consumption of raw materials, reagents, power resources of the target product), data of raw materials' processing facilities and finished products release, the warehouses (inventory of raw materials, semi-finished and finished products).
Keywords: Commercial bankcredit portfoliointernet of thingspotentially troubled assets
Introduction
The status of the country's financial sector is not satisfactory. In 2019, the growth rate of assets and lending volumes significantly slowed to 2.7% and 1.2% per year (Central Bank of the Russian Federation, 2020). The main asset of credit institutions are loans. The level of overdue debt of the corporate portfolio (Non Performing Loan PL90+) in 2019 increased by 0.7% compared to last year and is 5.4%. In 2019, the total revenue of the banking sector decreased by 23% compared to the same period last year. Non-interest incomes still accounts for a large share of the revenue structure (84% in 2019) (Pomulev, 2020a). The median value of the efficiency coefficient in the industry is 59%, the net interest margin is 3%, the net profitability of equity is 12%, and the return on assets is only 1.5% (Central Bank of the Russian Federation, 2020). Among the reasons for the decline in performance indicators, we should note the slowdown in economic growth, increased competition (including from financial and technical companies), and regulator's requirements strengthening. Due to epidemiological factors, the results of 2020 will be objectively worse, taking into account the need to create reserves for possible loan losses. In conditions of reduced marginality and instability, it is necessary to look for ways to reduce all non-operating expenses and focus on identifying potentially problematic assets in order to prevent a decrease in the quality of the loan portfolio (69% of the assets of credit institutions are credit products).
The topic is particularly relevant in the context of the crisis caused by COVID-19, when there is a widespread decline in household and business incomes. It is very important to use tools to identify problems of borrowers at an early stage at an early stage in order to prevent falling in arrears in the future using the full potential of modern technologies. It is obvious that many researches assess the potential of modern technologies as very high. Banks' business is influenced by the following technologies: a) mobile technologies and cross-channel communications, b) virtualization technologies, remote access, remote office, etc., C) distributed ledger technology (blockchain); d) big data; e) artificial intelligence and machine learning (HSE, 2019). However, according to a Gartner survey, about 20% of global IT spendings ($600 billion dollars a year) do not bring any additional revenue for companies (Pomulev, 2020b). The different experience of foreign colleagues warns that the choice of technologies should be approached responsibly. The paper attempts to solve the problem of diagnostics of the credit portfolio of corporate borrowers of a commercial bank using the potential of modern technologies.
Problem Statement
The purpose of managing the quality of the credit portfolio is to identify high-risk credit operations, adequate assessment and formation of reserves for possible loan losses. Indicators for assessing the quality of a commercial bank's assets are not objective enough. The cost of risk is estimated using the amount of reserves on the possible loanes losses and the level of overdue debt in the portfolio (Non Performing Loan PL90+). The NPL indicator does not reflect the full situation on the amount of overdue debt, because it is a relative indicator and the share of overdue debt decreases with the growth of the portfolio. To assess the quality of the credit portfolio, many banks develop internal risk assessment models (probability of default, client rating), which include a variety of quantitative and qualitative factors. The credit portfolio is segmented into several levels according to risk groups and the degree of return. However, the used metrics are lagging and do not allow to quickly respond to emerging business problems of borrowers, which ultimately leads to a deterioration in solvency and the occurrence of default. Often, the bank recalculates the client's rating upon the occurrence of arrears. Leading indicators are missed, or due to the slowness of the system, even if a sign of a problem is detected, or due to bureaucratic obstacles, the credit institution does not have time to develop measures plan to solve the problem.
Many authors studied the problem of identifying potentially problematic assets and managing portfolio quality (Akwaa-Sekyi & Moreno, 2017; Alali et al., 2012; Ali et al., 2018; Ames et al., 2018; Andries & Brown, 2017; Chen & Lin, 2016; Kolozhvari, & Bersenev, 2018; Korkina & Kukharev, 2015; Pomulev & Kalmykov, 2021). However, it cannot be said that the topic is sufficiently worked out.
With the development of modern technologies and the emergence of a large amount of data on borrowers on the Internet, other problems have appeared that are associated with the accumulation, storage, processing and analysis of data. Often there are problems with the processing of structured data (for example, customer transactions, information on credit transactions). Not to mention big data (a lot of unstructured data) How to make the most out of them? What technology to choose? Use ready-made solutions or develop your own system? What should be the architecture of the system? How to build a management system to ensure a quick response? How to build a business process correctly?
Research Questions
A well-organized management process plays an exceptional role in improving the quality of the loan portfolio. However, in this process there is not enough time to understand the whole essence of economic phenomena that occur in the economic activities of borrowers. In fact, modern methods of risk assessment are compiled for separate groups of indicators, which, like a mosaic, form a collective image of the borrower's risk profile. The relative values of some coefficients of financial analysis (for example, liquidity, financial stability, profitability) do not interpret the reasons for the current situation, and even more, do not allow making any reliable forecasts. Data on transactions, which have recently been actively used by banks, increase the accuracy of the forecast, but identify the fact of the movement of financial cash flows in business and do not explain the essence of operating activities. With the appearance of modern technologies of data transmission (IoT) from industrial and other equipment, there is a unique chance to simulate the production processes of an enterprise and to assess risks online. In this research, we study the potential of sensors data (IoT), an algorithm for their analysis and business architecture for solving the problem of proactive diagnostics of the corporate borrowers business. The ways of monetizing IoT technologies for corporate borrowers and the bank are evaluated, and problems that may hinder the implementation of the technology are considered.
Purpose of the Study
The purpose of the study is to develop approaches to managing a commercial bank's loan portfolio using modern technologies.
Study objectives:
1.To form the principles of systematic management of the loan portfolio quality.
2.To study data that can be used to quickly diagnose the loan portfolio
3.To analyze the potential of the Internet of things technology for managing the quality of a bank's loan portfolio.
4.To develop an algorithm for diagnosing a loan portfolio using the selected technology.
5.To assess the economic feasibility of implementing the approach used in managing the quality of the loan portfolio.
Study hypothesis: the Internet of things technology is economically justified when implementing a systematic approach to managing the quality of a commercial bank's loan portfolio.
Research Methods
The management process of the loan portfolio quality is based on the principles of risk management (identification, analysis, assessment and response). The key driver of the process efficiency is the identification of risk sources, their classification and assessment using the tools of mathematical statistics. To develop a qualitative model, two types of data are used: discrete and continuous, which allows you to choose appropriate methods for their analysis. Correlation-regression analysis tools are used to analyze continuous data. Analysis and identification of relationships in discrete data is carried out using the Chi-Square, ANOVA, Bartletts, Moods Median, Levenes methods. However, the mathematical approach does not always explain the economic essence of the relationship between the variables. In this regard, the work used the methods of financial and economic analysis to increase the level of interpretability of the identified relationships between the variables.
Methods of net present value, internal rate of return, payback period were used to assess the effectiveness of the technology implementation. The empirical basis of the study is the activities of borrowers of the industrial sector of the economy of one of the largest banks in Russia.
Findings
Improving approaches to managing the quality of the loan portfolio
The quality of the loan portfolio depends on the qualifications of the bank's management, the risk culture formed among the employees, and the bank's credit policy. The analysis of the quality of the loan portfolio and related management considers both the portfolio as a whole and each loan separately. This is a systematic observation and study of credit and collateral transactions in dynamics, in comparison with average indicators, work with deviations and elimination of managerial and operational errors. The analysis of the quality of the loan portfolio and related management considers both the portfolio as a whole and each loan separately. This is a systematic observation and studying of credit and collateral transactions in dynamics, in comparison with average indicators, work with deviations and elimination of managerial and operational errors. The authors understand the criteria of portfolio quality as profitability, the amount of credit risks, the percentage of collateral.
Principles of quality management of the loan portfolio.
Identification of potentially problematic assets should be based on a continuous credit portfolio management system.
- financial indicators of the enterprise - revenue, costs, net profit, circulate assets, accounts payable, etc. (data from the enterprise's ERP system, SPARK);
- transaction data of the borrower (turnover dynamics, arrests, card files, etc.);
- the physical volume of production output and raw material consumption (sensors are a fundamentally new direction, the bank does not have such data);
- credit record, new loans, delinquencies in other banks (National Bureau of Credit Records);
- claims against the borrower, the borrower is the defendant, the amount of the claim, bankruptcy, etc. (Casebook).
Analysis of modern technologies in credit portfolio quality management
The rapid growth in the number of data in the world over the past few years on the Internet has defined a new type of production-information factor. However, a significant part of the data that is generated by all people becomes outdated quite quickly. Not all data is useful, and a significant part of it is simply useless. To extract value from this data, you need to be able to store, manage, process, and analyze it effectively. Unstructured data (big data) requires the use of new technological solutions. Various sensors on equipment (CNC equipment is a source of a huge amount of data), surveillance cameras placed on various objects are widely used. This data can be transmitted using Internet of things technology. Of all disruptive technologies, the Internet of things technology is currently the most promising (in terms of the delivered data value). The term Internet of things is used to refer to a heterogeneous class of industrial and household appliances, devices that combine with each other the possibility of their joint functioning and interaction via wireless communication (HSE, 2019). The technology of the Internet of things is correlated with big data, cloud computing, artificial intelligence and machine learning. The logical connection can be traced as follows: the Internet of things and other sources (data delivery), storage (cloud resources), data processing and analysis using cognitive analytical systems using ML, AL.
Prospects of IoT technology in credit portfolio quality management
The most important problem is getting access to continuous data (client ERP, IOT equipment sensors). Otherwise, the bank receives a model for estimating the probability of default with a default prediction at least on the quarterly range. For more accurate forecasts, you need to solve the problem of monthly forecasting. This is only possible if you have access to the enterprise's financial data and accounting records. To do this, it is necessary to provide information exchange with the bank's system. IoT technologies allow digitizing the
commercial accounting;
technological facilities for processing raw materials and production of finished products;
warehouses (state of raw materials stocks, semi-finished products and finished products);
on supporting industries and systems;
To determine the typical parameters that characterize possible risks, it is needed:
costs (specific consumption rates of raw materials, reagents, energy resources for the production of the target product);
quality (compliance of raw materials and target products with regulations);
condition of critical (non-redundant) equipment (number of failures, limited remaining life);
industrial safety (operation of the emergency shut down, the unblocking keys status, deviation from the norms of technical regulations).
The obtained data allow us to model the normal state and production risks of the level of technological objects, to create a statistical model of the normal state of a technological object under various operating modes for:
predicting its degradation;
monitoring high-frequency, relatively insignificant failures and alarms (for routine analysis);
monitoring rare, but more critical events (which the system should signal immediately).
The data will allow creating a model for a comprehensive assessment of deviations from the normal state of the chain: processing of raw materials – finished products, in case of insufficient number of primary measurements for each technological object/warehouse, etc. Ultimately, this will allow tracking production volumes, raw material consumption, and equipment status in real time. Getting indications on raw material consumption, we can predict the volume of output, predict the volume, we can track the viability of the financial model and compare it with the current level of financial loading and other sources of information. The problem can be solved using correlation and regression analysis (Formula 1, an example of forecasting the volume of output of an industrial enterprise by key cost elements).
(1)
The business architecture for implementing a project for managing the quality of a credit portfolio using IoT technologies is shown on Figure
connecting to the enterprise's real-time database as part of the MES system;
trial performance of real data;
Analytics and fine-tuning of events generation rules based on accumulated statistics.
The forecast budget of the pilot project is set at 39,852 thousand rubles, which consists from developing a data with the ability to process data and to build forecasts, synchronize with existing systems. Purchasing and installing sensors on the client side.
The efficiency of technology implementation for the bank on the example of a project that is implemented on a ready-made cloud architecture of the bank is 8,000 thousand rubles (NPV), the payback period (PBP) is 5.5 years. The internal rate of return (IRR) is 29%. The most important value for the bank is early identification of potential problems of corporate clients based on trigger signals (new source) for a more detailed assessment of the business activities of corporate clients. The effect of timely identified risk levels of borrowers, taking into account detailed and implemented measures with the credit and customer service (for example, timely restructuring) can reduce potential losses by hundreds of millions of rubles and increase marginality due to effectively formed reserves.
The IoT-based approach to managing the quality of the credit portfolio is not applicable to all groups of borrowers, but only for large credit transactions over 1 billion rubles. Or clients with signs of problems with the remaining loan debt in the amount of at least 0.8% of the credit portfolio for an in-depth understanding of the client's activities.
It is necessary to motivate the enterprise to share information with the bank. Solve this problem through flexible pricing when issuing a loan (reduced interest rate). Benefits for the client: 1. Effective control of its production process; 2. Reducing of the operational risks of your production process; 3. Savings on the interest rate by reducing the interest rate on the loan due to reduced credit risks (savings on the bank reserves).
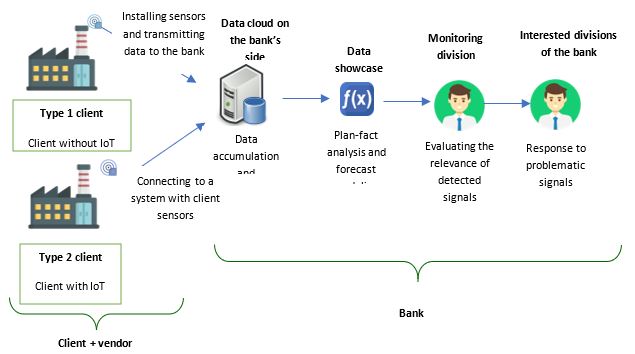
Problems impeding the implementation of IoT technology for credit portfolio diagnostics
External:
2. Client's fear for their data determines a high degree of responsibility to clients for data security and a reason to spend a significant part of the current budget on developing and maintaining a security system.
Internal:
Lack of experience in implementing such projects.
The dichotomy of unit’s objectives when working out distressed signals.
The increasing level of operational risks associated with data security.
Low level of technological literacy of the staff (difficulty in implementing all new methods of work).
Difficulties in finding vendors to implement the project.
Difficulties in selecting and configuring individual sensors for the client for their specific products.
Difficulties in integrating internal IT systems.
Conclusion
The analyzed type of industrial data (IoT) is a valuable source of information for a complex solution of a number of problems for the bank and for the corporate borrower. For an enterprise, this means diagnostics of the technological system, generating statistical data to form a normal state model for assessing and predicting production and financial risks. For the bank, this is an early diagnostic of problematic situations in the borrower's business and an assessment of the adequacy of operating cash flow for debt servicing. The overall economic effect of implementing the system is evaluated positively, taking into account the systematic approach to implementation of a certain group of borrowers (over 1 billion rubles of the remaining loan debt or at least 0.8% of the credit portfolio). For small and medium-sized enterprises, the technology is expensive, especially when implementing sensors based on old technological equipment. Further areas of research are to refine the technological details of production processes to select the correct sensor for data collection and algorithms for data interpretation, taking into account the industry specifics of customers, and to clarify the technical requirements for the analytical system.
Acknowledgments
We express our great gratitude to our relatives and friends for their patience. Special thanks to the management of the Financial University under the Government of the Russian Federation for technical support.
References
- Akwaa-Sekyi, E. K., & Moreno, G. J. (2017). Internal controls and credit risk relationship among banks in Europe. Intangible Capital, 1(1), 25-50.
- Alali, F., Anandarajan, A., & Jiang, W. (2012). The effect of corporate governance on firm's credit ratings: Further evidence using governance score in the United States. Accounting and Finance, 52(2), 291-312.
- Ali, S., Liu, B., & Su, J. J. (2018). Does corporate governance quality affect default risk? The role of growth opportunities and stock liquidity. International Review of Economics & Finance, 58, 422-448.
- Ames, D. A., Hines, C. S., & Sankara, J. (2018). Board risk committees: Insurer financial strength ratings and performance. Journal of Accounting and Public Policy, 37(2), 130-145.
- Andries, A. M., & Brown, M. (2017). Credit booms and busts in emerging markets. The role of bank governance and risk management. Economics of Transition and Institutional Change, 25(3), 377-437.
- Central Bank of the Russian Federation (2020). Review of the banking sector of the Russian Federation. https://cbr.ru/statistics/bank_sector/review/
- Chen, H.-J., & Lin, K.-T. (2016). How do banks make the trade-offs among risks? The role of corporate governance. Journal of Banking & Finance, 72, 39-69.
- HSE (2019). Digital economy: Global trends and practice of Russian business. https://imi.hse.ru/pr2017_1
- Kolozhvari, E. S., & Bersenev, A. B. (2018). Process approach to the problem assets management in a commercial bank. Transport Business of Russia, 6, 25-29.
- Korkina, V. S., & Kukharev, N. A. (2015). Bank infrastructure for monitoring of the credit and pledge operations. Vestnik of the South Russian State Technical University (Novocherkassk Polytechnic Institute). Series: Socio-Economic Sciences, 4, 40-46.
- Pomulev, A. A. (2020a). Banking sector of Russia: problems and prospects in the face of new challenges. Tenevaya Ekonomika, 4(3), 127-138.
- Pomulev, A. A. (2020b). Problematic aspects of implementing modern technologies in a commercial bank. Russian Economic Internet Magazine, 2, 46.
- Pomulev, A. A., & Kalmykov, V. V. (2021). Methodological aspects of credit portfolio management in financing innovative projects. In S. Ashmarina, V. Mantulenko, M. Vochozka (Eds.), Engineering Economics: Decisions and Solutions from Eurasian Perspective. Lecture Notes in Networks and Systems, 139 (pp. 372-383). Springer.
Copyright information
This work is licensed under a Creative Commons Attribution-NonCommercial-NoDerivatives 4.0 International License.
About this article
Publication Date
30 April 2021
Article Doi
eBook ISBN
978-1-80296-105-8
Publisher
European Publisher
Volume
106
Print ISBN (optional)
-
Edition Number
1st Edition
Pages
1-1875
Subjects
Socio-economic development, digital economy, management, public administration
Cite this article as:
Pomulev, А. A., Bogatyrev, S. Y., & syushkova@fa.ru, S. D. Y. (2021). Modern Technologies In Managing Quality Of Commercial Bank Credit Portfolio. In S. I. Ashmarina, V. V. Mantulenko, M. I. Inozemtsev, & E. L. Sidorenko (Eds.), Global Challenges and Prospects of The Modern Economic Development, vol 106. European Proceedings of Social and Behavioural Sciences (pp. 345-353). European Publisher. https://doi.org/10.15405/epsbs.2021.04.02.42