Abstract
The Internet of Things (IoT) has enticed a lot of attention from academicians, professionals, and government officials. In fact, it is an essential technology that is driving the Industrial 4.0 revolution. Many governments in the world are capitalizing on the Internet of things in developing their own nations. However, in many emerging countries, like Malaysia, the adoption of the IoT technology is still characterized by slow pace, especially among the small and medium size companies. It is critical and urgent to understand the barriers and drivers of the IoT adoption. This study, therefore proposes a conceptual framework that extends Universal Theory of Acceptance and Use of Technology (UTAUT) to new constructs in order to develop a model that can be more precise and suitable for the IoT assessment. The conceptual model includes constructs like ICT self-efficacy, automation, personal innovativeness. Based on the proposed conceptual framework, propositions were developed. Future research could empirically test the proposed model in order to draw some conclusions about the explanatory power of the suggested theoretical framework.
Keywords: Attitude towards intention to adopt and personal innovativenessautomationICT self-efficacyinternet of thingsuniversal theory of acceptance and use of technology
Introduction
The IoT is a new and evolved technology, which is believed to reach 8.4 billion IoT devices connected and 2 billion USD business opportunities by 2020 ( Cisco, 2017). IoT is driving innovation and new opportunities by bringing all connected “things”, consumers and activities into the digital realm. The connected things or connected devices will allow the consumer to interact with them through standard protocols. With the availability of cost-effective sensors, the proliferation of connected devices is getting a lot of attention. Gregory ( 2015) mentioned that with these new smart connected devices and the fast and affordable broadband connectivity, enterprises can offer new services to new markets by creating digital ecosystems (channels and supply chain) which will generate a new stream of revenues.
In addition, as per Gregory ( 2015), consumer adoption of IoT devices is expected to expand rapidly in wearable and connected homes as well as the business enterprise. IoT technologies can be used in many applications and segments like retail, manufacturing, agriculture and so forth. The key applications of the IoT will be happening in the smart home-related applications including home automation, security, and smart appliances ( Torchia, 2018). Torchia ( 2018) highlighted in the Telecoms and Computing Market Research Reports that the IoT market will be growing rapidly in the next 5 years, which is about 5x the growth rate from $360m (2016) to $1.8B (2021). From unit perspective, the unit devices will exceed 4.6 billion in 2021 and most of them will be in the smart home and manufacturing sectors representing about 80% of the total adoption. Despite the growing importance of Internet of Things, the related studies are in a very early or infancy stage. Most of the previous IT adoption related studies used different theories like TRA ( Fishbein & Ajzen, 1975), DIT ( Rogers, 2003), TPB ( Ajzen, 2011), TAM ( Davis, 1989; Venkatesh & Bala, 2008), and UTAUT ( Venkatesh et al., 2012).
However, due to the changes of environment and the progress of the IT world, TAM and TPB models have been revised and extended. UTAUT was proposed as the latest model, because it is more precise in predicting individual adoption behaviour in technology ( Mitzner et al., 2016). Despite the extensions of these models, researchers are not satisfied with the use of UTAUT model alone to predict the adoption behaviour of a new technology area like Internet of things ( Gangwar et al., 2015; Kim & Garrison, 2009). This means that there is a need for a more comprehensive model that takes into consideration constructs such, as perceived security risk, automation and social influence to the existing UTAUT and TPM models that have been empirically verified by other scholars ( Salimon & Gorondutse, 2018). Hence, the current study will propose a conceptual framework that incorporates UTAUT, TAM and TPB and new constructs to expand our understanding of the adoption of IoT.
Literature review and conceptual framework of IoT adoption
IoT can help organisations by saving operational cost and improving manufacturing productivity. The benefit of using the IoT are well understood, however, not all country adoption rates are the same. Developed countries like US, Japan, North Korea and Singapore score high in IoT adoption, as compared to Malaysia ( Mann & Sahni, 2013). In addition, a lot of IoT related studies in developed countries are based on the generic users or working adults as they have more exposure to the new technology compared to developing country users ( Yau et al., 2016). Thus, there is a need to have the right target group like ICT professionals for the IoT study in the developing country like Malaysia. The next section will present a brief description of the constructs incorporated in the proposed conceptual framework (Figure
ICT self-efficacy
Bandura ( 1997) and Ajzen ( 2011) stated that ICT self-efficacy is a very critical determinant of the decision to use a product. Indeed, a novel technology is usually adopted by a person with a stronger self-efficacy, as he /she are eager to deal with challenges and unfamiliar situations through perseverance and persistence. ICT self-efficacy measures the degree of a person’s ability to perform a specific task in the technology usage ( Venkatesh & Bala, 2008). Past studies have proved that the intention to adopt ICT and self-efficacy are positively related ( Kessler & Martin, 2017; Salimon & Gorondutse, 2018). However, others reported a negative relationship ( Ozturk et al., 2016). These studies have indicated that there is a need for more studies especially within the Malaysian context.
Perceived behavioral control
Ajzen ( 2011) introduces the construct of Perceived Behavioral Control (PBC) to reflect control factors that may motivate or demotivate the people to act. Shim et al. ( 2001) stated that perceived behavioral control should be taken into consideration during ICT research because adopting new technology requires skills, opportunities and resources. In the area of ICT adoption, perceived behavioral control can be defined as the confrontation between internal and external reticence when someone wants to execute certain activity ( Yang & Lee, 2018). Past studies have shown that PBC is positively influenced by the capability to control the internet ( Al-Jabari et al., 2012; Gopi & Ramayah, 2007; Lim et al., 2015). Al-Swidi et al. ( 2012) suggested that the higher the PBC is, the higher the intention is to use internet. However, a number of studies concluded that both constructs are not related. Al-Smadi ( 2012) suggested further research on this are needed.
Perceived usefulness
Performance expectancy is about the consumer’s expectation that a specific technology usage will help in accomplishing certain tasks ( Venkatesh et al., 2012). Researchers agreed that both performance expectancy (UTAUT) and the perceived usefulness (TAM) are quite the same ( Coughlan et al., 2012; Venkatesh et al., 2012). In the context of IoT Kowatsch & Maass ( 2012) concluded that the US consumers find that IoT services are very useful in their daily life. However, in another study, Al-Smadi ( 2012) found a non-significant relationship between both constructs, and then suggested further research in this regard.
Perceived ease of use (PEU)
This construct describes an individual belief about the degree of controlling a system with the minimum effort, especially the technology ( Davis, 1989). In their study, Gao and Bai ( 2014) showed that PEU has a significant impact on IoT adoption. Similarly, Ngoo et al. ( 2018) found that ease of use is related to the online shopping decision among Malaysians. Nonetheless, there are also some scholars who found that there is no relationship of PEOU and attitude. Similarly, other studies confirm the indirect link between PEU and the behaviour of using the technology ( Chau & Hu, 2002; Igbaria et al., 1995).
Perceived security risk (PSR)
The perceived security risk has direct and indirect impacts on intentions, mediated by attitude. The PSR is a key driver of the intention of IT adoption ( Davis et al., 1989). Evidence has shown that consumers do not accept or use technology without first safeguarding the security and the privacy of their transactions ( Susanto et al., 2013; Zhou, 2011).
Roca et al. ( 2009) described security risk as the event of destructing the regular protected system to be unusable by modifying its original data and creating a fraud of it. As Internet of Things is about online connected thing, which can expose the data to hackers and fraudulent ( Kowatsch & Maass, 2012; Yang & Lee, 2018). In the current study, perceived security risk is operationalized as a person’s concern about his or her data being hacked by any criminals or hackers. Such concern prevents people’s intention from adopting the IoT. However, some previous studies, found that PSR is not related to the intention to use ICT, such as online purchase ( Chou & Yutami, 2014). Likewise, Aboobucker ( 2019) concluded that the security risk has a negative influence on online shopping behaviour.
Personal innovativeness (PI)
Consumers’ personality characteristics influence their risk taking behavior ( Blake et al., 2003; Donthu & Garcia, 1999; Limayem et al., 2000). Lu et al. ( 2005) said that the decision of trying something new (as an early technology adopter) is related to the personal innovation character. Individual innovativeness is a potentially an important determinant of new technology adoption.
Moderating role of attitude towards adoption of IoT
Ajzen ( 2011) believed that the attitude towards behavior will influence the result and outcome of personal evaluation positively or negatively ( Lee et al., 2017). Similarly, Zhang and Mao ( 2008) also concluded that the attitude to adopt a novel expertise is a cognitive process, which, affects the behaviour positively or negatively such as IoT ( Yang & Lee, 2018). Some scholars confirm that the decision of accepting new technology is a complex situation, which differs in various scenarios ( Shen & Chiou, 2010). Applying this scenario on IoT, the adoption of new IoT technology will depend on the individual attitude towards the behaviour ( Kessler & Martin, 2017). However, there are also studies that found that the attitude is not related to the adoption intention ( Pires et al., 2004). Besides the direct influence of attitude on intention, it is also believed that the attitude plays a mediating role between the PSR and the decision to adopt novel technology ( Brauner et al., 2017).
Lu et al. ( 2005) also confirmed in their study that PI is positively associated with the attitude to accept a new technology, which will further determine the intention to adopt a novel technology.
Subjective norms (SN)
Subjective norms relates to a person's thinking that a referent group influences his/her decision ( Ajzen, 2011). According to Venkatesh et al. ( 2012) SN is about the perceived effect of family members and friends on the decision of using a technology. Individuals will incorporate people’s opinion, after being influenced by them. Later on, such influence becomes a personal belief ( Patil, 2017). People’s intention can be influenced by the opinions of family, friends, and media. IoT can be viewed as a new innovation system and its adoption can be influenced by mass media and word of mouth. Subjective norms have been found to significantly and positively influence the adoption of new technology in various studies. However, a number of other studies found no relationship with technology adoption ( Pham et al., 2013; Ndubisi & Sinti, 2006).
Automation
Automation is hardly researched among ICT marketing scholars ( e.g. Yang & Lee, 2018). Instead, it is commonly researched in the operation technology (OT) area, such as manufacturing, and logistics ( Parasuraman & Riley, 1997). Ghazizadeh et al. ( 2012) proposed that Automation Acceptance Model (AAM), which is basically the extension of the TAM framework, needs to include automation, so as to perfectly address the ICT and the OT relationship and therefore, complement each other.
Automation is a key function in manufacturing, which replaces human manual work ( Parasuraman & Riley, 1997). It is believed that IoT will replace human intervention. This study defines automation as an IoT implementation that ceases human operation ( Yang & Lee, 2018). With the internet connectivity, machine automation will improve manageability and simplicity to control the systems ( Luor et al., 2015; Salimon & Gorondutse, 2018; Yang & Lee, 2018). The data which can be collected through connectivity makes artificial-intelligence analytics possible for smart manufacturing ( Augusto & Nugent, 2006).
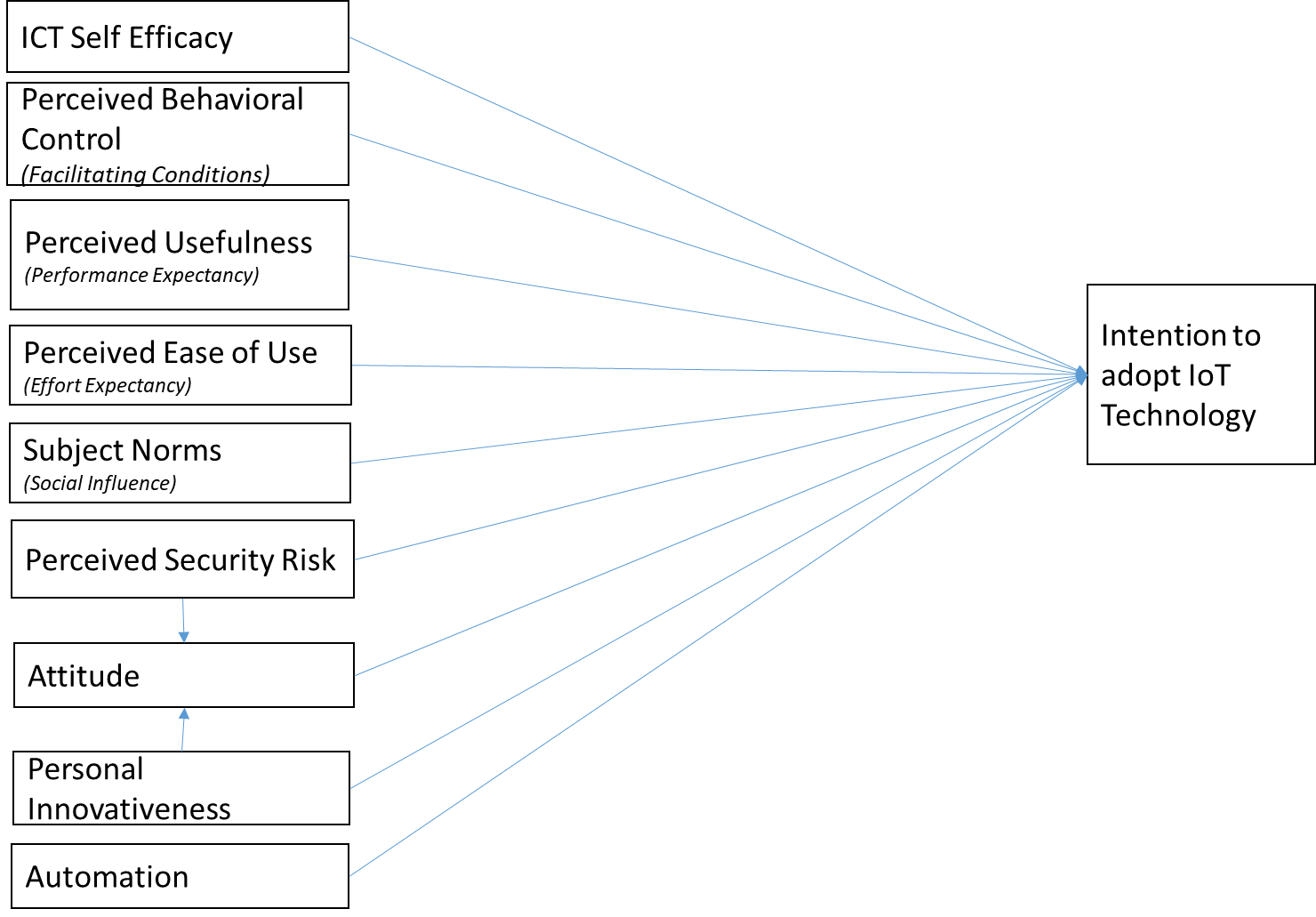
Problem Statement
Past experiences about the human adoption rate of new technology show that enterprises and industrial practitioners needed a deeper understanding of the consumer adoption rate of new technology before committing such huge investment. Over the years, consumers have been able to adopt a new technology but with a slow pace. For instance, Internet adoption takes about 15 years to reach 50M users worldwide ( Mace, 2013). Even if a country strongly promotes IoT-based manufacturing, communication and service industries to create an extended value chain, there are still some obstacles that hinder the adoption of IoT ( Moghavvemi et al., 2011). Many countries funded and supported the research on the IoT, yet the technology adoption is still unsatisfactory as there are risks in the implementation process ( Atzori et al., 2010). Malaysia is among those who are slow in adopting Internet of Things with less than 16.4% ( Asia IoT business platform, 2017). Many government agencies in Malaysia are calling for more detailed studies regarding the barriers and the drivers of Internet of things adoption. In answering this call the current study will attempt to answer the question of: What are the key factors that may affect the adoption of IoT?
Research Questions
Following the above, one of the biggest potential concerns that leads to failure or slow adoption of IoT is related to unfamiliarity of IT professionals with the technology itself, its security and risk concerns, as well as its benefits. The main research questions that the current study attempts to answer is: what are the determinants of Internet of Things adoption?
Purpose of the Study
This study attempts to explore what influences the adoption of IoT. This objective can be achieved by extending the UTAUT developed by Venkatesh and Bala ( 2008). Thus, the main objective of the current study is to find out are the determinants of IoT adoption.
Research Methods
The above conceptual framework and propositions were developed based on detailed literature reviews. The first round, of the past studies review was about the Internet of Things. Papers generally related to the topic were downloaded and screened in order to include only relevant ones. In the second round, articles about the new technology acceptance behaviour and its determinants were searched and filtered. Combining both sets of literature researchers developed a conceptual framework on the adoption of Internet of Things based on nine propositions. The authors used mainly the Scopus and Web of Science databases in searching for studies related to the current research topic ( Wang and Waltman, 2016). In order to guarantee the quality and consistency of the research only the peer-reviewed journals written in English language were included.
Findings
This is an exploratory study. The objective of this research is to develop a conceptual model depicting the determinants of the IoT adoption. It is not the objective of the current study to empirically test the proposed conceptual model. Thus, there are no qualitative or quantitative analysis and hence no findings to be discussed. Future research could empirically test the proposed model in order to draw some conclusions about the explanatory power of the suggested theoretical framework.
Conclusion
The Internet of Things is inevitably going to have a major and critical role in the near future. Many countries, especially emerging ones like Malaysia are slow in adopting the IoT. The study attempts to identify the determinants of IoT acceptance. Based on detailed literature reviews on Internet of Things, new technology acceptance behaviour and its determinants the researchers proposed a conceptual model linking nine predictors with the Internet of Things adoption. The model was built on nine propositions. This is an exploratory study and there were no qualitative or quantitative analyses were carried out. Future research could empirically test the proposed model in order to draw some conclusions about the explanatory power of the suggested theoretical framework.
References
- Aboobucker, I. (2019). Consumers’ perceived security risks in online shopping : A survey study in Sri Lanka. SSRN Electronic Journal, 19(4/1), 10-15. https://doi.org/10.2139/ssrn.3344634
- Ajzen, I. (2011). The theory of planned behaviour: Reactions and reflections. Psychology and Health, 26(9), 1113-1127. https://doi.org/10.1080/08870446.2011.613995
- Al-Jabari, A. M., Othman, N. S., & Kamariah, N. M. N. (2012). Actual online shopping behavior among Jordanian customers. American Journal of Economics, 2(4), 125-129. https://doi.org/10.5923/j.economics.20120001.28
- Al-Smadi, M. O. (2012). Factors affecting adoption of electronic banking : An analysis of the perspectives of banks ’ customers. International Journal of Business and Social Science, 3(17), 294-309.
- Al-Swidi, A. K., Behjati, S., & Shahzad, A. (2012). Antecedents of online purchasing intention among MBA students: The case of University Utara Malaysia using the partial least squares approach. International Journal of Business and Management, 7(15), 35-49. https://doi.org/10.5539/ijbm.v7n15p35
- Asia IoT business platform. (2017). Asia Internet of Things business platform. Retrieved from https://iotbusiness-platform.com/iot-malaysia/
- Atzori, L., Iera, A., & Morabito, G. (2010). The Internet of Things: A survey. Computer Networks, 54(15), 2787-2805. https://doi.org/10.1016/j.comnet.2010.05.010
- Augusto, J. C., & Nugent, C. D. (2006). Smart homes can be smarter. In Augusto J.C., Nugent C.D. (Eds.), Designing smart homes (pp. 1-15). https://doi.org/10.1007/11788485_1
- Bandura, A. (1997). Self-efficacy: The exercise of self-control. Freeman. https://doi.org/10.1007/SpringerReference_223312
- Blake, B. F., Neuendorf, K. A., & Valdiserri, C. M. (2003). Innovativeness and variety of Internet shopping. Internet Research, 13(3), 156-169. https://doi.org/10.1108/10662240310478187
- Brauner, P., van Heek, J., Ziefle, M., Hamdan, N. A., & Borchers, J. (2017). Interactive furniture – evaluation of smart interactive textile interfaces for home environments. In Proceedings of the 2017 ACM International Conference on Interactive Surfaces and Spaces (pp. 151-160). http://doi.acm.org/10.1145/3132272.3134128
- Chau, P. Y. K., & Hu, P. J. (2002). Examining a model of information technology acceptance by individual professionals: An exploratory study. Journal of Management Information Systems, 18(4), 191-229. https://doi.org/10.1080/07421222.2002.11045699
- Chou, J. S., & Yutami, G. A. N. (2014). Smart meter adoption and deployment strategy for residential buildings in Indonesia. Applied Energy, 128(2014), 336-349. https://doi.org/10.1016/j.apenergy.2014.04.083
- Cisco (2017). Industrial edge networking components intelligence service. Retrieved from https://www.cisco.com/c/dam/en_us/solutions/iot/ihs-report.pdf?oid=wprit017016
- Coughlan, T., Brown, M., Mortier, R., Houghton, R. J., Goulden, M., & Lawson, G. (2012). Exploring acceptance and consequences of the Internet of Things in the home. In Proceedings - 2012 IEEE Int. Conf. on Green Computing and Communications, GreenCom 2012, Conf. on Internet of Things, IThings 2012 and Conf. on Cyber, Physical and Social Computing, CPSCom 2012. https://doi.org/10.1109/GreenCom.2012.32
- Davis, F. D. (1989). Perceived usefulness, perceived ease of use, and user acceptance of information technology. MIS Quarterly, 13(3), 319-340. https://doi.org/10.2307/249008
- Davis, F. D., Bagozzi, R. P., & Warshaw, P. R. (1989). User acceptance of computer technology: A comparison of two theoretical models. Management Science, 35(8), 982-1003. https://doi.org/10.1287/mnsc.35.8.982
- Donthu, N., & Garcia, A. (1999). The internet shopper. Journal of Advertising Research, 39(3), 52-58.
- Fishbein, M., & Ajzen, I. (1975). Belief, attitude, intention, and behavior: An introduction to theory and research. Addison-Wesley. https://doi.org/10.1002/cncr.26402
- Gangwar, H., Date, H., & Ramaswamy, R. (2015). Understanding determinants of cloud computing adoption using an integrated TAM-TOE model. Journal of Enterprise Information Management, 28(1), 107-130. https://doi.org/10.1108/JEIM-08-2013-0065
- Gao, L., & Bai, X. (2014). A unified perspective on the factors influencing consumer acceptance of internet of things technology. Asia Pacific Journal of Marketing and Logistics, 26(2), 211-231. https://doi.org/10.1108/APJML-06-2013-0061
- Ghazizadeh, M., Lee, J. D., & Boyle, L. N. (2012). Extending the technology acceptance model to assess automation. Cognition, Technology and Work, 14, 39-49. https://doi.org/10.1007/s10111-011-0194-3
- Gopi, M., & Ramayah, T. (2007). Applicability of theory of planned behavior in predicting intention to trade online: Some evidence from a developing country. International Journal of Emerging Markets, 2(4), 348-360. https://doi.org/10.1108/17468800710824509
- Gregory, J. (2015). The Internet of Things: Revolutionizing the retail industry. Retrieved from Accenture website:https://www.accenture.com/_acnmedia/Accenture/Conversion-Assets/DotCom/Documents/Global/PDF/Dualpub_14/Accenture-The-Internet-Of-Things.pdf
- Igbaria, M., Livari, J., & Maragahh, H. (1995). Why do individuals use computer technology? A Finnish case study. Information and Management, 29(5), 227-238. https://doi.org/10.1016/0378-7206(95)00031-0
- Kessler, S. K., & Martin, M. (2017). How do potential users perceive the adoption of new technologies within the field of Artificial Intelligence and Internet-of-Things? A revision of the UTAUT 2 model using Voice Assistants (Master's thesis). Lund University.
- Kim, S., & Garrison, G. (2009). Investigating mobile wireless technology adoption: An extension of the technology acceptance model. Information Systems Frontiers, 11, 323–333. https://doi.org/10.1007/s10796-008-9073-8
- Kowatsch, T., & Maass, W. (2012). Critical privacy factors of internet of things services: An empirical investigation with domain experts. Lecture Notes in Business Information Processing, 129, 200-211. https://doi.org/10.1007/978-3-642-33244-9_14
- Lee, D. C., Lin, S. H., Ma, H. L., & Wu, D. B. (2017). Use of a modified UTAUT model to investigate the perspectives of internet access device users. International Journal of Human-Computer Interaction, 33(7), 549-564. https://doi.org/10.1080/10447318.2016.1261249
- Lim, Y. J., Osman, A., Abdul Manaf, A. H., & Abdullah, M. S. (2015). The mediating effect of consumers’ purchase intention: A perspective of online shopping behavior among generation Y. Journal of Marketing and Consumer Research Journal, 18, 101-112.
- Limayem, M., Khalifa, M., & Frini, A. (2000). What makes consumers buy from Internet? A longitudinal study of online shopping. IEEE Transactions on Systems, Man, and Cybernetics Part A:Systems and Humans, 30(4), 421-432. https://doi.org/10.1109/3468.852436
- Lu, J., Yao, J. E., & Yu, C. S. (2005). Personal innovativeness, social influences and adoption of wireless Internet services via mobile technology. Journal of Strategic Information Systems, 14(3), 245-268. https://doi.org/10.1016/j.jsis.2005.07.003
- Luor, T., Lu, H. P., Yu, H., & Lu, Y. (2015). Exploring the critical quality attributes and models of smart homes. Maturitas, 82(4), 377-386. https://doi.org/10.1016/j.maturitas.2015.07.025
- Mace, M. (2013). Map the future. http://mapthefuture.mikemace.com/2013/02/excerpts-from-map-future.html
- Mann, B. J. S., & Sahni, S. K. (2013). Profiling adopter categories of internet banking in india: An Empirical study. Vision: The Journal of Business Perspective, 16(4), 283–295. https://doi.org/10.1177/0972262912460187
- Mitzner, T. L., Rogers, W. A., Fisk, A. D., Boot, W. R., Charness, N., Czaja, S. J., & Sharit, J. (2016). Predicting older adults’ perceptions about a computer system designed for seniors. Universal Access in the Information Society, 15, 271-280. https://doi.org/10.1007/s10209-014-0383-y
- Moghavvemi, S., Salleh, N. a. M., Zhao, W., & Hakimian, F. (2011). An empirical study of IT innovation adoption among small and medium sized enterprises in Klang Valley, Malaysia. Social Technologies, 3(2), 243–260.
- Ndubisi, N. O., & Sinti, Q. (2006). Consumer attitudes, system’s characteristics and internet banking adoption in Malaysia. Management Research News, 29(1), 16-27. https://doi.org/10.1108/01409170610645411
- Ngoo, S. B., Kebaili, B., & Belkhamza, Z. (2018). An integrating model of the factor influencing online shopping for the consumer goods. International Academic Journal of Social Sciences, 5, 23-31. 10.9756/IAJSS/V5I2/18100023
- Ozturk, A. B., Bilgihan, A., Nusair, K., & Okumus, F. (2016). What keeps the mobile hotel booking users loyal? Investigating the roles of self-efficacy, compatibility, perceived ease of use, and perceived convenience. International Journal of Information Management, 36(6), 1350-1359. https://doi.org/10.1016/j.ijinfomgt.2016.04.005
- Parasuraman, R., & Riley, V. (1997). Humans and automation: Use, misuse, disuse, abuse. Human factors: The Journal of the Human Factors and Ergonomics Society, 39(2), 230–253. https://doi.org/10.1518/001872097778543886
- Patil, K. (2017). Retail adoption of Internet of Things: Applying TAM model. In International Conference on Computing, Analytics and Security Trends, CAST 2016 (pp. 404-409). https://doi.org/10.1109/CAST.2016.7915003
- Pham, L., Cao, N. Y., Nguyen, T. D., & Tran, P. T. (2013). Structural models for e-banking adoption in vietnam. International Journal of Enterprise Information Systems, 9(1), 31-48. https://doi.org/10.4018/jeis.2013010102
- Pires, G., Stanton, J., & Eckford, A. (2004). Influences on the perceived risk of purchasing online. Journal of Consumer Behaviour, 4(2), 118-131. https://doi.org/10.1002/cb.163
- Roca, J. C., García, J. J., & de la Vega, J. J. (2009). The importance of perceived trust, security and privacy in online trading systems. Information Management and Computer Security, 17(2), 96-113. https://doi.org/10.1108/09685220910963983
- Rogers, E. M. (2003). Diffusion of innovations (Fifth Edition). Free Press.
- Salimon, M. G., & Gorondutse, A. H. (2018). User adoption of smart homes technology in Malaysia: Integration TAM 3,TPB, UTAUT 2 and extension of their constructs for a better prediction. IOSR Journal of Business and Management, 20(4), 60-69. https://doi.org/10.9790/487X-2004016069
- Shen, C. C., & Chiou, J. S. (2010). The impact of perceived ease of use on Internet service adoption: The moderating effects of temporal distance and perceived risk. Computers in Human Behavior, 26, 42–50. https://doi.org/10.1016/j.chb.2009.07.003
- Shim, S., Eastlick, M. A., Lotz, S. L., & Warrington, P. (2001). An online prepurchase intentions model: The role of intention to search: Best Overall Paper Award - The Sixth Triennial AMS/ACRA Retailing Conference, 2000. Journal of Retailing, 77(3), 397-416. https://doi.org/10.1016/S0022-4359(01)00051-3
- Susanto, A., Lee, H., Zo, H., & Ciganek, A. P. (2013). User acceptance of Internet banking in Indonesia: Initial trust formation. Information Development, 29(4), 309–322. https://doi.org/10.1177/0266666912467449
- Torchia, M. (2018). Telecoms and computing market research reports. Retrieved from https://makinganewreality.org/r/?url=http%3A%2F%2Ftelecoms.report%2Ftelecoms-reports%2Fconsumer-iot-forecast-2016-21%2F
- Venkatesh, V., & Bala, H. (2008). Technology acceptance model 3 and a research agenda on interventions. Decision Sciences, 39(2), 273-315. https://doi.org/10.1111/j.1540-5915.2008.00192.x
- Venkatesh, V., Thong, J. Y. L., & Xu, X. (2012). Consumer acceptance and use of information technology: Extending the unified theory of acceptance and use of technology. MIS Quarterly: Management Information Systems, 36, 157-178.
- Wang, Q. & Waltman, L. (2016). Large-scale analysis of the accuracy of the journal classification systems of web of science and scopus. Journal of Informetrics, 10(2), 347-364.
- Yang, H., & Lee, H. (2018). Exploring user acceptance of streaming media devices: An extended perspective of flow theory. Information Systems and E-Business Management, 16, 1–27. https://doi.org/10.1007/s10257-017-0339-x
- Yau, K. L. A., Lau, S. L., Chua, H. N., Ling, M. H., Iranmanesh, V., & Kwan, S. C. C. (2016). Greater Kuala Lumpur as a smart city: A case study on technology opportunities. In 2016 8th International Conference on Knowledge and Smart Technology, KST 2016 (pp. 96-101). https://doi.org/10.1109/KST.2016.7440496
- Zhang, J., & Mao, E. (2008). Understanding the acceptance of mobile SMS advertising among young Chinese consumers. Psychology and Marketing, 25, 787–805. https://doi.org/10.1002/mar.20239
- Zhou, T. (2011). Examining the critical success factors of mobile website adoption. Online Information Review, 35(4), 636-652. https://doi.org/10.1108/14684521111161972
Copyright information
This work is licensed under a Creative Commons Attribution-NonCommercial-NoDerivatives 4.0 International License.
About this article
Publication Date
06 October 2020
Article Doi
eBook ISBN
978-1-80296-087-7
Publisher
European Publisher
Volume
88
Print ISBN (optional)
-
Edition Number
1st Edition
Pages
1-1099
Subjects
Finance, business, innovation, entrepreneurship, sustainability, environment, green business, environmental issues
Cite this article as:
Boon, N. S., Mohamed Noor, M. N., & Kebaili, B. (2020). A Proposed Conceptual Framework On The Adoption Of Internet Of Things (Iot). In Z. Ahmad (Ed.), Progressing Beyond and Better: Leading Businesses for a Sustainable Future, vol 88. European Proceedings of Social and Behavioural Sciences (pp. 352-361). European Publisher. https://doi.org/10.15405/epsbs.2020.10.31