Abstract
For Russia, an effective foreign economic activity could turn into one of the major drivers of economic growth alongside with investments in fixed capital and human capital. Being an integral part of foreign economic activity, export represents the way to raise money for Russian budget. It indicates the state of the country's economy and needs constant monitoring of the main trends and dynamics of its development for timely adjustment of foreign economic policy. In this paper, econometric models of export of goods by time series in monthly dynamics are constructed. At the stage of preliminary analysis, time series of dependent and independent variables were studied for the seasonal component and the order of integration. To account for structural shifts caused by economic crises, dummy variables were included in the model. The hypothesis of structural instability in the export time series was tested using the Chow test. Based on the modeling results, a high degree of dependence of exports on the dollar exchange rate and prices for energy resources was confirmed. Retail trade turnover, the volume of paid services, and the unemployment rate also have a significant impact on the export of goods. With the constructed models, the forecast for exports of goods with seasonal adjustments for December 2020 and January 2021 was made. The method applied in this paper allows both identifying factors that affect the export of goods and its elements, and taking into account possible structural shifts associated with economic, political crises and pandemic.
Keywords: Export of goodseconometric modelforecastingstructural shifttime series
Introduction
Undoubtedly the year 2020 will go down in the world history as the year that began with the collapse in oil prices and continued with the coronavirus pandemic. There was a slowdown or decline in the growth of production and foreign trade, and an increase in unemployment in the world economic system. Many small businesses are on the verge of bankruptcy. States have to allocate huge amounts of money to fight the pandemic. Anecdotal evidence suggests that the degree of the socio-economic downturn in Russia due to the coronavirus will be higher than during the 2008 crisis. According to Aganbegyan’s (2020) forecast, Russia's GDP will decrease by 7-8 % in 2020 due to the coronavirus pandemic and reduced revenues from the oil and gas business. In the current circumstances, Russia needs new sources for economic recovery.
Along with investments in fixed capital and human capital, effective foreign economic activity can become the main driver of our country's economic growth. Export as an integral part of foreign economic activity has long been one of the main ways to raise money for Russian budget. After the introduction of sanctions by the United States and European countries and counter sanctions by the Russian Federation in 2014, our country's export policy changed (Vorotnikov et al., 2019). The volume of exports to Ukraine and other countries has significantly decreased. The last oil crisis, which began in March 2020 and was accompanied with the pandemic, brought down the world oil prices and significantly reduced the demand for this commodity. For Russian exports, whose distinctive feature is the commodity dependence, this has caused significant changes in both the structure and dynamics. The Russian economy, which is dependent on world energy prices, needs anti-crisis measures. Currently to effectively implement anti-crisis policy, it is necessary to constantly monitor the state of Russia's foreign economic activity in general and exports, in particular, the main trends and dynamics of its development.
This study offers an econometric model for exports of goods dependence on various socio-economic indicators. The structural shifts caused by economic crises and the features of modern sanctions policy are taken into account. Research, modeling and forecasting of indicators of foreign economic activity is carried out with the application of various econometric and statistical methods.
When studying foreign trade turnover statistical methods such as cluster analysis (Sukhanova & Shirnaeva, 2012), factor analysis (Glebkova & Kachanova, 2015), index method (Gnidchenko, 2020), and others are used. Lapin (2016) applied linear and nonlinear regression equations. The demand for import is explored in (Suleymanova, 2018) with a vector error correction model (VEC). Shirnaeva (2020) uses autoregression model and integrated moving average ARIMA model.
A number of econometric models are developed specifically for the research and forecasting of foreign trade turnover. Gravity models are used in many papers to study exports (Dong & Truong, 2020; Keogh, 2019). In particular, in (Skvarciany et al., 2020) the gravity models are used in conjunction with the grouping method. Some papers use elements of simulation modelling in combination with econometric methods for studying foreign trade turnover (Bräuning & Koopman, 2020).
Problem Statement
One of the problems of the Russian export policy is related to its commodity orientation. It is known that the export of oil and gas products is the main source of replenishment of the Federal budget (more than 46% are revenues from the export of hydrocarbons). Thus, the Russian economy is constantly significantly dependent on the demand and price for a limited range of goods. Moreover, the value of the dollar exchange rate was not always adequate to price and economic conditions, which had an additional impact on the dynamics of Russian foreign trade turnover. Since 2014 Russia has been facing sanctions from the European Union and the United States. Under the sanctions, the structure of exports also has changed. Currently it is still urgent to analyze and constantly monitor the state of Russian foreign economic activity in general and exports, in particular, the main trends and dynamics of its development for effective planning of foreign trade.
Research Questions
Thus, the questions that are raised in this paper are as follows.
What are the properties of the time series for exports of goods and their components in the Russian Federation?
What economic indicators do affect the size and dynamics of the RF exports of goods?
Have the economic crises and sanctions affected the volume of exports of goods?
Can the export model take into account the structural shift caused by these factors?
What are the properties of the export models built in the work?
Are the constructed models suitable for analysis and forecasting?
What conclusions can be drawn from the results of forecasting?
Purpose of the Study
So far, there has been little agreement on a generally accepted methodology for analyzing and forecasting foreign economic activity of one particular country. Big companies and various economic organizations (the World Trade Organization, the International Monetary Fund, etc.) develop their own methods and models. To study the indicators of foreign economic activity, expert assessments, extrapolation methods, and econometric methods are traditionally used. The latter have proven to be effective and reliable for analyzing various macroeconomic processes. These methods allow taking into account the specifics of Russian exports, their dependence on socio-economic and political factors, and the instability of their structure when modeling and forecasting.
The purpose of this study is to construct an econometric model of exports of goods and its components (exports of goods to the countries of Commonwealth of Independent States (CIS) and non-CIS countries). The structural changes caused by the global economic crises and the specific features of modern sanctions policy should be taken into account. Then, using the constructed model, a forecast of export values for the following time periods should be made.
Research Methods
The approach to empirical research adopted for this study was the combination of econometric methods for analyzing, modeling, and forecasting time series, methods for studying the stationarity and structure of time series, methods for identifying and eliminating the seasonal component of time series, and methods for evaluating the quality of the forecast.
Export of goods of the Russian Federation (Y1), export of goods to non-CIS countries (Y2) and export of goods to CIS countries (Y3) was considered as dependent variables of the study. Various socio-economic indicators were considered as independent variables, grouped into the following clusters: industrial production index and production indices by the activity type (Х1, Х21 - Х25); transport turnover and construction indicators (Х2 - Х4); exchange rate indicators (Х5, Х6); indicators for retail trade turnover and its types (Х7 - Х9); volume of paid services (Х10); price indicators (Х11, Х15, Х16); average producer prices for energy resources and refined products (Х17 - Х20); social sphere indicators (labor market of the Russian Federation, wages) (Х12 - Х14).
All the indicators under study are time series in monthly dynamics (Short-Term Economic Indicators of the Russian Federation, 2020). Most of the indicators were analyzed for the period from January 1999 to July 2020. The exception was the industrial production indicator and production indicators by the type of activity (Х1, Х21 - Х25). Due to changes in the calculation methodology, data on these indicators are available only since 2015. Excel and Gretl packages were used for calculations.
Findings
Commodity focus, dependence on the world oil prices and the dollar exchange rate affected the dynamics and structure of Russian exports. Figure
Since 2008, there have been sharp fluctuations in the value of exports with the lowest values in February 2009 and January 2016. The drop in the value of exports during these periods, due to the global economic crises, the sanctions by the United States and the European Union, as well as the counter sanctions by Russia, caused changes in the structure of exports of Russian goods during these periods. The graph also shows a decrease in export volumes that began in April 2020. This downward trend could be explained by the collapse in oil prices and the beginning of the pandemic.
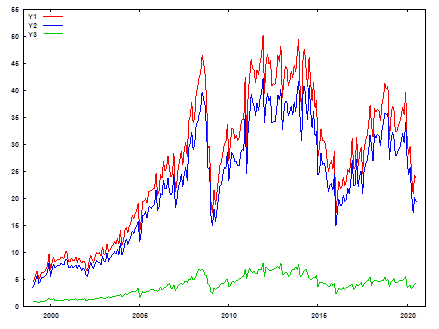
Source: authors based on Federal State Statistic Service (2020).
Comparing the volume of exports in 2013 and in 2019 (Figure
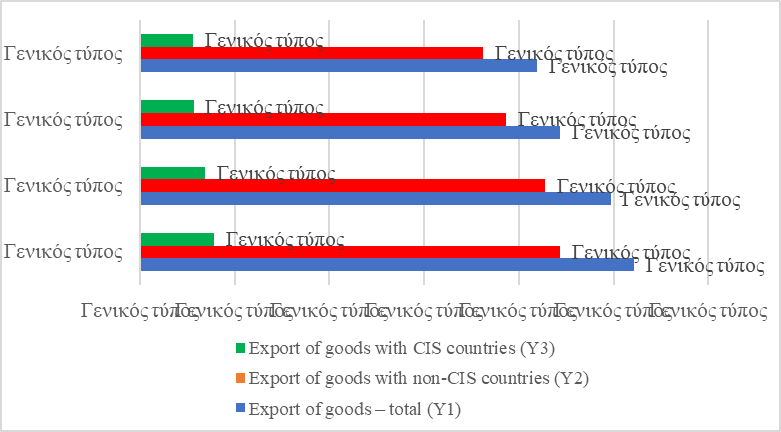
Source: authors based on Federal State Statistic Service (2020).
A preliminary analysis of the time series of the variables both dependent and independent comprised the following steps:
A study of the seasonal component. The conclusion about the presence of a seasonal component was made based on the analysis of autocorrelation and partial autocorrelation functions (AKF and PAKF). For further work, the seasonal component has been detected and eliminated.
The order of integration was determined using the extended Dickey–Fuller test (extended ADF-test). The test results show that the time series of all the variables considered are first-order integrated.
Multiple regression models were then constructed for each of the dependent variable (1)-(3) ( :
(1)
(2)
(3)
The quality indicators of the constructed models (the determination coefficient
The constructed models are statistically significant at the 5% significance level, but not all estimates are statistically significant in models (2) and (3). According to the results of the extended
To test the hypothesis of structural changes in the time series of dependent variables, the Chow test for structural stability was performed (Ghilagaber, 2004). The test was performed for
Thus, in February 2009 and January 2016, the impact of structural changes is significant.
In order to account for significant structural shifts in export models, the set of independent variables was supplemented with the following dummy variables:
,
.
Next, the simulation was carried out taking into account the dummy variables Z1 and Z2. The following models for the RF export of goods and its components were obtained:
(4)
(5)
(6)
Table
Comparing the data in Tables
Thus, the export model and its components include the following independent variables:
Based on the modeling results, a high dependence of Russian exports on the dollar exchange rate and prices for energy resources and refined products was confirmed. Retail trade turnover, the volume of paid services, and the unemployment rate also significantly impact Russian exports.
The predicted values of the dependent variables
Source: authors (interval estimations for the predicted values of the corresponding variables with reliability 95% are given in brackets).
Mean absolute percentage error (
According to the forecast results, if everything else is a constant, a further decrease in export volumes and its components is expected in December 2020 to January 2021.
Conclusion
The new sources for the revival of Russian economy are needed in present-day conditions. Effective foreign economic activity can become one of the drivers of the recovery of both the Russian and global economy. Export is an integral part of foreign trade turnover. It is considered to be an indicator of the country's economy. Therefore, constant monitoring of the main trends and dynamics of its development for timely adjustment of foreign economic policy are needed. An econometric model can be one of those monitoring tools. The models for export of goods constructed in this study have good predictive properties and an insignificant forecast error. The empirical findings in this study, which are based on the modeling results, confirm high dependence of Russian exports on the dollar exchange rate and prices for energy resources and refined products. Moreover, it was documented that retail trade turnover, the volume of paid services, and the unemployment rate also have a significant impact on the export of goods. The present study provides the method that allows not only identifying factors that affect the export of goods and its components, but also taking into account possible structural shifts associated with economic, political crises and pandemic.
References
- Aganbegyan, A. G. (2020). Suggestions on socioeconomic development in the context of the current crisis. Economic Recovery of Russia, 2(64), 33-44.
- Bräuning, F., & Koopman, S.J. (2020). The dynamic factor network model with an application to international trade. Journal of Econometrics, 216(2), 494-515.
- Dong, C.V., & Truong, H.Q. (2020). The determinants of creative goods exports: Evidence from Vietnam. Journal of Cultural Economics, 44(2), 281-308.
- Federal State Statistic Service (2020). Short-term economic indicators. https://gks.ru/compendium/document/50802
- Ghilagaber, G. (2004). Another look at Chow's test for the equality of two heteroscedastic regression models. Quality & Quantity, 38(1), 81-93.
- Glebkova, I. Y., & Kachanova, N. N. (2015). Factor analysis of foreign trade indicators. Eurasian Union of Scientists, 2(11), 56-59.
- Gnidchenko, A. A. (2020). Measuring quality-based competitiveness: What's wrong with high-tech exports? Voprosy Economiki, 6, 80-103.
- Keogh, G. (2019). A gravity model analysis of Irish merchandise goods exports under Brexit. Journal of the Statistical and Social Inquiry Society of Ireland, 48, 41-70.
- Lapin, A. V. (2016). Construction of econometric models and analysis of export and import factors in Saint Petersburg. Young Scientist, 13(117), 44-46.
- Shirnaeva, S.Yu. (2020). Econometric modeling and forecasting indicators of export of goods of the Russian Federation. Fundamental Research, 6, 172-177.
- Skvarciany, V., Jureviciene, D., & Vidziunaite, S. (2020). The impact of Russia's import embargo on the EU countries' exports. Economies, 8(3), 62.
- Sukhanova, E. I., & Shirnaeva, S. Y. (2012). Typologization of indices that reflect the stabilization processes of the Russian economy, according to the criteria of stability. Vestnik of Samara State University of Economics, 5(91), 103-109.
- Sukhanova, E. I., Shirnaeva, S. Y., & Repina, E. G. (2019). Statistical research and modeling of retail turnover in the Russian Federation. In V. Mantulenko (Ed.), Eurasia: Sustainable Development, Security, Cooperation – 2019, SHS Web of Conferences, 71 (02004). Les Ulis: EDP Sciences.
- Suleymanova, M. Z. (2018). Analysis of the demand function for aggregated imports in Russia. In V. S. Mkhitaryan, M. Y. Arkhipova, L. A. Rodionova, V. P. Sirotin, & N. V. Zvezdina (Eds.), Proceedings of the 9th International Scientific and Practical Conference of Students and Postgraduates «Statistical Methods of Analysis of Economy and Society» (рр. 232-234). HSE University.
- Vorotnikov, I. L., Glukhova, M. I., Sukhanova, I. F., Lyavina, M. Y., & Petrov, K. A. (2019). Economic sanction and import substitution. Entrepreneurship and Sustainability Issues, 6(4), 1872-1883.
Copyright information
This work is licensed under a Creative Commons Attribution-NonCommercial-NoDerivatives 4.0 International License.
About this article
Publication Date
30 April 2021
Article Doi
eBook ISBN
978-1-80296-105-8
Publisher
European Publisher
Volume
106
Print ISBN (optional)
-
Edition Number
1st Edition
Pages
1-1875
Subjects
Socio-economic development, digital economy, management, public administration
Cite this article as:
Sukhanova, E. I., & Shirnaeva, S. Y. (2021). Modeling And Forecasting Of Russian Foreign Economic Activity Indicators: Econometric Approach. In S. I. Ashmarina, V. V. Mantulenko, M. I. Inozemtsev, & E. L. Sidorenko (Eds.), Global Challenges and Prospects of The Modern Economic Development, vol 106. European Proceedings of Social and Behavioural Sciences (pp. 1268-1276). European Publisher. https://doi.org/10.15405/epsbs.2021.04.02.150