Industrial Revolution 4.0 Digital Competencies Instrument for Measuring Aircraft Maintenance Technicians
Abstract
The integration of Industrial Revolution (IR) 4.0 technologies in the aviation industry requires well-trained and adaptable aircraft maintenance technicians (AMT). This study examines the gap in digital competency assessment in the initial training of Royal Malaysian Air Force (RMAF) technicians. To address this gap, the study develops a survey based on IR 4.0 digital competencies on seven dimensions, namely: problem-solving, communication, active learning, technical knowledge, analytical and critical thinking, technology skills and experience and lessons learned required for aircraft maintenance. The survey is validated by three expert panels specialising in aircraft maintenance and IR 4.0 readiness. A total of 234 AMTs participated in the survey, providing valuable demographic information about the respondents. Findings from the survey show a higher proportion of skilled AMT in the 21-30 and 31-40 age groups compared to low-skilled AMT. It is noteworthy that there are significant numbers of low-skilled and skilled AMTs in all squadrons. Many Air Corporals, Air Sergeants, and Flight Sergeants have high qualifications, but some have low qualifications. In addition, No. 12 Squadron has the highest percentage of low-skilled AMTs with less than five years of experience, while No. 10 Squadron has the highest percentage of those with more than ten years of experience. The descriptive analysis of the study sheds light on the skills and competencies of the AMT. From the results, there is a need to address and improve digital competencies, especially in the context of IR 4.0, to ensure a highly competent workforce in the aviation industry.
Keywords: Aircraft Maintenance, Digital Skills, Industrial Revolution 40, Skills Assessment
Introduction
The Royal Malaysian Air Force (RMAF) has established its genuine capacity, credibility, and supremacy as a national air power in terms of the effectiveness with which air operations defend national sovereignty. Every day, hundreds of flights are made by military planes for freight, air surveillance, and training. Each fleet of aircraft needs engineers and aircraft maintenance technicians (AMT) at least 100 over. The engineering branch of the RMAF annually verifies that all AMTs are adequately trained and skilled to perform their duties. Maintenance is necessary to keep the aircraft airworthy and reliable. Aircraft maintenance, which includes engine systems, armament systems, flight controls, electrical systems, avionics systems, on-the-job training, and advanced courses, are all part of this field (Thulasy et al., 2021). Aircraft mechanics are responsible for the maintenance and repair of planes and helicopters. Institute Technology of Aerospace (ITAS) is a training institute at Air Force College responsible for conducting basic aircraft technical training programs. Instruction in the classroom includes learning how to examine and repair aircraft parts, such as engines and airframes, as well as the usage of proper tools and other machinery. An aircraft maintenance technician’s speciality determines the duration of the modules and courses they attend.
However, as new aircraft or weapon systems are introduced, technicians must continually update their knowledge and expertise in order to keep up with the current advanced technology (Güneş et al., 2020). Fast-paced technological development and enormous variety characterise the aerospace industry. Though most aircraft last for 40 years, every 10 to 15 years, an upgrade is done with the latest technology and flying capabilities that can be customised to a given company's needs (International Air Transport Association, 2020). Therefore, it is essential for aviation industry professionals like aircraft engineers and technicians to continue to upgrade their skills in order to remain current and responsive to meet the changing needs of emerging technologies (Skills Future Singapore, 2017). Consequently, assessing the competence of aircraft maintenance specialists is essential. In addition, the results of the skill gap analysis might be helpful for RMAF training and education facilities to decide what IR 4.0-related programs and courses to offer to technicians. Therefore, modern aviation maintenance requires aircraft maintenance employees to undergo training and retraining so that they can incorporate new aircraft technologies and systems, especially those of the IR 4.0.
The goal of this study is to determine the level of IR 4.0-based skills possessed by RMAF AMT. IR 4.0-based skills are related to the digital competency of each technician in dealing with and handling aircraft maintenance tasks, which include aircraft maintenance information systems, sensor troubleshooting tasks, and avionics systems in preventive maintenance (Thulasy et al., 2022). This paper aims to discuss survey questionnaires used to assess the level of digital competencies to analyse technicians’ digital skills and competency levels and profiles. In addition, the paper also describes the descriptive analysis of the technician’s digital skill and competency levels based on seven (7) dimensions of digital constructs: problem-solving, communication, active learning, technical knowledge, analytical and critical thinking, technology skills and experience, and lessons learned in pertaining to IR 4.0 requirements.
The paper is structured as follows: Section 2 discusses background and related work on aircraft maintenance and digital competencies. Section 3 elaborates on the flows and methodology of the data analysis and visualisations. Followed by Section 4 presents the findings and analysis of AMT’s skills and competencies. Finally, Section 5 concludes with a summary of the research.
Background and Related Works
The RMAF's maintenance technicians encompass a range of roles, such as aircraft technicians, assistance technicians, and aircraft mechanics. These technicians are entrusted with a variety of responsibilities, including aircraft inspection, repair, alteration, servicing, and ground handling. In order to carry out their duties effectively within the domain of aircraft maintenance and repair operations, aviation maintenance technicians must possess the necessary technical knowledge, skills, and attitudes (Güneş et al., 2020). Ensuring the airworthiness of RMAF aircraft is an implicit requirement of their job. The trend of relying on instructor-led instruction for this task-oriented field has contributed significantly to its historical growth. Key professional attributes and qualities of an aviation maintenance technician encompass effective communication, problem-solving abilities, self- and time management skills, cooperative mindset, adaptability, sound judgment, and the ability to anticipate future needs (Thulasy et al., 2022).
It is crucial to assess how IR 4.0 will impact the current organisational structure, what new needs RMAF will meet, how these requirements differ from RMAF's previous work, and what training and expertise RMAF will need to do these jobs efficiently. As we enter the next phase of industrialisation, the maintenance sector will witness a tremendous deal of automation and integration (Thulasy et al., 2021). External considerations influence which tools, improvements, and automated systems can be used in the present. Intelligent robotic systems will communicate with on-site technicians, intelligent transportation systems will move items and maintenance responsibilities will be automated. With the aid of tablets, wearables, and other smart devices, real-time data will be collected and evaluated (Xu et al., 2018).
Numerous technical advances and pieces of equipment have been used to automate further and integrate plant devices via a base network (Liao et al., 2018). There will be ongoing data collection and analysis. The system's parameters will be fine-tuned using quality standards and historical data. In maintenance operations, condition-based monitoring will be emphasised more and more. Shortly before a machine breaks down, maintenance staff will be too concerned by the predicted repair activity for efficient management to do proactive or corrective maintenance. Inventory administration will become more efficient. From the time we get our raw materials until our equipment is operational, we will be able to offer you a more precise completion estimate.
Meanwhile, the organisation's structure is becoming more decentralised and adaptable. In general, future factories will have an organisational structure that is flatter, more fluid, decentralised, and flexible. A complex web of producing zones will exist. The requirement for data management and technology-savvy technicians will not be limited to a single industry, like the paint or welding shop. If technology is prioritised, jobs will be cycled and enhanced more regularly (Liao et al., 2018).
Additionally, technicians with many skill sets will be more difficult to categorise within a specific profession. There will be significantly fewer physically and cognitively demanding duties, such as those that are present in industries such as manufacturing (Lee et al., 2018). Therefore, technicians must learn to coexist with intelligent machines. Support systems will be very helpful, but experienced professionals must make the ultimate decisions. The implementation of these types of support systems requires cooperation not only between departments but also among employees.
As a result of the digital transformation, technicians will largely process information and data on a daily basis. Eventually, artificial intelligence (AI) will open the door for productive collaboration between machines and humans. In addition, interaction will be limited to voice and gesture alone, as opposed to pressing or touching buttons. As the major means of communication and control for many testing equipment, technicians will rely on smart smartphones and tablets. New technicians will be required to participate in process planning and optimisation efforts (Lee et al., 2018). Technicians would be required to perform fewer mundane tasks but would be tasked with greater process monitoring and management.
Numerous vocations, including those needing skills in diagnostics, machine problems, resource management (including human management and goal formulation), and so on, will be rendered obsolete by the widespread use of automation and artificial intelligence (World Economic Forum, 2014). Due to the escalating diversity and complexity of digital skills, businesses require systematic methods for measuring present competencies and preparing for the future (International Telecommunication Union, 2021).
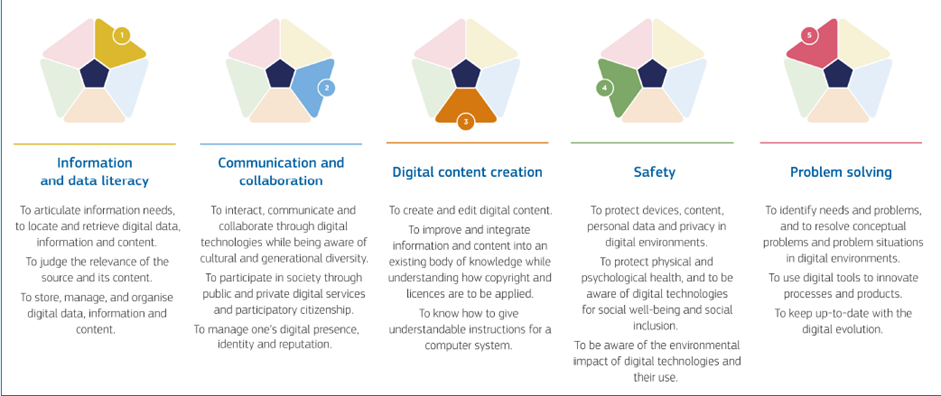
Figure 1 depicts a number of the digital competencies required in the IR 4.0 era. To be digitally competent, employees must be able to use ICT [Information and Communications Technology] and digital media to independently and effectively conduct tasks, solve problems, communicate and collaborate, digital content creation, safety, information and data literacy (Lappas & Kourousis, 2016).
Recently, new technologies have been the key incentive for the continual alterations in upkeep procedures. The underlying issues that need adjustments in viewpoint and strategy on the part of maintenance professionals and technicians, notably in the domains of competence and education, remain constant. Digital skills are not a binary but rather exist on a spectrum with varying levels of proficiency. This spectrum ranges from basic to more advanced skills. The types of digital skills can be categorised into three levels: basic, intermediate, and advanced (International Telecommunication Union, 2021). McKinsey & Co. determined that 60% of all vocations, including maintenance professionals, conduct at least 30% of the procedures that can be automated (Gahbauer, 2019). The estimate predicts that automation will disrupt economies worldwide by a whopping fifty per cent. Therefore, AMTs of the future will require training in digital troubleshooting so that they can go beyond what technology can tell them about an issue. They must get a fundamental knowledge of these tools and technologies in order to interact effectively, critically, and securely with new and emerging technology, particularly systems powered by AI, Vuorikari et al. (2022). Aircraft technicians of all ages will find basic troubleshooting skills and experience with computers and digital platforms useful in the aviation business in the future.
Method
For the experimental setup, the researcher is particularly interested in all RMAF aircraft maintenance technicians from the fleet that involved No. 8 Squadron (Transport aircraft), No. 10 Squadron (Helicopter) and No. 12 Squadron (Fighter aircraft), who work with IR 4.0 based aircraft maintenance and meet certain criteria. These AMTs come from various trade backgrounds and are involved in aircraft maintenance tasks across the RMAF's squadrons. They are also involved in providing the highest level of commitment to their work, and their contribution to the organisation's performance management is critical in ensuring that the engineering branch meets its objectives. A sample is a subset of a population. In many types of scientific research, samples are essential since it is impossible to include all of the individuals in a population for study design from both a vital and an asset standpoint. A basic random sample, a stratified sample, and a multi-stage sample are all examples of sampling (Setia, 2016). As a whole, there are about 600 technicians who are directly or indirectly involved in the daily operations of RMAF aircraft maintenance. Study sample based on ‘Determining Sample Size from a given population, 1970 table’ (Krejcie & Morgan, 1970). For the total population N = 600, its corresponding sample size, is S = 234. Hence, the minimum sample size to collect is 234 respondents.
Survey questionnaires development and validation
A research questionnaire is used to pursue dependable data collection. Thus, many researchers rely on survey or questionnaire validity and reliability. Case studies uncover fresh themes and confirm previous findings. The data can be analysed using descriptive approaches (Nimvyap & Benjamin, 2020) and organising sampling at a certain level dependent on parameters from previous stages, which improves conclusion accuracy (Mallik et al., 2020).
In the earlier stage of this research, there are seven dimensions of digital competency, which are problem-solving, communication, active learning, technical knowledge, analytical and critical thinking, technology skills and experience and lessons learned, for evaluating the skills and competence of AMT based on IR 4.0 that were identified in this study through systematic evaluations (Thulasy et al., 2022). These dimensions are regarded to give valuable scopes and boundaries for developing methods, instruments, or procedures for evaluating the acquisition, transmission, and growth of an individual's technical skills and competency (Güneş et al., 2020). Consequently, management can analyse the effectiveness and efficiency of training and certification programs for digital skills within the RMAF environment.
Step 1: Questionnaires development
Specifically, survey questionnaires are developed for inquiring about digital competency related to these multidimensional. The developed survey instrument consists of seven dimension digital competency constructs, which are listed in Table 1. Each construct has four items (questions) that assess the digital competency of the AMT, for example, “I can APPLY problem resolutions using the latest digital tools which may assist me in solving problems.” and “I can UNDERSTAND and communicate with other technicians during discussing aircraft rectification using digital test equipment.”. In the study, a Likert scale with five response options, namely (1- Strongly Disagree, 2 – Disagree, 3- Neutral, 4 – Agree, and 5- Strongly Agree), was used to assess AMT's responses to the questionnaires. This scale enables a more nuanced measurement compared to a nominal scale, as it captures degrees of agreement or disagreement.
Step 2: Instrument validation
Expert questionnaire evaluations are common. A few parties will assess the researcher's questionnaires. The groups can analyse whether the questions can expand on the issue. A question construction expert should analyse the survey to check for frequent mistakes, including unclear questions and linguistic issues. Taherdoost (2016) recommended that new instruments' content validity be assessed. Content validity ensures a new survey instrument covers all necessary elements and excludes undesired items from the concept domain. The judgmental content validity method (Quiroz et al., 2017) starts with a literature review and expert panel evaluation. For questionnaire validation, researchers and experts discuss content validity. Three expert panels were used to assess this study. Two panels have aircraft maintenance and a panel of IR 4.0 background. The panels evaluated questions using a validation and comment form, as stated in Table 2.
The next step in the validation process involves analysing the survey for content validity based on the marks given in the validation forms by the panels. The Context Validity Index (CVI) is an important tool for determining whether or not a questionnaire has been properly validated CVI (Elangovan & Sundaravel, 2021). Figure 2 illustrates the results of validation of Item-CVIs of 0.78 or higher, as well as Scale-level CVI Universal Agreement (S-CVI/UA) and S-CVI/Average of 0.8 and 0.9 or higher.
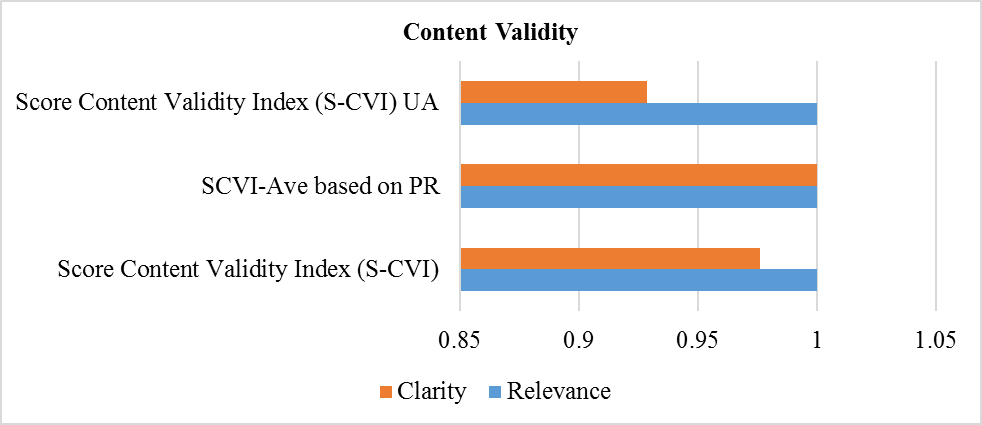
Step 3: Pilot study
During this phase, the researcher conducted a pilot survey utilising a questionnaire developed and verified by a panel. Before obtaining the final data, the preliminary survey responses helped the researcher make the necessary adjustments (Leon et al., 2011; Rahim et al., 2018). Convenience sampling was used to select the sample for the pilot survey because it is ideally suited for rapidly collecting data from easily accessible instances (Stratton, 2021). A group of 54 respondents were selected for this pilot survey.
Step 4: Validation using principal component analysis
Principal Components Analysis (PCA) is a statistical technique used to reduce the dimensionality of a dataset (Sainani, 2014). In this study, PCA is also used to determine the question of whether the same items should be included in the same factor, which is known as factor loading (Barry et al., 2017; Ghazali et al., 2021). Then, uniqueness denotes the variance that is 'unique' to the question and not shared with other questions. Table 3 depicts the Problem-Solving Analysis that the factor loading for each construct (RC1) value is between 0.840 and 0.898 to denote the item significance. The uniqueness values between 0.193 and 0.294 indicate that less than 30% of the variance in each question is not shared with other questions in the overall factor model. Fewer variance values among questions show they are highly relevant to the construct.
For Communication Analysis, Table 4 deliberates that the factor loading for each construct is between 0.831 and 0.913 values to denote the item significance. The uniqueness values between 0.167 and 0.309 indicate the variance of each question.
For Active Learning Analysis, Table 5 describes the factor loading each construct is between 0.724 and 0.893 values to denote the item significance. The uniqueness values between 0.203 and 0.476 indicate the variance of each question.
For Technical Knowledge Analysis, Table 6 shows the factor loading each construct is between 0.821 and 0.925 values to denote the items significant. The uniqueness values between 0.144 and 0.326 indicate the variance of each question.
For Analytical and Critical Thinking Analysis, Table 7 depicts the factor loading each construct is between 0.805 and 0.857 values to denote the item significance. The uniqueness values between 0.266 and 0.353 indicate the variance of each question.
For Technology Skills Analysis, Table 8 shows the factor loading each construct is between 0.823 and 0.903 values to denote the item significance. The uniqueness values between 0.184 and 0.322 indicate the variance of each question.
For Experience and Lesson Learned Analysis, Table 9 shows the factor loading each construct is between 0.906 and 0.936 values to denote the item significance. The uniqueness values between 0.125 and 0.179 indicate the variance of each question.
Seven Dimension Skills Scoring
These skills of the seven dimensions of the digital competency construct are problem-solving, communication, active learning, technical knowledge, analytical and critical thinking, technology skills and experience, and lessons learned. The scoring for calculating the dimensions of digital competency is based on a 5-point Likert scale in which the minimum score is one and the maximum is 5. The scores 1 to 3 are considered low to basic scores, and 4 to 5 are considered exceptional and high scores (Alkharusi, 2022; International Labour Organization, 2023). Then, each dimension consists of 4 items or questionnaires. The total score for each dimension is between 4 and 20. If the respondent scores between 4 and 12, he or she is considered low-skilled, whereas someone who scores between 13 and 20 is considered skilled.
Result and Analysis
Finally, the researcher administers data collection using the developed survey that has already been designed and validated by a panel of experts. Based on the total population of 600 technicians, the researcher selected a sample size 234. A stratified random sampling approach was employed to select a total of 234 AMTs for participation in the study. The AMTs were chosen randomly from three operational squadrons. Stratified random sampling involves dividing the population into distinct subgroups or strata, in this case, the three operational squadrons (Taherdoost, 2016). By ensuring representation from each squadron, the sampling technique increases the likelihood of obtaining a diverse and representative sample.
The analysis in this section is expected to discover the gaps in digital competency among selected RMAF aircraft maintenance technicians between squadrons. The descriptive analysis involves the respondent AMT from No. 8 Squadron, No. 10 Squadron, and No. 12 Squadron on differences of age, ranks, and years of experience compared to skills analysis, which are compiled in the following Figure 3.
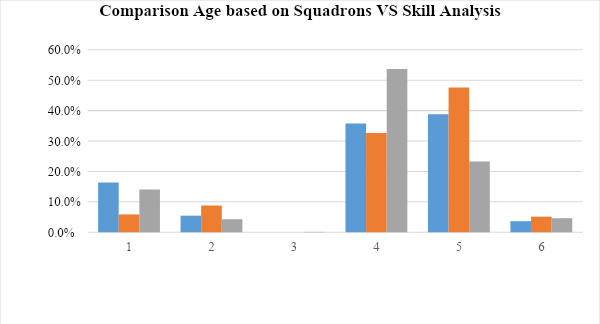
Figure 3 depicts the analysis based on Age group and skill among the technicians from three (3) squadrons. The bar graph divides the result into two (2) categories: Low Skilled and Skilled technicians in terms of their digital skills related to seven (7) dimensions of digital competency construct as assessment criteria. In the Low Skilled category, technicians aged between 21 -30 years old from No. 8 Squadron scored the highest with 16.36%, followed by No. 12 Squadron with 14.06% and No. 10 Squadron with 5.86%. Technicians who are aged between 31-40 years old from No. 10 Squadron scored 8.79%, No. 8 Squadron scored 5.45%, and No. 12 Squadron scored 4.27%; however, for those above the age of 40, only No. 12 Squadron scored 0.11%, and the other squadrons scored 0%.
In the Skilled category, the technician’s age group between 21 and 30 years old from No. 12 Squadron got 53.66%, followed by No. 8 Squadron with 35.76% and No. 10 Squadron with 32.26%. Technicians aged between 31-40 years old from No. 10 Squadron scored the highest with 47.62%, followed by No. 8 Squadron with 38.79% and No. 12 Squadron with 23.28%. Then, technicians who are above 40 years old from No. 10 Squadron scored 5.13%, No. 12 Squadron scored 4.61%, and No. 8 Squadron scored 3.63%. It can be concluded that although there were aircraft technicians with Low Skills in the age group of 21 to 30 years old, there were more Skilled aircraft technicians in the age groups of 21 to 30 years old and 31 to 40 years old. Nevertheless, there is still a percentage of Low Skilled technicians across the squadrons related to IR4.0 skills.
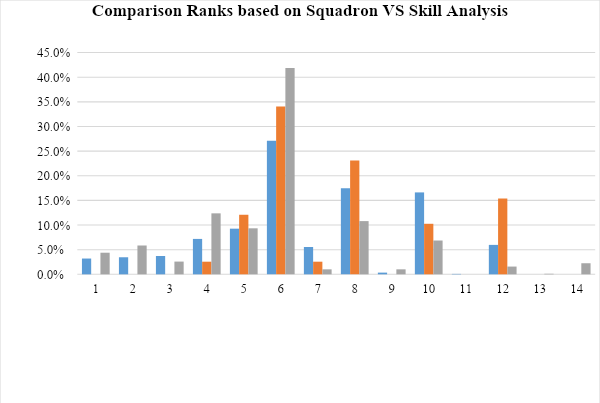
Based on three (3) squadrons, Figure 4 analyses the gaps between ranks and skills. Starting with the Low Skilled category, No. 12 Squadron scored 4.39% and No. 8 Squadron for Aircraftman Rank. No. 8 Squadron scored 3.72% and No. 12 Squadron 2.59% for the rank of Lance Corporal. In contrast, No. 10 Squadron got 12.09% for Air Corporal Rank, followed by No. 12 Squadron with 9.34% and No. 8 Squadron with 9.26%. No. 8 Squadron attained 5.54% for Air Sergeant Rank, No. 10 Squadron 2.56%, and No. 12 Squadron 1.01%. No. 12 Squadron scored 1.01%, and No. 8 Squadron scored 0.35% for Flight Sergeant Rank. The lowest scores for Warrant Officer II were obtained by No. 8 Squadron and No. 12 Squadron, respectively.
In the Skilled category, No. 12 Squadron got 5.85% for Aircraftman Rank, followed by No. 8 Squadron with 3.45%. No. 12 Squadron scored 12.37%, No. 8 Squadron scored 7.19%, and No. 10 Squadron scored 2.55% for Lance Corporal Rank. No. 12 Squadron has the highest percentage of Skilled technicians for Air Corporal Rank at 41.84%, followed by No. 10 Squadron at 34.07% and No. 8 Squadron at 27.74%. No. 10 Squadron scored 23.08% for Air Sergeant Rank, No. 8 Squadron scored 17.49%, and No. 12 Squadron scored 10.20%. No. 8 Squadron got 16.62% for Flight Sergeant Rank, followed by No. 10 Squadron with 10.26% and No. 12 Squadron with 6.86%. In terms of Warrant Officer Rank, No. 10 Squadron scored 15.38%, No. 8 Squadron got 5.97%, and No. 12 Squadron earned 1.5%. Although there were aircraft technicians with Low Skills in Air Corporal Ranks and Air Sergeant Ranks, there were more Skilled aircraft technicians in Air Corporal Ranks, Air Sergeant Ranks, and Flight Sergeant Ranks.
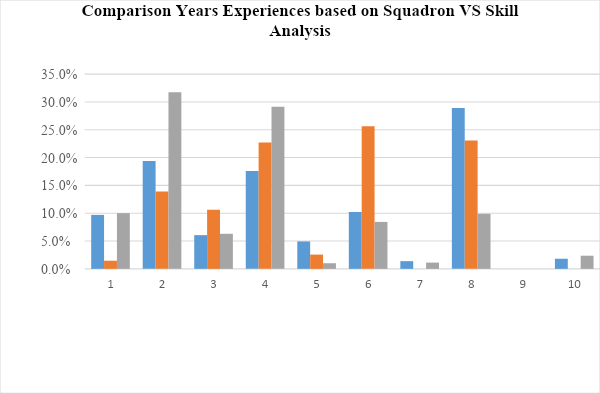
Figure 5 illustrates the differences between years of experience and skill analysis. In Low Skilled categories with 1 to 5 years of experience, No. 12 Squadron earned 10.01%, whereas No. 8 Squadron scored 9.78%, and No. 10 Squadron scored 1.47%. No. 10 Squadron earned 10.62% for years of experience between 6 and 10 years, No. 12 Squadron scored 6.3%, and No. 8 Squadron got 6%. The technician's experience between 11 and 15 years reveals that No. 8 Squadron scored 4.94%, No. 10 Squadron scored 2.56%, and No. 12 Squadron scored 1.01%. The set of experiences spanning 16 to 20 years reveals that No. 8 Squadron scored 1.39% and No. 12 Squadron scored 1.12%.
No. 12 Squadron's experienced technicians with 1 to 5 years of experience scored 31.72% in the Skilled category, followed by No. 8 Squadron with 19.31% and No. 10 Squadron with 13.98%. Next, between 6 and 10 years of experience, No. 12 Squadron earned the best with 29.13%, followed by No. 10 Squadron with 22.71% and No. 8 Squadron with 17.53%.
The group of experiences between 11 and 15 years reveals that No. 10 Squadron scored 25.64%, No. 8 Squadron scored 10.22%, and No. 12 Squadron got 8.44%. The next set of experiences between 16 and 20 years indicates that No. 8 Squadron scored 28.92%, followed by No. 10 Squadron with 23.08% and No. 12 Squadron with 9.9%. In terms of experience above 21 years, No. 12 Squadron scored 2.36%, No. 8 Squadron scored 1.82%, and No. 10 Squadron scored 0%.
All in all, No. 12 Squadron has the highest number of Low Skilled AMTs with less than five years of experience in operational squadrons, while No. 10 Squadron's score of 10.62% indicates that aircraft technicians with less than ten years of experience are still Low Skilled. In addition, the research reveals that skilled AMTs with 1- 5 years, 6- 10 years, and 11-15 years of experience are more prevalent.
Discussion
From the survey, there were more Skilled aviation technicians in the age ranges of 21-30 and 31-40, even though there were some Low-Skilled technicians in the 21-30 age range. However, a sizable fraction of technicians across all squadrons remains Low Skilled in terms of IR 4.0 proficiency. There were some Air Corporals and Air Sergeants with Low Skills, but many more Air Corporals, Air Sergeants, and Flight Sergeants with High Skills. Within active squadrons, No. 12 Squadron has the highest percentage of technicians with fewer than five years of experience who are Low Skilled in aircraft maintenance, while No. 10 Squadron has the highest percentage of technicians who are Low Skilled despite having more than ten years of experience. The data also shows that technicians with 1- 5 years of experience, 6- 10 years of experience, and 11-15 years of experience are the norm in the field of aircraft maintenance.
RMAF AMT has variations in training and experience. Each squadron may have different training programs, resources, and exposure to diverse aircraft models. Comparing the squadrons allows for an examination of how these variations impact the development of digital skills and competencies among technicians (Alomar & Yatskiv, 2023; Moin et al., 2019; Thulasy et al., 2022).
Conclusion
Digital competence represents the latest framework in defining skills associated with technology. Consequently, various terms such as ICT skills, technology skills, information technology skills, 21st-century skills, information literacy, digital literacy, and digital skills have been employed to describe the proficiencies and abilities necessary for effectively utilising digital technologies (Ilomäki et al., 2016). Therefore, in the IR 4.0 era, it is vital to evaluate the skills and competencies of the workforce to operate and manage ICT-related systems and devices. Jashari et al. (2021) described a self-assessment that frequently results in respondents overestimating their own digital skills based on a review of existing techniques and methodologies used to assess the digital skills of diverse groups.
Aligned with the integration of IR4.0, the incorporation of digital skills and capabilities has become imperative for aviation maintenance technicians. To meet the demands of the aerospace industry, it is essential to attract and retain a highly skilled workforce that possesses a natural aptitude for digital technologies. These individuals should also exhibit qualities such as creativity, adaptability to change, and a commitment to lifelong learning. Furthermore, they must be capable of designing and operating intricate, interactive, and collaborative systems. However, the existing basic training curriculum in the RMAF falls short of comprehensively evaluating the digital abilities and competencies of AMT. As a result, the objective of this study was to develop and refine assessment components that incorporate elements of digital skills and competencies required for aircraft maintenance tasks in the context of IR 4.0. By doing so, the study aims to address the existing gap and ensure that technicians are well-equipped to navigate the challenges posed by the digital era.
References
Alkharusi, H. (2022). A descriptive analysis and interpretation of data from Likert scales in educational and psychological research. Indian Journal of Psychology and Education, 12(2), 13-16.
Alomar, I., & Yatskiv, I. (2023). Digitalisation in aircraft maintenance processes. Aviation, 27(2), 86-94. DOI:
Barry, J. A., Mollan, S., Burdon, M. A., Jenkins, M., & Denniston, A. K. (2017). Development and validation of a questionnaire assessing the quality of life impact of Colour Blindness (CBQoL). BMC Ophthalmology, 17(1). DOI:
Elangovan, N., & Sundaravel, E. (2021). Method of preparing a document for survey instrument validation by experts. MethodsX, 8, 101326. DOI:
Gahbauer, S. (2019). Skills for the future: Digital competence will be essential: Canada continues to lag in development efforts. Plant. https://www.plant.ca/features/skills-for-the-future-digital-competence-will-be-essential/
Ghazali, N., Nordin, M. S., & Tunku Ahmad, T. B. (2021). Development and Validation of Student's MOOC-efficacy Scale: Exploratory Factor Analysis. Asian Journal of University Education, 17(4), 327. DOI:
Güneş, T., Turhan, U., & Açıkel, B. (2020). An Assessment of Aircraft Maintenance Technician Competency. International Journal of Aviation Science and Technology, vm01(is01), 22-29. DOI:
Ilomäki, L., Paavola, S., Lakkala, M., & Kantosalo, A. (2016). Digital competence - an emergent boundary concept for policy and educational research. Education and Information Technologies, 21(3), 655-679. DOI:
International Air Transport Association. (2020). Aircraft Technology Roadmap to 2050. https://www.eflight.com/wp-content/uploads/2021/05/Aviation-Technology-Roadmap-IATA-2050.pdf
International Labour Organization. (2023). Guidance on using the five-point rating scale for the overall performance rating. https://www.ilo.org/wcmsp5/groups/public/@dgreports/@exrel/documents/instructionalmaterial/wcms_745729.pdf
International Telecommunication Union. (2021). Digital Skills Toolkit. https://www.itu.int/en/ITU-D/Digital-Inclusion/Documents/ITU%20Digital%20Skills%20Toolkit.pdf
Jashari, X., Fetaji, B., Nussbaumer, A., & Gütl, C. (2021). Assessing Digital Skills and Competencies for Different Groups and Devising a Conceptual Model to Support Teaching and Training. Cross Reality and Data Science in Engineering, 982-995. DOI:
Krejcie, R. V., & Morgan, D. W. (1970). Determining Sample Size for Research Activities. Educational and Psychological Measurement, 30(3), 607-610. DOI:
Lappas, I., & Kourousis, K. I. (2016). Anticipating the Need for New Skills for the Future Aerospace and Aviation Professionals. Journal of Aerospace Technology and Management, 8(2), 232-241. DOI:
Lee, M., Yun, J. J., Pyka, A., Won, D., Kodama, F., Schiuma, G., Park, H., Jeon, J., Park, K., Jung, K., Yan, M.-R., Lee, S., & Zhao, X. (2018). How to Respond to the Fourth Industrial Revolution or the Second Information Technology Revolution? Dynamic New Combinations between Technology, Market, and Society through Open Innovation. Journal of Open Innovation: Technology, Market, and Complexity, 4(3), 21. DOI:
Leon, A. C., Davis, L. L., & Kraemer, H. C. (2011). The role and interpretation of pilot studies in clinical research. Journal of Psychiatric Research, 45(5), 626-629. DOI:
Liao, Y., Loures, E. R., Deschamps, F., Brezinski, G., & Venâncio, A. (2018). The impact of the fourth industrial revolution: a cross-country/region comparison. Production, 28(0). DOI:
Mallik, A., Banerjee, M., & Michailidis, G. (2020). M-estimation in Multistage Sampling Procedures. Sankhya A, 82(2), 261-309. DOI:
Moin, I., Harun, K., Rahman, N., & Mohammad, F. (2019). Personnel Competency Development for a Successful Digital Transformation in Aircraft Maintenance, Repair & Overhaul (MRO) Industry-A Conceptual Framework. Advances in Transportation and Logistics Research, 2, 426-432.
Nimvyap, N., & Benjamin, M. W. (2020). The prospects and challenges of entrepreneurship and venture creation among graduates of tertiary institutions in plateau state Nigeria. International Journal of Management Studies, Business & Entrepreneurship Research, 5(1), 1-14.
Quiroz, V., Reinero, D., Hernández, P., Contreras, J., Vernal, R., & Carvajal, P. (2017). Development of a self-report questionnaire designed for population-based surveillance of gingivitis in adolescents: assessment of content validity and reliability. Journal of Applied Oral Science, 25(4), 404-411. DOI:
Rahim, N. A., Mohamed, Z. B., Masrom, M., & Amrin, A. (2018). Construct Validity in Pilot Study: Application in Academic Entrepreneurship Research. Advanced Science Letters, 24(5), 3224–3228. DOI:
Sainani, K. L. (2014). Introduction to Principal Components Analysis. PM&R, 6(3), 275-278. DOI:
Setia, M. (2016). Methodology series module 5: Sampling strategies. Indian Journal of Dermatology, 61(5), 505. DOI:
Skills Future Singapore. (2017). Skills Framework for Aerospace: A Guide to Occupations and Skills. https://dokumen.tips/documents/skills-framework-for-aerospace-barnes-aerospace-windsor-airmotive-asia-aoethe-aerospace.html
Stratton, S. J. (2021). Population Research: Convenience Sampling Strategies. Prehospital and Disaster Medicine, 36(4), 373-374. DOI:
Taherdoost, H. (2016). Sampling Methods in Research Methodology; How to Choose a Sampling Technique for Research. SSRN Electronic Journal. DOI:
Thulasy, T. N., Nohuddin, P. N. E., Nusyirwan, I. F., Rahim, N. A., Amrin, A., & Chua, S. (2022). Skills Assessment Criteria for Aircraft Maintenance Technician in the Context of Industrial Revolution 4.0. Journal of Aerospace Technology and Management, 14. DOI:
Thulasy, T. N., Nohuddin, P. N., Nusyirwan, I. F., Rahim, N. A., & Amrin, A. (2021). Competencies and Skills Criteria for Aircraft Maintenance Technical Education Assessment for Technicians in Addressing Industrial Revolution 4.0. Human Systems Management, 1-11.
Vuorikari, R., Kluzer, S., & Punie, Y. (2022). DigComp 2.2: The Digital Competence Framework for Citizens - With new examples of knowledge, skills and attitudes, EUR 31006 EN. Publications Office of the European Union, Luxembourg. https://publications.jrc.ec.europa.eu/
World Economic Forum. (2014). Education and Skills 2.0: New targets and innovative approaches. http://www3.weforum.org/docs/GAC/2014/WEF_GAC_EducationSkills_TargetsInnovativeApproaches_Book_2014.pdf
Xu, M., David, J. M., & Kim, S. H. (2018). The Fourth Industrial Revolution: Opportunities and Challenges. International Journal of Financial Research, 9(2), 90. DOI:
Copyright information
About this article
Publication Date
29 November 2023
Article Doi
eBook ISBN
978-1-80296-131-7
Publisher
European Publisher
Volume
132
Print ISBN (optional)
-
Edition Number
1st Edition
Pages
1-816
Subjects
Accounting and finance, business and management, communication, law and governance
Cite this article as:
Thulasy, T. N., Nohuddin, P. N., & Nusyirwan, I. F. (2023). Industrial Revolution 4.0 Digital Competencies Instrument for Measuring Aircraft Maintenance Technicians. In N. M. Suki, A. R. Mazlan, R. Azmi, N. A. Abdul Rahman, Z. Adnan, N. Hanafi, & R. Truell (Eds.), Strengthening Governance, Enhancing Integrity and Navigating Communication for Future Resilient Growth, vol 132. European Proceedings of Social and Behavioural Sciences (pp. 218-232). European Publisher. https://doi.org/10.15405/epsbs.2023.11.02.17