Abstract
The paper presents one of the first stages of the project which aims to design a classifier of Internet texts in Russian by emotional tonality. The relevance of the study not only consists in the Russian language data, but also in the attempt of creating a multiclass classifier. For the most accurate realization of the idea of the project, the Lövheim cube model including eight emotions was chosen, as well as methods that tend to increase the objectivity of the results, such as an independent assessors’ data labeling, a use of corpus linguistics tools, etc. In previous linguistic analysis of the dataset taken from social network VKontakte and subsequent validation of features of emotions with the help of the corpus manager and the prototype of the classifier it has been stated that the classes Shame and Disgust, unlike other classes, did not demonstrate any peculiarities regarding lexico-morphological level. Due to the hypothesis of the reliability of syntactic features of these classes, as well as the fact of them being characterized as low-noradrenaline emotions, it has been suggested that these two must be correlated. Methods of contextual analysis, corpus linguistics and statistics have proved the relevance of specific syntactic configurations as predictors of Shame and Disgust in the Internet texts in Russian, for instance, “subject in dative case with a verb
Keywords: Disgustemotionnoradrenalinesentiment analysisshamesyntactic features
Introduction
The article presents some results of the project conducted on the field of sentiment analysis and supposed to resolve the problem of attributing Internet text in Russian to the particular class of emotions.
Although the technologies of binary and ternary sentiment analysis are rather developed (Nakov, Ritter, Rosenthal, Sebastiani, & Stoyanov, 2016; Yang, He, & Chen, 2019; Yousefpour, Ibrahim, & Hamed, 2017), the multiclass emotional text classifier represents yet a new task worth to be accomplished.
Our aim is to run a computer classifier able to detect and define the emotion mostly represented in the Internet text in Russian or to attribute the text to the emotionally neutral class.
For this purpose, we use the classification of emotions proposed by Swedish neuroscientist H. Lövheim and visualized in the form of cube. The model is built to explain a direct relation existing between specific combinations of the levels of such signal substances as dopamine, noradrenalin and serotonin in the blood of a human or an animal and eight basic emotions. The mentioned above signal substances or neurotransmitters form the axes of a coordinate system, and eight basic emotions are placed in the eight corners of cube model. The most of emotions have double names since H. Lövheim uses the first for denoting the softer expression of the emotion, the second – for the stronger: Interest / Excitement, Enjoyment / Joy, Surprise, Distress / Anguish, Anger / Rage, Fear / Terror, Contempt / Disgust, Shame / Humiliation.
To train the classifier based on machine learning technology (Nikolaev, Mitrenina, & Lando, 2016) we need a training sample of texts and a range of features for each emotional class of texts.
Our current training sample is built up with the texts extracted from the public group Overheard of Russian social network VKontakte and then annotated by native Russian speaking informants according to the criterion of the dominate emotion verbalized in them (Kalinin, Kolmogorova, Nikolaeva, & Malikova, 2018). Thus, we have nine subsets (or subcorpora) of texts – eight of them represent selections corresponding to the emotions in Lövheim’s model and the ninth is a collection of neutral texts.
To detect some features of emotional text classes, i. e. words or linguistic constructions whose presence in the text or whose rate predicts its dominant emotion, we elaborated a complex methodology combining context analysis and tools of corpus linguistics.
Problem Statement
While working with the texts of eight emotional classes we have been searching for verbal markers featuring them. Linguistic analysis supported by corpus tools helped us to reveal about twelve lexical items and morphological elements marking six classes (Kolmogorova, Kalinin, & Malikova, 2019). They all were validated when running the classifier and confirmed, although with varying degrees, their relevance as classes features. However, two classes – Shame and Disgust – did not show any distinctive characteristic on the lexico-morphological level. The only lexemes those frequency was redundant were the names of emotions themselves.
According to the Lövheim’s cube, these two emotions are marked by the very low level of noradrenalin. This may be a cause of the lack of its lexical profiling in the texts. However, we persist in thinking that these emotions should be detectable in the text, even if not on the level of verbal means of expression of the thought, but on the level of patterns of sense formalization (Rubashkin, 2012, p. 257) – in syntax.
Research Questions
To deal with emotional text classes of Shame and Disgust we focused on the syntactic specificity of texts colored by emotions of Shame and Disgust. The problem is that the data set is rather large (45 613 items for Disgust and 57 800 ‒ for Shame) and as we need the help of corpus tools to analyze it, our research question is to firstly determine the syntactic particularities of such texts, which could be considered reliable feature candidates and detectable by using research tools of corpus linguistics.
According to Lövheim’s concept, both emotions under consideration form with fear and distress the group of low-noradrenergic basic emotions. As Lövheim and al. note (Talanov et al., 2019), noradrenaline assumes such characteristics of human intellectual behavior as attention, vigilance, and activity. We could anticipate that its lack leads to the passivity, apathy and inattention to the details. However, the main question is: if there any linguistic particularities of low level of this monoamine.
Purpose of the Study
The purpose of the current stage of our study whose results we discuss in present paper is to find such syntactic patterns in two emotional texts classes under consideration, which distinguish them from other 6 emotional classes. The task is complicated by the fact that such patterns should be available for formalization to fit the query type used by corpus managers.
If succeed, we will use them as features helping to the computer classifier to predict the emotion verbalized in the input text.
Research Methods
To achieve the results, we combined the “classical” linguistic methods of contextual analysis, comparative analysis with the methodology of corpus linguistics and elements of statistics.
As a technological support of the research, we used the corpus manager platform known as Sketch Engine. It is an online text analysis tool that works not only with its own text collection, but also with individual research corpora downloaded on the platform (Zakharov, 2019). Sketch Engine offers a range of tools and services to identify what is typical and frequent in a corpus and what is rare, to extract key words of two compared corpora and to find out the most typical collocations and multi-word combinations.
Statistical metrics we used was Students t-test (Kalpić, Hlupić, & Lovrić, 2014) ‒ parametric tests based on the Student’s or t-distribution. Researchers apply the test to determine if there is a statistically significant difference between the values of two data sets.
Comparative analysis helped us to realize the differences existing between 1) texts colored by emotions whose feeling is caused by the high level of noradrenaline in the human blood (Anger, Distress, Startle, Excitement) and texts showing the emotions caused by the low level of noradrenaline (Shame, Disgust, Fear and Enjoyment); 2) texts of Shame and those of Disgust, on one hand, and those of Anger, Distress, Startle, Excitement – on the other; 3) texts of Shame and texts of Disgust).
To interpret and validate the results of corpus manager tools application we implemented the contextual analysis of randomized samples of our data set.
Findings
In the scientific literature, there are several indications to the fact that speech producing and speech processing mechanisms are highly affected by any changes in human’s physiological and psychological structures due to the stress, depression, mental diseases or intoxications (Anikushina, Taratukhin, & von Stutterheim, 2018; Pashkovskiy, Piotrovskaya, & Piotrovskiy, 2009; Spivak, 2000).
In particular, researchers mention that alcoholic intoxication leads to the low level of noradrenalin in the human blood (Lelevich, Velichko, & Lelevich, 2017, p. 376). Guided by this fact, we have found the description of speech produced by persons in alcoholic intoxication while looking at the plot pictures (Sukhikh, 2006). Such texts consist of the phrases whose predication focus particularly on the state of the subject, not on the action. It means the intoxicated people with low level of noradrenaline use more often than normally nouns, adjectives and adverbs in predicative position and avoid using verbs of action (ex. 1):
Встреча дорогих людей. Он отсутствовал, и она его дождалась. Может, он с войны пришел, а она его ждала и дождалась. Они встретились, и они счастливы. Это сразу видно.
«Черный цвет. Просвет белый. Человек мужчина или женщина не знаю. Окно открыто. Больше что еще? Почему она тут?».
This observation leads to the hypothesis that the orientation of speakers to focus on the state and not on the actions is one of the properties of low-noradrenergic basic emotions. To verify it, we used a query language CQL of Sketch Engine for detecting the rate of nonverbal predicates based on adjectives and adverbs in eight emotional corpora of texts. We applied the search formula, including all forms of Russian verb of state
The obtained results are represented in the Table
The difference between the low-noradrenergic text classes and those who are high-noradrenergic exists, but how it is significant statistically?
To evaluate it, we have applied the Student’s t-value test. Our zero hypothesis (true if the p-value will be upper than 0,05) was that the difference of frequency values of mentioned above predicates in corpora of texts showing low-noradrenergic emotions and high-noradrenergic emotions is not significant. Our alternative hypothesis (true if the p-value will be lower than 0,05) was that such difference is statistically relevant. As the p-value was 0.011719, it means lower than 0,05, we accepted alternative hypothesis – the difference is statistically significant.
In our further discussion, we will compare the values of Shame and Disgust corpora with those of Anger corpus, using the latter as a reference corpus to prove the specificity of syntactic constructions of formers. It is due, first, to the fact that the corpora of Shame and Disgust are the focus point of our interest and, secondly, to the observation that they contain more of “nonverbal” predicates (“быть+Adj/ быть+Adv”) than Fear or Enjoyment corpora (Table
Our search formula cited above is targeting at two types of syntactic constructions:
one-member impersonal adverbial sentences (Bryzgunova et al., 1980) (быть+Adv: Было страшно (It was scary));
two-member sentences with compound nominal predicate (быть+Adj: Я была маленькой (I was little)).
Sketch Engine platform proposes to its users a tool able to compare two corpora and then to find n-grams which are frequent in one corpus, but do not occur or occur rarely in another. Such n-grams obtain the status of key-words.
After having compared in this way, first, the Shame corpus and the Anger corpus and, secondly, the Disgust corpus and the Anger corpus, we saw in the lists of key 3-grams of Shame and Disgust corpora the syntagmata corresponding to two, found earlier, syntactic structures ‒ one-member impersonal adverbial sentences (
Besides these two, in key 3-grams we also find syntagmata being parts of the possessive constructions like
Consider some illustrative examples from the Shame and Disgust corpora:
One-member impersonal adverbial sentences:
Провожали с подругой мальчика-одноклассника на поезд. Когда он отъезжал от платформы, шли параллельно его окошку и махали мило ручками. Поезд набирал скорость. Вскоре мы перешли на бег, но всё так же махали и смеялись. И поезд резко затормозил! Мы стояли и не могли понять, что произошло, почему он не едет. Боже, как мне неловко теперь перед тем машинистом. Добрый человек, наверное, подумал, что мы опаздывали, и остановил целый состав... (Shame);
Одно из самых отвратительных воспоминаний моего детства ‒ это как папа раздавил голой ногой огромную жирную саранчу. Она сидела на его тапке, он хотел обуться, но делал это, не глядя, и в итоге пяткой наступил на нее. Мне, как жесткому инсектофобу, было одновременно и страшно, и отвратительно (Disgust).
The syntactic combinatorics within such type of construction is rather different for Shame and Disgust:
Consider some examples of two-member sentences with compound nominal predicates:
As the emotion of shame is often evoked by souvenirs of childhood, the collocation “kogda ja byla mal’en’koj” (‘when I was a child’) occurs regularly in the Shame corpus and appears as one of its featuring verbal markers.
In the pull of examples, containing the construction
(7)
(8)
(9)
While continuing our work with three corpora, we succeeded to define and then to compare the frequency of three types of syntactic constructions considered as feature candidates for two emotional text classes (Figure
On the other hand, there is some inconsistency between the Shame and Disgust corpora: although they have an approximatively equal number of two-member sentences with compound nominal predicates, they are very different in use of impersonal adverbial sentences and do not show the same inclination to the possessive sentences.
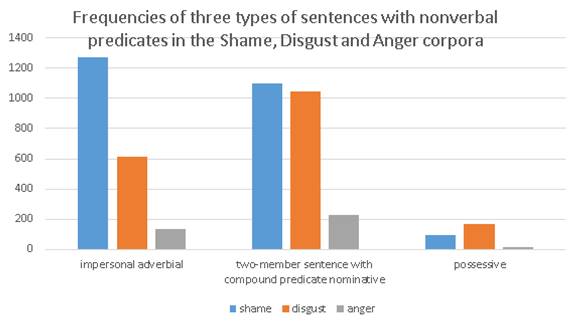
Thus, going from the most abstract level of hypothesis based on the general assumption, through the formal and then syntactic levels analysis and using the corpus tools we succeed to detect a range of syntactic patterns proper to the Shame and Disgust Internet texts in Russian. Even some features distinguishing Shame from Disgust were also found (Table
Conclusion
The applied algorithm including the steps on different levels of linguistic abstraction and largely supported by corpus tools has allowed us to detect some syntactic features of “ashamed” and “disgusted” Internet texts in Russian. We expect that their validation in classifier work will show their relevance and will help us to increase the accuracy of emotional texts classification.
Acknowledgments
The study is funded by the Russian Foundation of Basic Research, grant No. 19-012-00205.
References
- Anikushina, V., Taratukhin, V., & von Stutterheim, Ch. (2018). Natural Language Oral Communication in Humans Under Stress. Linguistic Cognitive Coping Strategies for Enrichment of Artificial Intelligence. Procedia Computer Science, 123, 24-28. https://doi.org/10.1016/j.procs.2018.01.005
- Bryzgunova, E. A., Gabuchan, K. V., Itskovich, V. A., Kovtunova, I. I., Kruchinina, I. N., … & Shvedova, N. Y. (1980). Narechnyi klass [Class of Adverbs]. In N.Y. Schvedova (Ed.), Russkaya grammatika, 2, Syntax (pp. 378-381). Moscow: Nauka.
- Kalinin, A., Kolmogorova, A., Nikolaeva, G., & Malikova, A. (2018). Mapping Texts to Multidimensional Emotional Space: Challenges for Dataset Acquisition in Sentiment Analysis. Digital Transformation and Global Society. DTGS 2018. Communications in Computer and Information Science, 859, 361-367. https://doi.org/10.1007/978-3-030-02846-6_29
- Kalpić, D., Hlupić, N., & Lovrić, M. (2014). Student’s t-Tests. In M. Lovrić (Ed.), International Encyclopedia of Statistical Science. https://doi.org/10.1007/978-3-642-04898-2_641
- Kolmogorova, A., Kalinin, A., & Malikova, A. (2019). Tipologiya i kombinatorika verbal'nykh markerov razlichnykh emotsional'nykh tonal'nostei v internet-tekstakh na russkom yazyke [The Types and Combinatorics of Verbal Markers of Different Emotional Tonalities in Russian-Language Internet Texts]. Tomsk State University Journal, 448, 48-58. https://doi.org/10.17223/15617793/448/6
- Lelevich, S. V., Velichko, I. M., & Lelevich, V. V. (2017). Neirokhimicheskie aspekty alkogol'noi intoksikatsii [Neurochemical Aspects of Alcoholic Intoxication]. Journal of the Grodno State Medical University, 15(4), 375-380. https://doi.org/10.25298/2221-8785-2017-15-4-375-380
- Nakov, P., Ritter, A., Rosenthal, S., Sebastiani, F., & Stoyanov, V. (2016). SemEval-2016 Task 4: Sentiment Analysis in Twitter. Proceeding of the 10th International Workshop on Semantic Evaluation (SemEval–2016), 1-18. https://doi.org/10.18653/v1/S16-1001
- Nikolaev, I. S., Mitrenina, O. V., & Lando, T. M. (2016). Prikladnaya i komp'yuternaya lingvistika [Applied and Computational Linguistics]. Moscow: Lenand.
- Pashkovskiy, V. E., Piotrovskaya, V. R., & Piotrovskiy, R. G. (2009). Psikhiatricheskaya lingvistika [Psychiatric Linguistics]. Moscow: Lenand.
- Rubashkin, V. (2012). Ontologicheskaya semantika. Znaniya. Ontologii. Ontologicheski orientirovannye metody informatsionnogo analiza tekstov [Ontological Semantics. Knowledges. Ontologies. Ontologically Oriented Methods of Information Analysis of Texts]. Moscow: FIZMATLIT.
- Spivak, D. L. (2000). Izmenennye sostoyaniya soznaniya. Psikhologiya i lingvistika [Altered States of Consciousness. Psychology and Linguistics]. Saint Petersburg: Juventa.
- Sukhikh, S. A. (2006). Yazykovaya reprezentatsiya izmenennykh sostoyanii soznaniya [Altered States of Consciousness Represented Verbally]. Language, Communication and Social Environment, 3, 78-93.
- Talanov, M., Leukhin, A., Lövheim, H., Viverdú, J., Toschev, A., & Gafarov, F. (2019). Modeling Psycho-Emotional States via Neurosimilation of Monoamine Neurotransmitters. Blended cognition. The Robotic Challenge, 127-157. https://doi.org/10.1007/978-3-030-03104-6_6
- Yang, G., He, H., & Chen, Q. (2019). Emotion-Semantic-Enhanced Neural Network. IEEE/ACM Transactions on Audio, Speech, and Language Processing, 27(3), 531-543. https://doi.org/10.1109/TASLP.2018.2885775
- Yousefpour, A., Ibrahim, R., & Hamed, H. N. A. (2017). Ordinal-Based and Frequency-Based Integration of Feature Selection Methods for Sentiment Analysis. Expert Systems with Applications, 75, 80-93. https://doi.org/10.1016/j.eswa.2017.01.009
- Zakharov, V. P. (2019). Funktsional'nost' instrumentov korpusnoi lingvistiki [Corpus Linguistics Tools Functionality]. In I.S. Nikolaev (Ed.), Structural and Applied Linguistics, 12 (pp. 81-95). Saint Petersburg: Saint Petersburg State University.
Copyright information
This work is licensed under a Creative Commons Attribution-NonCommercial-NoDerivatives 4.0 International License.
About this article
Publication Date
03 August 2020
Article Doi
eBook ISBN
978-1-80296-085-3
Publisher
European Publisher
Volume
86
Print ISBN (optional)
-
Edition Number
1st Edition
Pages
1-1623
Subjects
Sociolinguistics, linguistics, semantics, discourse analysis, translation, interpretation
Cite this article as:
Kolmogorova, A., Kalinin, A., & Malikova, A. (2020). Syntactic Specificity Of Texts Verbalizing Disgust And Shame. In N. L. Amiryanovna (Ed.), Word, Utterance, Text: Cognitive, Pragmatic and Cultural Aspects, vol 86. European Proceedings of Social and Behavioural Sciences (pp. 700-708). European Publisher. https://doi.org/10.15405/epsbs.2020.08.83