Abstract
The current trend of economic development is the formation of a digital economy, in which value added is no longer a physical product and there is an active blurring of the lines between the physical and virtual world. In this regard, there is a need to rethink practical and scientific approaches to the organization and management of logistics activities of all market participants, to form a roadmap for the development of each business entity in the new realities, to identify effective tools and technologies that can become drivers of enterprise development in the near and long term. In this regard, it becomes relevant to study the directions of digitalization of the logistics supply chain, which ensures the flow of flow processes and moves material flows in time and space, and to determine the tools for managing it. The purpose of the article is to develop theoretical provisions on the application of the process mining technology and determine its potential capabilities in decision-making in the field of supply chain management, which will determine scientific and methodological approaches to the accumulation and systematization of data from business objects as participants in the supply chain and the possibility of applying the knowledge found in the field of logistics management. As a result of the study, the following main conclusions were obtained: the main concepts are formed and the problems that occur in the mining process when managing logistics supply chains in the conditions of digitalization are identified.
Keywords: Data miningdigital economyintelligent supply chain managementlogisticsprocess mining
Introduction
Changes taking place in the modern economy affect different aspects of the activities of absolutely every market participant. Logistics activities are no exception, as they create the basis for making managerial decisions in the context of the need to comply with the principle of rationality and efficiency of its organization. As a link between production and the sphere of circulation, the postulate of its development is a quick response to all dynamic changes in the market space. The economy today is characterized by a combination of different technologies that can blur spatial and temporal boundaries and provide better quality of customer satisfaction and service. As part of the research on the transformation of economic relations, it is noted that the source of long-term economic growth in modern conditions is the digitalization of the economy. The digital economy is a new paradigm for accelerated economic development. The digital economy is transforming the business model, goods and services produced, and creating new criteria and parameters for evaluating the performance of companies. In the digital economy, a necessary condition for interaction is the strict integration of business processes and the active use of end-to-end technologies that ensure transparency of business, equal participation in management decisions and the ability to track the flow of flow processes in real time (Evtodieva et al., 2020).
Currently, States around the world have formed a set of strategic initiatives and tactical actions to maintain and develop the digital economy. GDP growth due to digitalization in a number of the world's leading developed economies will sharply increase by 1.8%, and the GDP of developing countries – by 3.4% by 2025. One of the economic areas that actively uses digital technologies and innovations is logistics. The logistics sector that has the third largest potential for automation among all sectors of the economy. The reasons for this are, on the one hand, significant logistics costs that negatively affect the cost parameters of the material flow and the desire to optimize them, on the other hand, the requirements of consumers for high - quality service and reduced service time. As a result, it becomes urgent to analyze the state of the actual logistics business process in real time based on actual data in order to identify bottlenecks and hidden problems and make management decisions to eliminate them. In this regard, the importance of the research is to determine the characteristics, advantages and possibilities of using process analytics in the practice of supply chain management and logistics, as well as the development of the theory of logistics organization in modern conditions in order to minimize errors in making management decisions and optimize costs. The results can be used for research in the field of logistics at the firm level and the regional economy.
Problem Statement
The paradigm of supply chain management in the digital economy corresponds to current trends in business development and is a widely discussed problem in scientific circles. Researchers from both Russian and foreign scientific schools are engaged in research on digitalization of supply chains in modern realities. These studies examine the transformation of logistics supply chains under the influence of digital trends and identify the most important technologies of the digital economy that affect the development of supply chain management systems. Currently, the key to the success and viability of any supply chain is intelligent technologies based on machine learning and artificial intelligence, which provide additional competitive advantages and sustainable development. It should be noted that the supply chain management system in the digital field should be based on adequate structured information that ensures the adoption of both operational and strategic decisions on the main issues of logistics interaction. In this regard, it is relevant to study the issues of Analytics based on big data, which allows not only to obtain systematic information about the current state of affairs, but also to form development forecasts taking into account objectively existing trends and factors of influence and by identifying implicit links between events or phenomena in the field of logistics. A promising technology is process Analytics, which allows to manage any business processes by obtaining analytical data of its execution.
Research Questions
There is no single understanding of the category under consideration till nowadays. However, the study of business process analytics in supply chains based on fundamentally new approaches is due to the new economic conditions in which modern supply chains operate. The key objectives of this study are to identify the distinctive features of smart supply chains. The second task is to define the concept of smart supply chain management. The third task is to research and Process mining definition and its’ goals. The fourth task is related to the definition of Smart Supply Chain Management using process mining. These concerns led to the formulation of the purposes of this study.
Purpose of the Study
Process mining is a relatively young research discipline that is positioned between machine learning and data extraction on the one hand, and process modelling and analysis on the other. Currently, this area is not widely sanctified and developed in the scientific literature in relation to logistics and supply chain management. However, it is in this area of activity that process Analytics has a huge potential. In this regard, it becomes important to systematize and generalize theoretical positions, practical knowledge and experience of using Process mining in the supply chain as a decision support technology that allows making changes to business processes based on identified deviations in order to improve them, which formed the purpose and direction of the study. Our study objective was to highlight the potential of Process Mining techniques to increase visibility on processes and therefore lead to improving Supply Chain Management performances. This project highlighted that even though Process Mining techniques and advanced data analytics are very promising in the field of Smart Supply Chain Management, they are not magic tools that can work out of the boy. In this regard, the Process mining technology is a tool that allows you to identify deviations from the "ideal" process by visualizing the real process in the supply chain and, based on the results obtained, make management decisions about making changes to business processes in order to improve them.
Research Methods
In the process of studying the problem of process Analytics and intelligent supply chain management in the digital environment, General scientific and private methods of cognition were used. The use of General scientific methods of cognition allowed us to objectively and comprehensively study the existing conceptual provisions in the field of process Analytics in the field of logistics and supply chain management in the modern economy, systematize and generalize the directions and assess the potential of its application in supporting decision-making in the supply chain in the digital economy. Particular research methods were used at the stage of processing, analysis and generalization of the received scientific information. The research was conducted at a company that engineers and produces mechanical solutions for precisely guided motion in challenging fields of application. The first, Process Discovery, was performed using custom software solutions for data cleaning combined with the Disco software for the process visual representation. Data were gathered from the company internal ERP system, then cleaned, structured and prepared for further processing. Inevitably, this phase of data extraction, cleaning and preparation is the most time-consuming step of any process mining project. Process discovery allowed recreating models depicting multiple machining and assembling processes and elaborate meaningful statistics.
Findings
Being largely globalized over the last decades, Supply Chains are composed of multiple internal and external stakeholders that execute different processes such as ordering, distributing and payments. However, none of these parties has a global understanding and visibility on the end-to-end Supply Chain. In a dynamic and global environment, the constraints are always changing, so deviations from plans occur regularly, which lead to inaccurate forecast, product out-of-stock, shipment delay, etc., and increase costs. Moreover, variability tends to propagate in supply chains across partners, resulting in the famous bullwhip effect (Liu et al., 2007). In addition, the current economic environment is characterized by the acronym VUCA: Volatility, Uncertainty, Complexity and Ambiguity, where, Volatility refers to speed and magnitude of changes, both in the information and in circumstances, as for example prices fluctuation after a natural disaster take a supplier off-line. Uncertainty is the opposite of predictability, a competitor pending products launch that disrupts the business and the market. Complexity is associated to a certain numbers of factors and their relationships in the cause and effect systems and in time, as doing businesses in many countries with unique regulatory environments, tariffs and cultural values. Ambiguity expresses the blur, lack of clarity and opacity in the meanings and events interpretation as well as the ambient conditions such as moving to an immature or emerging market or launching products outside core competencies (Bennett & Lemoine, 2014; Blackburn et al., 2015).
Moreover, according to the literature and multiple case studies, global Supply Chains face several challenges.
Cost containment is being rated number one — rapid wage inflation in previously low-cost labor markets, spikes in commodity prices, or even sudden credit freezes— (Butner, 2010).
Customer intimacy refers to inadequate customer collaboration which leads to high demand variability (Butner, 2010).
Lack of flexibility to respond to change, flexibility being the ability to adapt rapidly to events or issues, run various scenarios, without significantly increasing operational costs.
Globalization and risk management. Even though global Supply Chains exist since the 1980’s, it remains a challenge for companies to operate on such scale and mitigate risks (Elmuti et al., 2013).
Traceability and visibility on stakeholders, flows and processes. Linked to the previous point, the results of globalization is a lack of understanding and control of Supply Chains actors, flows and processes, which sometimes results in duplication of major core activities (Elmuti et al., 2013) and more serious issues like recalls for example.
Data inaccuracy, quality of information captured and lack of integrated IT systems, although more information is available, proportionally less is being effectively captured, managed, analyzed and made available to people who need it (Butner, 2010; Elmuti et al., 2013).
Corporate Social Responsibility. Due to multiple highly mediatized scandals in recent years, stakeholders are paying more attention towards companies’ behaviors in terms of safety, quality and ethics. In this context, enterprises are forced to build sustainable and responsible Supply Chains. The fragmentation of these Supply Chains in scattered geographical areas contribute to their fragility.
Challenged by competitive pressures, and enabled by information technologies (IT), organizations are forming strategic partnerships that share, collaborate, and make collective decisions across the supply chain (SC) (Singh & Teng, 2016). Therefore, in order to remain competitive and to address those challenges, companies must develop an agile and smart Supply Chain Management. Agility implies rapid strategic and operational adaptation to large scale, unpredictable changes in the business environment focusing upon eliminating the barriers to quick response (Elmuti et al., 2013). With regards to Smart Supply Chain, it has been defined as the new interconnected business system, which extends from isolated, local, and single-company applications to supply chain wide systematic smart implementations. The smart supply chain would possess features such as IoT, smart machines, and intelligent infrastructure, and capabilities such as interconnectivity, fully enabling data collection and real-time communication across all supply chain stages, intelligent decision making, and efficient and responsive processes to better serve customers (Wu et al., 2016). This definition suggests that a Smart Supply Chain Management is driven by advanced data analytics where data are captured and analyzed throughout the entire value chain. Although, Amazon has been recently mentioned several times in the literature for their use of big data analytics in order to predict what customers will buy before they actually placed their purchase order (Malik et al., 2018).
Data mining is the process of finding the patterns, associations or relationships among data using various analytical techniques. There is no single understanding of the category under consideration. Quite often, along with the term "Data Mining", you can find a synonym for "knowledge discovery in databases", the emergence of which is explained by new features that appear in data processing tools and methods. The most detailed understanding of Data mining is as follows: the process of finding unknown and useful interpretations of knowledge in a database that form the basis for making management decisions. It follows that using Data mining, you can identify non-obvious relationships that have a certain regularity, which allows you to take them into account when making management decisions and achieve better results. Using Data mining, you can define five standard patterns that allow you to logically identify events related to each other (Association), determine the presence of their time dependence (sequence), identify signs of belonging to a single class of objects (classification), identify various homogeneous groups of data (clustering), and determine possible scenarios for object actions in future periods (forecasting) (Figure
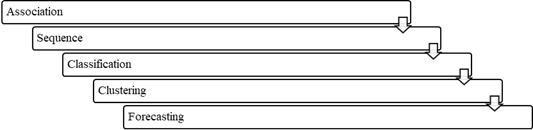
Often, data mining techniques involve the creation of a model, so that the obtained results will become useful information or knowledge. One of the goal of this type of analytics is to accurately project what will happen in the future and provide reasons as to why it may happen (Wang et al., 2016). Grounded on data mining, Process Mining enables predictive analytics, which involves the use of mathematical algorithms and programming, to discover explanatory and predictive patterns within process-related data. The principle of Process Mining is based on the understanding that if a business process is performed in a particular information system, then based on the" traces " of its execution, you can restore the actual algorithm of the process in a form that is convenient for subsequent analysis. This makes it possible to monitor the state of business processes in real time based on actual data, rather than information collected manually and having a subjective nature. Thus, Process Mining seems to be the ideal solution to address the Supply Chain challenges mentioned above. The next section introduces the main concepts and challenges in Process Mining; the following one shows how process mining is applied to end-to-end supply chain: the Smart Supply Chain Management.
Process mining definition and goals. With the term “Process mining” researchers englobe various techniques able to discover, monitor and improve real processes by extracting knowledge from event logs that are becoming more and more available in today’s information systems (Van Der Aalst, 2011).
Process mining final goal is to improve operational performance and monitor business core processes by reducing or eliminating time waste, resource waste, unnecessary costs, bottlenecks and errors using information automatically generated by an information system. A process mining project can be very concrete or more abstract. For instances, a concrete project would aim at achieving a cost reduction of 10% for some given processes and a more abstract one would aim at obtaining valuable insights regarding the performance of several processes allowing the creation of valuable KPIs (key performance indicators).
Process mining is grounded on the availability to a huge quantity of event logs representing a process and its evolution combined with the utilization of data mining techniques able to feed onto this lager amount of information. In order to be applied, process mining techniques assume 1) that a process generates logs in a sequential fashion, 2) that it is possible to link a log with a specific activity and 3) that it is possible to affect an activity to a specific process instance. Nevertheless, low quality or missing information in just one step of the process can easily led to the introduction of heuristics and approximations, finally leading to a rapid loss in the model quality. The main challenges and contemporary research questions in process mining are mainly related to the quality and relevance of the log event data, the technical issues for storage and real-time response, the complexity and scalability of the process model, the quality criteria of the targeted predictive model, and the temporal variation of the event observations.
In literature, researchers have classified process mining into three types: discovery, conformance checking and enhancement (Van Der Aalst, 2011). In process discovery, the event logs provided as input are analyzed in order to generate a process model without any a priori information. Multiple approaches and algorithms to discover a process exist from computational solutions as the alpha-miner algorithm presented in the seminal paper of Van der Aalst and its improvements, to artificial intelligence models such as heuristic or region-based mining, or genetic mining based algorithms.
The value of the analysis of a process is directly correlated to the quality of the models used for such analysis. Conformance checking deals with quantifying if a model is an appropriate representation of the real process underlying the data used to create the model. A typical conformance checking approach identifies conform or deviant behaviors by replaying event logs and checking if the created model is compliant to such data. It is important to notice that perfect compliance between a model and the data does not imply compliance with the process that we want to model. In fact such a model is often too generic or trivial that it has little or no use for the understanding of a process. Process enhancement aims at improving or extending an existing processor process model. Mining event logs or applying advanced AI techniques can make emerge knowledge that was not previously captured by the model. For instances, information about resources can be used to discover roles and shared pattern of activities of group of people. In addition, process enhancement is not restricted to offline analysis and can also be used for predictions and recommendations at runtime. Real-time predictive analytics supporting decision taking belong to this category. At the control flow level, the enhancement consists in forecasting the behavior of the traces under execution so as to predict the expected completion or remaining time, or recommend users the best next operation in order to optimize a process.
Smart Supply Chain Management using process mining. By applying the three types of Process Mining – process discovery, conformance checking and enhancement - to supply chain management allows increasing the visibility on end-to-end Supply Chain, support decisions making and ultimately improve overall Supply Chain performances (Malik et al., 2018; Van Der Aalst, 2011). Process Mining techniques offer a means to rigorously check compliance and verify the validity and reliability of information about an organization’s core processes, making sure that companies operate within their boundaries (Van Der Aalst, 2011). Additionally, Process Mining allows bringing out deviations between real and planned flows. As in a Lean Management approach, Process Mining aims at pointing out wastes within a company process such as extra-processing time, unnecessary movements of materials, bottlenecks, reworks, etc. Process discovery applied to a supply chain process can be associated to a kind of dynamic Value Stream Mapping. Process discovery could provide a clear picture on companies operations and helps breaking down silos within departments and overall Supply Chains. Furthermore, the knowledge discovery from the historical data captured allows better efficiency and forecast accuracy (Lau et al., 2009). Going further, the use of Process Mining techniques combined with Artificial Intelligence enable companies to proceed with predictive analysis (maintenance, demand...) and even predict non planned events (Liu et al., 2007). Ultimately, it can lead to a dynamic Supply Chain performance management by linking KPI dashboards with Process Mining techniques. Finally, big data analytics helps build resilience in Supply Chain networks for sustainability.
A recent exploratory applied project on Process Mining conducted by Haute Ecole Arc in Neuchâtel, Switzerland corroborates this statement. Our study objective was to highlight the potential of Process Mining techniques to increase visibility on processes and therefore lead to improving Supply Chain Management performances. The research was conducted at a company that engineers and produces mechanical solutions for precisely guided motion in challenging fields of application. The first two types of Process Mining defined by Van Der Aalst (2011) were applied. The first, Process Discovery, was performed using custom software solutions for data cleaning combined with the Disco software for the process visual representation. Data were gathered from the company internal ERP system, then cleaned, structured and prepared for further processing. Inevitably, this phase of data extraction, cleaning and preparation is the most time-consuming step of any process mining project. In fact, due to the variability on the sources of log data, such initial steps remain highly manual operations in most of the cases. In addition, except of trivial data manipulations and filtering, those initial steps require supply chain knowledge in order to be correctly applied and reduce noise. Process discovery allowed recreating models depicting multiple machining and assembling processes and elaborate meaningful statistics. Then, the second step was Conformance Checking. Jointly with process mining techniques, a detailed mapping of the processes was manually drawn based field observations. Such results was then compared to the outcomes from the first step (Processes Discovery). Field observations provide a static representation of a model where automatic Process Discovery is a dynamic process. However, if Process Discovery can show that data linked to some actions are missing, field observations rapidly provide an insight on the reason why such data are missing. For this project, this kind of conformance checking allowed analysts to rapidly highlight deviations between the model and the actual process. Firstly, data expected to be collected were missing from the ERP system. In addition, some intermediate activities were captured in a different information system and only aggregate, episodic views were accessible to the decision makers and process mining tools. Such issues made impossible the creation an end-to-end faithful process model. However, the combination of the two actions considerably speeded up the understanding of the process in the first phases of process modeling highlighting the required steps to improve the supply chain management.
This project highlighted that even though Process Mining techniques and advanced data analytics are very promising in the field of Smart Supply Chain Management, they are not magic tools that can work out of the boy. Data are the key. To be applicable, enterprises need to collect data regularly and the number of records should be significant. As stated before, process exploration techniques assume that minimal requirements about data quality are satisfied: a process mast generates log data sequentially (ideally with a precise timestamp) and that it must be possible to link such information to a specific activity and the activity to a specific process instance. If these requirements are not respected applying process mining techniques can only bring mitigated, if any, results.
Conclusion
Changes taking place in the modern economy affect different aspects of the activities of absolutely every market participant. Logistics activities are no exception, as they create the basis for making managerial decisions in the context of the need to comply with the principle of rationality and efficiency of its organization. The rapid pace of management decision-making in supply chains requires a large array of high-quality information that takes into account explicit and implicit system connections and allows you to improve their efficiency. In addition, it is necessary to ensure the transparency of the supply chain and provide an opportunity for all its participants to monitor business processes and make necessary adjustments to them in order to quickly respond to the dynamism of the market environment and consumer requests. In this regard, the Process mining technology is a tool that allows you to identify deviations from the "ideal" process by visualizing the real process in the supply chain and, based on the results obtained, make management decisions about making changes to business processes in order to improve them. Process Mining as a technology to support decision making in the supply chain in the digital economy allows:
–audit internal and external business processes of the entire chain and each individual participant;
–choose from alternative options the most effective solution with minimal risk for all participants;
–analyze in detail the complex chain of approvals when developing a solution and ensure transparency of business processes;
–reduce time and financial losses due to optimal alignment of the trajectory of movement of logistics flows;
–use mathematical algorithms and programming to perform predictive Analytics and determine the strategic direction of the supply chain development and the order of required actions.
Acknowledgments
This paper goes within the framework of scientific and academic cooperation on the project "Smart Supply Chain" between the HE ARC, Neuchatel (Switzerland) and Samara state University of Economics, Samara (Russia). The authors thank administration of organizations and all the members participated in the project.
References
- Bennett, N., & Lemoine, G. J. (2014). What VUCA really means for you. Harvard Business Review. https://hbr.org/2014/01/what-vuca-really-means-for-you
- Blackburn, R., Lurz, K., Priese, B., Göb, R., & Darkow, I.L. (2015). A predictive analytics approach for demand forecasting in the process industry. International Transactions in Operational Research, 22(3), 407-428.
- Butner, K. (2010). The smarter supply chain of the future. Strategy and Leadership, 38(1), 22-31. DOI:
- Elmuti, D., Khoury, G., Omran, O., & Abou-Zaid, A. S. (2013). Challenges and opportunities of health care supply chain management in the United States. Health Marketing Quarterly, 30(2), 128-143. DOI:
- Evtodieva, T. E., Chernovа, D. V., Ivanova, N. V., & Wirth, J. (2020). The internet of things: Possibilities of application in intelligent supply chain management. In S. Ashmarina, A. Mesquita & M. Vochozka (Eds.), Digital Transformation of the Economy: Challenges, Trends and New Opportunities. Advances in Intelligent Systems and Computing, 908 (pp. 395-403). Springer. DOI:
- Lau, H. C. W., Ho, G. T. S., Zhao, Y., & Chung, N. S. H. (2009). Development of a process mining system for supporting knowledge discovery in a supply chain network. International Journal of Production Economics, 122(1), 176-187.
- Liu, R., Kumar, A., & van der Aalst, W. (2007). A formal modeling approach for supply chain event management. Decision Support Systems, 43(3), 761-778. DOI:
- Malik, M. M., Abdallah, S., & Ala’raj, M. (2018). Data mining and predictive analytics applications for the delivery of healthcare services: A systematic literature review. Annals of Operations Research, 270, 287-312. DOI:
- Singh, A., & Teng, J. T. C. (2016). Enhancing supply chain outcomes through information technology and trust. Computers in Human Behavior, 54, 290-300. DOI:
- Van Der Aalst, W. (2011). Process mining: Discovery, conformance and enhancement of business processes (Vol. 2). Springer.
- Wang, G., Gunasekaran, A., Ngai, E. W. T., & Papadopoulos, T. (2016). Big data analytics in logistics and supply chain management: Certain investigations for research and applications. International Journal of Production Economics, 176, 98-110. DOI:
- Wu, L., Yue, X., Jin, A., & Yen, D. C. (2016). Smart supply chain management: A review and implications for future research. International Journal of Logistics Management, 27(2), 395-417. DOI:
Copyright information
This work is licensed under a Creative Commons Attribution-NonCommercial-NoDerivatives 4.0 International License.
About this article
Publication Date
30 April 2021
Article Doi
eBook ISBN
978-1-80296-105-8
Publisher
European Publisher
Volume
106
Print ISBN (optional)
-
Edition Number
1st Edition
Pages
1-1875
Subjects
Socio-economic development, digital economy, management, public administration
Cite this article as:
Carrino, S., Doan, K., Evtodieva, T. E., & Ivanova, N. V. (2021). Process Mining As A Decision-Making Support Technology In The Smart Supply Chains. In S. I. Ashmarina, V. V. Mantulenko, M. I. Inozemtsev, & E. L. Sidorenko (Eds.), Global Challenges and Prospects of The Modern Economic Development, vol 106. European Proceedings of Social and Behavioural Sciences (pp. 877-886). European Publisher. https://doi.org/10.15405/epsbs.2021.04.02.104