Abstract
This study explores adaptation as a factor of decision quality improvement. It stems from the assertion that the quality of a decision shall be determined by the ensuing reduction of uncertainty rather than by the decision implementation outcome. The paper builds upon the tenets of transformational theory, game theory, cognitive science as well as the ideas of a quantitative description of how information resources contribute to the output of products. We investigate causal relationships associated with the cognitive trap of equating the quality of the decision with its implementation outcome. The phenomenon of adaptation is proposed to be considered as a controllable process of uncertainty reduction. We advance the thesis that transformation of quantity of knowledge into quality that occurs in an adaptive system drives the uncertainty reduction thus increasing the decision-making quality. The conjecture is tested that, at each point in time, the adaptation speed is inversely proportional to the laboriousness of training. The results obtained with two training simulators are analyzed, and the patterns of the adaptation process revealed for two types of thinking: the reflexive mind and reasoning mind. Also, we identify the features of decision making as observed in the course of controlled adaptation and determine the efficiency limits of adaptation as such controllable process.
Keywords: Adaptationcausal relationshipsdecision making improvementinformation resourceslaboriousness of trainingthinking
Introduction
In today’s world, the statement that learning is an adaptation to changing environmental conditions is axiomatic in nature (Urintsov & Dick, 2008). We learn to make decisions by adapting the signals of the environment for the system of knowledge and experience that we have at that point in time. A signal is recognized by identifying the familiar and new characteristics (signatures "aware" and "alien", respectively) of the information received. Further, the existing system of knowledge and experience, through the adjustment process, accumulates this information. Thus, either the confirmation (update) process occurs for an existing element in the knowledge and experience system, or a new element is added to the system.
Problem Statement
In the course of development of the knowledge system and expansion of the experience basis, we learn to make decisions in a better way, which on its own can be viewed as an outcome of training. Although we use a variety of parameters to evaluate the quality of decisions, their effectiveness, i.e. whether the desired state has been achieved, is conventionally regarded as the single most significant evaluation factor (Dixon, 1966; Fathollahi-Fard et al., 2018).
This approach, however, is prone to a cognitive trap where a correctly made decision "fails to deliver" due to the effect of uncertainty rather than as a result of mistakes in the decision-making process. The negative experience thus acquired is recorded in our consciousness as an undesirable outcome — an error (failure). In the future, a similar environmental signal will be read by the knowledge and experience system as having a signature "aware." Furthermore, as there already exists a pattern where the cognitive sequence and the decision subsequently made are read as an error, the same pattern will be applied to a new signal. Oftentimes, this is because our model is overly simplified. Without a conscious post-mortem intended to isolate the uncertainty factor and critically look into the input information carried by a new environmental signal, we take a risk of persistent application of an erroneous pattern. By falling into a cognitive trap and failing to realize that the decision itself was right, we keep following the same pattern while missing new decision-making opportunities.
Thus, our knowledge and experience system are adaptive, which implies its ability to select a purposeful behavior adjusted to the environment (Komleva & Dneprovskaya, 2018). To guard against falling into a cognitive trap, one can consider a short memory or a conscious attitude to the adaptation process, the latter being preferable, in our opinion. For this purpose, we suggest considering adaptation as training. Here, we understand learning as a controllable process, and more precisely, as a process of transforming external information (with signature "alien") into internal (with signature "aware").
Research Questions
The question arises as to how to improve decision quality. We have already shown that the basic condition for this is the quality of knowledge, i.e. the power to apply the "fast thinking" capability for automatic information processing. To ensure the quality of knowledge for decision making, a certain amount of knowledge shall be acquired. Therefore, such a parameter as a laboriousness of training, which is usually measured in credit units in accordance with the European Credit Transfer and Accumulation System (ECTS), should be taken into consideration. The process of high-quality knowledge acquisition is provided by means of adapting the knowledge and experience system. We can influence this process by speeding up the adaptation. This is the second parameter, which, along with the laboriousness of the training parameter, makes it possible to improve the decision quality.
Purpose of the Study
We need to check how these parameters relate to each other. If we accept as a dogma that the decision quality and the ensuing outcome quality are not the same, we can define the conjecture of the study. Suppose that at each point in time the speed of adaptation considered as the process of transforming external information into internal is inversely proportional to the laboriousness of training expressed in units of training time.
Research Methods
To define the contours of internal and external information, we will apply the terminology commonly used in psychology and cognitive science. The external information contour supports "slow thinking," and the contour of internal information supports "fast thinking" (Kahneman, 2018). "Slow thinking" is responsible for concentration, reasoning and choice, which allows us to carry out purposeful, conscious activities. "Fast thinking" is in charge of automatic data processing and reflexes, and it is associated with instinctive, intuitive decision making. While "slow thinking" is considered to be a result of the brain’s evolutionary development, most of the decisions are believed to be realized by "fast thinking" (Duke, 2018). This has ensured the survival of the species and underlies the adaptive properties of the knowledge and experience system.
The process of transformation of external information into internal, when two types of thinking are taken into account, is as follows. A purposeful, conscious operation over a data array in the process of reasoning adapts this array for our knowledge and experience system. Further, the data array is transferred to the internal information contour, thus allowing to subsequently operate it automatically, as a reflex action. The efficiency of adaptation is defined by the speed of the data transfer from the external to the internal circuit. This, in turn, allows to view adaptation as a factor determining the decision quality.
To establish the quality parameter for a decision, we will use the idea of a quantitative description of the contribution of information resources to the output of products (Solow, 2017). If the received information is taken as an information resource, the adaptation of the input data for the knowledge and experience system becomes a production process. The relationship between the effective application of knowledge and the intensification of capital utilization revealed by Solow by means of production functions implies that the quantity of knowledge in an adaptive system transforms into the quality of knowledge. The quality of knowledge, in turn, determines the quality of products, which, in our case, is the decision quality. Therefore, a high-quality decision is the result of the completed process involving an accurate mandatory assessment of the amount of knowledge available (Dik et al., 2014; Komleva & Dneprovskaya, 2018). In our opinion, however, one shall only judge a decision quality when the uncertainty factor is accounted for.
If we choose not to consider the decision quality through the prism of the quality of its outcome, thereby avoiding the cognitive trap, then the decision quality shall be assessed based on the uncertainty factor. Let’s make a reservation that, in the process of adaptation, we shall not strive to eliminate uncertainty. We see our task as learning how to make decisions with due consideration of the uncertainty factor. That is, to assess the amount of uncertainty, it is necessary to determine what exactly it is that you do not know. When you have determined what you do not know, you can seek to correct this situation. For this purpose, you consciously search for relevant information and process the identified information resource in an effort to transfer information from the external contour to the internal. Thus, the knowledge and experience system is adapted, and "quick thinking" is readying for automatic data processing and transmission for the purpose of decision making. In an adaptive system, transformation of quantity of knowledge into quality reduces the amount of uncertainty while increasing the decision quality. It follows that high-quality decision making is conditioned upon training with a given scope and objectives.
We believe that adaptation, in accordance with the provisions of transformation theory (Vernadsky, 1977), has a wave-like character with an ambiguous ratio of the heights of consecutive maximums and a necessary slump between them. The presence of slumps is a significant property as they require special support in the course of learning. Transformational theory based on the wave-like representation of development processes allows consideration of the adaptation process from the standpoint of determination of the normal distribution for this process. We believe that the criterion for the normality of distribution is the excess of the learning effect (adaptation speed) over the effect of an increase of the learning laboriousness.
Containment of an adaptation process within the limits of normal distribution makes this process controllable. The control function is performed by an operator, i.e. the subject who monitors the process of learners’ adaptation. Within the range of normal distribution, the operator can gradually increase the pace of training and expand the list of operations performed on data arrays. The position outside the normal distribution range means that the adaptation occurs beyond the limits of the expected learning path. This implies the use of special learning process support and adjustment measures based on the principles of individualization of training.
Findings
To test the conjecture, we analyzed the results of students' work with training simulators. Work with a simulator perfectly illustrates the students’ adaptation dynamics, with continuous monitoring of the learning process and a possibility to summarize and evaluate the results. The results analysis has been performed for the following simulators:
training simulator for the application of mathematical statistics methods to solve economic problems (Ivanova et al., 1991);
training simulator for the use of Adobe Captivate for the development of an electronic course (Doronina et al., 2016).
While establishing the relevance of these data to the study objectives, we found that the basic parameters of the simulators are similar, namely the types of tasks, the procedures for a learner to operate the simulators, the training periods. The number of students for the study was normalized by the linear normalization method and was subsequently summed up to collate the results for all simulators. The sample size was 64 individuals. Regarding the parameter "procedure to operate the simulator," individual and group data (4 persons per group) were analyzed separately. Regarding the "task types" parameter, the following was offered:
1. Test questions ("many out of many" answer type; outputting a result immediately after answering a question; shuffling questions and sampling 75 out of 100 in 3 units of different complexity; invariable wording of the question and answer options; shuffling the answer choices).
2. Test questions ("many out of many" answer type; outputting a result upon the test completion; shuffling questions and sampling 75 out of 100 in 3 units of different complexity; variable wording of the question and answer choices; shuffling the answer choices).
3. Situational tasks (answer option: recognizable combinations of metadata by keywords; outputting a result after completing a task; shuffling tasks and sampling 40 out of 50 in 2 units of different complexity; variable wording of the task questions). The analysis of students' work on situational tasks was carried out for two cases: solving situational tasks after passing the test and without a test.
Figures
,(1)
where
The zone of the normal distribution is shaded, it corresponds to the values between the line of the minimum value of the normal distribution and the upper boundary of the correlation field illustrating the proximity of scattering points (point coordinates for the pairwise results of a simple and complex search).
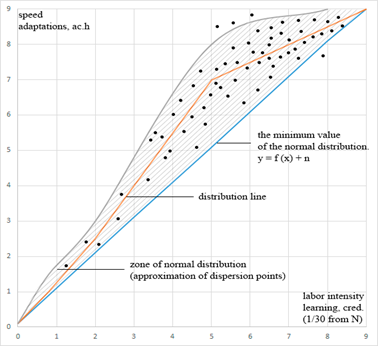
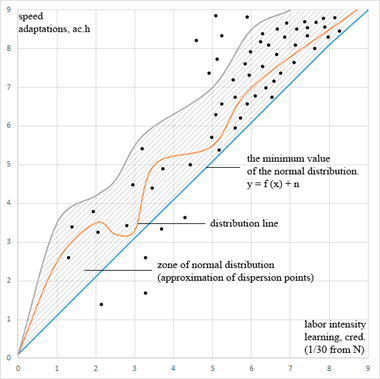
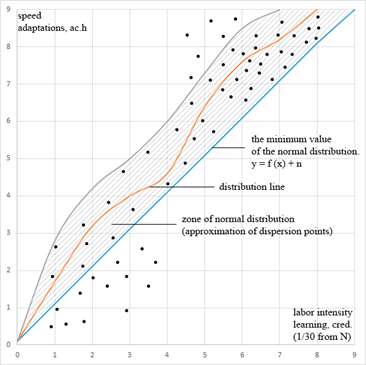
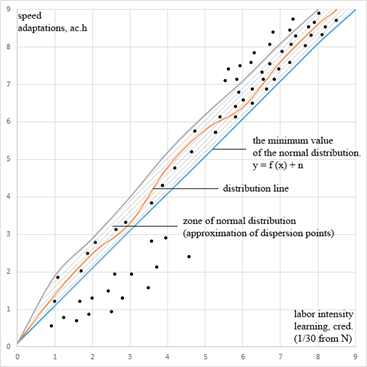
Containment of a result within the zone of normal distribution means that the adaptation speed is suitable for the training objectives, and the set mode of the simulator does not require any additional adjustment. A result outside this zone can be interpreted as follows. If a result is above the upper boundary of the correlation field, the adaptation speed is higher than expected. This result is considered as a positive deviation from the standard. Conversely, the result below the line of the minimum value of normal distribution is considered as a negative deviation from the standard where the adaptation speed is lower than expected.
For each of these cases, the operator makes a decision on the additional setting of the simulator parameters as the adaptation speed does not show a positive trend while the training laboriousness increases. This violates the criterion of distribution normality defined above, i.e. the excess of the learning effect (adaptation speed) over the effect of increasing of the learning laboriousness. It should be noted that to make a decision, the operator of the simulator has to track the individual trajectory of a learner. One needs to understand whether the deviations of the trajectory from the zone of the normal distribution are significant, whether these deviations have become a regular pattern or represent a special case of reaction to a specific task unit. Regarding a work result of the group of students, the operator makes a decision based on the analysis of the role and contribution of each member of the group.
By collating the results of students working with simulators, we drew a number of conclusions that give an idea of the basic laws of the adaptation process. We have already mentioned the types of thinking (fast and slow) to illustrate the transition of external information to the internal state. This transition is a pattern of human cognitive activity (Doronina et al., 2016), and we can influence its characteristics by developing a conscious behavioral line with respect to adaptation as a learning process. However, it is quite obvious (and proven by the analysis of the work with the simulators) that people adapt differently. There are not so many indications of dissimilarity (which is also demonstrated by the ratio of results inside and outside the zone of normal distribution), but differences in the adaptation dynamics still exist.
We believe that these differences depend on individual psychological and cognitive characteristics of thinking, which are polarized along with two directions dubbed "reasoning mind" and "reflexive mind" by Markus (as cited in Еrgunova et al., 2017). The propensity to display one of them by inertia as a reaction to the need for a decision affects the speed of adaptation and the quality of the decision itself. Taking into account these characteristics of thinking, the following patterns of the adaptation process have been identified:
the decision quality is improved if the decision-making process is dominated by the "reflexive mind" and a reaction to the inputs occurs; (the decision quality here and further down the list is understood as the ratio of the adaptation speed to the training laboriousness;
the volume of knowledge boosts the activity of the "reflexive mind" (knowledge applied at a level of automatic reaction has an advantage with respect to the decision quality as compared to the knowledge used as an evaluation factor);
the factor of time limitation for decision making determines the primacy of the reaction – the activation of the "reflexive mind";
the duration of the slump between the maximums of the activity waves is more typical for the "reflexive mind";
a significant difference between the maximums of the waves (ratio of the activity peaks) is more typical for the "reflexive mind";
the need for external support (from the operator) during the activity slump is more typical for the "reflexive mind".
In addition to the adaptation process patterns depending on the characteristics of thinking that were revealed by the results of data generalization, some features of decision making in the process of controlled adaptation were found:
the quality of an individual decision is lower than the quality of the decision made in the group (collectively);
the value of a group member contribution to a collective decision is determined, by and large, by a social role in the team (as a result of the demonstration of an individual behavior model in the group);
the quality of the decision is inversely proportional to the volume of new input data (control over the input of new data reduces the degree of uncertainty);
the duration of the decision-making process is directly proportional to the number of individuals that make up a group (if the group is randomly sampled; their dependency is not observed for established teams);
the quality of an individual decision is determined by the degree of conscious behavior (the presence of intrinsic motivation increases the interval between the beginning of the process and the deliberate abandoning further attempts to improve one’s own result);
the restriction of access (number of attempts) to verification of inputs improves the decision quality;
an increase in the number of evaluation factors increases the error probability by increasing the variability of the solution;
the quality of decision making is inversely proportional to the number of universal solutions;
the quality of the decision is inversely proportional to the degree of detail of the required state (the establishment of limitations on decision making reduces the quality of the decision).
Conclusion
Turning to the proposed conjecture, it should be recognized that not for all cases at each point in time the speed of adaptation is inversely proportional to the training laboriousness (Marcus, 2008). Summary of the analysis of the results for simulators showed that the feedback principle is violated if the adaptation speed does not change or changes slightly. In these cases, we observe a gradual departure of the adaptation process parameters beyond the limits of the zone of normal distribution. Thus, we have established the limits of the efficiency of adaptation as a controllable process. A controllable process outside its boundaries calls for a change in the adaptation format. A controllable process within its efficiency boundaries is aimed at improving the quality of decision making. The path we propose involves the development of a conscious attitude towards the assessment of the decision quality through the determination and reduction of the amount of uncertainty as opposed to the assessment of quality through the prism of the ensuing outcome. The determination, in the process of controlled adaptation, of the amount of uncertainty expressed in the paradigm of "that what you do not know" helps to overcome the evolutionary inertia of the reflexive and reasoning mind. The controllable adaptation has yet another positive feature. It does not allow the appeal of reduction of the amount of uncertainty to replace the decision-making process, thereby focusing attention on the adaptation effect.
Acknowledgments
The research was funded by the grant of the President of the Russian Federation for national support to leading academic schools (grant No. NSh-5449.2018.6).
References
- Dik, V., Urintsov, A., Dneprovskaya, N., & Pavlekovskaya, I. (2014). Prospective of the e-learning toolkit enhanced by ICT development. Naukovyi Visnyk Natsionalnoho Hirnychoho Universytetu, 4, 152-156.
- Dixon, J. R. (1966). Design Engineering: Inventiveness, Analysis, and Decision Making. McGraw-Hill.
- Doronina, I., Kulikova, N., Razzhivin, O., Kostyushkin, Y., Silnov, D., & Sadovnikova, N. (2016). Human resource management features of an innovative cluster. Review of Management and Marketing, 6, 57-62.
- Duke, A. (2018). Thinking in Bets: Making Smarter Decisions. Penguin.
- Еrgunova, О. Т., Lizunkov, V. G., Malushko, E. Yu., Marchuk, V. I., & Ignatenko, A. Yu. (2017). Forming the system of strategic innovation management at the high-tech engineering enterprises. OP Conference Series: Materials Science and Engineering, 177(1), 012046. https://doi.org/10.1088/1757-899X/177/1/012046
- Fathollahi-Fard, A. M., Hajiaghaei-Keshteli, M., & Tavakkoli-Moghaddam, R. (2018). The Social Engineering Optimizer (SEO). Engineering Applications of Artificial Intelligence, 72, 267-293.
- Ivanova, V., Emelyanov, S., & Urintsov, A. (1991). Using Statistical Criteria for Solving Economic Problems Using an IBM PC PC. Social and Economic Research Using Mathematical Statistics Methods, 57-59.
- Kahneman, D. (2018). Thinking, Fast and Slow. Penguin.
- Komleva, N., & Dneprovskaya, N. (2018). Technologies for creating and acquiring knowledge in the open information environment. Technologies for the European Conference on E-Learning (ECEL), 254-262.
- Marcus, G. F. (2008). The Birth of The Mind: How A Tiny Number Of Genes Creates The Complexities Of Human Thought. Basic Books.
- Solow, R. (2017). Economy for the Curious. What Nobel Laureates are Thinking about. Gaidar Institute Publishing.
- Urintsov, A., & Dick, V. (2008). Systems of Formation and Decision Making under the Informatization of Society. Eurasian Open Institute Publishing.
- Vernadsky, V. (1977). Reflections of a Naturalist: Scientific Thought as a Planetary Phenomenon. Science.
Copyright information
This work is licensed under a Creative Commons Attribution-NonCommercial-NoDerivatives 4.0 International License.
About this article
Publication Date
28 December 2020
Article Doi
eBook ISBN
978-1-80296-098-3
Publisher
European Publisher
Volume
99
Print ISBN (optional)
-
Edition Number
1st Edition
Pages
1-1040
Subjects
Multicultural context, learning environment, modern society, personality formation, informatization of the society, economics and law system of the region
Cite this article as:
Mamedova, N. A., Urintsov, A. I., Staroverova, O. V., Afanasev, M. A., Haritonov, S. V., & Galahov, D. V. (2020). Adaptation As Controllable Process For Decision Making Improvement. In N. L. Shamne, S. Cindori, E. Y. Malushko, O. Larouk, & V. G. Lizunkov (Eds.), Individual and Society in the Modern Geopolitical Environment, vol 99. European Proceedings of Social and Behavioural Sciences (pp. 643-652). European Publisher. https://doi.org/10.15405/epsbs.2020.12.04.74