Abstract
The rapid growth of technology in the era of Industry 4.0 has caused the dynamic labor market to grow faster than ever before. This resulted in a mismatch between the jobs offered and the skills required. Thus, it raised the number of unemployability. The objective of this paper is to analyze the measurement of skill mismatch. Shortcomings and flaws in previous measurement methods and a broad definition of skill mismatch hindered the issues to be solved. The introduction of online job analysis has been seen as increasingly more valuable in measuring labor market conditions. Overcoming the issues such as cost, time lag, and biases, this measurement has been seen to be the new trend among scholars to shed the light on skill mismatch measurement. This paper analyzed 402 papers on online job data (vacancy, advertisement, portal) published from 2017 to 2022 from Scopus and Web of Science databases. Preferred Reporting Items for Systematic Review & Meta-Analyses (PRISMA) were used for this study. After the inclusion and exclusion criteria, ten papers from Scopus and five papers from the Web of Science database that matched with the criteria objective have been selected. Therefore, the study found that analyzing online job data is the new trend to be used in improving the labor market with more of the data could be used for the improvement to the previous method of measuring the skill mismatch problem.
Keywords: Skill mismatch, online job analysis, online job data, measurement issues
Introduction
The effect of the Covid-19 pandemic has brought unprecedented changes all over the world, especially in the economic and labor market (Hayashi & Matsuda, 2020). In Malaysia, it was reported that almost 100,000 workers were laid off and these included highly skilled workers where 13% of whom were managers, 26% were professionals, and 19% were technicians and associate professionals (Malaymail, 2020). Increasing job losses will lead to changing the field amongst the jobless to seek a new job. Some will retire, continue their study, or equip themselves with new skills (Tomaney et al., 1999). Thus, learning new skills, getting a more in-demand skill certificate, learning a trade, going to graduate school, or finishing college education will help them market themselves again in the job market (www.forbes.com). However, the problem occurs when the number of jobs offered is not equal to the demand. This situation has been rising long before the pandemic comes. The most obvious issue is the tight job market, leading to an occupational mismatch and unemployment. Thus, in the era of Industry 4.0, the growing rates of technology had changed the dynamism labor market to grow faster than before and caused the mismatch of jobs offered and the skill required. As supported by OECD (2017), rapid technological advances, demographic change, globalization, and shifts in labor market institutions are four megatrends that cause changes like work and skills mismatch. Thus, the emergence of technology from the Fourth Industrial Revolution (4IR) such as robotics, big data analytics, and artificial intelligence requires current organizations' different repercussion approaches to business decisions. Changes in technology bring innovation for the organization to immerse and enhance human capital and knowledge and, hence, replace millions of low- and middle-skilled workers.
Discussing the real definition of skill is still ambiguous and there is no consensus on it (Taweel, 2018). As supported by Green (2011), qualifications, competencies, education, and aptitudes are related elements in defining skills. Thus, different fields such as economics or psychology have different understandings of the meaning of skill. As various factors and causes contribute to this issue, there is a need for more studies to explore, define, and understand the other contexts of skills as they have broad typologies. Affirming the importance of employment skills, a study by Rodrigues et al. (2019) mentioned that skills are important in getting the job instead of certification but having a certificate is a requirement to get the job and recognition of the skills acquired. However, the debate about the mismatch occurs as certification does not indicate competency (JRC, 2014). Thus, this study only focused on skill mismatch, which is intertwined with the skills gap, skill shortage, and skill obsolesces as this type of mismatch is given more significance, greater use, and the rise of this concern among scholars to explore more on this issue (CEDEFOP, 2010; Senkrua, 2021).
Problem Statement
When discussing skill mismatch, the most highlighted issue is the method used to measure skill mismatch. As mentioned by Maltseva (2019), an unequivocal method has not been agreed upon among scholars or practitioners regarding the measurement of skill supply and demand, thus, most of the measurements are more focused on education as an optimal methodology of skill mismatch measurement. Therefore, when there is no exact diagnosis, no solution can be achieved to solve the issue. Instead of the best approach to fix the issue (Bosworth, 1993), measuring skill mismatch is affected by various factors that might be related, such as wages or social factors (wages increase and low discrimination) (Shah & Burke, 2003). Previous studies (Cárdenas Rubio, 2020; Maltseva, 2019; McGuinness & Pouliakas, 2017, Senkrua, 2021) mentioned skill mismatch measurements, both subjective (self-assessment) and objective, have their flaws that need to be understood. Table 1 describes the summary of skill mismatch measurement between subject/directive subjective measurement and objective/direct objective measurement. Measuring skill mismatch at the macro and micro levels has different approaches. The debate on measuring the micro-level of skill mismatch has been divided into three conditions (McGuinness & Pouliakas, 2017; Quintini, 2011), namely direct/indirect self-assessment, normative (job analysis), and statistical (realized match). However, Nedelkoska and Neffke (2019), and Rodríguez et al. (2021) defined four approaches where three are the same as those mentioned by Quintini (2011), except for the direct measurement of skills.
Amongst the listed measurements above, there is no agreement on the correct or exact way to measure skills mismatch (Nedelkoska & Neffke, 2019). As there are pros and cons to each measurement, the combination of each approach is the most recommended solution for measuring skills mismatch (Desjardins & Rubenson, 2011). Validity has become an issue in measuring all the approaches even though there are past studies that combined one to two approaches; however, no study has compared all the approaches (Nedelkoska & Neffke, 2019). Thus, the unavailability of data and the quality of data (McGuinness & Pouliakas, 2017) has made this measurement challenging to measure (Senkrua, 2021). Other than that, the three measurements that are listed above are more to measure skills mismatch in terms of over/under education. It has been argued that using education as a proxy ignores the linked job content and human capital which are associated with the skills required for the job whether through learning or working experience (Mavromaras et al., 2013. Even though the measurement of skills mismatch in terms of over-skilling or under-skilling applied almost the same concept such as direct assessment by HR specialists or Reflect Project data, this concept is subjective and prone to bias same as the measurement of over-education or under-education. The issue is the employee’s response whether their thinking is based on the current skills required or the skills expected in the future. Thus, what differentiates the measurement of over-skilling or under-skilling is a separate set of questionnaires is required compared to measuring over-education or under-education which can only be measured using the same set of questionnaires (McGuinness & Pouliakas, 2017).
Online job method
The transition of using online analysis emerged due to the flaws that occur in the traditional analysis measurement of skill mismatch. The traditional analysis was costly as it required a complex process to get complete information and had a time lag in understanding and acting, especially to the relevant parties such as policymakers, educational institutions, training providers, and job seekers to align with the labor market requirements, which were among the reasons it was adapted to online data. This is supported by a report from CEDEFOP (2019) which mentioned that providing information on the right skill at the right time are important elements for educational institutions, training centers, and career guidance for all sectors, regions, and countries to plan for their action. Besides that, responding promptly to the labor market requirements, especially for adults in upskilling and reskilling, and those younger in the TVET system is required by real-time information.
Thus, the analysis of online job portals has been seen as the source to measure the matching conditions for employees and employers due to the number of jobs offered for all types of occupations and the skills required. A study from Cárdenas Rubio (2020) also pointed out that skills required, occupational demand, details requirements from employers, and information about the labor market remain relatively unknown due to a survey conducted by the Columbia Office for National Statistics (DANE) that was not detailed enough to figure out all the situations. Thus, the use of Big Data in analyzing labor information has been seen as an approach to overcoming problems and providing valuable insight into the labor market (Cárdenas Rubio, 2020; Mytna Kurekova et al., 2014; Vankevich & Kalinouskaya, 2021). This method is considered relevant to be used as it offers advantages such as understanding the dynamic and skill demand in the labor market, especially for the labor market actors, and better career and skill development choices for the individual. Meanwhile, for employers or HR, the analysis of information will help in adjusting and developing policies, for better-informed decisions among policymakers, and for a training provider, guidance service, and employment service in improving their target for the future job market. The combination of the conventional method with online job analysis (OJV) has been seen as increasingly more valuable such as comprehensive, detailed, and timely in measuring labor market conditions.
Research Questions
The formulation of a research question for this study is guided by PICo which stands for Population or Problem, Interest, and Context. It is one of the tools used by researchers to conduct SLR and assist in formulating suitable research questions for review (Shaffril et al., 2021). For this study, skill mismatch has been defined as Problem, online data analysis is the Interest and the Context is measurement issues. As the main objective of this paper is to explore the trend and figure out the limitation of online data in measuring the skill mismatch, these research questions below have been formulated to a guided researcher in this study:
How many related literature studies of skill mismatch using online data have been published since
2017 to 2022)?
Does the existing literature solve the issue of measuring the skill mismatch?
What will be the direction of the future study in using online job data?
Purpose of the study
The emergence of big data has provided more data to discover. The comprehensive use of the statistical method by analytic systems and software allows the stakeholder such as educational institutions, companies, and governments to make the right decision, especially in solving complicated issues (Othman & Abdullah, 2019). As previous studies of skill mismatch measurement have discovered flaws in measurement, this study will extend the literature on skill mismatch measurement by investigating the trend and use of online job data which includes job advertisements, job portals, and job vacancies as a new way to measure the skill mismatch. This paper arrangement in Section 2 explains the method of searching for the literature review. Next Section 3 summarized the findings of the previous method of measuring skill mismatch by listing the advantages dan disadvantages of measurement. The findings of the paper will propose an alternative to measure the skill mismatch to outweigh the flaws in the previous measurement. The final section will conclude and state the limitation and future recommendations for the next study.
Research Method
Identification
The first step of this study started with the identification of keywords that are related to the topic that has been chosen. Similar terms of skill mismatch and online data have been searched using dictionaries, encyclopedias, thesauruses, and previous works. The authors broadened search terms and strategies by listing the skill mismatch words with skill shortage/skill gap, job mismatch, and online data with online job advertisement/online vacancy/online portals. Web of Sciences and Scopus are the two selected online databases that have been used to analyze the article's topic of skill mismatch and online job data. The collection consists of more than 35,000 journal articles from different subjects and publishers, which is the relevant justification for the selection of these two databases (Shaffril et al., 2019). Thus, as mentioned by Younger (2010), the use of more than one database is to ensure the selection of relevant articles on a particular topic. Below are the keywords used in this study (see Table 2):
Screening
Identifying and excluding redundancy is the first phase of screening the article. The criteria selected are based on the objective of this study. This is supported by Kitchenham and Charters (2007) who mentioned that research questions can be guided in determining the inclusion and exclusion criteria for screening. Thus, the criteria piloted were able to reliably interpret and classify the study correctly. The first screening phase has identified 1,104 papers that are related to the keywords, with a total of 62 papers published in Scopus and 1,042 papers from the Web of Science.
Eligibility
After completing the screening phase, the next step is to determine the eligibility of the articles. The authors have listed the inclusion and exclusion criteria as shown in the Table 3 to guide the selection of the article during the reviewing phase. The eligibility was conducted by database exclusion by selecting a paper timeline that was published between 2017 - 2022. Out of 58 papers, only one non-English paper was excluded. To match the author’s background, only related subjects from the social sciences, computer science, business management and accountancy, economics, econometrics, and finance were selected to be reviewed. Meanwhile, in the second stage of eligibility, the manual process of reading the abstract, keywords, and content of the article was examined throughout. This process is adopted by (Shaffril et al., 2019) to determine the eligibility of the selected papers. Finally, only fifteen articles have been selected to be reviewed, and the articles that are not related to the topic/or out of field area have been excluded.
Quality Appraisal
According to Kitchenham and Charters (2007), a quality appraisal is mandatory in preparing for an SLR. However, the issue of quality remains unsolved as it is hard to determine quality when it comes to the research method and validity of the findings (Yang et al., 2021). To complete the evaluation process, the researchers have adopted six quality assessments (QA) proposed by Kitchenham and Charters:
QA1: Is the purpose of this study clearly stated?
QA2: Is the interest and usefulness of the work clearly presented?
QA3: Is the study methodology clearly established?
QA4: Is the concept of approach clearly defined?
QA5: Is the work compared and measured with other similar work?
QA6: Are the limitations of work clearly mentioned?
A quality appraisal has been performed by the two authors of this paper. All the listed papers will be evaluated based on the score Yes = 1 Partially =0.5 No =0 and the final score has been combined to determine the selection based on the score given. All the 15 papers selected scored at least 0.5, and none of the papers scored 0.
Data abstraction and analysis
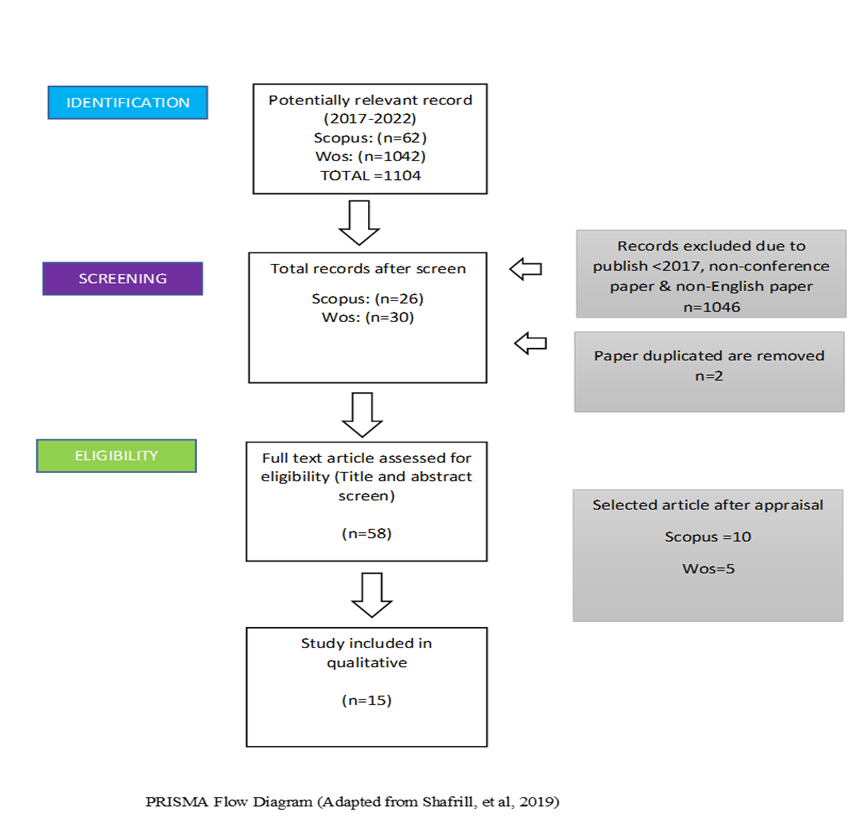
Findings
Research Question 1: How many relevant literature studies of skill mismatch using online data have been published since 2017- 2022?
The figure above shows the trend of papers published using online job data from the Scopus and WoS database as shown in the Table 4 based on the guideline show in the Figure 1. An advanced search from two online databases filtering “online job advertisement”, “online job vacancies”, and “online job portal” has shown 15 papers that have been published from 2017 to 2022, and the trend has greatly increased from 2018 to 2021. Most documents published were ruled by developed countries such as US and UK as the country already has established data such as European Standard Labor Market taxonomy (ESCO) and EURES, followed by Australia, Saudia Arabia, and one paper from the UAE. The rest of the studies came from developing countries such as Belarus, Russia, France, Malaysia, and China. The types of data used can be divided into two; the first is cross-sectional data and the second is in time-series data. Only two studies did not mention the year of data used: Mohamad Zamani et al. (2019) and Vankevich and Kalinouskaya (2021). However, both studies can be defined as using cross-sectional types of data as both used several subjects at the same point in time. This can be proven as Mohamad Zamani et al. (2019) used job advertisements posted on Facebook and Twitter based on public opinion to get more information about the job vacancy while Vankevich and Kalinouskaya, (2021) analyzed job vacancies and CVs to measure the mismatching in both requirements.
Since the focus of this paper is to analyze the use of online job data, fourteen related articles used online job data whether job advertisements, CVs, and both. The subject of studies was varied as some studies aimed directly at solving skill gaps (Almaleh et al., 2019; Baruah et al., 2018; Benhayoun & Lang, 2021; Hoang et al., 2018; Kranov & Khalaf, 2016; Lavrynenko et al., 2018). Meanwhile, the other studies focused on skill shortage (Dawson et al., 2019, 2020), skills mismatch (Ward et al., 2017), occupational mismatch (Turrell et al., 2021), and matching of demand and supply in the labor market (Vankevich & Kalinouskaya, 2021) and education ratio (de Pedraza et al., 2021). Even though different terms have been used, all terms refer to the skill mismatching issue as skill gaps, skill shortage, occupational mismatch, demand and supply in the labor market, and mismatching of qualification in education, referring to the situation of imbalance in demand and supply in the labor market. Therefore, one article from Hoang et al. (2018) has created a system to analyze the skill, demand, and supply of the labor market which indirectly overcomes the issue of mismatching in using online job data which is a job advertisement. The proposed system known as SKILL is a system to detect skill gaps in online recruitment. The analysis of job advertisements and job resumes is the data that use to identify labor market demand and supply. The automated system needed to be built up to detect the skill gaps and facilitate training and reskilling. The system has already been tested on CareerBuilder and the new version has made an improvement based on customer feedback. Hence, another study from Mohamad Zamani et al. (2019) also proposed features visualization of the job availability to overcome the lack of information on job advertisements which has led to the mismatch between applicants and the vacancy. The researchers proposed to analyze job advertisements based on public opinion from social media to get more information about the job vacancy. Assisting the job seeker in the searching process and saving time for the employer in arranging for the interview are the expected results of the proposed approach.
Research Question 2: Does the existing literature solve the issue of measuring the skill mismatch?
Skill gap
Out of fifteen reviewed articles, seven articles focused more on skill gaps as the main issue in the labor market when discussing skill mismatch. Each study has used various subjects and fields to confirm the situation of the skill gap in the labor market. This is supported by the finding from Baruah et al. (2018), which figured out the skills gap in renewable energy entrepreneurship, focusing on technical-oriented and management-oriented skills. Although management skills are preferred, certain technical skills remain necessary, as the entrepreneur must understand how to operate the wind system. Meanwhile, the other three authors (Almaleh et al., 2019; Benhayoun & Lang, 2021; Kranov & Khalaf, 2016) explored the skill gap issue from an educational perspective. Almaleh et al. (2019) stated there is a skill gap between a curriculum and a job advertisement. Therefore, the study has developed the Align My Curriculum framework to close the gap between the skills required by the market and curriculum developer. Next, Kranov and Khalaf (2016) conducted a study on the skills gap of engineering graduates. The preliminary study analysis stated there were different skills required for electrical engineers and mechanical engineers in terms of professional skills listed in the job advertisement, although the main responsibility is more on the technical and the advertisement is from the same company. Besides, the study also mentioned a challenge faced in continuing the study as the job advertisement for the entry-level engineer only contains three advertisements. Even though there is no result stated to confirm the skills gap issue, the situation stated there is an issue for the engineering graduates to enter the labor market.
Demand and supply
Studies by Ward et al. (2017); Tijdens et al. (2018); Zheng et al. (2021) and Vankevich and Kalinouskaya (2021), pointed out there is an imbalance of demand and supply in the labor market that leads to mismatching issues. Although the studies covered different scopes, however the use of online job data such as job advertisements and CV’s able to conclude the same scenario of the labor market. Vankevich and Kalinouskaya (2021) explore the mismatch by analyzing the job vacancy and CV from an online job portal. Results showed the imbalance of demand and supply for the competencies in CV and the vacancy. The findings reveal the need for training for the selected occupations such as accountant, sales engineer, and software engineer as there is a mismatch between competencies listed in CV and demanded by the labor market. Next is a study by Tijdens et al (2018) which confirmed that there is a mismatch between demand and supply in occupations from the job seeker perspective. The labor market analysis shows the high demand for a certain occupation, the lower skills, and education requirements. Thus, this has condensed the job market for low-skilled occupations and vice versa to skilled and highly skilled occupations in terms of educational requirements. A study by Zheng et al. (2021) which explores the demand and supply of the labor market by using education as a proxy for the measurement also indicates the same situation. The study reveals job seeker does overeducate between two and more years and are being penalized for wages. Compared to the finance industry, those in the IT industry will be penalized if they are overeducated. The findings of the study revealed those who are living in cities and graduating from key universities become less overeducated and are penalized as overeducated. Lastly, Ward et al. (2017) analyzed the mismatch of adjective words that have been used in the job advertisement. As generic skills are the transferable skill that is expected from graduates, this study explores the different expectations from the employer and higher education. The findings reveal that there was a gap between supply and demand requirements. However, the most questionable issue is the measurement or benchmark to state the generic skills owned by graduates as required by the employer. The unclear definition of skill required leads to an unclear measurement of skill earned by the graduates.
Skill shortage
On the topic of skill shortage, the use of online job data job advertisements, and CVs have been confirmed to predict and detect the shortage in the labor market. This is supported by a study from Dawson et al. (2019) which confirmed the skill shortage in the Australian labor market for the Data Science and Data Analytics and proposed five key variables for detecting shortage: (1) posting frequency; (2) salary levels; (3) education requirements; (4) experience demands; and (5) job ad posting predictability. Meanwhile, Dawson et al. (2020) explored the skill shortage measurement by machine learning prediction method and analyzed the demand and supply of labor. This method has been proven and aligns with the previous study stating the use of labor demand and supply is the best predictor of the skill shortage in the labor market. Instead of the variable stated in the previous study, the authors highlighted the hours worked variable as another indicator in measuring the skill shortage.
Occupational/Job mismatch
Only two studies explored occupational mismatch measured using online job vacancies. Therefore, both studies also covered the different scope of occupational mismatch. A study conducted by Turrell et al. (2021) explores mismatch and productivity. The interesting part of this paper is the clear explanation of how the authors overcome the bias of using online data. Therefore, the study has used online data and official statistics to compare the results. Meanwhile, Mohamad Zamani et al. (2019) explored the lack of information on job advertisements has led to the mismatch between applicants and the vacancy. The researcher proposed to analyze job advertisements based on public opinion from social media to get more information about the job vacancy.
Research Question 3: What is the future study direction?
The limitations and recommendations stated in this paper are based on the author’s suggestions from fifteen selected reviewed articles. The scope and subject of each paper are different, so the limitations that are reported are also different. This is due to the variety of online data that could be utilized to be covered and explored. However, several points could be highlighted for future studies that combine the analysis of online job data with other sources as stated by Lavrynenko et al. (2018) and Vankevich and Kalinouskaya (2021). The online source gives the real picture of the labor market, and when combined with another method such as an interview with an expert or compared with other data, will validate the findings of measuring the skill mismatch. This is aligned with the method applied in Figure 1 on how to overcome bias in using online data. It can be seen that different studies used different methods. However, some studies did not use comparison with other data or sources, such as Ward et al. (2017); Baruah et al. (2018); Mohamad Zamani et al. (2019), and Vankevich anf Kalinouskaya, (2021). All of these studies just confirmed the situation in the labor market, thus extensive solutions for the root causes of the problems are recommended for future study as it involves several important parties such as higher education, training centers, the government, and the job seekers themselves. However, the studies proposed by Mohamad Zamani et al. (2019) and Hoang et al. (2018) only focused on improving the system that has been built. Meanwhile, in measuring the skill mismatch, the use of education as a proxy is a proven weak indicator, as proven by Tijdens et al. (2018). Future studies on skill shortages could apply and test the variables proposed by Dawson et al. (2019) & Dawson et al. (2020) in other labor markets using other data sources, such as government databases. Overall, the author’s recommendation is to explore the study in a wider area as stated in the table 5 below.
Conclusion
In conclusion, the most highlighted issue of methods used to measure skills mismatch due to the differences in the definition of skills itself as different fields have different perspectives, has led the researchers to do a comprehensive literature review focusing on online job data research papers published between 2017 to 2022 from the Scopus and Web of Science databases. The research questions were answered through the review of seven articles that focused on skill gaps as the main issue in the labor market when discussing skill mismatch and four articles on supply and demand which pointed out that there is an imbalance of demand and supply in the labor market that leads to mismatching issues. In addition, two articles on skills shortage stated that the use of online job data job advertisements, and CVs have been confirmed to predict and detect the shortage in the labor market while another two studies explored the different scopes of occupational mismatch which was measured using online job vacancies. The method of searching for the literature review as explained in Section 2 and the findings of the previous method of measuring skills mismatch summarized in Section 3 concluded that the use of online job data which included job advertisements, job portals, and job vacancies has been seen as a new way of measuring rising skill mismatch issues such as skills gaps, skill shortages, and occupational mismatch.
Based on the literature review, as suggested by the authors, the researchers believe that further research should be undertaken to improve the methods used to measure skills mismatch by combining online data with other sources such as surveys and interviews with HR representatives, applying different variables and features as well as using deep learning to explore more about the skills shortage. Exploring the profession category and analyzing the experience required while widening the coverage for international and higher education levels and including the sample of jobs could be taken into consideration in coming out with a better method to measure skills mismatch.
Limitations
This study solely focuses on the issue of measuring skill mismatch from the previous journal that has been published with keywords such as skills shortage, skill gaps, and occupational/job mismatch. Thus, exploring the current journal and article using an online job advertisement confirmed the new measurement of the skill mismatch issue. Since there is a broad range of data that could be utilized from the job advertisement analysis, future study is recommended to explore more topics such as the analysis of skills demanded by industries and types of new and obsolete occupations that could be useful for the relevant parties in solving the skills mismatching issue in the labor market.
References
Alghamlas, M., & Alabduljabbar, R. (2019). Predicting the suitability of IT students' skills for the recruitment in Saudi Labor Market. In 2019 2nd International Conference on Computer Applications & Information Security (ICCAIS) (pp. 1-5). IEEE. DOI:
Allen, J., & Van der Velden, R. (2001). Educational mismatches versus skill mismatches: effects on wages, job satisfaction, and on‐the‐job search. Oxford economic papers, 53(3), 434-452. DOI:
Allen, J. P., Levels, M., & Van der Velden, R. K. W. (2013). Skill mismatch and skill use in developed countries: Evidence from the PIAAC study (No. 017). Maastricht University, Research Centre for Education and the Labour Market (ROA). DOI:
Almaleh, A., Aslam, M. A., Saeedi, K., & Aljohani, N. R. (2019). Align My Curriculum: A Framework to Bridge the Gap between Acquired University Curriculum and Required Market Skills. Sustainability, 11(9), 2607. DOI:
Baruah, B., Ward, T., Jackson, N., & Gbadebo, A. (2018). Addressing the skills gap for facilitating renewable energy entrepreneurship - An analysis of the wind energy sector. In 2018 Majan International Conference (MIC) (pp. 1-7). DOI:
Benhayoun, L., & Lang, D. (2021). Does higher education properly prepare graduates for the growing artificial intelligence market? Gaps’ identification using text mining. Human Systems Management, 40(5), 639-651. DOI:
Bosworth, D. (1993). Skill shortages in Britain. Scottish Journal of Political Economy, 40(3), 241-271. DOI:
Cárdenas Rubio, J. (2020). A web-based approach to measure skill mismatches and skills profiles for a developing country: the case of Colombia. Editorial Universidad del Rosario. DOI:
CEDEFOP. (2010). The skill matching challenge: Analysing skill mismatch and policy implications. Publications Office of the European Union.
CEDEFOP. (2019). The online job vacancy market in the EU: driving forces and emerging trends. Luxembourg: Publications Office. Cedefop research paper; No 72. http://data.europa.eu/doi/
Dawson, N., Rizoiu, M. A., Johnston, B., & Williams, M. A. (2019). Adaptively selecting occupations to detect skill shortages from online job ads. In 2019 IEEE international conference on big data (big data) (pp. 1637-1643). IEEE. DOI:
Dawson, N., Rizoiu, M. A., Johnston, B., & Williams, M. A. (2020). Predicting skill shortages in labor markets: A machine learning approach. In 2020 IEEE International Conference on Big Data (Big Data) (pp. 3052-3061). IEEE. DOI:
de Pedraza, P., Guzi, M., & Tijdens, K. (2021). Life satisfaction of employees, labour market tightness and matching efficiency. International Journal of Manpower, 42(3), 341-355. DOI:
Desjardins, R., & Rubenson, K. (2011). An analysis of skill mismatch using direct measures of skills.
Flisi, S., Goglio, V., Meroni, E. C., Rodrigues, M., & Vera-Toscano, E. (2017). Measuring occupational mismatch: Overeducation and overskill in Europe—Evidence from PIAAC. Social Indicators Research, 131(3), 1211-1249. DOI:
Green, F. (2011). What is Skill? An Inter-Disciplinary Synthesis. Centre for Learning and Life Chances in Knowledge Economies and Societies.
Green, F., & McIntosh, S. (2007). Is there a genuine under-utilization of skills amongst the over-qualified? Applied economics, 39(4), 427-439. DOI:
Hayashi, R., & Matsuda, N. (2020). COVID-19 Impact on Job Postings: Real-Time Assessment Using Bangladesh and Sri Lanka Online Job Portals. Asian Development Bank. DOI:
Hoang, P., Mahoney, T., Javed, F., & McNair, M. (2018). Large-scale occupational skills normalization for online recruitment. AI Magazine, 39(1), 5-14. DOI:
Hartog, J. (2000). Over-education and earnings: where are we, where should we go? Economics of education review, 19(2), 131-147. DOI:
JRC. (2014). European commission joint research centre, Occupational mismatch in Europe: Understanding overeducation and overskilling for policy making, Luxembourg: Publications Office of the European Union
Kitchenham, B., & Charters, S. (2007). Guidelines for performing systematic literature reviews in software engineering.
Kranov, A. A., & Khalaf, K. (2016). Investigating the employment gap: What employers want from engineering graduates. In 2016 IEEE Global Engineering Education Conference (EDUCON) (pp. 1198-1201). IEEE. DOI:
Lavrynenko, A., Shmatko, N., & Meissner, D. (2018). Managing skills for open innovation: the case of biotechnology. Management Decision. DOI:
Maltseva, V. (2019). The Concept of Skills Mismatch and the Problem of Measuring Cognitive Skills Mismatch in Cross-National Studies. Voprosy obrazovaniya / Educational Studies Moscow, 3, 43–76. DOI:
Mavromaras, K., Healy, J., Richardson, S., Sloane, P., Wei, Z., & Zhu, R. (2013). A system for monitoring shortages and surpluses in the market for skills. Report prepared by NILS, Flinders University for the Australian Workforce and Productivity Agency, 1-65.
McGuinness, S., & Pouliakas, K. (2017). Deconstructing Theories of Overeducation in Europe: A Wage Decomposition Approach. In Skill mismatch in labor markets. Emerald Publishing Limited. DOI:
Mohamad Zamani, N. A., Kamaruddin, N., Wahab, A., & Saat, N. S. (2019). Visualization of job availability based on text analytics localization approach. Indonesian Journal of Electrical Engineering and Computer Science, 16(2), 744. DOI:
Mytna Kurekova, L., Beblavy, M., & Thum, A. E. (2014). Using internet data to analyse the labour market: A methodological enquiry. IZA Discussion Paper 8555. DOI:
Malaymail. (2020, December 3). Nearly 100,000 lost jobs since January due to Covid-19, says deputy minister. https://www.malaymail.com/news/malaysia/2020/12/03/nearly-100000-workers-lost-jobs -due-to-covid-19-says-deputy-minister/1928475
Nedelkoska, L., & Neffke, F. (2019). Skill mismatch and skill transferability: Review of concepts and measurements. Papers in Evolutionary Economic Geography.
Organisation for Economic Co-operation and Development (OECD). (2013). OECD skills outlook 2013: First results from the survey of adult skills. OECD Publishing.
Organisation for Economic Co-operation and Development (OECD). (2017). Key Issues Paper, Meeting of the OECD Council at Ministerial Level, Paris 7-8 June 2017, https://www.oecd.org/mcm/documents/C-MIN-2017-2-EN.pdf
Othman, W. N. A. W., & Abdullah, A. (2019). Determining the Potential of Graduate Analytics Based on the iCGPA System: A Systematic Literature Review. IIUM Journal of Educational Studies, 7(2), 3-21. DOI:
Pellizzari, M., & Fichen, A. (2013). A new measure of skills mismatch: Theory and evidence from the survey of adult skills (PIAAC). DOI:
Perry, A., Wiederhold, S., & Ackermann-Piek, D. (2014). How can skill mismatch be measured? New approaches with PIAAC. Methods, data, analyses, 8(2), 38.
Quintini, G. (2011). Right for the Job: Over-qualified or Under-skilled?
Rodríguez, S. P., van der Velden, R., Huijts, T., & Jacobs, B. (2021). Identifying literacy and numeracy skill mismatch in OECD countries using the job analysis method.
Rodrigues, R., Butler, C. L., & Guest, D. (2019). Antecedents of protean and boundaryless career orientations: The role of core self-evaluations, perceived employability and social capital. Journal of Vocational Behavior, 110, 1-11. DOI:
Senkrua, A. (2021). A Review Paper on Skills Mismatch in Developed and Developing Countries. International Journal of Sustainable Development & World Policy, 10(1), 8-24. DOI:
Shah, C., & Burke, G. (2003). Skills Shortages: Concepts, Measurement and Implications. Working Paper No. 52. Centre for the Economics of Education and Training, Monash University.
Shaffril, H. A. M., Samah, A. A., & Samsuddin, S. F. (2021). Guidelines for developing a systematic literature review for studies related to climate change adaptation. Environmental Science and Pollution Research, 28(18), 22265-22277. DOI:
Shaffril, H. A. M., Samah, A. A., Samsuddin, S. F., & Ali, Z. (2019). Mirror-mirror on the wall, what climate change adaptation strategies are practiced by the Asian's fishermen of all? Journal of Cleaner Production, 232, 104-117. DOI:
Taweel, M. (2018). Technical and vocational education and training to address skills mismatch and unemployment: the case of Saudi Arabia [Doctoral Dissertation]. Kingston University.
Tomaney, J., Pike, A., & Cornford, J. (1999). Plant closure and the local economy: the case of Swan Hunter on Tyneside. Regional Studies, 33(5), 401-411. DOI:
Turrell, A., Speigner, B., Copple, D., Djumalieva, J., & Thurgood, J. (2021). Is the UK's productivity puzzle mostly driven by occupational mismatch? An analysis using big data on job vacancies. Labour Economics, 71, 102013. DOI:
Tijdens, K., Beblavý, M., & Thum-Thysen, A. (2018). Skill mismatch comparing educational requirements vs attainments by occupation. International Journal of Manpower, 39(8), 996-1009. DOI:
Van der Velden, R., & Bijlsma, I. (2017). Skill effort: A new theoretical perspective on the relation between skills, skill use, mismatches, and wages. (ROA Research Memoranda; No. 005). Research Centre forEducation and the Labour Market
Van der Velden, R. K., & Van Smoorenburg, M. S. M. (1997). The measurement of overeducation and undereducation: self-report vs. job-analyst method. Research Centre for Education and the Labour Market, Faculty of Economics and Business Administration, Maastricht University.
Vankevich, A., & Kalinouskaya, I. (2021). Better understanding of the labour market using Big Data. Ekonomia i Prawo. Economics and Law, 20(3), 677-692. DOI:
Ward, A., Baruah, B., & Gbadebo, A. (2017). How universities and employers specify competence in generic skills findings from an analysis of job advertisements. In 2017 16th International Conference on Information Technology Based Higher Education and Training (ITHET) (pp. 1-6). IEEE. DOI:
Yang, L., Zhang, H., Shen, H., Huang, X., Zhou, X., Rong, G., & Shao, D. (2021). Quality assessment in systematic literature reviews: A software engineering perspective. Information and Software Technology, 130, 106397. DOI:
Younger, P. (2010). Using Google Scholar to conduct a literature search. Nursing Standard, 24(45). DOI:
Zheng, Y., Zhang, X., & Zhu, Y. (2021). Overeducation, major mismatch, and return to higher education tiers: Evidence from novel data source of a major online recruitment platform in China. China Economic Review, 66, 101584. DOI:
Copyright information
This work is licensed under a Creative Commons Attribution-NonCommercial-NoDerivatives 4.0 International License.
About this article
Publication Date
18 August 2023
Article Doi
eBook ISBN
978-1-80296-963-4
Publisher
European Publisher
Volume
1
Print ISBN (optional)
-
Edition Number
1st Edition
Pages
1-1050
Subjects
Multi-disciplinary, Accounting, Finance, Economics, Business Management, Marketing, Entrepreneurship, Social Studies
Cite this article as:
Saad, Z. A., Abdul Rahman, H., Hussain, S., & Hasan, H. (2023). Systematic Literature Review: An Analysis of Skill Mismatch Measurement. In A. H. Jaaffar, S. Buniamin, N. R. A. Rahman, N. S. Othman, N. Mohammad, S. Kasavan, N. E. A. B. Mohamad, Z. M. Saad, F. A. Ghani, & N. I. N. Redzuan (Eds.), Accelerating Transformation towards Sustainable and Resilient Business: Lessons Learned from the COVID-19 Crisis, vol 1. European Proceedings of Finance and Economics (pp. 391-408). European Publisher. https://doi.org/10.15405/epfe.23081.34