Abstract
The article deals with the problems of education in the context of modern globalization, namely the organization of personalized student distance learning. A special approach is proposed on the decision making support in the distance learning educational service management. It is based on the selection of educational strategy that takes into account individual characteristics of the recipient of educational services. The article studies the methods on how to determine the distance learning student expertise profile which contains information on the student’s aptitude for distance learning, their knowledge and representative system, followed by classification of students according to the profile data and the expert’s opinion. An individual study plan will be developed for each student according to the analysis of the student profile data which is the main priority of the personalized educational service approach. The article analyzes the specifics of the distance education, its pros and cons, identifies existing problems. A special approach has been developed to determine the profile of a student taking into account their individual characteristics so as to determine the informational, functional and structural aspects of the system and define its requirements. There is a special approach on classifying distance learning students using a fuzzy logic method that allows for the subjectivity of the student profile data. This method and the collective expert opinion help to reduce the subjective influence in assessing the student profile.
Keywords: Clusteringcollective expert opiniondecision making supportdistance learningfuzzy decision treestudent profile
Introduction
The modern society understands the term Globalization as a complex and large-scale process of changes that erase the borders between countries. Globalization influences all spheres of our society - economic, political and legal spheres, art, science and education.
The appearance and widespread use of distance learning (DL) is the result of global changes in our society. The modern education system should keep up with the fast pace of human life, be convenient and available around the world. In order to be in demand and competitive in the modern labor market a person needs to constantly improve their qualifications, to acquire new skills and competence. The basis of distance learning development is the distribution and introduction of information and communicative technologies in the educational system. The learning process that is based on the use of distance technologies is more flexible in educational program and study time matters. The key task here is to develop a special approach that will help to organize distance educational process that takes into account the individual characteristics of a student when creating the study plan and deciding on the way how the information should be presented. The distance educational system is a complex social and economic structure that implies the development of special methods and decision making support models in the educational service management.
The social economic system is a complex of resources and economic entities that form the social and economic structure with its elements interconnected and interacting with each other in the field of production and consumption, exchange and distribution. The authors of the work The Social System Approach to Institutions: Examples from Western Economic History (Solomon, 2016) view the social economic system as a complex, multi-agent system that changes over time. The authors believe that the interaction of agents between subsystems affects the whole social system.
The role of educational services in the formation of the social economic system is significant. The educational service development has a direct impact on the living standards. The authors of the work Modeling Technical and Service Efficiency (Tsionas, Assaf, Gillen, & Mattila, 2017) present a new model that is suitable for evaluating both service and technical efficiency. This model incorporates an output distance feature using Bayesian output methods arranged around the Monte Carlo Markov Chain (MCMC). These conclusions suggest that modern methods of evaluation of the educational service efficiency are needed.
Foreign and Russian scientists have made a significant contribution to the study of service management. The current issue is the management of distance learning educational services. A large number of publications are devoted to this topic.
The author of the work Perspectives of Development of the Educational Services Market in Regions of Russia in the Conditions of the Knowledge Economy Formation (Baranova, 2018) proves the thesis that the development of the educational services market in regions of Russia is possible in the context of knowledge economy formation. The author studies the problems of the educational services market development through the prism of international trends and gives recommendations on creating favorable conditions for the development of the educational services market in the Russian regions.
The authors Reikhanova and Bukina (2017) present their own mechanism of educational services management optimization designed to ensure the continuity of all levels of education using new information and communicative technologies.
The problem of educational service quality is examined in the article Determinants of Service Quality in Education: Service Provider's Perspective and Academician's Perspective (Kamakoty, Sohani, & Sohani, 2015). The authors list the determinants of the educational service quality. In conclusion they give recommendations on improving the service quality in educational institutions.
Courtney and Wilhoite-Mathews (2015) study the evolutionary development of the distance education. The authors give a detailed description on how to use various tools to create an educational platform and how this will improve the cooperation between teacher and student.
Gerasimova, Melamud, Tutaeva, Romanova, and Zhenova (2018) study the use of information technologies and systems at the distance education faculty in the Plekhanov Russian University of Economics. The authors study and analyze a series of student surveys on the distance learning efficiency. The study results show the tendency of moving away from the traditional education to education based on the use of information technologies.
The issue was also studied by Ozcan and Zeynep (2016). The purpose of their study was to determine how students themselves can assess the distance education efficiency. The results showed a positive feedback.
Swart (2015) in his study shows how students use the learning management system at the Public Distance Education Institute in South Africa. The author studies the correlation between the use of special systems and the student academic performance. The final results show that students who use the learning management system are more likely to succeed in public distance learning.
Liu and Yen (2014) raise the question of improving the distance education quality and the education efficiency at the universities under study. For data analysis the authors use SPSS19.0 quantitative survey which is based on factor analysis, reliability analysis, regression analysis and deviation analysis. The results of the study show that distance technologies have a positive impact on learning process. The authors note the correlation between the use of distance education and the education efficiency.
The article by Mudrakova and Bindyukova (2015) underlines the importance of distance learning technologies in the traditional school educational system. The model is based on the use of such distance learning technologies as gamification, credit-distance technology, inverted lesson technology, social educational networks as the distance education technology. These technologies are considered a new form of knowledge acquisition and solve a number of educational problems.
The article by Leontev (2016) deals with the innovative structures and the distance education components. The author proposes an innovative nonlinear andragogical learning model. The model is based on constructive and problem-oriented approach.
The study by Labus, Simic, Barac, Despotovic-Zrakic, and Radenkovic (2012) is devoted to the issue of integration of social networks into educational process. The aim of their research is to improve the e-learning process quality by encouraging communication, cooperation and interaction between students and teachers. The research results showed that the students who use Facebook for educational purposes succeed better compared to those who use it for entertainment.
The article by Ekren, Karatas, and Demiray (2015) emphasizes the importance of leadership in distance education management. The authors give definition of leadership in the context of distance education which is different from that of traditional education.
Ling (2019) notes the importance of high-quality technologies of live broadcasting and video playback in the distance learning process. The author studies digital image processing technologies that that can improve the quality of distance learning.
Problem Statement
Distance learning is a complex social and economic system that can be easily adjusted to suit any environment. The distance learning process management is a combination of technologies and methods that allow a student to receive all the necessary education materials through electronic interaction with the teacher during the learning process.
The specificity of DL-process requires theoretical justification of various problems of the distance learning process, i.e. organizational, methodological, legal, psychological problems, etc. Those include: lack of clear learning objectives and requirements for student’s basic skills; poor knowledge-check system; lack of content requirements for the distance learning course and teaching aids; lack of centralized planning and accurate responsive control over the educational material development process; poor economic stimulation of teacher’s educational and research activity in the field of innovative educational technologies; poor regulation and standard database on determining the composition and content of the distance learning educational materials; tendency to use the traditional teaching methods and ‘’computer phobia’’ present with some of the teaching staff; insufficient consideration of student’s individual characteristics in creating e-learning educational materials. One more problem is the lack of effective tools, methods and models that support the distance learning management. Thus, the aim of the study is to develop a special mathematical and algorithmic support for a personalized distance learning management support system.
To provide high-quality education the university should ensure the distance learning efficiency. Traditionally, distance learning management involves: deciding on the course (individual and group, automatic (according to the rules of the system), according to the test results or other forms of assessment); the course termination; the study program timing (sending out notifications, analytical reports) (Startseva & Bogdanova, 2015).
The DL-educational process is provided by the course-developer teachers and teacher advisors (tutors); managers and heads of departments, information resources system administrators; designers and programmers; technical support staff. To ensure the coordinated work of all specialists it is necessary to use the distance learning management support system. Figure
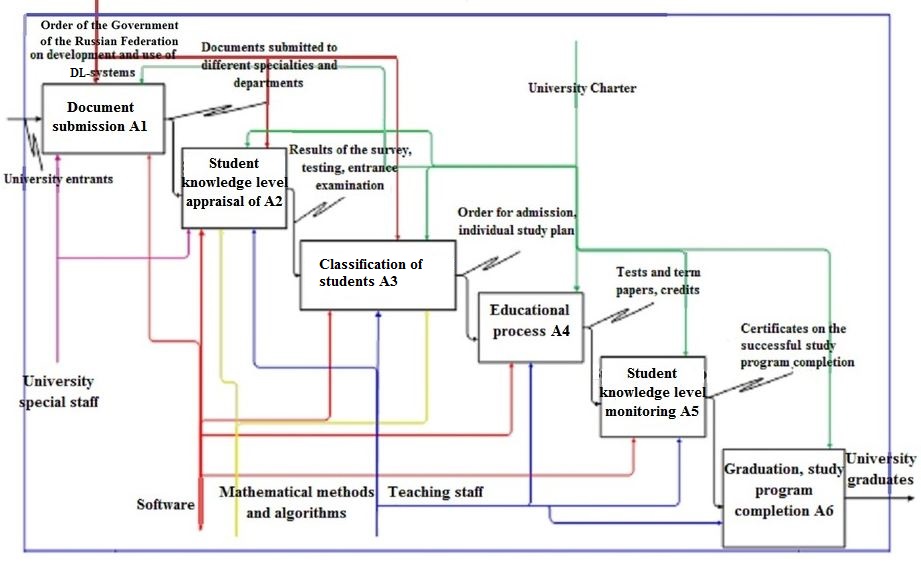
To organize the personalized distance learning process it is necessary to prepare an appropriate study plan that will suit every student. The personalized DL-process approach consists of the following stages: student self-assessment through a questionnaire, evaluation of the student profile parameters, student profile compilation, student clustering and creating of an appropriate study plan.
Research Questions
In modern DL-education systems the profile provides the key information about the student: name, surname, study field, information on courses and grades. However, there is no unified student profile standard, as well as no requirements on what information it should include. Current profiles are of no use as a student cannot even choose a suitable education mode, i.e. when the student receives an assignment their personal characteristics and individual capabilities are not taken into account. The profiles are only used to show the information. According to the proposed approach a new profile standard will allow the student to organize their studies as they wish.
In the course of the analysis of distance learning student’s activities and the DL-system operation three main criteria for assessing student abilities were formulated: psychological, intellectual and individual. Although the system that takes into account DL-student’s individual characteristics has been primarily designed to create an accurate student profile, it will not be able to predict the student’s psychological behavior during studies, as there are no technologies that could completely imitate this process. The intellectual criterion will represent the student’s specialized knowledge, as well as the general knowledge and skills. The individual criterion will represent the knowledge acquisition process.
Thus, the student profile consists of three categories (figure
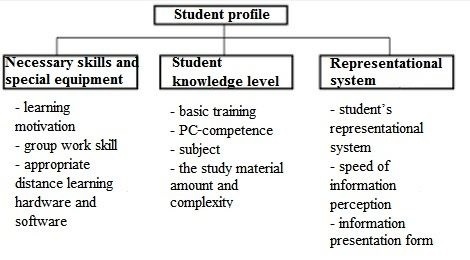
This profile most fully reflects the student’s abilities and skills and will promote the distance learning personalization. All the parameters will be determined through testing and survey. The student profile, as well as teaching recommendations will be made according to the results of all tests/questionnaires.
Ten criteria are divided into three categories; each category has its own characteristics but they can be assessed according to one assessment scale. Thus, to simplify student clustering, we can remove several criteria that do not seriously affect the student profile compilation. However, it is impossible to remove them completely, since these criteria contain information on the student’s study field preference.
All profile criteria represent a certain characteristic of a student and yet they describe different aspects (Table
Purpose of the Study
Since determining of the profile parameters and the level of student’s knowledge is carried out through survey and testing, it should be taken into account that this assessment is carried out by the students themselves and, consequently, is subjective. It is human nature that we are unable to think numerically but make a qualitative evaluation of events, phenomena and objects. Thus, the assessment of the profile categories is qualitative and represented through the language variables which justifies the use of fuzzy logic method applied to process the questionnaire results and then to divide students into groups. The group classification helps to unify and standardize the study plan. In order to minimize the student profile subjectivity, the assessment should be carried out by a group of experts formed from the teaching staff. Figure
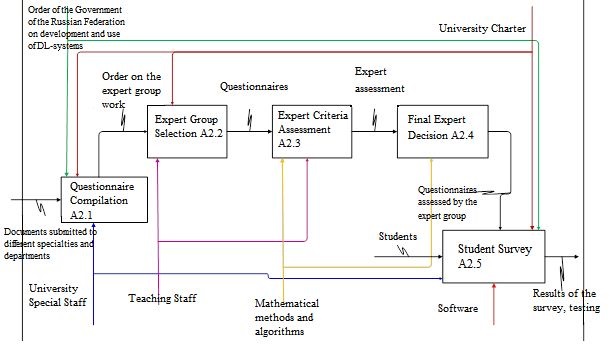
As the profile categories analysis is carried out by several experts the differences in assessment are unavoidable. The extent to which these assessments differ is significant. A group assessment can be considered reliable only if there is unanimity between experts.
For profile categories evaluation Bof’s method is used. This method allows to combine both quantitative and qualitative index to receive a generalized index using the expert point-evaluation, initial index ranking, standardizing and coding. The method can be used to evaluate any set of objects according to a set of indexes (criteria).
Each assessment criterion and each expert weight index should be ranked.
Suppose the expert rating vector is represented by where is the number of experts and N is the number of criteria. And is the k-expert rating to i-criterion. is a generalized group rating. Suppose the relative expert rating is determined by the weight vector with where and . So we get the following group distance function based on OWA aggregation operator: , where , . [18, 19]
The main feature of OWA-operator is that it determines the weight index according to the input values and highlights the highest, lowest and average level of particular differences (distances). To reach an optimal decision we assume that D index does not exceed the specified threshold. Next through Kendall concordance coefficient we specify this threshold, that is, we assume that the experts have unanimous opinion. Thus, the generalized expert rating formula is: , with where is a number of experts, is Kendall concordance coefficient.
The optimization task is solved by the Lagrange factors method. Thus, the function is where is the additional task data.
Thus, the suggested method minimizes the difference between the expert assessment and the generalized average rating when assessing student profile categories.
Research Methods
The classification based on the fuzzy decision tree method (Fedrizzi & Molinari, 2013) helps to determine class, feature or attribute membership and also the degree of membership. A fuzzy decision tree also indicates if the object has several features that are characteristic of more than one attribute. The main idea of this approach is to combine decision tree and rough logic methods.
A distinctive feature of the decision tree is that each example definitely belongs to a particular node. However, this is not the case with fuzzy logic. It is necessary to specify some of the linguistic values for each attribute and to determine the degree of membership of a particular example. Instead of the number of particular node examples the fuzzy decision tree groups their degree of membership. The coefficient is the ratio of the N node examples to i target value and is calculated by the formula:
where μN(Dj) is the Dj example membership degree to N node, μi(Dj) is the example membership degree to i target value, SN is the set of all N node examples. Then we calculate the coefficient
that shows the general characteristic of the N node examples. The standard decision tree algorithm calculates the ratio of the number of examples belonging to a particular attribute to the total number of examples. In case of the fuzzy decision tree PNi/PN ratio is used, which is calculated using the membership degree value. The formula
assesses the average amount of information to determine the class of an object from PN set.
In the next stage of the fuzzy decision tree the algorithm calculates the entropy to classify objects by A attribute with aj value:
where N|j node is a branch of N node.
The algorithm selects Ax attribute with the highest information growth: G(SN,A):
The N node is divided into several N|j subnodes. The membership degree of the N|j node Dk example is calculated step by step using N node value by the formula:
where μi(Dk,aj) shows Dk membership degree to aj attribute. N|j subnode is deleted if all its examples have a zero membership degree. The algorithm is repeated until all examples of the node are classified or all attributes are used.
The target class membership of the new record is calculated by the formula:
where Plk is the coefficient of the ratio of the l tree leaf examples to the k target class value, μl(Dj) is the node l membership degree of the example, χk is the membership degree of the k target class value to the positive value of the classification result.
Findings
A numerical experiment was conducted in a survey form. 100 students were given questionnaires consisting of 30 questions with 3 sections on determining the student’s psychological aspect, the level of knowledge and the representational system. The experiment was judged by 3 experts.
Questionnaires of 60 students were included in the training sample, 40 questionnaires made up a test sample. Each question in the questionnaire has the options “I know well enough”, “I don't know well enough”, “I don't know or I know poorly.”
As a result, several student clusters were formed which can be divided into the following groups:
1. Students with a good level of knowledge and a discrete representational system, able to acquire information represented in any form.
2. Students with a good level of knowledge, able to acquire the audio information only.
3. Students with an average and below average level of knowledge, able to learn through visual images.
4. Students with poor academic performance who are slow in acquiring information in any form.
5. Students with a high level of knowledge, able to acquire information represented in any form.
The results of the experiment showed that according to the sample suggested, the largest amount of information was gained through the questions on the student’s knowledge level. The experts managed to reach an agreement in their assessment. The Lagrange value obtained was 1.184 that is considered minimal in our case.
Conclusion
The article proposes a special decision making support approach in the distance education management. It is based on the student profile data and student’s individual characteristics, such as mental fitness, level of knowledge and the representational system. The article analyses the specifics of distance education, its pros and cons, identifies the existing problems. A special approach that takes into account student’s individual characteristics is proposed to help to determine the profile of a student. The approach determines the information, functional and structural aspects of the distance education system and proposes the system requirements. The student profile is based on the student’s individual characteristics, i.e. psychological and technical fitness, representational system, and level of academic knowledge. A proposed approach helps to classify distance education recipients using the fuzzy logic method and teacher-aided smart computer technologies. The fuzzy logic and the collective expert opinion method help to minimize the subjective influence when assessing the student profile.
References
- Baranova, I. (2018). Perspectives of development of the educational services market in regions of Russia in the conditions of the knowledge economy formation. The Impact of information on modern humans, 114-120. https://doi.org/10.1007/978-3-319-75383-6_15
- Courtney, M., & Wilhoite-Mathews, S. (2015). From distance education to online learning: Practical approaches to information literacy instruction and collaborative learning in online environments. Journal of library & information services in distance learning, 9(1-2), 261-277. https://doi.org/10.1080/01930826.2015.1038924
- Ekren, G., Karatas, S., & Demiray, U. (2015). Understanding of leadership in distance education management. Identification, evaluation, and perceptions of distance education experts. https://doi.org/10.4018/978-1-4666-8119-4.ch004
- Fedrizzi, M., & Molinari, A. (2013). A multi-expert fuzzy TOPSIS-based model for the evaluation of e-learning paths. Proceedings of the 8th conference of the European society for fuzzy logic and technology. https://doi.org/10.2991/eusflat.2013.84
- Gerasimova, V., Melamud, M., Tutaeva, D., Romanova, Y., & Zhenova, N. (2018). The adoption of e-learning technology at the faculty of distance learning of Plekhanov Russian University of Economics. Journal of social studies education research, 9(2), 172-188.
- Kamakoty, J., Sohani, N., & Sohani, N. (2015). Determinants of service quality in education: Service provider's perspective and academician's perspective. International journal of services and operations management, 20(2), 141-164. https://doi.org/10.1504/IJSOM.2015.067474
- Labus, A., Simic, K., Barac, D., Despotovic-Zrakic, M., & Radenkovic, M. (2012). Integration of social network services in e-education process. Metalurgia international, 17(7), 161-169.
- Leontev, M. (2016). Innovative approaches in distance education in the field of environmental management and environmental technologies. XV international conference “Topical problems of architecture, civil engineering, energy efficiency and ecology-2016, 73. Management, organization and planning of construction works and municipal facilities. https://doi.org/10.1051/matecconf/20167307005
- Ling, M. (2019). Research on distance education image correction based on digital image processing technology. EURASIP journal on image and video processing. https://doi.org/10.1186/s13640-019-0416-9
- Liu, H., & Yen, J. (2014). Effects of distance learning on learning effectiveness. Eurasia journal of mathematics, science and technology education, 10(6), 575-580. https://doi.org/10.12973/eurasia.2014.1218a
- Mudrakova, O., & Bindyukova, T. (2015). Problema ispolzovaniya tekhnologij distantsionnogo obucheniya v shkolnom obrazovanii [The problem of using distance learning technologies in school education]. Integratsiya obrazovaniya, 19, 3(80), 29-35.
- Ozcan, D., & Zeynep, G. (2016). Pedagogical formation education via distance education. Eurasia journal of mathematics, science and technology education, 12(2), 347-360. https://doi:10.12973/eurasia.1205a
- Reikhanova, I., & Bukina, Y. (2017). Optimization of educational services management in Russia for provision of succession of all levels of education with the help of new information and communication technologies. Advances in intelligent systems and computing, 726, 957-963. https://doi.org/10.1007/978-3-319-90835-9_107
- Solomon, K. (2016). The social system approach to institutions: examples from western economic history. Journal of institutional studies, 8(3), 6-34. https://doi:10.17835/2076-6297.2016.8.3.006-034
- Startseva, O. G., & Bogdanova, D. R. (2015). Podhod k podderzhke prinyatiya reshenij pri upravlenii sistemoj distantsionnogo obrazovaniya s uchetom individualnykh kharakteristik obuchayushchikhsya [The decision making support approach in the distance education management based on student’s individual characteristics]. Intellektualnye tekhnologii obrabotki informatsii i upravleniya, 1, 88-92.
- Startseva, O. G., Poptsova, A. I., & Bogdanova, D. R. (2015). Podhod k formirovaniyu profilya studenta dlya personifikacii processa distancionnogo obucheniya [The student profile compilation approach to personalize the distance education process]. Informacionnye tekhnologii intellektual'noj podderzhki prinyatiya reshenij, 2, 211-218.
- Swart, A. (2015). Student usage of a learning management system at an open distance learning institute: A case study in electrical engineering. International journal of electrical engineering & education, 52(2), 142-154. https://doi:10.1177/0020720915575925
- Tsionas, E., Assaf, A. G., Gillen, D., & Mattila, A. S. (2017). Modeling technical and service efficiency. Transportation research. Part B: Methodological, 96, 113-125. https://doi.org/10.1016/j.trb.2016.11.010
Copyright information
This work is licensed under a Creative Commons Attribution-NonCommercial-NoDerivatives 4.0 International License.
About this article
Publication Date
15 November 2020
Article Doi
eBook ISBN
978-1-80296-092-1
Publisher
European Publisher
Volume
93
Print ISBN (optional)
-
Edition Number
1st Edition
Pages
1-1195
Subjects
Teacher, teacher training, teaching skills, teaching techniques, special education, children with special needs, computer-aided learning (CAL)
Cite this article as:
Startseva, O., Bogdanova, D., Netsvetaeva, K., & Kotelnikov, V. (2020). Decision Making Support Approach In The Distance Learning Educational Service Management. In I. Murzina (Ed.), Humanistic Practice in Education in a Postmodern Age, vol 93. European Proceedings of Social and Behavioural Sciences (pp. 875-885). European Publisher. https://doi.org/10.15405/epsbs.2020.11.91