Abstract
The dynamics of modern professional methodological language demands an unprecedented accelerated renewal of school technology. Approbation of interdisciplinary technologies in schools, which contribute to effective mastering of the content of a particular lesson, remains very important. Thus, the purpose of the study is to examine the intersubject technology of adaptive learning and testing in schools. The authors of the article determine methodological aspects of implementing adaptivity in school educational programs. The main approach is the modeling of the methodological system of teaching in primary and secondary schools including the interdisciplinary technology of adaptive learning and testing. Intersubject technology contributes to the achievement of unified learning outcomes for schoolchildren in a short time due to the most relevant content for each student. The research differentiates the main components of the adaptive learning technology, technologies of development and implementation of adaptive testing. The study offers some important insights into the development of scientific ideas on interdisciplinary learning technologies aimed to master new knowledge according to the level of the pupil's abilities. Practitioners can use the described technology to organize work on step-by-step introduction of the intersubject technology of adaptive learning and testing. The article can be of interest for educational theorists, practitioners, educators, in-service and pre-service teachers.
Keywords: Pedagogical technologyintersubject technologyadaptive learningadaptive testing
Introduction
The rapidly increasing pace of the fourth industrial revolution calls for a revision of the basic paradigms of education and a radical transformation of the models and mechanisms of school teachers’ work.
It should be noted that according to the results of the study of the Russian Academy of Education, a reported number of gifted students increases. They are children with developed thinking; children who are able to influence others (leaders); children who are very skilful; children who see the world in images (artistically gifted children); children with motor talents (Feldstein, 2010).
On the other hand, it is significant that for modern teenagers it is more important to search for their own special meaning of life than to entertain. Their critical stance towards adults changes, and new characteristics in their social development appear. It strengthens the dynamics of modern professional methodological language and affects the unprecedented accelerated renewal of school technology. Moreover, testing of interdisciplinary technologies in schools, which improve learning content of a particular lesson, is quite urgent.
Komenskiy (1875) pointed out the principle of natural teaching which is in the need to match given knowledge with student’s abilities and level of development. Therefore, teaching materials should meet the needs of ‘various’ children, which corresponds to the first distinctive feature of modern children. According to the second feature, it is necessary to teach students in different forms and contexts: formal, informal and informational; individual and co-educational; in the classroom, at home and in real life situations. All this accompanies the child's social development and should be taken into account when choosing the teaching technology. Adaptive learning is one of the most effective interdisciplinary technologies which helps schoolchildren to achieve unified learning outcomes in a short period of time due to the most relevant content in each student’s case.
Adaptive learning can be described as a dynamic individual education based on data analysis, the level of preparedness, abilities, purpose, motivation and other characteristics of the student. The basic principle of adaptive learning is that pupils, starting learning with different levels of knowledge, skills and preparedness, achieve unified learning outcomes determined by the educational program through mastering individual educational trajectories.
Adaptive learning as a method was first described by Skinner (1957) in his work ‘Verbal Behavior’. He invented a mechanical device in the form of a box. Students’ correct answers were followed by new academic material; in case of incorrect answers material was revised. It motivated students to adapt quickly and learn to give correct answers.
Adaptive learning systems aim at providing students with an educational environment that adapts to their needs, giving relevant information, teaching materials, and formulating recommendations based on their individual learning characteristics. Adaptive learning system, therefore, facilitates the educational process.
The term ‘adaptive’ refers to the functionality of a process that automatically provides various educational paths to learners with different learning characteristics and needs. Adaptive learning takes into account students' preliminary knowledge, preferences, learning goals, ‘knowledge gaps’, learning styles and cognitive abilities.
Problem Statement
Research into the transformation of interdisciplinary educational technologies, which adapt to characteristics and needs of the student, conducted by Russian and international scholars, is quite diverse.
The first adaptive educational systems were developed by Brusilovsky, Schwartz and Weber in the 1990s (1996a; 1996b). They took into account the analysis of hypermedia-content and the basics of building intelligent online learning systems. De Bra (1996) examined the adaptive hypermedia course. Recently, researchers of adaptive learning described numerous systems. The first adaptive learning systems were focused on determining the state of knowledge and educational progress of students during the course or while solving a specific problem (Nakabayashi, Koike, Maruyama, Touhei, Ishiuchi & Fukuhara, 1995). Since then, a wider set of characteristics, educational needs and student’s states have been examined and considered (learning styles, cognitive abilities, emotional states, learning goals, interests, knowledge gaps, and so on). These new systems are designed to dynamically identify these characteristics, needs and states, observing the behavior, progress and interaction of students with the system and applying methods of artificial intelligence in order to create and update the student’s model.
For example, Mirea and Preda (2009) analyze e-learning systems that provide personalized content to their users with a step-by-step adaptation of the learning material based on the student's progress. They describe various types of adaptation that take into account the content and navigation in the course of studies. Student's profile is studied and learning activities are modeled in order to create such adaptive conditions. According to Laroussi (2012), if adaptation conditions are realized, then there is a need to adapt the student's educational profile. Therefore, adaptive learning systems should use the approach of reusing adapted teaching material for the purposes of other students. The student’s profile can be a key element of the curriculum adaptation pattern and the basis for constructing the student's educational path (Qodad, Seghroucheni, Al Achhab, El Yadari, El Kenz & Benyoussef, 2017). Another object of study in Yang and Wu’s (2009) works is the development of rules for determining how students with different learning outcomes can find more important learning objects for their development.
Effectiveness of using adapted online training courses depends directly on feedback and current learning outcomes of the proposed content (Wirastuti, Sukadarmika, Suyadnya & Krishne, 2016; Wu, Chen, Yan & Lee, 2017). Feedbacks on an adaptive learning environment are effective because most students have different personal characteristics, such as prior knowledge, learning achievement and training preferences. Bimba, Idris, Al-Hunaiyyan, Mahmud and Shuib (2017) analyze various types of feedback on an adaptive learning environment (dialogues, smart e-learning systems and adaptive hypermedia systems).
Feedback system that directs learners to achieve educational outcomes can improve the learning process (Muñoz-Merino, et al, 2011). Feedback is often provided during the lesson in the classroom. Most of the times, however, it does not allow to draw an objective conclusion about current educational progress as the feedback comes from the whole group. Therefore, students do not consider such feedback as relevant to them (Hattie & Gan, 2011). Today teachers and administrators often face the problem of the gap between the average result of a group and the initial results of a student (Luckin, & Holmes, 2016). Feedback can also be integrated into social networks based on computer adaptive testing methods (Advisors, 2013; Dascalu, Nitu, Alecu, Bodea & Moldoveanu, 2017).
Guo, Zhou and Chen (2010) describe the technical scheme of managing adaptive learning, proving its effectiveness through action simulators. The scheme for designing an adaptive learning system can be found in the research conducted by Liu, Sun and Lee (2013) where they describe computer adaptive learning platforms. Chang and Cheng (2016) give a description of the platform for studying units of mathematical analysis. Students are given the opportunity to test themselves at any time. The system provides learners with adaptive elements of testing to review their learning progress. After analyzing the student's educational portfolio, the system sends information to the teacher.
Researchers also consider models of adapted testing. Thus, Jia’s model, based on the theory of fuzzy sets, evaluates the level of the student’s knowledge through the test, cognitive abilities and preferences of the student (Jia, Zhong, Zheng & Liu, 2010). According to Chen (2012), computer adaptive testing has the same advantages as computer testing and allows to assess the progress of each student in the class. Veldkamp (2016) describes another advantage of computer adaptive testing which is the ability to record not only the student’s response to each question, but also the amount of time that students spend on thinking and answering each question. Taking into account these advantages, various methods of using response time data were studied when selecting appropriate questions for a particular test subject. Four strategies for including response time data and the accuracy of student’s final score, comparing it with a reference value were evaluated.
Thus, to date, the development of adaptive learning technology has many different forms and contexts. Learning environments range from desktop learning systems, such as learning management systems that create ubiquitous educational environments for students. In each of these forms and contexts, adaptive support has great potential to make such educational environments more personalized, learner-friendly and effective.
Research Questions
The research aimed to answer the questions:
Does intersubject technology contribute to the achievement of unified learning outcomes for schoolchildren in a short time due to the most relevant content for each student?
What are the main components of the adaptive learning technology, technologies of development and implementation of adaptive testing?
Purpose of the Study
The purpose of the study is to examine the intersubject technology of adaptive learning and testing in schools. The authors of the article determine methodological aspects of implementing adaptivity in school educational programs.
Research Methods
The methodological basis of the research is the introduction of interdisciplinary technology of adaptive learning and testing. Intersubject technology contributes to the achievement of unified learning outcomes for schoolchildren in a short time due to the most relevant content for each student.
Adaptive learning
Implementation of adaptive learning is possible on electronic educational platforms that have a number of basic components: a data collection system, a system of conclusions, a personalization system.
The first component is the data collection system. It collects and processes data on each student’s knowledge and skills. It consists of an adaptive ontology and model calculations means. The ontology displays connections between individual concepts, which integrates into the required taxonomy, learner's goals and algorithms, model calculations means that process the data in real time and analyze them for later use.
The second component is the system of conclusions. The component is responsible for converting the data and generating conclusions based on all the collected information about the progress of the student. It consists of qualimetry, training strategy and feedback mechanism. Qualimetry describes tools for assessing students' knowledge and skills, parameters of the educational material, and the effectiveness of teaching. Each new level specifies information about the student. The learning strategy takes into account a student's sensitivity to changes in teaching, assessment, and the pace of learning. Feedback mechanisms combine all the data and transfer it back to the data collection system.
The third component is the personalization system. Personalization is responsible for the didactic value of the entire adapted learning system that is for building an individual educational route for each subject or section. It consists of qualimetry of recommendation tools, forecasting analytics, learning chronology. Recommendation tools provide students with ranked suggestions about what the student should do next, regulating the learning goals, the strengths and weaknesses of the student, their involvement. The tools of forecast analysts calculate the degree and probability of achieving the goals set by the teacher (for example, the probability that the student will get excellent marks in upcoming tests), the expected assessment, the level of knowledge and skills. The learning chronology tools are formalized through a student's private office, which allow tying together the learning experience obtained at various training courses, using different training formats.
The generalized logic of adaptive learning can be represented by a system of four consecutive stages: interactive content; managed educational environment; qualimetry; personalized path (Figure
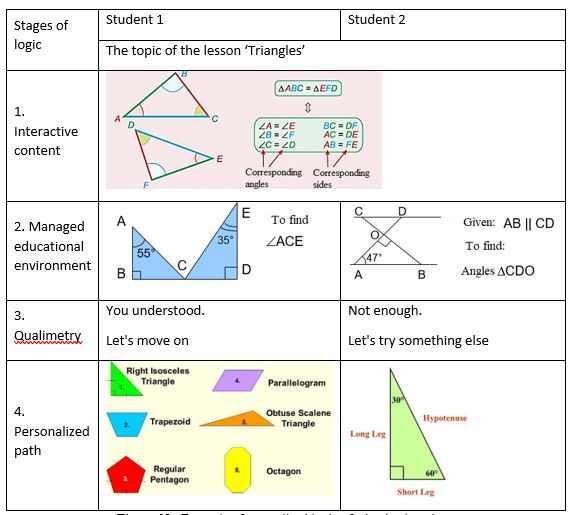
Adaptive testing
Adaptive testing is a technology for testing students, where the subsequent question is selected automatically, based on answers to previous questions and a predetermined level of complexity.
The main difference between adaptive testing and traditional tests is the dynamic (real-time) definition of the list of questions that the student will be asked. Trajectory, according to which the student passes the tests, is individual. The choice of the next question is determined by the personal characteristics of each student, and not by general rules. It should be noted that in order to create adaptive testing, it is necessary to separate the testing module and the module responsible for computer evaluation (Del Sorbo & Balzano, 2011). An example can be the system of questions of a teacher who conducts an oral examination.
Adaptive testing is most effective for students' self-testing, accurate evaluation of learning outcomes, prediction of learning outcomes through pre-testing, identifying ‘knowledge gaps’ and dividing students into subgroups, taking into account the level of their preparedness.
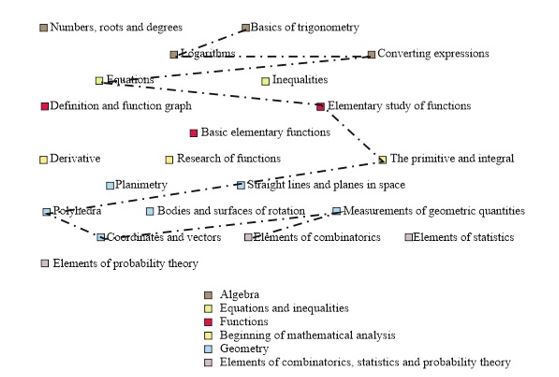
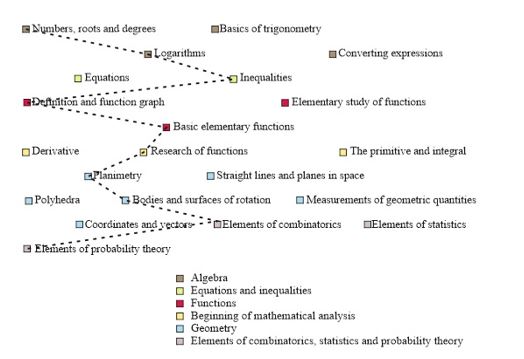
Here are methodological aspects of developing and conducting adaptive testing (Katkalo & Volkova, 2017).
Development of test questions
The minimum size of the question bank, from which an individual list of questions will be formed for each participant, is recommended to be determined by the following formula: question bank = 15 × number of topics (thematic blocks of the test).
The optimal number of questions in the individual test is calculated by the formula: total number of questions = 5 × number of topics (thematic blocks of the test).
Otherwise, the adaptive algorithm may not have enough questions to sufficiently and reliably determine the level of knowledge of a certain topic.
Variability of test responses
To automatically verify the answers to the tests, each question must have at least one correct answer. If the question contains only one answer and this answer is correct, then this question is defined as an open-ended question. A question can have any number of correct answers. All answers can also be correct at the same time.
Levels of difficulty
Questions in the question bank are ranked according to levels of complexity. There should be at least 3 levels, optimal number is from 5 to 10. The larger the number of levels of complexity (for example, 10) the more accurate the distribution of questions on the scale of complexity after the start of testing which ensures a higher accuracy of the final assessment of knowledge. Each topic should contain questions of various complexities as it directly affects the variability of the test and adaptive capabilities of the technology. Within each topic, it is better to distribute questions in groups of at least three questions at each level.
Variants of distribution
Uniform distribution. It is recommended to have at least 10 questions of each level of complexity.
‘Pyramid’. Questions in the form of a ‘pyramid’ are allowed when the number of complicated questions is less than the number of simple questions (since they are much less frequent). In this case, it is recommended to have at least 6 questions of higher levels of complexity (9-10), at least 8 questions of average levels of complexity (7-8) and at least 10 questions of lower levels of complexity (1–6).
Automatic distribution. It is possible to automatically distribute questions according to levels of complexity. In this case, one needs to have initial testing, and the degree of complexity of questions is determined in accordance with participants’ responses. Questions that get most of the correct answers are considered to be the simplest, and questions with the fewest correct answers are the most difficult.
Correction of adaptive tests based on analytics
Frequency analysis of results. It allows to make an adjustment to the distribution of questions according to levels of complexity in a balanced model based on the analysis of the distribution of students' answers that is one can identify abnormally complex or simple questions and get a recommendation where to transfer these questions.
Forecasting learning outcomes. Statistical analysis of the results of the adaptive test is based on the number of attempts to pass the test and the average result achieved during all attempts.
These methodological recommendations allow to take into account the experience of increasing the effectiveness of the developed adaptive testing. It should be noted that Choe, Kern & Chang (2018) have an additional parameter of adaptive testing – the response time of the test, which can be taken into account in the test results without lowering the accuracy of the evaluation. There, however, arises the problem of increasing the computational complexity of the evaluation, which must be investigated (Huang, Lin & Cheng, 2009). As a consequence, in adaptive testing it is necessary to take into account balance between the evaluation complexity and the parameters of adaptive testing. For example, Cechova, Neubauer & Sedlacik (2014) describe the successful experience of selecting the parameters of the introductory adaptive test for predictability of academic performance in higher education.
Thus, we see the differentiation of the main components of adaptive learning technology, the development and implementation of adaptive testing, which allows to organize step-by-step introduction of interdisciplinary technology of adaptive learning and testing in general education.
Findings
The proposed interdisciplinary technology of adaptive learning and testing in schools was tested while working with teams of teachers of the regional innovative sites of the Ministry of Education of the Kirov region. For example, as a result of the work of the regional innovation site in the lyceum No. 21 in Kirov on the topic ‘Interconnection of content, forms and methods of basic and additional mathematical education of schoolchildren’ studies which used key aspects of adaptive learning and testing technologies were initiated. They are ‘Technology of using the bank of non-standard problems in the additional mathematical education of students of the 5-6th grades of the secondary school’, ‘Technology of organizing the distance education system in Moodle environment while preparing for the final school exams’, ‘Development of the meta-subjectivity of secondary school students through open-type tasks in the creative lesson of mathematics’, ‘Comprehensive system for learning mathematical problems in the course ‘Developing Mathematics’ of the 5th-6th grades of the secondary school’ and ‘Activity of students at the lessons of mathematics in the course ‘Design based on scientific creativity’ in the 10th grade of secondary school’. The results of each work are presented by scientific and methodical works carried out jointly by scientists and students of Vyatka State University and teachers of mathematics of Lyceum No. 21 of Kirov city.
Conclusion
The development of adaptive learning and testing as an intersubject technology is closely connected with the development of electronic educational platforms that have a number of basic elements considered in the article. The authors come to the conclusion that on the one hand, further development of technology is possible through the promotion of requirements for electronic platforms, taking into account availability of these resources in a wide range of educational subjects. On the other hand, overestimated requirements for adaptive learning and testing opportunities lead to the use of complex software and technical solutions and limit further mass use of this technology. Educational solutions for large online platforms can solve this problem. They will have to provide their support by raising contributions for the use of resources, which will require high awareness of the educational business in order to differentiate the payment for using the online platform for different subjects of the educational process.
Another discussion issue today is the development of the mathematical and statistical apparatus for correcting the adaptive test based on the analysis of a particular student, class, educational organization and representatives of a particular age group.
A significant result of the study is the description of the intersubject technology of adaptive learning and testing in schools. The article defines methodological aspects of the implementation of adaptability in school educational programs. The considered interdisciplinary technology contributes to the achievement of unified results of schoolchildren's education in shorter terms due to the relevant content for each student. As a result of the study, the authors of the article have differentiated the main components of adaptive learning technology, development and implementation of adaptive testing. The practical use of this technology makes it possible to organize a step-by-step introduction of the interdisciplinary technology of adaptive learning and testing in general education.
References
- Advisors, E. G. (2013). Learning to adapt: A case for accelerating adaptive learning in higher education. Education Growth Advisors.
- Bimba, A. T., Idris, N., Al-Hunaiyyan A., Mahmud R. B., & Shuib N. L. (2017). Adaptive feedback in computer-based learning environments: A review. Adaptive Behavior, 25(5), 217–234.
- Brusilovsky, P., Schwarz E., & Weber G. (1996a). A tool for developing hypermedia-based ITS on WWW. In C. Frasson, G. Gauthier, A. M. Lesgold (Eds.) Workshop on Architectures and Methods for Designing Cost-Effective and Reusable ITSs at the International Conference on Intelligent Tutoring Systems (pp. 198–203). Montreal, Canada.
- Brusilovsky, P., Schwarz E., & Weber G. (1996b). Elm-Art: An intelligent tutoring system on World Wide Web. In C. Frasson, G. Gauthier, A. M. Lesgold (Eds.) International Conference on Intelligent Tutoring Systems, Lecture Notes in Computer Science (pp. 261–269). Montreal, Canada: Springer.
- Cechova, I., Neubauer, J., & Sedlacik, M. (2014). Computer-adaptive testing: Item analysis and statistics for effective testing. Proceedings of the European Conference on e-Learning, ECEL, 106-112.
- Chang, S. L., & Cheng, S. C. (2016, October). Computer Adaptive Learning Platform for Calculus. In International Symposium on Emerging Technologies for Education (pp. 153-162). Springer, Cham.
- Chen, Y. (2012). Adaptive testing system based on diagnosis mechanism and normal distribution. International Journal of Computational Science and Engineering, 7(2), 155–161.
- Choe, E. M., Kern, J. L., & Chang, H. (2018). Optimizing the use of response times for item selection in computerized adaptive testing. Journal of Educational and Behavioral Statistics, 43(2), 135-158. doi:10.3102/1076998617723642.
- Dascalu, M. I., Nitu, M., Alecu, G., Bodea, C. N., & Moldoveanu, A. D. B. (2017). Formative Assessment Application With Social Media Integration Using Computer Adaptive Testing Techniques. In ICEL 2017-Proceedings of the 12th International Conference on e-Learning (p. 56-65). Academic Conferences and publishing limited.
- De Bra, P. (1996). Teaching hypertext and hypermedia through the Web. Journal of Universal Computer Science, 2(12), 797–804.
- Del Sorbo, M. R., & Balzano, W. (2011). E-Xamina: An experimental multi-user assessment platform for computer adaptive testing. In Technology for Education (T4E), 2011 IEEE International Conference on (pp. 237-240). IEEE.
- Feldstein, D. I. (2010). Priority directions of psychological and pedagogical research in conditions of significant changes of the child and the situation of his development. Moscow: MPSI, Modec.
- Guo, Y., Zhou, C., & Chen, Q. (2010). Combined adaptive learning control for a class of LTV systems. In Chinese Control and Decision Conference, CCDC, 2010 (pp. 3986–3991).
- Hattie, J., & Gan, M. (2011). Instruction based on feedback. Handbook of research on learning and instruction, 249-271.
- Huang, Y., Lin, Y., & Cheng, S. (2009). An adaptive testing system for supporting versatile educational assessment. Computers and Education, 52(1), 53-67. doi:10.1016/j.compedu.2008.06.007.
- Jia, B., Zhong, S., Zheng, T., & Liu, Z. (2010). The study and design of adaptive learning system based on fuzzy set theory. In Transactions on edutainment IV (pp. 1-11). Springer, Berlin, Heidelberg.
- Katkalo, V. S., & Volkova, D. L. (2017). Corporate training for the digital world. Moscow: ANO DPO ‘Corporate University of Sberbank’.
- Komenskiy, Y. A. (1875). The Great Didactics. St. Petersburg: Printing house of A.M. Kotomina.
- Laroussi, M. (2012). Ontology in adaptive Learning environment. In Workshop on Learning Technology for Education in Cloud (LTEC'12) (pp. 167-177). Springer, Berlin, Heidelberg.
- Liu, C. M., Sun, Y. J., & Li, H. Y. (2013). Adaptive Learning System Designed and Learning Program Optimization Algorithm. In Applied Mechanics and Materials (Vol. 347, pp. 3114-3118). Trans Tech Publications.
- Luckin R., & Holmes W. (2016). Intelligence unleashed: An argument for AI in education. L.: Pearson.
- Mirea, A. M., & Preda, M. C. (2009, September). Adaptive Learning Based on Exercises Fitness Degree. In Proceedings of the 2009 IEEE/WIC/ACM International Joint Conference on Web Intelligence and Intelligent Agent Technology-Volume 03 (pp. 215-218). IEEE Computer Society.
- Muñoz-Merino, P. J., Pardo, A., Scheffel, M., Niemann, K., Wolpers, M., Leony, D., & Kloos, C. D. (2011). An ontological framework for adaptive feedback to support students while programming. In International Semantic Web Conference (pp. 1-4). Bonn, Germany.
- Nakabayashi K., Koike Y., Maruyama M., Touhei H., Ishiuchi S., & Fukuhara Y. (1995). An intelligent tutoring system on World Wide Web: Towards an integrated learning environment on a distributed hypermedia. In World Conference on Educational Multimedia, Hypermedia, and Telecommunications (pp. 488–493). Graz, Austria: AACE Press.
- Qodad, A., Seghroucheni, Y. Z., Al Achhab, M., El Yadari, M., El Kenz, A., Benyoussef, A. (2017). An adaptive learning system based on a job model, the differentiated instruction and felder and silverman's learning styles model. In Colloquium in Information Science and Technology (pp. 506-510). CIST. doi:. 10.1109/CIST.2016.7805100.
- Skinner, B. (1957). Verbal Behavior. Acton, MA: Copley Publishing Group.
- Veldkamp, B. P. (2016). On the issue of item selection in computerized adaptive testing with response times. Journal of educational measurement, 53(2), 212-228.
- Wirastuti, N. D., Sukadarmika, G., Suyadnya, I. A., & Krishne, D. C. (2016, October). Adaptive online learning design using moodle. In Smart Green Technology in Electrical and Information Systems (ICSGTEIS), 2016 International Conference on (pp. 98-101). IEEE.
- Wu, C. H., Chen, T. C., Yan, Y. H., & Lee, C. F. (2017, May). Developing an adaptive e-learning system for learning excel. In Applied System Innovation (ICASI), 2017 International Conference on (pp. 1973-1975). IEEE. doi: 10.1109/ICASI.2017.7988583.
- Yang, Y. J., & Wu, C. (2009). An attribute-based ant colony system for adaptive learning object recommendation. Expert Systems with Applications, 36(2), 3034-3047.
Copyright information
This work is licensed under a Creative Commons Attribution-NonCommercial-NoDerivatives 4.0 International License.
About this article
Publication Date
05 September 2018
Article Doi
eBook ISBN
978-1-80296-044-0
Publisher
Future Academy
Volume
45
Print ISBN (optional)
-
Edition Number
1st Edition
Pages
1-993
Subjects
Teacher training, teacher, teaching skills, teaching techniques
Cite this article as:
Utemov, V. V., & Simonova, G. I. (2018). Inter-Subject Technology Of Adaptive Learning And Testing In Schools. In R. Valeeva (Ed.), Teacher Education - IFTE 2018, vol 45. European Proceedings of Social and Behavioural Sciences (pp. 526-536). Future Academy. https://doi.org/10.15405/epsbs.2018.09.60