Abstract
Free speech is not a licence for racists to spread racist propaganda. However, the outbreak of COVID-19 and its subsequent dissemination through social media has left a shock wave of disbelief resulting xenophobia in society. By looking from the lens of ‘Othering,’ this study tries to delve into revealing the representation of xenophobia resulting otherness amidst Twitter during the COVID-19 pandemic. This study considers a set of tweets related to #clustertabligh and several types related to the tabligh cluster to represent the otherness. The data were collected through a random sampling technique using machine learning tools Twitter API and processed using JSON format. The results lead to a discussion of power between social networks by intertwining the machine learning tools in social science and humanities studies. This study also looks at blame allotting, a typical social reaction to an outbreak, and an even more typical general media system. Malaysia needs to take actions by recognizing the distinctive levels of hateful racial online content brewing and addressing it systematically and proportionately.
Keywords: Coronavirus, otherness, power relations, racism, xenophobia
Introduction
The outbreak of COVID-19 pandemic continues to wreak havoc globally by relentlessly spreading and infecting and killing tens of thousands, this outbreak simultaneously creates fear among people. The COVID-10 pandemic has sparked a rise in worldwide accounts of racial incidents. The condition feeds terror in which xenophobia and bigotry transform into manifestation. This pandemic has revealed social and political instabilities in societies with racist and discriminative responses to fears which excessively affecting marginalised groups (Haynes, 2020; Nature, 2020). One of the increased anxiety effects is that it can exacerbate prejudice and bigotry by concentrating on one’s attention to health as a human being.
Asian descent has experienced more violent reactions in a growing xenophobia trend and turns out to be phenomenal in online forums and social media. The rapid Internet advancement has resulted in an exponential increase in the number of people using online platforms. It is estimated that around 350,000 Twitter posts (tweets) are generated on Twitter (Twitter Usage Statistics, 2018). Reflected from social media users’ news feed, millions of people use Facebook, Twitter, and Instagram platforms to provide public opinions, discussions, and disseminating race-related issues. On the other hand, speeches contained racist sentiments typically refer to the socially formed opinion of discrepancies between appearance, heritage, culture, or faith-based social classes (Paradies, 2006) and includes race, ethnicity, and faith.
In Malaysia, it has been discovered that most racially charged social media comments were sentiments of anti-Islam/ Malay, followed by annotations addressed to Chinese and Indians during pandemic (Chin, 2020). Considering that the content posted in social media also represents their users’ real-world interactions, there is an increased expectation that social media environments should often follow society’s norms.
Despite wide-ranging social psychological research into hate, prejudice, and stereotyping (Wigerfelt et al., 2015), there are still unexplored areas about power relations and otherness that contribute to hateful offending behaviour (Eliassi, 2015). In particular, little is known about the degree to which hateful online content is a possible risk factor that constructs negative attitudes and subsequent hateful acts (Synnott et al., 2017). Hence, this study addressed the xenophobia and racialisation issues by looking into Twitter users’ consciousness during the Covid-19 outbreak, and at the same time, deconstructing the power relations embedded in the hashtag used.
Problem Statement
The topic of racism is not new (Hall, 1980), therefore with the euphoria of the Internet and the public sphere of the mass media, the discursive mode of racism has greatly changed. According to Devakumar et al. (2020), infectious diseases have been linked with othering throughout history. While specific expressions for othering like racism and ethnocentrism are well-documented in the literature, this wider phenomenon under study cannot be identified as such.
There is a rising body of work that examines race and ethnicity problems in digital environments. It has emerged that social networking relationships, online contact forms, and digital identities are far from race neutral (Kolko et al., 2013). Research has posed concerns about how current racial segregations and disparities have expanded into the public world, stressing the emergence of different forms of digital divisions. While scholars identify this trend, there is a lacuna in the existing literature in how and why it might be so predominant on social media (Bartlett et al., 2014). There is not much work investigating the latest digital story of racial and racist discourse in response to Twitter during this pandemic season.
Ironically, COVID-10 has become a tool to reveal xenophobia. Xenophobia has been widely described as a universal phenomenon as an extreme detest, hate, or fear of outsiders. On the contrary, racial discrimination reflects racial thoughts, a manner, and a belief that entails racial supremacy or inadequacy. Hall (1990) hypothesises that the basic characteristics of racism are important are amended and changed by the circumstances appear in society. This transformation can be lured via social media platforms.
In Malaysia, racism and xenophobia arise when a video of a Chinese lady made an accusation and blamed Malacca General Hospital of not attending to patients went viral on Twitter on March 25, 2020. This was followed by a thread of online harassments against the woman via Twitter (Tham, 2020). Few incidents that also lead to xenophobia occur when social media users, especially on Twitter, insulted a group of Tabligh or known as #clustertabligh, as a dramatic rise of cases in Malaysia (Chin, 2020).
Apparently, both xenophobia and racism create the notion of ‘otherness’ that casts other groups of people from the community. This notion is in line with Edward Said’s Orientalism- the dominant Occident and the inferior of the Orient (Said, 1978). Thus, it is crucial to understand the dichotomy between West and Orient, which subsequently implied in this context of racism and xenophobia. As a result, society’s interpretation of reality and its organisation of discursive practices such as chatting and thinking are determined by knowledge production discourses (Zamri, 2015). The notion of the future freeing you from your old self has crashed, and no alternate identification exists. The social media micro-blogging site Twitter has become one of the public spheres to disseminate hatred.
The uncertainty of online racial structures raises the question of whether proper measures are being paid to the significance of the race-existing online environments in how are race and digital networks changed through their mutual encounter, particularly during the pandemic? This study provides an analysis centred on the exploration of race technological production, which focuses on hateful racial online content expressed on Twitter in shaping people’s views towards others during the outbreak of COVID19. Although some work has explored the prevalence of bigotry in online news forms (Abd Karim Zamri, 2015; Meyers, 2007), this study is intended to expose the portrayal of xenophobia arising from Twitter’s otherness during the COVID-10 pandemic.
Research Questions
This study questions how xenophobia expressed on Twitter shapes people’s views towards others during the outbreak of COVID-19?
Purpose of the Study
This study’s central aim is to reveal xenophobia’s representation resulting in otherness amidst Twitter during the COVID-19 pandemic.
Research Methods
This study was pragmatic and selected methods that could stem answers to the questions of the research. An interpretative approach was used, which did not aim to test a hypothesis; rather, it helps to understand the issue and generate a certain theory. The interpretive approach notes that reality is socially constructed (Mertens, 2005), and the result is dependent on participants’ views of the situation that is studied (Creswell, 2003), which refers to tweets by Twitter users. The study addressed the issue by delving into consciousness among Twitter users during the outbreak of COVID-19. At the same time, deconstructing the power relations embedded in the hashtag used. The language Python was used in conjunction with the following external libraries to construct the programs used in this study: Tweepy, JSON Lint, and Matplotlib.
Data Gathering and Processing
Twitter has been considered a growingly potent tool for social science study (Maddock et al., 2015). A range of search terms was chosen to acquire tweets with the hashtag “Tabligh Cluster” and “Kluster Tabligh” during COVID-19. The hashtag was chosen because it is the most debated in social media during the pandemic and causes a major stir due to the sudden jump in Malaysia’s number of cases (Chin, 2020; Joharry & Turiman, 2020). Public tweets with hashtag published between April 10- May 12, 2020, were gathered under a brief period of few minutes. Tweets were gathered using Twitter Application Programming Interface (API) (a complete tweet set using an authorised reseller of Twitter data) to compile a random selection of open tweets continuously. The Streaming API for Twitter provides users access to a random one percent of tweet samples. To this purpose, the third-party python module Tweepy was used. This module is used to directly stream live tweets from Twitter in real-time. Whenever a status update contained a keyword in the filter, its contents were saved in their JSON format for further use. The machine learning tool, such as API, was used in this study due to returning more complete results than the REST API. In fact, web-based data collection has gained immense popularity among social science researchers in social media analysis (Golder & Macy, 2014).
The information extracted from the selected tweets dataset was in raw forms. To use them, we had to clean and transform it into a more usable structured dataset to ensure that the next phase of the process is smooth and efficient (Figure 01). As the data are randomly sampled, the study expects that there is no bias introduced in the results of the analysis. Each tweet is rendered in a JSON format containing lots of information, such as generation timestamp, identifier, tweet content, IP address, and more.
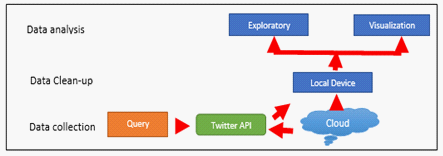
Tweet cleaning is one of the steps in the data transformation. All duplicate tweets were dropped according to their ‘tweet id’ (it has its unique identifier for each tweet). In our datasets, we manually analysed outliers in our datasets (the top 99th percentile of tweeters) and omitted automated accounts and accounts for which most of the tweets were advertisements. In total, we collected 11,0000 general topic tweets. The data were plotted using Matplotlib 3.0.3 on Python 3.7.2.
Findings
This study conglomerates a dataset of 11,000 tweets over the past April 10 until May 12, 2020. These tweets show the early discussion and consciousness around the #cluster tabligh over the pandemic. All the collected data were searched using the keyword “cluster tabligh”, and all tweets containing this keyword posts with its hashtag (#clustertabligh) version have been retrieved. Initially, the data extraction was planned to begin on March 12 until May 12; however, due to the limited data extraction from free API, the data were only available from April 10 until May 12, 2020. The analysis was divided into two phases.
Tweet Trends and Otherness
The analysis focuses on tweet creation using #cluster tabligh from April 10 until May 12, 2020. The graphic in Figure 02 depicts the result of this analysis. The resulting bar chart describes a particular trend. Indeed, it presents some peaks in correspondence between April 13 until April 19, 2020. According to Table 1, April 18 shows the highest tweets amongst all. Hence, we can argue that this day is the major Twitter user’s activity. The increased frequency of tweets publishing can be attributed to numerous factors, for instance, a number of recovered patients being discharged on April 18, 2020.
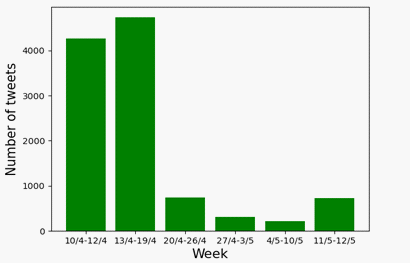
In Figure 03, the comparative incidence of two-sided othering expressions in both hateful and non-hateful samples among different types of datasets on Twitter is exhibited. It can be seen that the #cluster tabligh dataset holds the most recurrent content of the two-sided othering language. It is also noted that the percentage of two-sided othering was higher in the hateful elucidated samples than non-hateful elucidated samples for all different kinds of cyberhate.
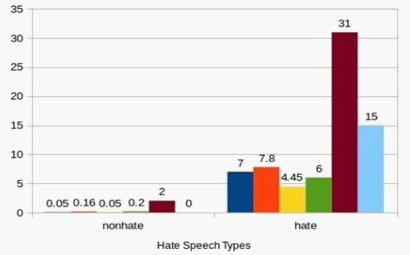
In this study, Foucault’s (1980) theory of power and knowledge shapes the data analysis and interpretation of the empirical corpus of tweets. The findings revealed that #cluster tabligh is framed as an evil actor in the most tweet during the pandemic. This is defined by the use of words in the tweets associating the character with the otherness stereotypes. Entman (2003) argues that it is important to integrate current cultural frames into texts and merge media frames with the audience schemas (Entman, 1993) or equate them with public consciousness (Entman, 2003). The signs used for Othering the #cluster tabligh like ‘penyebab kami kena Covid’ (the reason why we are infected with Covid) and ‘Masa kluster tabligh menjadi titik risau negara’ (the time where tabligh becomes country’s point of concern) are traditional representations of the negative in cultural imagination (Alexander & Jacobs, 1998). These are historically perceptible, understandable, and emotionally charged constructs used in media coverage to create the impression of hate speech and prejudice (Entman, 1993). Constructing the #cluster tabligh as an imaginary Other with bad qualities is a manipulated activity to indicate to bigotry a cluster of meanings. There is no intrinsic significance in the material object or significance constructs in the object’s conceptualisation (Hall, 1997). Hall (1997) argues that a term expressed in linguistic forms constructs a material entity’s meaning.
Word Cloud
For the word cloud analysis, this study employed Wordle’s online text analysis tool to display commonly occurring words. Based on the Figure 04, it exemplifies Wordle’s usage for preliminary analysis. Larger words indicate the word appears more often throughout the text. All words are normalised, and stop words are removed from the corpus before visualisation. The frequency and usage of purple colour denoting the most used word in the postings can be seen from the word cloud. Prominent words like ‘diisytiharkan tamat’ (declared over), ‘Mei satu’ (First May), and ‘terkini’ (latest) were used to reflect the sentiments in the tweets.
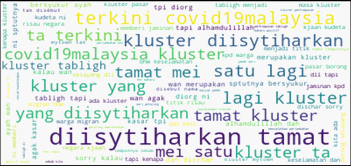
Conclusion
Considering that Twitter encourages users to express their views through a brief 140 character span, this combined with Twitter’s increased sense of privacy has been speculated to contribute to a rise in judgmental and prejudiced comments because of the less thought-out existence of ‘tweet’ (Kahn et al., 2013). Hence, it allows the certain imagined collective to emerges as a with the public. Throughout the pandemic, in #clustertabligh creation, the dichotomised cultural association structure created by the Other and the core identity is seen. Often driving the formulation of the opposition between them is the justification of cultural distinction that purifies the common sense of core identity. The capacity of the invader’s message to represent culturally resonant sense, discrimination, and resistance is due to their being the signs of fear and insecurity. This research also shows that the outbreak paradigm laden with its contagion concerns came to cover the narratives to promote security and otherness policies. Khosravinik (2017) concerns that the advancement of media communication technologies has transformed new interpretations of relations between text makers and users, as well as new and prospective fields for open debates. This shows the application of social relations in the social media construct the power dynamics- the process of power constructions and relations as Foucault (1980) explained, by which the magnitudes of power that is unfixed and continuously shifting based on the today’s world. At the same time, the functionalities of Twitter, which enable certain forms of communication within and between the networked publics, add to a broader communications framework, and this was resulting in a new form of power resistance and projecting the subjugated knowledge.
Acknowledgments
The authors extend special thanks to Universiti Teknologi MARA Malacca Branch, who granted us the TEJA Internal Grant Scheme (GDT2020-4).
References
Abd Karim Zamri, N. (2015). Review of the Representation of History in the New Media. Social Science Postgraduate Seminar (SSPIS) 2015, 125–134. http://media.wix.com/ugd/6efb65_22d4d641a12c45d8b31ae317d5232e1e.pdf
Alexander, J. C., & Jacobs, R. N. (1998). Mass communication, ritual and civil society. Media, ritual and identity, 23-41.
Bartlett, J., Reffin, J., Rumball, N., & Williamson, S. (2014). Anti-social media. Demos, (2014), 1-51.
Chen, S. H., & Yu, T. (2018). Big Data in Computational Social Sciences and Humanities: An Introduction. In Big Data in Computational Social Science and Humanities (pp. 1-25). Springer, Cham.
Chin, E. (2020, April 04). Think tank: Xenophobia, racism rampant on social media amid global Covid-19 lockdowns, Malaysia included: Malay Mail. November 08, 2020, https://www.malaymail.com/news/malaysia/2020/04/04/think-tank-xenophobia-racism-rampant-on-social-media-amid-global-covid-19-l/1853502
Creswell, J. W. (2003). Research design: Qualitative, quantitative, and mixed methods approaches (2nd ed.) Thousand Oaks: Sage.
Devakumar, D., Shannon, G., Bhopal, S. S., & Abubakar, I. (2020). Racism and discrimination in COVID-19 responses. The Lancet, 395(10231), 1194.
Eliassi, B. (2015). Constructing cultural otherness within the Swedish welfare state: The cases of social workers in Sweden. Qualitative Social Work, 14(4), 554–571. DOI:
Entman, R. M. (1993). Framing: Toward clarification of a fractured paradigm. Journal of Communication 43(4), 51–58.
Entman, R. M. (2003). Cascading activation: Contesting the White House’s frame after 9/11. Political Communication 20(4): 415–432.
Foucault, M. (1980). Power/knowledge: Selected interviews and other writings, 1972-1977. Vintage.
Golder, S. A., & Macy, M. W. (2014). Digital Footprints: Opportunities and Challenges for Online Social Research. Annual Review of Sociology, 40(1), 129–152. DOI:
Hall, S. (1990). Cultural identity and diaspora.
Hall, S. (1980). Cultural studies: Two paradigms. Media, culture & society, 2(1), 57-72.
Hall, S. (1997). The work of representation. Representation: Cultural representations and signifying practices, 2, 13-74.
Haynes, B. Y. S. (2020, March 06). As Coronavirus Spreads, So Does Xenophobia and Anti-Asian Racism. Time.Com, 5–8. https://time.com/5797836/coronavirus-racism-stereotypes-attacks/
Joharry, S. A., & Turiman, S. (2020). Collocation networks and COVID-19 in letters to the editor: A Malaysian case study. Asia Pacific Journal of Corpus Research, 1(1), 1-30.
Kahn, K. B., Spencer, K., & Glaser, J. (2013). Online prejudice and discrimination: From dating to hating. The social net: Understanding our online behavior, 2, 201-219.
Khosravinik, M. (2017). Critical Discourse Studies and Participatory Web View project. In Handbook of Critical Discourse Analysis (pp. 582–596). Routledge. https://www.researchgate.net/publication/283213789
Kolko, B., Nakamura, L., & Rodman, G. (Eds.). (2013). Race in cyberspace. Routledge.
Maddock, J., Starbird, K., & Mason, R. M. (2015). Using historical twitter data for research: Ethical challenges of tweet deletions. In CSCW 2015 Workshop on Ethics for Studying Sociotechnical Systems in a Big Data World. ACM.
Mertens, D. M. (2005). Research methods in education and psychology: Integrating diversity with quantitative and qualitative approaches (2nd Ed.). Thousand Oaks: Sage
Meyers, O. (2007). Memory in journalism and the memory of journalism: Israeli journalists and the constructed legacy of Haolam Hazeh. Journal of Communication, 57(4), 719–738. DOI:
Nature. (2020). Stop the coronavirus stigma now. In Nature. DOI:
Paradies, Y. (2006). A systematic review of empirical research on self-reported racism and health. In International Journal of Epidemiology 35(4), pp. 888–901. DOI:
Said, E. (1978). Orientalism (New York, 1978). Said Orientalism.
Synnott, J., Coulias, A., & Ioannou, M. (2017). Online Trolling the Case of Madeleine McCann. Computers in Human Behavior, 71, 70–78.
Tham, J. (2020, April 2). Like a Virus: How Racial Hate Speech Looks Like in Malaysia During the Covid-19 Pandemic. November 08, 2020, https://www.centre.my/post/how-covid-19-influencing-racial-hate-speech-malaysia
Twitter Usage Statistics. (2018). http://www.internetlivestats.com/twitter-statistics/
Wigerfelt, A. S., Wigerfelt, B., & Dahlstrand, K. J. (2015). Online Hate Crime- Social Norms and The Legal System, 08, 1859. DOI:
Copyright information
This work is licensed under a Creative Commons Attribution-NonCommercial-NoDerivatives 4.0 International License.
About this article
Publication Date
10 June 2021
Article Doi
eBook ISBN
978-1-80296-109-6
Publisher
European Publisher
Volume
110
Print ISBN (optional)
-
Edition Number
1st Edition
Pages
1-497
Subjects
Technology, communication, social media, crisis management, organisational communication, pandemic, advertising
Cite this article as:
Karim Zamri, N. A., Mohammad, N., Mohamad Noh, L. M., Ab Aziz, A. A., Hussin, H., Ridzuan, A. R., Sah Allam, S. N., Mohideen, R. S., & Zulkipli, N. Z. (2021). Coronavirus Exacerbates Xenophobia: Deconstructing Otherness In The Twitter. In C. S. Mustaffa, M. K. Ahmad, N. Yusof, M. B. M. H. @. Othman, & N. Tugiman (Eds.), Breaking the Barriers, Inspiring Tomorrow, vol 110. European Proceedings of Social and Behavioural Sciences (pp. 332-340). European Publisher. https://doi.org/10.15405/epsbs.2021.06.02.43