Abstract
The paper contains material on the choice of the form of distribution of income of the population and the assessment of the parameters of the stochastic volatility model, which are based on beta distributions. Since the mathematical description of complex systems is often built on the basis of distribution models that are given on the positive semi axis, then for these purposes, beta distribution of the second kind and its moments is often used. As the output arrays of complex processes are scaled in units of controlled physical quantities, it makes difficult to applied the classical moments method to analysis of the shape parameter of beta distributions. For this reason, the method of standardized moments is used to preliminary determine the type of distributions. However, this method makes not possible to estimate the shapes parameters of beta distributions. The objective of this paper is to study the possibility of using entropy coefficients to construct a display space for estimates of the shape parameters of the beta distribution of the second kind. Also, this paper addresses the problem of constructions of the diagram for the modelling shapes of the beta distribution of the second kind.
Keywords: Entropy coefficient, income distribution, standardized moment, volatility model
Introduction
Income distribution plays a key role both in theory and in solving practical problems of the modern financial economy. Central to many financial theories is the assumption about the shapes of income distribution.
In Chotikapanjch (2008) and McDonald (1984) alternative statistical distributions were explored which adequately reflect their empirical characteristics. The search research shows that the generalized beta distribution of the second kind is applicable to the distribution of wealth and income of the population of different regions. Stochastic models of economic exchange and distribution of wealth and income are based on distributions that are given on a semi-axis. For these purposes, the beta distribution of the second kind is used, which includes many shapes and it borders on the forms of the lognormal and gamma distributions.
In modeling the relaxation and correlation of the distribution of market data, Beta distributions of the second kind are also used (Moghaddam et al., 2021). The main difficulty of using beta distributions is related to the choice of the most suitable form of its implementation, which satisfies empirical data. There is also limited information about the uniqueness of the properties of the beta distribution for modeling the distribution of wealth and income of the population.
Despite the fact that the Gauss distribution is convenient for theoretical modeling of the return on equity, the assumption of normality contradicts empirical data (Harris & Kucukozmen, 2001). As noted there, in the short term, the profitability of stocks demonstrates significant leptokurtosis and asymmetry.
Also, in the article (Moghaddam et al., 2021) classes of quadratic stochastic volatility models were investigated by the beta probability density function of the second kind for the stationary regime. If the stochastic volatility model is defined with the inverse Heston mean multiplicative value, then its steady-state distribution is a Beta distribution of the second kind, which was named as beta prime distributions.
Problem Statement
The use of flexible beta distribution models of the second kind in economic theory raises a number of theoretical and practical issues. Despite the frequent use of beta distributions of the second kind in solving modern problems of econometrics and financial economics, many issues of estimating the parameters of the distribution form remain unresolved. For the preliminary selection of distributions, moment ratio diagrams have been of great use among engineers and statisticians, since they have allowed to construct several distributions on the same axes. The main usefulness of the diagram is as follows. Moment diagrams allow you to provide a ready-made "snapshot" of the relative universality of various distributions in terms of representing a range of shapes. There is the most complete version of the moment diagram, in which the coefficient of variation, skewness and kurtosis are used as axis coordinates (Vargo et al., 2010).
Visualization of models is often used in various scientific studies (Berkman, 2017; Bachioua, 2013; Gubarev, 2011; Voloshchuk et al., 1974). There visualization models are located in space of probability distribution parameters. At the same time, these well-known schemes of the parameter space usually allow us to establish only a class of models and are limited in estimating its parameters. For this reason, it is relevant to study the signs of the distribution shape to assess its parameters, as well as to develop more effective schemes for classifying the distributions.
Research Questions
In the course of the study, the following issues were considered:
- How are the models of the beta distribution of the second kind related to the models and tasks of the modern financial economy?
- What shapes features are used to analyze beta-distributions of second kind?
- How are localized the realizations of the beta distribution of the second kind in the space of information and parametric features?
- What properties are characteristic of implementations of the beta distribution of the second kind with different shape parameters?
Purpose of the Study
Presumably, the use of entropy coefficients for plotting diagrams in the parameter space of the beta distribution form of the second kind will reveal the characteristic properties of various realizations of the distribution.
Research Methods
The study is based on the use of the diversity of the form of the beta distribution of the second kind and its probabilistic-informational characteristics to solve tasks of the modern financial economy.
Model beta Distribution of the Second Kind and its Moments
In probability theory, the beta distribution of the second kind is an absolutely continuous probability distribution defined on the positive axis of real values (x> 0). A random variable X follows a beta distribution of the second kind with shape parameters of u and v that are more zero if its cumulative distribution function is defined as (Vadzinsky, 2001)
(1)
Where/(1+) (,) are the ratios of the incomplete beta functions, that is given by
(2)
Here(,) is the beta function given as an Euler integral of the first kind, and is first and second shape parameters.
The probability density of the standardized beta distribution of the second kind is associated with the cumulative probability function of (1) that is given by
(3)
The paper also notes the limited choice of the model, which, apparently, is due to the lack of formalized methods for analysing the shape of the model (Hertzler, 2003). For these purposes, methods are classically used that is based on distribution moments. The initial moment of order for the beta distribution of the second kind is given as the ratio of the beta functions (Bourguignon & de Castro, 2021)
(4)
If the order of the moment is less than the second shape parameter, then to calculate the moment of the beta distribution of the second kind, you can use an expression of the shape
(5)
Thus, it can be seen that the moments of the generalized scaled beta distribution of the second kind are proportional to the moments of the standard beta distribution that is determined only by the scaling parameter. In this case, the moments of the standardized beta distribution of the second kind are completely specified by the shape parameters. Since the arrays of real processes are always scaled, this creates difficulties in choosing the shape of the model and estimating its parameters directly from the observed results. For these goals, standardized moments are classically used, such as skewness and kurtosis.
In practice, when using different conventional units, the random variable is scaled with a scale parameter. Then the random variable is given as a product
( 6 )
А scaled beta distribution of second kind is given as
( 7 )
The beta distribution density function (7) is convenient for modeling arbitrary scaled responses of complex systems given on the positive half-axis of values. When modeling the responses of real systems, the data is almost always scaled according to the chosen system of units. In most cases, using directly models based on a standardized beta distribution is not convenient due to the scaling the data. This limits the application of forms of beta distribution models in practice.
It is discussed in (Moghaddam et al., 2021) that if a stochastic volatility model is defined with the mean reverting multiplicative Heston (Johnson et al., 1994), then its stochastic differential equation is given by
( 8 )
Where is the stochastic variance, is the relaxation time parameter, and are the weight coefficients of the multiplicative model and the Hestona model, is a normally distributed Wiener process, is the average value of the stochastic variance: =<>.
The parameters of the stochastic equation (8) completely determine the parameters of the beta distribution of the second kind (2). In case, if the relaxation time parameter is known and the parameters of the scaled beta distribution of the second kind (7) were estimated, then the weight parameters of the stochastic equation (8) are given as
( 9 )
The mean value of the stochastic variance is related to the scale parameter of the beta distribution of the second kind, which is given as
( 10 )
Relations (9) and (10) allow us to calculate the parameters of the stochastic volatility equation model with known beta distribution parameters.
Standardized Moments
In probability theory, various methods of moments are traditionally used to study the distribution shape, that it is presented in Craig (1936), Han and Shapiro (1969), Kobzar (2006), Vadzinsky (2001). The method of moments was introduced by Pafnuty Chebyshev in 1887 when proving the central limit theorem (Chebyshev, 1955; Rybnikov, 1974). For data analysis and preliminary selection of a family of distributions in regression analysis, standardized normalized second, third, and fourth moments of distributions are used, which are known as the coefficient of variation, skewness and kurtosis of the distribution. These are the most important indices of the distribution shape. Expressions for calculating the standardized moment of the beta distribution of the second kind in many reference books on distributions are contained, for example (Gubarev, 1992; Vadzinsky, 2001).
For calculating of the third standardized moment, the expression of skewness is given as
( 11 )
For calculating the fourth standardized moment, the expression of kurtosis is defined as
( 12 )
An important property of the standardized moments of equation (11) and equation (12) is that they are insensitive to changes in the distribution scale parameter. For this reason, the standardized moments of equation (11) and equation (12) are used for a preliminary assessment of the belonging of the sample data to the distribution model. For these purposes, the visualization of the parameter space is used, that was proposed by A. Rhind in 1909 for the Pearson distribution system. Rhind and Pearson used the third and fourth standardized moments as space coordinates (Pearson, 1895; Rind, 1909). Moment-ratio diagrams were later developed and popularized by Craig (1936), Han and Shapiro (1969), Johnson et al. (1994).
Anti-Kurtosis
The use of distribution kurtosis is not convenient for illustrating the position of models of beta distribution of the second kind, since theoretically the kurtosis tends to infinity, provided that the first shape parameter tends to zero. This is due to the presence of tails of the distribution that is slowly converging to zero. In the region of constraints of the second shape parameter, the kurtosis is positive and greater than the kurtosis of the normal distribution. Therefore, to visualize the parameter space, it is convenient to use the anti-kurtosis that is given as
( 13 )
The use of anti-kurtosis makes possible to place all models of beta distributions of the second kind on a limited range of values, that is limited by the range from 0 to 0.6. Since the beta distribution models include symmetrical shapes, the third standard moment is used to set the model skewness parameter.
Entropy Coefficient of Non-Shifted beta-Distributions of Second Kind
In the entropy coefficient of the non-shifted distribution is proposed as an information feature for estimating the shape of distributions, that is given as (Polosin, 2020a; Polosin, 2020b).
( 14 )
Where is entropy interval of uncertainty of non-shifted distribution,2 is the initial moment of the second order.
The entropy coefficient for the non-shifted beta distribution of the second kind is given as
( 15 )
Since the second initial moment of the beta distribution of the second kind imposes a restriction on the range of change of the second shape parameter from two to infinity, then this restriction is also imposed on the entropy coefficient of the non-shifted beta distribution of the second kind. It should be noted that this limitation is lower than for space of skewness and anti-kurtosis. Therefore, when constructing entropy-parametric spaces, the order of the standardized moment imposes the restriction on the range of the second shape parameter.
Findings
As shown above, for the analysis of beta distributions of the second kind, such statistical features of distribution forms as standardized distribution moments are often used. The author of the article suggests using to visualize the properties of beta distribution models of the second kind a joint space of information and parametric features. The article provides examples of a space using standardized moments and entropy coefficient. Since two-dimensional projections of space are convenient for analysis, two typical examples of these projections are considered below. The first two-dimensional space is based on the features of anti-kurtosis and entropy coefficient. In the second space, the features of skewness and entropy coefficient are used.
Space of Anti-Kurtosis and Entropy Coefficient
Figure 1 illustrates the features of the possible locations for models of beta distribution of the second kind in the space of the entropy coefficient and anti-kurtosis, where the following notation is used. The numbers of 1, 2, 3, 4, 5, 6, 7, 8, 9, 10 and 11 are the trajectories of possible models of the beta distribution of the second kind for various ratios of the second shape parameter to the first parameter, that it is respectively equal to 0.001, 0.1, 0.5, 1, 2, 3, 5, 10, 20, 100 and 400. Attention should be paid to trajectories of 1 and 11, which are shown by a dotted line. These trajectories are the boundaries of the surface of possible positions of the beta distribution models. The numbers of 12, 13, 14, 15, and 16 are curves of the equivalent values of the second shape parameter that is respectively equal to 4.1, 5, 7, 10, and 120. The numbers of 17, 18, 19, 20 and 21 are curves of the equivalent values of the first shape parameter that is respectively equal to 0.2, 0.5, 1, 2 and 7.
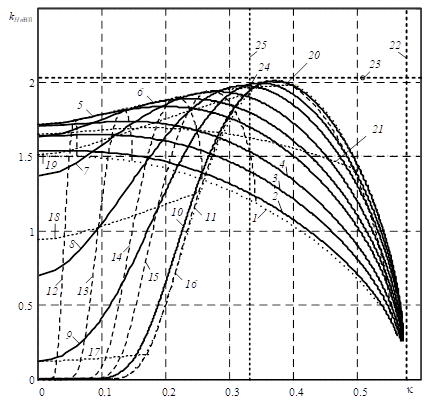
Figure 1 also shows the positions of the mesokurtic distributions with dashed line 22, where the anti-kurtosis is 0.577. The dashed line 25 defines the cutting plane for model that anti-kurtosis is 0.333. This corresponds to the anti-kurtosis of exponential distributions. The position of the asymmetric distribution of the modulus of the normal value is shown by the point with the number 23.
For this point, the anti-kurtosis and the entropy coefficient are 0.508 and 2.066, respectively. The position of the exponential distribution is illustrated by point 24 with the anti-kurtosis and the entropy coefficient that are 0.333 and 1.922, respectively.
The family of beta distributions of the second kind contains a distribution for which the entropy coefficient and the anti-kurtosis are equal to the corresponding characteristics of the exponential distribution, the position of which is given by point 24. If the first shape parameter is greater than 1 and the range of the second parameter is from 4 to 7, then the anti-kurtosis of the beta distribution of the second kind is less than the anti-kurtosis of the exponential distribution.
The beta distribution of the second kind contains a set of shapes for which the anti-kurtosis is greater than the anti-kurtosis of the exponential distribution, provided that the first shape parameter is greater than 1.5 and the second shape parameter is greater than 7.
Due to the restrictions imposed on the second shape parameter by the standardized moment of the fourth order, there are no models of the beta distribution of the second kind in the space, provided that the second shape parameter is less than 4. This significantly reduces the significance of the space of anti-kurtosis and skewness when this is used to analyse or preliminary estimate the parameters of the beta distribution second kind.
At the same time, the analysis in the space of the entropy coefficient and anti-kurtosis is more informative than in the space of anti-kurtosis and asymmetry. In particular, it follows from consideration of the space of the entropy coefficient and the counter-kurtosis that the family of beta distributions of the second kind does not contain distributions with shapes close to mesokurtic distributions. Wherein, a high value of the entropy coefficient allows you to set additional restrictions on the trajectories of distributions.
Space Based on Skewness and Entropy Coefficient
Figure 2 illustrates the possible positions for models of the beta distribution of the second kind in the space of the asymmetry and the entropy coefficient that it is given by trajectories and curves of the equivalent values of the shape parameter. Figure 2 retains the designations previously used to describe Figure 1 for trajectories and for curves of equivalent values of both the first and second parameters. The dotted plot 22 illustrates the position of the models, the skewness of which is equal to the distribution skewness of the normal value modulus.
Points 23 and 24 correspond to the position of the distributions of the modulus of the normal value and the exponential distribution with asymmetries equal to 0.9953 and 2, respectively. Point 23 in Figures 1 and 3 is outside the surface of possible patterns of beta distribution of the second kind.
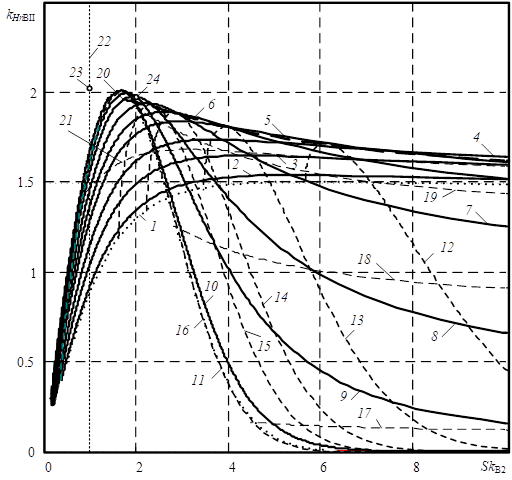
Obviously, among the models of the beta distribution of the second kind, there are no models whose shape corresponds to the shape of the distribution of the normal value modulus that is given as point 23 in Figure 1 and 2 near the surface of possible models. It can be assumed that the distribution of the second kind contains the shape set close to exponential distribution, provided that the first shape parameter is equal to 1.5, the second shape parameter is more than 10.
Conclusions
It follows from the considered material that the moments of higher orders impose the constraints on the mapping space if the axes of this space are moment’s ratios and the entropy coefficient. Constraint is imposed on minimum value of the shape second parameter that is equal to the j-th order of the standardized moment. Unfortunately, this space does not display a huge number of models most commonly used in practice.
For example, so anti-kurtosis and kurtosis for these models are absent then due to the heavy weight of the distributions tails of probability these models are not possible be investigated on value interval of the shape second parameter from zero to four in the space of anti-kurtosis and entropy coefficients. However, despite the fact that a huge number model in this range of the second shape parameter is located, the space of anti-kurtosis and entropy coefficients illustrate the set important properties of the beta distributions of the second kind. Firstly, Figure 1 show that all models of beta distributions of the second kind are leptokurtosis, since they are located on space of the anty-kurtosis and the entropy coefficient to the left of the mesokurtic line. Moreover, if the second shape parameter is greater more than four and less than seven then the anti-kurtosis of such models is less than the antikurtosis of the exponential distribution. Also, there are a lot of information about the relation of entropy uncertainty intervals to the intervals of the second initial moment of many models, which expands the possibilities of preliminary establishing the shape of the model if its second shape parameter is more than four.
The space of skewness and entropy coefficient of the non-shifted beta distribution of the second kind contains models that the second shape parameter is greater than three. This softer restriction on the surface of possible implementations imposes a third standardized moment.
Nevertheless, Figure 2 also shows a series of important general properties for the beta distribution of the second kind. As all these models are more asymmetric in comparison with the exponential distribution. As well there are no symmetrical models among beta distributions of the second kind. For this reason, the use of the family in modelling distributions is only possible to recommend if the model asymmetry is greater than one. Moreover, if the second shape parameter is in the range from three to four, then such models the skewness parameter is given in the range from 6 to 60. If the model skewness is more than 60, then the entropy coefficient is less than 0.7.
Thus, this follows from the study that the displaying of the beta distribution of the second kind in the space of the ratio of moments and entropy coefficient it made possible to get additional information as about properties these models as and about shape parameters.
References
Bachioua, L. (2013). On Pearson families of distributions and its applications. African Journal of Mathematics and Computer Science Research, 6(5), 108-117.
Berkman, I. N. (2017). Vysshaya matematika: matematicheskij apparat diffuzii [Higher Mathematics: mathematical apparatus of diphusion]. Publishing House Urright. https://urait.ru/bcode/437291
Bourguignon, M. Santos-Neto M., & de Castro, M. (2021). A new regression model for positive random variables with skewed and long tail. Metron, 79, 33-55.
Chebyshev, P. L. (1955). Izbrannye proizvedeniya. Seriya klassika nauki [Selected works. Series classics of science]. Publishing House of the USSR Academy of Sciences. https://booksee.org/book/319298
Chotikapanjch, D. (2008). Modeling Income Distributions and Lorenz Curves. Springe. https://link.springer.com/content/pdf/
Craig, C. C. (1936). A new exposition and chart for the Pearson system of frequency curves. Annals of Mathematical Statistics, 7(1), 16-28.
Gubarev, V. V. (1992). Veroyatnostnye modeli [Probabilistic Models]. Novosibirsk: Handbook. Publishing House of Novosibirsk Polytechnic Institute. https://search.rsl.ru/ru/record/01001666017
Gubarev, V. V. (2011). Identifikaciya empiricheskih raspredelenij [Identification of empirical distributions]. Bulletin of Samara State Aerospace University, 6(30), 205-215. https://journals.ssau.ru/vestnik/article/view/7485/7336
Han, G., & Shapiro, C. (1969). Statisticheskie modeli v inzhenernyh zadachah [Statistical models in engineering problems. Publishing house “Mir”. https://search.rsl.ru/ru/record/01007051787
Harris, D. F., & Kucukozmen, C. C. (2001). The empirical distribution of uk and us stock returns. Journal of Business Finance & Accounting, 28(5-6), 715-740.
Hertzler, G. (2003). Classical probability distributions for stochastic dynamic models. 47th Annual Conference of the Australian Agricultural and Resource Economics Society. https://www.researchgate.net/publication/314637987_Classical_Probability_Distributions_for_Stochastic_Dynamic_Models
Johnson, N. L., Kotz, S., & Balakrishnan, N. (1994). Continuous univariate distributions, volume 1. John Wiley & Sons. http://bayanbox.ir/view/5343019340232060584/Norman-L.-Johnson-Samuel-Kotz-N.-Balakrishnan-BookFi.org.pdf
Kobzar, A. I. (2006). Prikladnaya matematicheskaya statistika. Dlya inzhenerov i nauchnyh rabotnikov [Applied mathematical statistics. For engineers and scientists]. Publishing House of Physical, mathematical and technical literature. https://lib-bkm.ru/13677
McDonald, J. B. (1984). Some Generalized Functions of the Size Distribution of Income. Econometrica, 52(3), 647-663
Moghaddam, M. D., Liu, Z., & Serota, R. A. (2021). Distributions of historic market data: relaxation and correlations. The European Physical Journal B, 94(83).
Pearson, K. (1895). Contributions to the mathematical theory of evolution, II: Skew variation in homogeneous material. Philosophical Transactions of the Royal Societ, 186, 343-414.
Polosin, V. G. (2020a). Mapping distributions in the entropy-parametric space. Journal of Physics: Conference Series, 1515 032044.
Polosin, V. G. (2020b). Space of entropy-parametric characteristics for control of the non-symmetri c distributions. University proceedings. Volga region, 3(55), 44-57.
Rind, A. (1909). Tables that facilitate the calculation of probable errors of the main constants of asymmetric frequency distributions. The biometrics, 7(1/2), 127-147.
Rybnikov, K. A. (1974). Istoriya matematiki [History of mathematics]. Publishing House of Moscow State University. http://booktech.ru/books/istoriya/16243-istoriya-matematiki-1974-k-rybnikov.html
Vadzinsky, R. N. (2001). Handbook of Probability Distributions. Nauka Publishing House of the Russian Academy of Sciences. https://booksee.org/book/747803
Vargo, E., Pasupathy, R., & Leemis, L. M. (2010). Moment-ratio diagrams for univariate distributions. Journal of Quality Technology, 42(3), 1-11.
Voloshchuk, A. M., Zolotarev, P. P., & Ulin, V. (1974). Use of the method of moments for the determination of the coefficients of internal diffusion in the case of adsorbents with a bidispersed porous structure and linear adsorption isotherms. Bulletin of the Academy of Sciences of the USSR, Division of chemical science, 23, 1178–118.
Copyright information
This work is licensed under a Creative Commons Attribution-NonCommercial-NoDerivatives 4.0 International License.
About this article
Publication Date
29 August 2022
Article Doi
eBook ISBN
978-1-80296-126-3
Publisher
European Publisher
Volume
127
Print ISBN (optional)
-
Edition Number
1st Edition
Pages
1-496
Subjects
Economics, social trends, sustainability, modern society, behavioural sciences, education
Cite this article as:
Polosin, V. G. (2022). Moment Ratio And Entropy Coefficient Display For Beta Distribution Of Second Kind. In I. Kovalev, & A. Voroshilova (Eds.), Economic and Social Trends for Sustainability of Modern Society (ICEST-III 2022), vol 127. European Proceedings of Social and Behavioural Sciences (pp. 217-227). European Publisher. https://doi.org/10.15405/epsbs.2022.08.24