Abstract
This paper intended to examine the effect of technostress on student performance expectancy. Utilizing the mediating effects of student satisfaction, four main factors, namely techno-overload, techno-complexity, techno-insecurity and techno-uncertainty, were used to predict student performance expectancy. A total of 402 students of Universiti Teknologi Mara (UiTM) completed survey-based online questionnaires. The proposed hypothetical model was statistically tested using Structural Equation Modelling (SEM) using AMOS 27.0 based on the data. The findings indicated that technostress was the significant predictor that influenced student satisfaction. Meanwhile, student satisfaction was a significant predictor that influenced performance expectancy. Additionally, the findings also indicated that student satisfaction played a mediating role in the technostress-performance expectancy relationship in the university student context. This finding further revealed that reducing technostress would increase student satisfaction, and by increasing their satisfaction, it would increase student performance expectancy. This study provides the institution with an effective method for lowering technostress during open and distance learning (ODL). It is advised that the institution creates an effective academic plan and schedule, set up the greatest educational technology resources, and provides training to assist them advance their knowledge and abilities. The institution needs to be mindful of the negative impacts of ODL, which will affect students' academic performance if improperly implemented. The mismatch between students' technological proficiency and the ODL environment put in place during the Covid-19 outbreak may be the cause of technostress's negative effects on performance expectancy.
Keywords: Performance expectancy, student satisfaction, technostress, university students
Introduction
Currently, most universities in Malaysia have implemented online teaching and learning to continue lecture sessions while the Covid-19 pandemic is in full swing. The implementation of Open and Distance Learning (ODL) throughout the Movement Control Order (MCO) is a new phenomenon for lecturers and students. To maintain the continuity of the university's academic calendar, all parties are compelled to adopt and utilize this distinctive teaching and learning method to the fullest extent possible. ODL requires students to be skilled in using technology, including mastering all software and applications to pursue learning, and complete all assignments within the stipulated time frame. Many previous researchers assumed that university students were from Generation-Z (Gen-Z) who were technologically proficient and digitally literate (Warren et al, 2020), thus having no difficulty pursuing online learning. Such assumptions cause the management to overlook the actual experience of stress faced by students concerning the use of technology in ODL. Our fear is that failing to determine the degree of technostress that students are experiencing could have an adverse effect on their academic performance.
Generally, technostress is defined as stress created by the technology used (Jena, 2015a; Tarafdar et al., 2007); incorporating adaptations to the rapidly changing physical, social, and cognitive demands associated with the technology used. According to some researchers (Agogo & Hess, 2015), technology can cause people to feel unfavorable feelings like mental weariness, skepticism, inefficiency, and anxiety, as well as a direct or indirect decrease in satisfaction. This suggests that technostress has a negative impact on students' academic performance and satisfaction. Excessive technostress has been linked to a number of harmful effects on people, including decreased performance and satisfaction (Suharti & Susanto, 2014; Tarafdar, Pullins et al., 2015). However, the majority of recent study on this topic has been conducted in the business and government sectors (Hwang & Cha, 2018; Marchiori et al., 2019). Almost all of the study on technostress in education has been conducted on teachers (Joo et al., 2016; Jena, 2015a).
However, despite the considerable importance of technostress, prior studies related to university students have only looked at the impacts of technostress on academic performance (Tarafdar, D’Arcy et al., 2015; Tarafdar et al., 2010). Others explored the direct relationship between technostress and student satisfaction (Ragu-Nathan et al., 2008; Upadhyaya & Vrinda, 2020). Since earlier studies have shown that technostress has a negative impact on academic performance (Tarafdar, D’Arcy et al., 2015, 2010), there should be a strategy to lessen the stress that technology causes in students. Because some studies have revealed a direct correlation between student satisfaction and student performance, student satisfaction with using technology in online learning should be examined as a mediator (Lee, 2010; Mullen et al., 2005; Martirosyan et al., 2015). Although the direct relationship between satisfaction and academic performance has been empirically proven, researchers (Lee, 2010; Mullen et al., 2005; Martirosyan et al., 2015) have not provided empirical evidence when investigating whether student satisfaction can mediate the relationship between technostress and performance expectancy. By examining the role of student satisfaction as a mediator in the relationship between technostress and performance expectancy among university students, this study aimed to fill the research gap. In a nutshell, the purpose of this study was to investigate how technostress affects student satisfaction and the connection between that factor and performance expectancy. This study also aimed to identify how student satisfaction could be used to measure the indirect effects of technostress on performance expectancy.
There are seven sections in this essay. The context of the phenomenon, the importance of the study, and how to grasp the study's objective are all presented in the introduction. The literature review, which is the second section, covers the results of the most recent studies on technostress, student satisfaction, and performance expectancy. The research's methodological design, which is the third section, describes how the findings are produced. The results are presented in the fourth section, which is separated into two parts: an evaluation of the structural model and an assessment of the measurement model. The discussion and implications come in at part five, and the conclusion comes in at part six. The bibliographic references are in the final section.
Literature Review
Person-environment fit theory
A good fit between a person's characteristics and the environment is referred to as "person and environment (P-E) fit" (Tang et al., 2018). While the environment element includes organization, tasks, and people, the person component relates to the need, abilities, and skills of the individual to demonstrate a successful performance (Edwards et al., 1998). The environment has an impact on the person, but so does the other way around. The degree to which a person is motivated, their behaviour, and their mental and physical health can all be impacted by how well they fit into their environment. The P-E fit theory is frequently applied to examine personal commitment (Zhang & Cui, 2018), performance, and satisfaction (Chuang et al., 2016).
In education, P-E fit describe how comparable students' traits are to their academic environment (Rocconi et al., 2020). Therefore, for the purpose of this study, P-E fit theory thus concentrates on how a student is matched with the ODL environment. When these two things mismatch, stress occurs. When a student's needs are not met by the ODL environment or when they are unable to meet the demands of the ODL environment, stress results. The impact of technology on students in higher education is a topic that researchers are discussing more and more, especially in light of the Covid-19 pandemic. Students must use online learning, and they need to complete their academic assessments, projects, and assignments using technology. A misfit between an individual and their ODL environment may result in technological stress, which can lead to unfavourable feelings, health issues, and discontent (Wang et al., 2020; Jena, 2015b). In addition, people also experience technostress when they are unable to manage technology in a healthy way.
The effects of technology that push students to finish assignments faster and overusing technology such as mobile phones will contribute to technostress. During the first online assessment, Davies (2015) found that students experienced technology anxiety and technostress, which emphasized the value of knowledge and ability to deal with the environment. However, Qi (2019) discovered that students’ use of technology and online learning did not affect their technostress. According to Upadhyaya and Vrinda (2020), technology could enhance learning with minor modifications to the teaching method, and students experienced a moderate level of technostress if they suited with the method. Although the advantages of using technology are apparent, there has been growing interests in understanding the impacts of technostress on student’s performance.
Therefore, when applying this theory to the situation of university students, we may expect that the results in terms of their academic performance will be better the bigger the increase in students' P-E fit. Researchers in the current study hypothesised that the mismatch between students' competence (i.e., their IT skills) and the new learning environment (i.e., using ODL) has led to technostress, which in turn has impacted student satisfaction and performance expectancy. In other words, technostress developed when a student's needs were not met by the environment or when their abilities were insufficient to handle the demands of the ODL setting.
Technostress and student satisfaction
Due to government incentives and to meet the expectations of students, the usage of technology in academia has increased dramatically (Dunn & Kennedy, 2019). Through applications like student life cycle management (Wang et al., 2020), learning management systems (LMS), MOOCs, integrated digital-based assessment (Barana et al., 2016), and attendance management system, technology is being used in academic administration and student self-service (Ofelia et al., 2017). It has been discovered that MOOCs and online learning can help students afford their higher education (Deming et al., 2015). Students were seen to view the use of ICT in the classroom favourably (Vahedi et al., 2021). ICT decreased bureaucracy and distance constraints for institutions of higher learning (Agarwal & Mittal, 2018). In addition, ICT enables institutions of higher learning to streamline academic data processing, introduce transparency, and integrate academic administration. It is thought that incorporating technology into the classroom will enhance the quality of instruction (Mirzajani et al., 2016).
Technostress results from widespread usage of technology. Technostress was originally mentioned by Brod (1984). It is an adaptation issue brought on by people's inability to deal with technology. In the context of the current study, the term "technostress" specifically refers to stress related to the use of technology in ODL contexts. The intensity of technostress is made worse by the rapid expansion of applications and technology-based learning (Lee et al., 2014). But in order to create meaningful teaching and learning processes, the usage of information and communication technology is crucial. To boost productivity, efficiency, and accuracy, it is therefore essential to study and employ the most recent technologies. Therefore, we anticipated that student participation in ODL would raise technostress, leading to higher levels of stress in students. Five components of technostress were identified by Tarafdar et al. (2007) and highlighted common instances where computer technology could potentially cause technostress. They also further created and validated a technostress assessment scale. In this study, four factors, namely techno-overload, techno-complexity, techno-insecurity, and techno-uncertainty, were chosen to represent technostress in the context of ODL.
First, techno-overload refers to circumstances when the usage of ICT compels students to complete more work more quickly in a shorter amount of time (Walz, 2012). Information overload, interruptions, and multitasking occur from the ability to digest several real-time information streams simultaneously provided by social networking, collaborative, and online learning platform devices. Information fatigue results from users being exposed to more information than they can adequately process and utilise. Users are under pressure to respond to information as soon as it is received by interruptions like WhatsApp messages and text-based workflow-related notifications. This causes tension, anxiety, and workflow detachment and makes it challenging to pay attention for an extended period of time. Students who multitask use several programmes and tasks at once, completing more work in less time, and feeling stressed Second, techno-complexity refers to circumstances in which ICT's complexity compels students to invest time and energy in learning and comprehending software or applications (Tarafdar et al., 2007). Some users find unfamiliar software, apps, and operations intimidating and challenging to understand, which leads to inadequacy and failure to finish the task (Ayyagari, 2012). Learning new software can take months, and some instructions are cumbersome and difficult to understand. As a result, users may find different programmes, features, and lingo frightening and difficult to grasp, which can lead to stress.
Third, techno-insecurity develops when users worry that others who are more knowledgeable about new ICT may surpass them in terms of their ability to learn. As a result, the current student can feel uneasy or sceptical of ICT, which might cause tension and stress. Techno-insecurity arises from instances where ICT users fear they will do worse than others who are more knowledgeable about new ICT tools (Tarafdar et al., 2007). There is a growing need for students who can utilise and operate ICT tools as ICT tool availability grows. Existing students may thus feel insecure, which causes stress and tension (Ayyagari, 2012).
Fourth, techno-uncertainty describes situations when ongoing ICT changes and upgrades prevent students from building a foundation of experience for a specific application or system (Tarafdar et al., 2007). This worries them since their knowledge quickly becomes outdated. Students and users must constantly learn new skills and applications due to computer system upgrades and changes (Ayyagari, 2012). Although they could be excited to learn new programmes and technologies at first, the ongoing need for upgrading and refreshing might eventually cause dissatisfaction and anxiety.
Research on technostress among students is crucial since higher education has adopted advanced teaching and learning on a large scale. This is certainly relevant with university students, who frequently experience more technology exposure than students in other school levels (Dunn & Kennedy, 2019). The extensive use of mobile technologies, social media, and other educational technology including e-learning platforms, online resources, online tests, and others could be the cause of certain university students experiencing high levels of technostress (Lee et al., 2014). It is crucial to comprehend how students' use of educational technologies is impacted by technostress. In addition to having a negative effect on the adoption and continued use of information systems (Joo et al., 2017; Maier et al., 2015), technostress can also have an adverse effect on useful information systems.
Different disciplines have different views on what constitutes student satisfaction with online learning. Feedback and support services from instructors (Lee, 2010), social presence (Richardson & Swan, 2003), flexibility in a course and course technology (McGorry, 2003), and learner interaction and collaboration (Lorenzo & Moore, 2002) are essential to the student learning experience, and thus, student satisfaction. According to a case study by Davies (2015), technostress, test anxiety, and computer anxiety were all mentioned by first-year psychology undergraduate students who completed their first online MCQ Assessment.
In a study of 740 undergraduate students from three public institutions in China, Wang et al. (2020) looked at the relationships between the dimensions of technostress and how they anticipated students' exhaustion, endurance in online learning, and performance expectancy. The frequency of technostress among youth population, aged 18 to 28, was evaluated by Upadhyaya and Vrinda (2020). The technostress instrument was cross validated in their study using a sample of 673 Indian undergraduate students. Students are now required to use technology to complete all of their coursework, including exams. The results showed that students who have a moderate level of technostress, have a significant impact on their satisfaction with education and their productivity in classroom. Therefore, we derive the first hypothesis based on the aforementioned discussions:
- Hypothesis 1: There is a significant relationship between technostress and student satisfaction.
Student satisfaction and performance expectancy
Satisfaction refers to an individual's emotional evaluation of a service based on encounters or past experiences (Aziz et al., 2022). The degree to which a learning environment promotes academic performance and the gap between expectations and what is received from the service provider are all factors that contribute to student happiness (Aziz et al., 2022; Lo, 2010). Introducing more interactions, providing students feedback promptly (Sun et al., 2008), diversifying learning and evaluation activities as well as assuring the effectiveness of the digital technologies employed (Kranzow, 2013; Sun et al., 2008) can all contribute to higher student satisfaction.
According to research on the association between student satisfaction and learning outcome (Lee et al., 2011), student satisfaction with online learning is related to performance expectancy. Another study discovered a connection between students' course satisfaction and their final grades (Lee, 2010; Mullen & Tallent-Runnels, 2006; Martirosyan et al., 2015). Their findings show that students’ academic results are improved when they are satisfied. Additionally, Dhaqane and Afrah (2016) found that student satisfaction promotes learning achievement and student retention. The aforementioned findings suggest that student satisfaction is vital, and as a result, issues regarding their performance should gain crucial attention (Suldo et al., 2015). As a result, we suggest that student satisfaction is an important factor for determining performance expectancy. Hence, this leads to our second hypothesis:
- Hypothesis 2: There is a significant relationship between student satisfaction and performance expectancy.
Effects of student satisfaction as a mediator in the technostress and performance expectancy relationship
Technology is a useful teaching tool, but Tang and Austin (2009) found that using it in the classroom did not always result in better performance and satisfaction. According to Jena (2015a), in the organizational context, the rise of occupational stress due to technology inability negatively impacts individual satisfaction, thus lowering organizational performance and commitment. Jena’s study revealed that technostress negatively impacted personal satisfaction due to the fast-changing ICT trend and stressful environment. This claim is supported by further research, which demonstrates that disparities in students' academic performance are a result of their satisfaction with the use of technology in online learning (Dhaqane & Afrah, 2016; Lee, 2010; Mullen & Tallent-Runnels, 2006; Martirosyan et al., 2015). Specifically, in expecting high performance, students with high satisfaction towards online learning can reduce technostress.
On the other hand, dissatisfaction with online learning will increase technostress and lower performance expectancy. As previously suggested, we expect students experiencing technostress to be less satisfied with ODL. Furthermore, we expect that students who are less satisfied with ODL will lower their performance expectancy. Finally, we propose that student satisfaction disparities explain the relationship between technostress and performance expectancy by acting as a buffer against performance expectancy related to technostress experienced by students. Hence, we posit the following hypothesis:
- Hypothesis 3: Student satisfaction towards online learning mediates the relationship between technostress and performance expectancy.
Methodology
Research framework
A research model was created based on the literature to investigate how technostress affects student satisfaction and how this indirectly affects performance expectancy through student satisfaction. Figure 1 depicts the conceptual framework of the present research.
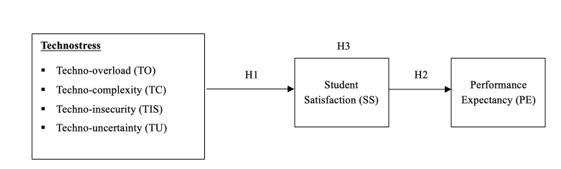
Overall, the research framework showed how technostress, student satisfaction, and performance expectancy are related. Technostress was an exogenous construct, student satisfaction was a mediator construct, and performance expectancy was an endogenous construct in the empirical model. It is also suggested that student satisfaction with ODL acts as a mediator in the interaction between technostress and performance expectancy.
Instrument
The questionnaire, which had 31 items and measured six variables and five demographic characteristics, was adapted from other studies (Aziz & Yazid, 2021; Aziz et al., 2021; Aziz et al., 2022; Ya'acob & Aziz, 2021). The four components of technostress, namely techno- overload, techno-complexity, techno-insecurity, and techno-uncertainty, were each measured by a total of 17 items, with a Likert scale of 1 for "strongly disagree" to 10 for "strongly agree". Eight questions rated student satisfaction, while six questions examined performance expectancy.
Results
Descriptive analysis
A cross-sectional study was performed by collecting data using an online self-administrative survey. Studies using cross-sections are commonly performed on a sample of university students (Abushammala et al., 2021; Kader, Aziz, Zaki, Ishak et al., 2022; Kader et al., 2022a; Kader et al., 2022b). The sample comprised of 402 students (234 female and 168 male) studying at Universiti Teknologi MARA (UiTM), which only admitted Bumiputera. With respondents’ ages ranging from 18 to 21, their average age was 19.5 years, and there were no appreciable gender disparities. 25.87% of the participants (104 in total) resided in an urban residential area while attending ODL. In contrast, 26.36% (106 participants) lived in a rural area, while 47.76% (192 participants) resided in a suburban residential area.
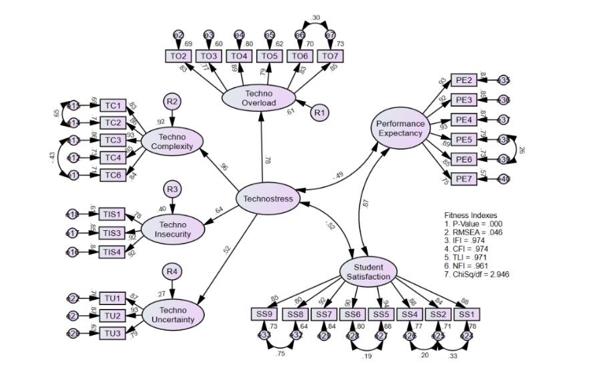
The findings of the CFA for the six variables and 31 items are displayed in Figure 2. The CFA was performed to see if the proposed factor model fit the data under investigation. According to the CFA results, the goodness-of-fit indices of the proposed model of technostress seem to correspond to the data very well, which are verified by the RMSEA = 0.046, IFI = 0.974, CFI =.974, TLI = 0.971, NFI = 0.961, and ChiSq/df = 2.946. The factor loadings for all items were larger than 0.60 and exceeded the threshold value of 0.50, further supporting the convergence validity. The outcomes are consistent with findings of Wang et al. (2020).
The average variance extracted (AVE) and composite reliability (CR) of CFA are shown in Table 1. All constructs demonstrated strong internal consistency. As shown in Table 1, all constructs had factor loadings that ranged from 0.825 to 0.964, exceeding the cutoff value of 0.60 (Zainudin, 2014). The outcomes also demonstrated the construct was reliable and the average variance score derived from the various components. The AVE values of the constructs, which ranged from 0.553 to 0.776 and were all higher than 0.5 (Zainudin, 2014), further proving the validity of the convergent constructs. As a result, we came to the conclusion that the construct validity satisfied convergent validity.
The correlation coefficients between the constructs are shown in Table 2. The values for the diagonals (shown in bold in Table 2) are higher, compared to those for the rows and columns. Since the square root of the average variance extracted (AVE) of each construct (shown in bold) was larger than the correlation coefficients between the construct and all other constructs, the results demonstrated that the requirements for discriminant validity were satisfied (Zainudin, 2014). As a result, we deduced that the discriminant validity was met. Together, we came to the conclusion that the model had significant path coefficients, acceptable reliability, and validity, and it fit the observed data.
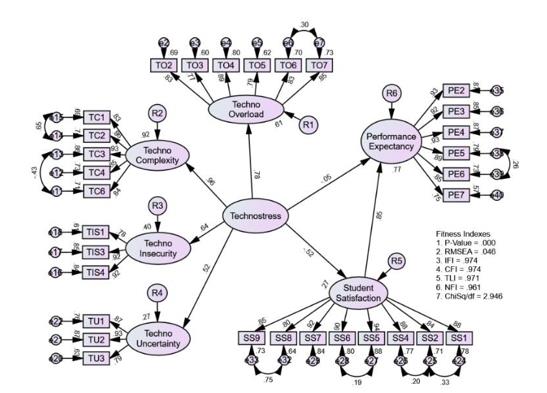
SEM-AMOS was employed in the current investigation to assess the measurement model and test the hypotheses. The regression path coefficients of the direct association between the constructs are displayed in Figure 3 and Table 3. Figure 3 shows the correlation between technostress and student satisfaction with an R2 value of 0.27. According to the data, all four dimensions reduce student satisfaction with ODL by 27%. With an R2 value of 0.77, Figure 3 further supports that student satisfaction is a crucial determinant in performance expectancy. According to the data, student satisfaction contributes 77% to performance expectancy. Technostress thus had a substantial impact on student satisfaction with ODL (β =-0.888 p=0.001). Additionally, the results showed a strong positive relationship between student satisfaction and performance expectancy (β = 0.912, p=.001). Thus, H1 and H2 were supported.
Mediation test
The outcomes showed that student satisfaction mediated the interaction between technostress and performance expectancy, which addressed the direct association between technostress and student satisfaction (see Table 4). The mediating impact showed how technostress was linked to performance expectancy via student satisfaction. Furthermore, it could be inferred that student satisfaction had full mediation effects since, after the mediator was included in the model, the direct impact of technostress on performance expectancy became insignificant. The third hypothesis (H3) is therefore supported.
Discussion
This study examines the role of student satisfaction in mediating the relationship between technostress and performance expectancy. Regarding the study's goals, the results revealed a significant negative relationship between technostress and student satisfaction (β = -0.888, p = 0.000). As a result, H1 was validated and supported by Wang et al. (2020), that indicated technostress was positively associated with student burnout, negatively impacting their academic performance. This suggests that when students face high technostress, they might lose their motivation to engage in academic endeavours (Kader et al., 2022a). It could be challenging for the pupils to adjust to the ODL environment. The students might feel threatened or frustrated by having no option but to face this new environment. Accordingly, technostress is in the dimension of mismatch between the student need and abilities, and the sources and requirements from the university related to technology-overload, technology-complexity, technology-insecurity, and technology- uncertainty when facing with education learning technology, consequently affecting their performance.
Apart from the study, it was discovered that student satisfaction was positively significant to performance expectancy (β = 0.912, p = 0.000). Thus, H2 was supported. The result was consistent with those of Ghaderizefreh and Hoover (2018), who demonstrated a significant and positive association between student satisfaction and academic success. Besides that, Sae et al. (2016) studied technology-induced stressors like work overload and invasion, and found the stressors to cause tremendous stress, reducing job satisfaction among the workers and lowering workers’ productivity. Therefore, this study suggests that students will have lower ODL satisfaction because of techno-overload when students have more work and need to work faster in a shorter period of time. Moreover, the complexity associated with ICT will decrease student satisfaction towards ODL. The high level of technostress also contributes to techno-complexity when the students do not understand how to use a software or application, and cannot adapt with any upgrades or changes to ICT, leading to lower satisfaction among them and decreasing the performance expectancy in the academic.
According to the findings of H3, the association between technostress and performance expectancy is totally mediated by student satisfaction with ODL. A prior study shows some similarities with our research; university students with high levels of stress caused by advance technology experienced low levels of satisfaction with life, lowering their academic performance (Samaha & Hawi, 2016). Technostress affected university students’ performance expectancy indirectly through the student satisfaction in online learning. This result was in line with a prior study by Wang et al. (2020), which found that technostress on multiple dimensions of misfit frequently had an inverse impact on individuals’ well-being, making them feel frustrated, incapable and exhausted, eventually leading to a decline in cognition and academic performance (Salanova et al., 2009).
Theoretical and Managerial Implication
This research expands understanding and knowledge by presenting significant empirical evidence on the association between technostress and performance expectancy, which is fully mediated by the student satisfaction towards open and distance learning (ODL). There is very little empirical evidence concerning this issue, particularly the impact on students themselves. Previous studies only focus on the effects of academician rather than students. The research's findings are compatible with the person- environment (P-E) fit paradigm. Our result shows that technostress will impact the performance expectancy when the students are satisfied with ODL. We believe such linkage is essential to manage the effects of technostress on student satisfaction, consequently leading to a better performance expectancy of students (Fuglseth & Sørebø, 2014). The current study results support the theory of P-E, suggesting that a good fit between the students’ ability and ODL environment will lead to a reasonable outcome, and affect students’ level of stress and performance expectancy.
The findings of this study also have a useful bearing on the university's efforts to lessen technostress during ODL. The institution must schedule and plan academic work so that students don't feel overly stressed about finishing assignments on time and maintaining a healthy lifestyle. The institution must also locate appropriate, approachable, and well-known software or apps so that students may readily grasp them. In addition, the university may provide students with appropriate training on how to use the application or software in order to introduce them to it early, to improve their ICT abilities, and to promote the exchange of ICT knowledge among them (Chung et al., 2020). As a result, student satisfaction with ODL rises, which eventually raises expectations for their performance expectancy.
Conclusion
This research study enriches the body of literature by analysing the mediating effects of student satisfaction on the link between technostress and performance expectancy among university students. Technostress has a considerable, unfavourable impact on student satisfaction. The incompatibility between the students' technological proficiency and the ODL environment, which was abruptly implemented during the Covid-19 pandemic, may be the cause of the students' stress. The management of the university must be aware of any potential negative impacts of ODL since, if they are not properly managed, they will directly affect the academic performance of the students. The management of the institution must always ensure that the students are tech-ready and have the necessary skills to prevent negative consequences from technostress, especially for Gen-Z students (Samat et al., 2020). Since our study was restricted to the study population, the results had limited generalizability. Personality traits may be used as a potential moderator in future studies that are replicated to other universities with larger sample sizes in order to obtain more understanding insights.
References
Abushammala, M., Qazi, W., & Manchiryal, R. K. (2021). The impact of COVID-19 on the private higher education system and students in Oman. Journal of University Teaching and Learning Practice, 18(3). DOI:
Agarwal, A. K., & Mittal, G. K. (2018). The role of ICT in higher education for the 21st century: ICT as change agent for education. Multidisciplinary Higher Education, Research, Dynamics & Concepts: Opportunities & Challenges for Sustainable Development, 1(1), 76–83.
Agogo, D., & Hess, T. J. (2015). Technostress and Technology Induced State Anxiety: Scale Development and Implications. Thirty Sixth International Conference on Information Systems (ICIS), (December), 1–11.
Ayyagari, R. (2012). Impact of information overload and task-technology fit on technostress. SAIS 2012 Proceedings. Retrieved from https://aisel.aisnet.org/sais2012/4/
Aziz, N. N. A., Kader, M. A. R. A., & Halim, R. A. (2021). The Impact of Technostress on Student Satisfaction and Performance Expectancy. Asian Journal of University Education, 17(4), 538-552. DOI:
Aziz, N. N. A., & Yazid, Z. N. A. (2021). Exploratory Factor Analysis of Technostress among University Students. International Journal of Academic Research in Progressive Education and Development, 10(3), 161–175. DOI:
Barana, A., Bogino, A., Fioravera, M., & Marchisio, M. (2016). Digital support for university guidance and improvement of study results. Procedia – Social and Behavioral Sciences, 228, 547–552. DOI:
Brod, C. (1984). Technostress: The Human Cost of the Computer Revolution. Addison- Wesley.
Chuang, A., Shen, C. T., & Judge, T. A. (2016). Development of a multidimensional instrument of person– environment fit: the Perceived Person–Environment Fit Scale (PPEFS). Applied Psychology, 65(1), 66-98. DOI:
Chung, E., Subramaniam, G., & Dass, L. C. (2020). Online learning readiness among university students in Malaysia amidst Covid-19. Asian Journal of University Education, 16(2), 45–58. DOI:
Davies, G. (2015). Online MCQ assessment anxiety amongst first year undergraduate psychology students: A case study. Journal of Perspectives in Applied Academic Practice, 3(1), 84–89. DOI:
Deming, D. J., Goldin, C., Katz, L. F., & Yachtman, N. (2015). Can online learning bend the higher education cost curve? American Economic Review, 100(5), 496–501. DOI:
Dhaqane, M. K., & Afrah, N. A. (2016). Satisfaction of student and academic performance in Benadir University. Journal of Education and Practice, 7(24), 59–63.
Dunn, T. J., & Kennedy, M. (2019). Technology Enhanced Learning in higher education, motivations, engagement and academic achievement. Computers and Education, 137(April), 104–113. DOI:
Edwards, J. R., Caplan, R. D., & Harrison, R. V. (1998). Person-environment fit theory: Conceptual foundations, empirical evidence, and directions for future research. In C. L. Cooper (Ed.), Theories of organizational stress (pp. 28-67). Oxford University Press.
Fuglseth, A. M., & Sørebø, O. (2014). The effects of technostress within the context of employee use of ICT. Computers in Human Behavior, 40, 161–170. DOI:
Ghaderizefreh, S., & Hoover, M. L. (2018). Student satisfaction with online learning in a blended course. International Journal of Digital Society, 9(3), 1393–1398. DOI:
Hwang, I., & Cha, O. (2018). Examining technostress creators and role stress as potential threats to employees’ information security compliance. Computers in Human Behavior, 81, 282–293. DOI:
Jena, R. K. (2015a). Impact of Technostress on Job Satisfaction: An Empirical Study among Indian Academician. The International Technology Management Review, 5(3), 117. DOI:
Jena, R. K. (2015b). Technostress in ICT enabled collaborative learning environment: An empirical study among Indian academicians. Computers in Human Behavior, 51, 1116–1123. DOI:
Joo, Y. J., Lim, K. Y., & Kim, N. H. (2016). The effects of secondary teachers’ technostress on the intention to use technology in South Korea. Computers and Education, 95, 114–122. DOI:
Joo, Y. J., Park, S., & Shin, E. K. (2017). Students' expectation, satisfaction, and continuance intention to use digital textbooks. Computers in Human Behavior, 69, 83–90. DOI:
Kader, M. A. R. A., Aziz, N. N. A., Zaki, S. M., Ishak, M., & Hazudin, S. F. (2022). The Effect of Technostress on Online Learning Behaviour among Undergraduates. Malaysian Journal of Learning and Instruction, 19(1), 183-211. DOI:
Kader, M. A. R. A., Aziz, N. N. A., Zaki, S. M., Mustapha, M., & Yazid, Z. N. A. (2022a). Understanding the Antecedents of Emotional Well-Being among University Students: Do Personality Traits Make a Difference? International Journal of Academic Research in Progressive Education and Development, 11(1), 771-784. DOI:
Kader, M. A. R. A., Aziz, N. N. A., Zaki, S. M., Mustapha, M., & Yazid, Z. N. A. (2022b). The Relationship between Online Learning Acceptance and Emotional Well-Being among Undergraduates. International Journal of Academic Research in Business and Social Sciences, 12(5), 1791–1808.
Kranzow, J. (2013). Faculty leadership in online education: Structuring courses to impact student satisfaction and persistence. Journal of Online Learning and Teaching, 9(1), 131.
Lee, J. (2010). Online support service quality, online learning acceptance, and student satisfaction. Internet and Higher Education, 13, 277-283. DOI:
Lee, S. J., Srinivasan, S., Trail, T., Lewis, D., & Lopez, S. (2011). Examining the relationship among student perception of support, course satisfaction, and learning outcomes in online learning. The Internet and Higher Education, 14(3), 158–163. DOI:
Lee, Y. K., Chang, C. T., Lin, Y., & Cheng, Z. H. (2014). The dark side of smartphone usage: Psychological traits, compulsive behavior and technostress. Computers in Human Behavior, 31, 373–383. DOI:
Lo, C. C. (2010). How student satisfaction factors affect perceived learning. The Journal of Scholarship of Teaching and Learning, 10(1), 47–54.
Lorenzo, G., & Moore, J. (2002, November). he Sloan Consortium Report to the Nation Five Pillars of Quality Online Education. https://www.immagic.com/eLibrary/ARCHIVES/GENERAL/ SLOANCUS/S021106L.pdf
Maier, C., Laumer, S., Weinert, C., & Weitzel, T. (2015). The effects of technostress and switching stress on discontinued use of social networking services: A study of Facebook use. Information Systems Journal, 25(3), 275–308. DOI:
Marchiori, D. M., Mainardes, E. W., & Rodrigues, R. G. (2019). Do Individual Characteristics Influence the Types of Technostress Reported by Workers? International Journal of Human-Computer Interaction, 35(3), 218–230.
Martirosyan, N. M., Hwang, E., & Wanjohi, R. (2015). Impact of english proficiency on academic performance of international students. Journal of International Students, 5(1), 60–71. DOI:
McGorry, S. Y. (2003). Measuring quality in online programs. The Internet and Higher Education, 6(2), 159-177. DOI:
Mirzajani, H., Mahmud, R., Fauzi Mohd Ayub, A., & Wong, S. L. (2016). Teachers’ acceptance of ICT and its integration in the classroom. Quality Assurance in Education, 24(1), 26–40. DOI:
Mullen, G. E., Narvaez, D., & Turner, J. (2005). Student Perceptions of Climate Influence Character and Motivation. Paper presented at the annual meeting of the American Educational Research Association in Montreal, Canada.
Mullen, G. E., & Tallent-Runnels, M. K. (2006). Student outcomes and perceptions of instructors' demands and support in online and traditional classrooms. The Internet and Higher Education, 9(4), 257-266. DOI:
Ofelia, M., Pedro, Z. S., & Heffernan, N. T. (2017). An integrated look at middle school engagement and learning in digital environments as precursors to college attendance. Technology, Knowledge, and Learning, 22(3), 243–270. DOI:
Qi, C. (2019). A double-edged sword? Exploring the impact of students’ academic usage of mobile devices on technostress and academic performance. Behaviour and Information Technology, 38(12), 1337–1354.
Ragu-Nathan, T. S., Tarafdar, M., Ragu-Nathan, B. S., & Tu, Q. (2008). The consequences of technostress for end users in organizations: Conceptual development and validation. Information Systems Research, 19(4), 417–433.
Richardson, J. C., & Swan, K. (2003). Examining social presence in online courses in relation to students’ perceived learning and satisfaction. Journal of Asynchorous Learning Networks, 7(1), 68–88. DOI:
Rocconi, L. M., Liu, X., & Pike, G. R. (2020). The impact of person-environment fit on grades, perceived gains, and satisfaction: an application of Holland's theory. Higher Education, 80, 857-874. DOI:
Sae, B. L., Sang, C. L., & Yung, H. S. (2016). Technostress from mobile communication and its impact on quality of life and productivity. Total Quality Management & Business Excellence, 27, 775-790.
Salanova, M., Schaufeli, W. B., Martinez, I., & Breso, E. (2009). How obstacles and facilitators predict academic performance: The mediating role of study burnout and engagement. Anxiety, Stress, & Coping, 26, 1–18. DOI:
Samaha, M., & Hawi, N. S. (2016). Relationships among smartphone addiction, stress, academic performance, and satisfaction with life. Computers in Human Behavior, 57, 321-325. DOI:
Samat, M. F., Awang, N. A., Hussin, S. N. A., & Nawi, F. A. M. (2020). Online distance learning amidst covid-19 pandemic among university students: a practicality of partial least squares structural equation modelling approach. Asian Journal of University Education, 16(3), 220–233. DOI:
Suharti, L., & Susanto, A. (2014). The impact of workload and technology competence on technostress and performance of employees. Indian Journal of Commerce and Management Studies, 5(2), 01-07.
Suldo, S. M., R. Minch, D., & Hearon, B. V. (2015). Adolescent Life Satisfaction and Personality Characteristics: Investigating Relationships Using a Five Factor Model. Journal of Happiness Studies, 16(4), 965–983.
Sun, P. C., Tsai, R. J., Finger, G., Chen, Y. Y., & Yeh, D. (2008). What Drives Successful e-Learning? An Empirical Investigation of the Critical Factors Influencing Learner Satisfaction, Computers & Education, 50, 1183–1202. DOI:
Tang, T. L. P., & Austin, M. J. (2009). Students’ perceptions of teaching technologies, application of technologies, and academic performance. Computers and Education, 53(4), 1241–1255. DOI:
Tang, Z., Chen, L., & Gillenson, M. L. (2018). How to keep brand fan page followers? The lens of person- environment fit theory. Information Technology & People, 31(4), 927-947. DOI:
Tarafdar, M., D’Arcy, J., Turel, O., & Gupta, A. (2015). The dark side of information technology. MIT Sloan Management Review, 56(2), 61–70.
Tarafdar, M., Pullins, E. B., & Ragu-nathan, T. S. (2015). Technostress: Negative Effect on Performance and Possible Mitigations. Information Systems Journal, 25(2), 103–132. DOI:
Tarafdar, M., Tu, Q., & Ragu-Nathan, T. (2010). Impact of technostress on end-user satisfaction and performance. Journal of Management Information Systems, 27(3), 303–334.
Tarafdar, M., Tu, Q., Ragu-Nathan, B. S., & Ragu-Nathan, T. S. (2007). The impact of technostress on role stress and productivity. Journal of Management Information Systems, 24(1), 301–328. DOI:
Upadhyaya, P., & Vrinda. (2020). Impact of technostress on academic productivity of university students. Education and Information Technologies, 26, 1647–1664.
Vahedi, Z., Zannella, L., & Want, S. C. (2021). Students’ use of information and communication technologies in the classroom: Uses, restriction, and integration. Active Learning in Higher Education, 22(3), 215-228. DOI:
Walz, K. (2012). Stress related issues due to too much technology: Effects on working professionals. MBA student scholarship paper 12. John & Wales University, Providence, RI.
Wang, X., Tan, S. C., & Li, L. (2020). Technostress in university students' technology-enhanced learning: An investigation from multidimensional person-environment misfit. Computers in Human Behavior, 105, 106208. DOI: 10.1016/j.chb.2019.106208
Warren, L., Reilly, D., Herdan, A., & Lin, Y. (2020). Self-efficacy, performance, and the role of blended learning. Journal of Applied Research in Higher Education, 13(1), 98-111. DOI:
Ya’acob, N. S., & Aziz, N. N. A. (2021). The technostress creator on educators’ job burnout in the virtual learning environment: a pilot study. Global Business and Management Research: An International Journal, 13(4s), 306-317.
Zainudin, A. (2014). A Handbook on Structural Equation Modeling. MPWS Rich Resources.
Zhang, J., & Cui, Q. (2018). Collaborative learning in higher nursing education: A systematic review. Journal of Professional Nursing, 34(5), 378–388.
Copyright information
This work is licensed under a Creative Commons Attribution-NonCommercial-NoDerivatives 4.0 International License.
About this article
Publication Date
18 August 2023
Article Doi
eBook ISBN
978-1-80296-963-4
Publisher
European Publisher
Volume
1
Print ISBN (optional)
-
Edition Number
1st Edition
Pages
1-1050
Subjects
Multi-disciplinary, Accounting, Finance, Economics, Business Management, Marketing, Entrepreneurship, Social Studies
Cite this article as:
Abd Aziz, N. N., Mat Yunus, N. K., Ishak, M., & Ya’acob, N. S. (2023). The Mediating Effects of Student Satisfaction on Technostress and Performance Expectancy. In A. H. Jaaffar, S. Buniamin, N. R. A. Rahman, N. S. Othman, N. Mohammad, S. Kasavan, N. E. A. B. Mohamad, Z. M. Saad, F. A. Ghani, & N. I. N. Redzuan (Eds.), Accelerating Transformation towards Sustainable and Resilient Business: Lessons Learned from the COVID-19 Crisis, vol 1. European Proceedings of Finance and Economics (pp. 834-848). European Publisher. https://doi.org/10.15405/epfe.23081.76