Abstract
As a lot of terrorist and extremist groups are increasingly using online forums to share their ideas and recruit new members, methods for detection of online radical content and authors are more crucial than ever. A great deal of scholarly effort has been focused on detecting extremist content, but the problem of detection of online traces of potential extremists is still in its infancy, mostly due to the lack of relevant studies of their language behavior in different contexts. In this paper, we aim to analyze the language behavior of authors from an extremist-oriented and neutral thread of a Russian-language extremist forum in many other threads. Using supervised and unsupervised text classification techniques and simple linguistic features, we have been able to separate these two groups of authors. In the next steps, we performed a comparative analysis to reveal the differences in language use by these two groups. We compare our findings with the results reported in the literature mostly for English language texts. We conclude by stressing the necessity to study the language behavior of different authors of the extremist forum in a variety of contexts to better understand the psychology of the radicalized mind.
Keywords: Extremist forumextremist groupsonline radical contentpsychology of the radicalized mindstylometric studyterrorist groups
Introduction
In recent years, radical extremists have been using the Internet to spread their propaganda and recruit new members more and more actively. Law enforcement agencies and scholars are searching for methods of revealing different types of radical content. Much less attention, however, is being paid to revealing radical authors. It is crucial to detect not only extremist content but prolific authors who intensively promote violent extremist ideas. By now, detection of potential extremists through analysis of their online activity is in its infancy (Scrivens et al., 2018). Existing “computer” approaches to identification of radical authors rely mostly on sentiment analysis combined with Parts-Of-Speech tagging (Scrivens et al., 2018) or using n-grams of words and symbols (Oussalah et al., 2018). Meanwhile, most recent studies show that adding psychological features yield higher accuracies of predictive models (Nouh et al., 2019).
Problem Statement
In order to develop the methods for identifying potential radical authors online, it is crucial to analyze their language to assess their personality as the way people express themselves in a language is a reflection of their personality and psychological states (Pennebaker, 2011). Although individuals expressing extreme views may never commit acts of violence, they represent the base of a pyramid-shaped radicalization typology (McCauley & Moskalenko, 2008), while terrorists are at the top of this pyramid. Understanding the linguistic patterns of this base of supporters could shed some light on the phenomena related to radicalization.
There have been some attempts to analyze the language style of radicalized authors as the projection of their personality. Baele (2017) investigated speech by a lone terrorist using LIWC, widely used content-analysis software (Pennebaker et al., 2015). He tested two hypotheses about the personality of the terrorists based on the previous findings. First, he stated that terrorists are characterized by a very high level of negative emotions, especially anger. The second claim is about the specific of cognitive style, namely hypothesis linking cognitive inflexibility and unsophistication with extremism and violence. In sum, lone-actor terrorists are indeed characterized by high levels of negative emotions. Another clear difference between nonviolent and violent radicals was their use of “they” pronouns, which is indicative of their polarizing way of thinking. Torregrosa et al. (2020) analyzed tweets by ISIS supporters on a large dataset as compared to general users of Twitter using LIWC as features. They concluded that ISIS supporters used more third-person plural pronouns; words with six letters or more; and that their language contained higher levels of anger and negative emotion. The outcomes of this study were generally consistent with similar studies using LIWC to investigate the language of political extremists. Therefore, the language of ISIS supporters can be broadly seen to share many characteristics of the language of other political extremists.
Two papers cited above used theory-driven methodology which relies on the existing theory of extremist personality and cognition and a dictionary-based software. However, this approach could be inapplicable to non-English texts. In the search for an approach which can be applied to any language, the authors of (De Smedt et al., 2018) used character trigrams as features, “so that the system can deal with spelling errors, word endings, etc., more efficiently” (De Smedt, Pauw, & Van Ostaeyen, 2018) and applied the keyword extraction approach. Although this work is aimed at detecting hate speech samples (they used a combined dataset consisting of the samples of different types of extremist texts), it is notable that the authors also performed cross-domain classification experiments in the search for universal signals of hate speech.
Nouh et al. (2019) used a combined feature set. They found that radical Twitter users exhibit distinguishable textual, psychological, and behavioural properties. One of the most important textual features was the Us-them dichotomy calculated based on the total number of pronouns used (I, they, we, you), word unigrams, violent word ratio, percentage of long words (more than 6 letters), and allCaps features.
All the cited papers are focused predominantly on English language data. Second, they compared texts by radical authors with those by general users. In our study, we aim at comparing the language behavior of two different groups of active members of the Russian-language extremist forum.
Research Questions
Our first research question is: do authors from a radical-oriented and a more general topic remain consistent in their language behavior under context change, i.e. in the other threads of the forum? If so, what are the typical characteristics of their language use and how do they relate to previous findings regarding the language behavior of radical authors?
Purpose of the Study
We analyzed posts from KavkazChat dataset which is part of a Dark Web Forum collection (Chen, 2012). KavkazChat is a Russian-language forum focusing on jihad in the North Caucasus. It is included on the Russian Federation Federal list of extremist materials.
First, we excluded all the messages starting with ‘Citation’ or ‘QUOTE’. Then we collected posts from two topics (threads) from the forum: a neutral “What would you say about the user above?” and a more extremist-oriented “Heroes of the Caucasian Jihad – Amirs, Mojaheds, Shahids”. Both of these threads are in the ten most frequent threads in KavkazChat dataset. For every thread described above 10 most active authors were chosen. The 10th most active user in “What would you say” (it will briefly be referred to as
The authors from
Research Methods
We analyze texts using different stylometry methods. Stylometry is a quantitative analysis of text characteristics (Eder). In the present study, we aim at classifying texts as written by radically oriented or neutral authors to reveal the strength of differences between two groups of authors through different forum topics. We use relative frequencies of most frequent words, word bigrams and character 5-grams as features since our data contain a lot of noise (errors, slang words, Arabic terms, non-Russian words written in Cyrillic, etc.) which can be a cause of poor accuracy of tools aimed at extraction of more sophisticated features (for example, morphological taggers). For this reason, we also did not perform lemmatization.
We use classifiers implemented in R package Stylo (Eder et al., 2016) (tokenization and feature extraction has been performed with this package). To find structure in data, we also applied unsupervised techniques implemented in Stylo (for more details on the stylometric analysis of extremist texts see (Litvinova & Litvinova, 2020). We purposely did not remove stop words as it has been shown in a large body of papers (see Introduction) that function words are crucial for linguistic analysis of extremist texts.
In order to identify words which distinguish groups of authors, we used RStylo function oppose (see details below) and performed the chi-square test.
Findings
Unsupervised and supervised approaches to post-classification
We performed a series of cluster analysis and constructed bootstrap consensus tree (BCT) (the technique used to avoid instability of cluster analysis depending on the number of features, see (Eder et al., 2016 for details) with different distance measures and numbers of features and obtained strikingly similar results: texts by the authors of the same group are (in general) closer to each other than those by the authors from different groups. For the sake of brevity, in Figure
We also performed an analysis with the same settings but using bigrams of words. Although tree structure is quite different (groups are smaller, often contain only two members, but still the authors from the same groups are clustering together, in one leaf). The same procedure has been performed for character 5-grams (punctuation marks were removed, but white spaces were preserved), but the same pattern has been revealed.
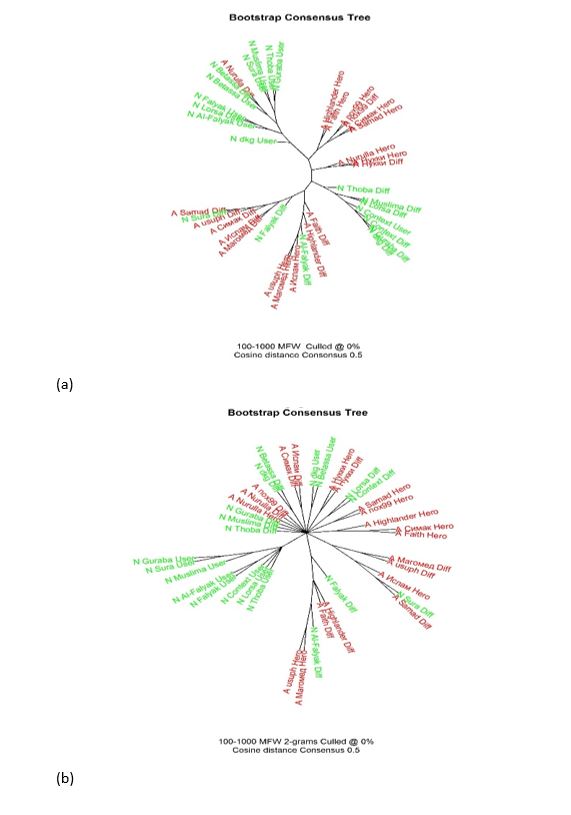
Next, in order to identify the strength of group signal in texts, we move to the classification phase. We performed classification in two scenarios: first, texts from
The best results for the first scenario and MFWs were obtained with Cosine Delta. SVM performed slightly worse (80%, sd =3.3%). For two other types of features, SVM outperformed other classifiers.
In the second scenario, all the classifiers exhibit high accuracies for MFWs (higher than 80%) but only SVM worked well (higher than 80%) for word bigrams. SVM with another type of features did not show accuracy lower than 80%. Overall, the first task turned out to be more difficult but still it is possible to detect the authors from Hero/User topic in the other topics.
Since we came to the conclusion that two groups of authors are separable through different topics, next we move on to searching for typical characteristics of their language use.
Comparison of the word frequencies in the texts by the two groups of authors
We performed a comparative analysis using Stylo function oppose. This function performs a contrastive analysis between two given sets of texts, using Burrows's Zeta in its different flavors, including Craig's extensions (for references and details about these measures we refer the reader to (Eder et al., 2016; Hoover, 2010). The function takes two sets of texts as input and outputs words significantly preferred and avoided by texts in one set (as compared to the other). The usefulness of this approach for comparison of several groups of texts is that Zeta ignores frequencies of the words and concentrates on their consistency. This helps to eliminate the effect of individual voices while comparing texts by the group of authors. It is known that Zeta analysis excludes the extremely common words and concentrates on the middle of the word frequency spectrum (Hoover, 2010).
For the ongoing study, we used the following settings: text slice length = 1000 words, text slice overlap = 500, rare occurrences threshold = 5, zeta filter threshold = 0.1 (default). We used Craig’s extensions of Zeta as the opposing methods.
Visualization of the results of this method is presented in Figure
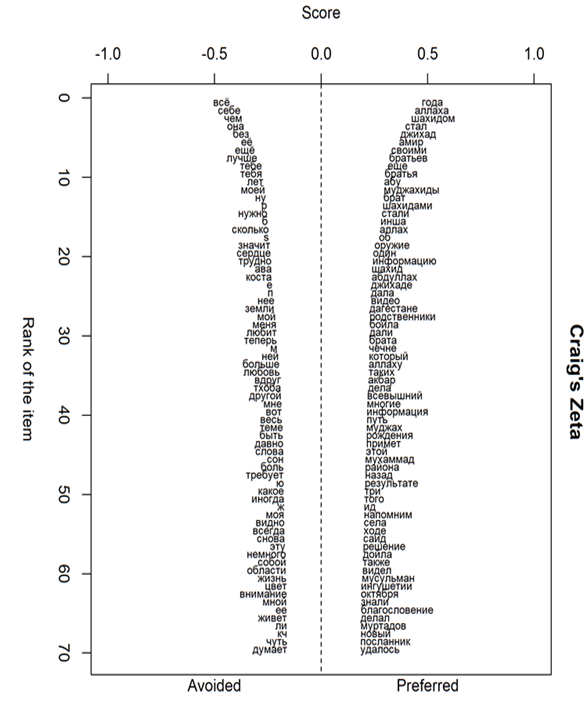
We limited our analysis to the first 100 words with the highest degree of discriminative strength.
After that, we manually tagged them as belonging to one or several categories which had been shown to be relevant to extremist discourse analysis (in addition to the categories described in the studies cited above we also used categories presented in (Baker & Vessey, 2018).
We have also compared word frequencies in all the texts written by radical and neutral authors using chi-squared test (p < 0.001) (De Smedt, Pauw & Van Ostaeyen, 2018) and tagged them in the same way.
Below we summarize the results across the above described experiments.
Pronoun usage
The neutral authors more frequently use singular personal pronouns (мне “me”, себе “to myself/herself/himself/themselves”, она “she”, ее “her”, он “he”, ему “him”), possessive pronoun сан (“my” in Chechen), моя (“my”).
Among the words preferred by the authors from Hero thread are the personal pronoun мы “we”, possessive pronouns своими “their”, них, их “them”. Similarly to the previous findings, our analysis shows that the radically-oriented authors create a dichotomy and promote the mentality of dividing the world into “us” versus “them”.
Emotion words
Words related to emotion (любит “love” in the present tense, любовь “love” noun, радость “joy”), other states and feelings (могу “I can”, трудно “it is hard”) are preferred by the neutral authors but avoided by those from Hero thread. No words denoting emotions and feeling were revealed in the top 100 words preferred by the authors from Hero thread. This contradicts the previous findings regarding preferences for words describing negative emotions as one of the typical characteristics of extremist authors.
Religion
Unsurprisingly, a lot of words related to religion were among preferred by the authors from Hero thread. The word related to this area, аллах “Allah”, is the second most discriminating word. This word was found to be most frequent in this category in the study (Baker & Vessey, 2018) performed on English and French extremist texts. The other frequent words from the category are “иншааллах “Insha'Allah” – Arabic “God willing”; всевышний “God”, ислам “Islam”, мусульман “Muslims”, Мухаммад “Muhammad, Arab religious, social and political leader and the founder of Islam”, etc.
Violence, war and death
Words from these group are characteristic of the radically oriented authors. One of the most frequent words in this group is джихад “Jihad” which is generally translated as a struggle with a praiseworthy aim but usually in the forum is normally interpreted in the violent sense as in the English texts described in (Baker & Vessey, 2018). Another frequent word is моджахед “mujahid” – the term for someone engaged in Jihad. One more frequent word is шахид “shahid”, a man who is considered to have accepted or even consciously sought out their own death in order to bear witness to their Islamic beliefs. The other words from this group are оружие “weapon”, убит “killed”. In the study dedicated to the analysis of jihadist rhetoric in tweets (De Smedt, Jaki et al., 2018), with an 81 % probability, a tweet constituted hate speech if keyword “jihad” appeared in it, with a 96% one – if keyword “mujahid” was used in a tweet, with a 67% one if the word “kill” was used.
References to names of countries, cities and more general references to place
These names are among the categories preferred by the authors from Hero thread: Дагестан “Dagestan”, Ингушетия “Ingushetia”, Нальчик “Nalchik”, Чечня “Chechnya”. While in the extremist texts analyzed in (Baker & Vessey, 2018) this category consisted of words related to the Western countries, in this forum words related to Caucasian region are used, which is caused by the thematic of the forum and interests of the authors.
Slang
The word братья “brothers” and its forms is among the most frequent words preferred by the authors from Hero thread. It is used as in-group expressions to promote group identity. The same was found in different types of extremists’ texts in (De Smedt, Jaki et al., 2018; De Smedt, Pauw, & Van Ostaeyen, 2018).
Overall, our results, on the one hand, support the previous findings regarding the dichotomy “us-they” with a concentration on in-group identity (no differences with respect to the notion of “them” were found), which has been shown to be typical of extremist texts as well as a high number of words related to violence, war and death. Of course, no one word or another linguistic unit could be a signal by itself but only a combination of them can be used for analysis.
In our data, no differences in the use of negative emotion words have been found, although the neutral authors used more positive words. This fact stresses the necessity to carefully choose the texts for comparison when dealing with extremist texts.
Our study has some obvious limitations caused by small sample size and possible bias in choosing forum topics. While fully aware of these limitations, we made an attempt at showing the importance of constructing language profiles of different groups of authors from the same community.
Conclusion
In this paper, we have analysed the language behavior of two groups of authors from the extremist forum in a variety of contexts, namely forum threads. We have discovered that irrespective of the thread topic, these two groups of authors have different preferences in word usage, which allows them to be separated using text classification techniques. Then we performed a contrastive analysis of the texts by these groups of authors and revealed some characteristics of language use, which is partially in line with previous findings but on the other hand, contradicts them. As the main goal of practical endeavours in this field is to detect most prolific users supporting and spreading violent ideas, more attention and scrutinity is required in order to analyse their speech production as a mirror of their personality.
We are planning to perform a comparative study of posts by different groups of the extremist forum authors using a wider range of linguistic features and construction of their psychological profiles by comparing their texts with those by individuals with the known personality traits. We argue that this will contribute to a better insight into the psychology of radicalized authors.
Acknowledgments
Funding of the project “Speech portrait of the extremist: corpus-statistical research (on the material of the extremist forum “KavkazChat”)” from RF President's grants for young scientists (no. МК-5718.2018.6) is gratefully acknowledged.
References
- Baele, S. J. (2017). 'Lone-Actor Terrorists' Emotions and Cognition: An Evaluation. Beyond Stereotypes. Political Psychology, 38(3), 449-68. https://doi.org/10.1111/pops.12365
- Baker, P., & Vessey, R. (2018). A corpus-driven comparison of English and French islamist extremist texts. Int. J. of Corpus Linguistics, 3, 255–78. https://doi.org/10.1075/ijcl.17108.bak
- Chen, H. (2012). Dark Web. Exploring and Data Mining the Dark Side of the Web. Springer. https://doi.org/10.1007/978-1-4614-1557-2
- De Smedt, T., Jaki, S., Kotzé, E., Saoud, L., Gwóźdź, M., De Pauw, G., & Daelemans, W. (2018). Multilingual Cross-domain Perspectives on Online Hate Speech. CLiPS Technical Report Series. arXiv:1809.03944 [cs.CL].
- De Smedt, T., Pauw, G., & Van Ostaeyen, P. (2018). Automatic Detection of Online Jihadist Hate Speech. CLiPS Technical Report Series. CTRS-007. arXiv:1803.04596 [cs.CL]]
- Eder, M., Rybicki, J., & Kestemont, M. (2016). Stylometry with R: A package for computational text analysis, R Journal, 1, 107-21.
- Hoover, D. L. (2010). The Craig Zeta Spreadsheet. Book of abstracts of Digital Humanities 2010. http://dh2010.cch.kcl.ac.uk/
- Litvinova, T., & Litvinova, O. (2020). Analysis and Detection of a Radical Extremist Discourse Using Stylometric Tools. In T. Antipova & A. Rocha (Eds), Digital Science 2019. DSIC 2019. Advances in Intelligent Systems and Computing, 1114. Springer. https://doi.org/10.1007/978-3-030-37737-3_3
- McCauley, C., & Moskalenko, S. (2008). Mechanisms of political radicalization: Pathways toward terrorism. Terrorism and Political Violence, 20(3), 415–433. https://doi.org/10.1080/09546550802073367
- Nouh, M., Jason, N., & Goldsmith, M. (2019). Understanding the Radical Mind: Identifying Signals to Detect Extremist Content on Twitter. 2019 IEEE International Conference on Intelligence and Security Informatics (ISI), Shenzhen, China, 2019, 98-103. https://doi.org/10.1109/ISI.2019.8823548
- Oussalah, M., Faroughian, F., & Kostakos, P. (2018). On Detecting Online Radicalization Using Natural Language Processing. In H. Yin, D. Camacho, P. Novais, & A. Tallón-Ballesteros (Eds.), Intelligent Data Engineering and Automated Learning – IDEAL 2018. IDEAL 2018. Lecture Notes in Computer Science (vol 11315, pp. 21-27). Springer. https://doi.org/10.1007/978-3-030-03496-2_4
- Pennebaker, J. W. (2011). Using computer analyses to identify language style and aggressive intent: The secret life of function words. Dynamics of Asymmetric Conflict, 4(2), 92-102. https://doi.org/10.1080/17467586.2011.627932
- Pennebaker, J., Boyd, R., Jordan, K., & Blackburn, K. (2015). The development and psychometric properties of LIWC2015. University of Texas at Austin.
- Scrivens, R., Davies, G., & Frank, R. (2018). Searching for signs of extremism on the web: an introduction to Sentiment-based Identification of Radical Authors. Behavioral Sciences of Terrorism and Political Aggression, 1, 39-59. https://doi.org/10.1080/19434472.2016.1276612
- Torregrosa, J., Thorburn, J., Lara-Cabrera, R., Camacho, D., & Trujillo, H. M. (2020). Linguistic analysis of pro-ISIS users on Twitter. Behavioral Sciences of Terrorism and Political Aggression, 12(3), 171-185. https://doi.org/10.1080/19434472.2019.1651751
Copyright information
This work is licensed under a Creative Commons Attribution-NonCommercial-NoDerivatives 4.0 International License.
About this article
Publication Date
28 December 2020
Article Doi
eBook ISBN
978-1-80296-098-3
Publisher
European Publisher
Volume
99
Print ISBN (optional)
-
Edition Number
1st Edition
Pages
1-1040
Subjects
Multicultural context, learning environment, modern society, personality formation, informatization of the society, economics and law system of the region
Cite this article as:
Litvinova, T. (2020). Two Different Voices On An Extremist Forum: A Comparative Stylometric Study. In N. L. Shamne, S. Cindori, E. Y. Malushko, O. Larouk, & V. G. Lizunkov (Eds.), Individual and Society in the Modern Geopolitical Environment, vol 99. European Proceedings of Social and Behavioural Sciences (pp. 526-535). European Publisher. https://doi.org/10.15405/epsbs.2020.12.04.61