Abstract
The paper considers such problems faced by the modern healthcare system as population ageing, aggravation of the problem of chronic diseases, as well as postoperative and follow-up care for elderly patients. In order to solve these problems, it is proposed to use wearable and implantable medical devices referring to the category of the Internet of Things. The area of vulnerability of this technology is the stage of transferring medical data to the cloud service for further analysis. It is proposed to encrypt data before sending it to the cloud service, as well as conduct continuous biometric identification of patients.The population of developed countries is gradually ageing, thus the problem of chronic diseases is aggravated. Medical care for the elderly people is an onerous burden on the budget. The success of postoperative and follow-up treatment largely depends on timely diagnosis of patients' medical parameters. One of the ways to improve the situation is to implement health monitoring programs.
Keywords: Internet of Thingssocietytelemedicine
Introduction
Wearable and implantable medical devices which refer to the Internet of things are used to implement measures improving the situation with ageing population. This kind of devices record physiological parameters of patients and send them to special “cloud” services for the attending doctor to analyze. Medical parameters of patients in western countries are used by pharmacists to make out prescriptions and by insurance companies to make recommendations for further treatment. Nowadays new medical technologies have some vulnerabilities, that could allow hackers to perform various fraudulent schemes. One of the ways to protect data is to encrypt medical parameters before sending them to the cloud, as well as conduct continuous biometric identification of patients. Implantable medical modules allow you to provide assistance to patients with severe ailments. Advances in technologies of remote monitoring and control of these devices require development of new telecommunication protocols.
Problem Statement
Medicine is an extremely important institution of human society. Achievements of public healthcare system allow increasing life expectancy and significantly improving the quality of life of the population. But modern medicine faces serious challenges. One such problem is population ageing. With age, patients get more diseases, including chronic ones. The problem of postoperative and follow-up treatment becomes relevant (Aloui, Nait-Ali, & Naceur, 2018; Altop, Seymen, & Levi, 2019; Bhurane, Sharma, San-Tan, & Acharya, 2019; Chukwunonyerem et al., 2016; Koya & Deepthi, 2018).
The authors note that the average life expectancy of men in the European Union, in particular in France, is close to 80 years, and the average life expectancy of women exceeds 85 years. The period from 80 to 85 years is of particular control by the authorities, because this period is associated with the manifestation of chronic diseases, weakness and loss of self-sufficiency. The increase in the number of people of such age in France poses certain problems for the national system of healthcare. In 1997, people of 60 years old and older made 20% of the total population, consuming almost a third of all medical expenses and almost half of the medical costs (Lozoya-Santos, Sepúlveda-Arróniz, Tudon-Martinez, & Ramirez-Mendoza, 2019, p. 180). In France, average medical expenses per person are 1,800 euros per year, while for people older than 65 it is 3,000 euros per year (Kang, Jo, & Kim, 2018, p. 95).
One of the ways to solve this problem is application of telemedicine technologies.
Research Questions
According to Russian law, telemedicine technologies are “a set of organizational, technical and other measures taken in the process of provision of medical aid to a patient using procedures, means and methods of transferring data through communication channels (lines) providing reliable identification of participants of the information exchange process - a doctor (medical worker) and a patient (or his legal representative)” (Aloui et al., 2018, p. 39).
Telemedicine is used for medical staff remote interaction: to make decisions on the issues of prevention, diagnosis,etc., to hold councils of physicians and make other medical decisions, as well as for doctor - patient communication: for consultation, health status monitoring and assessing the need for doctor's appointment (Lozoya-Santos et al., 2019).
In accordance with the law on telemedicine, the institution does not require any special license to take part in the council of physicians by means of telemedicine. Medical organization may conduct such consultations on the types of medical assistance for which the organization has valid license. To hold such consultations the clinic should have appropriately equipped room. However, it is not all that simple. The main condition for this is registration of the organization in the Federal Register of Healthcare Organizations of the Unified State Information Healthcare System - USIHS (EGISZ). It should also be noted that consultations can be carried out (and requested, respectively) only by employees included in the Federal Register of Healthcare Workers (Arteaga-Falconi, Al Osman, & El Saddik, 2018; Hu, Yang, Chen, Qian, & Rodrigues, 2018; Michael, 2018; Michel-Macarty, Murillo-Escobar, López-Gutiérrez, Cruz-Hernández, & Cardoza-Avendaño, 2018; Wang et al., 2018).
Telemedicine optimizes the time of specialists, increases the availability of medical services and allows introducing new methods of treatment (Bhurane et al., 2019, p. 142; Koya & Deepthi, 2018). Let us consider several examples of telemedicine technologies application.
Purpose of the Study
Current study aims to discuss the situation in the telemedicine field, to consider main development trends in the field of remote health monitoring technologies.
Research Methods
The number of Europeans, who suffer from chronic heart failure exceeds 15 million people, mainly elderly (Lozoya-Santos et al., 2019, p. 184). The development of the disease is characterized by a large number of cardiac collapse cases, providing increase of repeated hospitalizations as well as of considerable medical costs. Since 2007, a study of educational telemedicine has been conducted in Normandy on hospitalized with acute heart failure patients over 65(LVEF <45%). In a year the number of days such patients spent in hospitals was measured. The study included 73 patients: 35 randomly selected patients receiving traditional medical care and 38 patients receiving telemedicine services. By the end of this study, they recorded 1040 days of hospitalization due to the acute heart failure, of which 450 days were spent in hospitals by patients receiving telemedicine services, compared with 590 days spent by people in the control group. On the average, the patients were aged 76.8 (Altop, Seymen, & Levi, 2019, p. 112). When researchers compared the results with those of the control group, they noted decrease in morbidity rate and mortality in patients using telemedicine services (Altop, Seymen, & Levi, 2019; Kang et al., 2018).
Another study (Lozoya-Santos et al., 2019) examined possibility to use telemonitoring at home as possible alternative to hospitalization. Patients measured their weight, blood pressure and carried out electrocardiograms. The treatment could be adapted in real-time mode, as the communication between the patient and his/her cardiologist in the process of telemonitoring was direct. Telemonitoring was not used as an emergency alert system, but was aimed at the constant adaptation of treatment in accordance with the results of measured parameters. 29 women and 54 men took part in the experiment (Yildirim, 2018, p. 192). The patients’ age was on average 78. 4.4% of them died and 5% got re-hospitalized during the first 30 days after discharge from the hospital. 6 months later no difference was found in the time or duration of hospitalization (p = 0.28), though the mortality level decreased significantly (p <0.003).
An E-care telemonitoring project has been developed at Strasbourg University Hospital in order to streamline monitoring of patients suffering from cordiac collapse from home. An experiment, involving 175 patients, was conducted from February 2014 to April 2015, within the framework of the project. During the experiment, patients and healthcare experts used the E-care platform on a daily basis in accordance with the protocol developed for each patient. The E-care platform responds to the deterioration of patient’s health. Patients used non-intrusive sensors that recorded their blood pressure, their heart rate, the oxygen saturation and weight. Such sensorstransfer physiological data via Bluetooth in real time. The platform includes touch-screen tablet that connects through Wi-Fi access to a router or 3G/ 4G mobile network, which allows cardiologist to interact with his patient, to prescribe needed treatment, recommended diet and lifestyle. This system involves server storing patient’s data and virus-secure Internet portal to which both the patient and various medical workers working in and out of hospitals can get access. The patients participated in the experiment were aged 72, on average, and the gender ratio was 0.7. All participants suffered from multiple co-mobidities, their Charlson index was, on average, 4.1. 60 % of tested patients suffered from heart failure, 40 % - from anemia. A trial fibrillation was detected in more than 30% of patients. Other 30% of patients suffered from diabetes (type II), the last 30% - from chronic obstructive pulmonary disease. The researchers made 1,500 measurements, and then the (E-care) system generated 700 warnings for 68 participants of the experiment (Lozoya-Santos et al., 2019). The analysis of the system warnings showed that it automatically detects any deterioration in the health of the patient, especially in cases of cardiac decompensation. It has also demonstrated that it is able to detect any deterioration in the state of patients with numerous concomitant diseases with high accuracy (Deng, Wang, Tang, & Zheng, 2018; Ehatisham-ul-Haq, Azam, Naeem, Amin, & Loo, 2018; Ellouze, Rekhis, Boudriga, & Allouche, 2018; Moosavi, Nigussie, Levorato, Virtanen, & Isoaho, 2018). Extracting cardiac dynamics within ECG signal for human identification and cardiovascular diseases classification. Neural Networks, 100, 70-83.2018 (Dodangeh & Jahangir, 2018; Nellyzeth, Roberto, & Marco, 2018; Peris-Lopez, González-Manzano, Camara, & de Fuentes, 2018; Wazid, Das, & Vasilakos, 2018; Yildirim, 2018).
There are many people in the world suffering from one or more chronic diseases. Long-term treatment of chronic diseases is an onerous burden on patients, society and healthcare system as a whole. Improvements in the quality and life expectancy of such patients can be achieved by means of timely medical care and introduction of self-organization programs, when patients are involved in the process of managing their own health (Altop, Seymen, & Levi, 2019; Chukwunonyerem et al., 2016). Recent developments in the field of mobile technologies can help to create inexpensive, adaptive and easily accessible medical applications for self-use; moreover, in 6 out of 9 cases statistically significant improvements in the health status of patients using online diagnostic tools are recorded (Kang et al., 2018, p. 96). Most often, such technologies are used in the treatment of diabetes, then in the treatment of chronic pulmonary diseases, and thirdly, of cardiovascular diseases (Kang et al., 2018, p. 97).
Medical technologies based on machine learning and artificial neural networks play a very important role in adaptive treatment. These technologies allow automating the process of medical data processing and making treatment more personalized (Sun, Lo, & Lo, 2019, p. 242). An essential prerequisite for successful application of this technology is provision of the medical data safety during their transferring via communication channels (Pirbhulal et al., 2018, p. 550).
Remote patient health monitoring systems, based on the transmission of data via wireless networks, can be successfully used in various military and special operations. Moreover, they are planned to be used within the framework of implementing the concept of a smart house.
The chances of successful rehabilitation in patients who suffered a stroke are significantly increased by timely provision of medical data to the attending physician via teleconference.
Wireless capsule endoscopy uses high-resolution video cameras and wireless technologies, which allows performing real-time monitoring of important organs.
To provide successful treatment, diagnostics and improve the quality of life, patients are also proposed to use wearable or implantable medical devices (Implantable medical devices, IMD / wearable medical devices, WMD). Remote use of such devices for solution of various health problems provides more effective way of chronic and common diseases treatment.
The terms “wearable computing” or “body-area / body-sensor networks” were introduced approximately in 1995. They are used to denote wireless network of small computers including sensors and actuators, which are located in, on, or beside the patient’s body. This idea was well received by medical monitoring experts who understood the prospects of using physiological sensors to obtain real time patient information, as well as the prospects of using remotely controlled implantable medical devices.
Internet of Things devices allow to quickly organize and share information via the Internet and to provide new opportunities for medical devices, equipped with wireless communication, to monitor and manage data. Along with collecting medical data, this technology allows performing biometric identification of patients based on their physiological and behavioral patterns. Medical Internet of Things technologies allow, on the one hand, generating the flow of medical data, and, on the other hand, performing continuous biometric identification of patients (Altop, Seymen, & Levi, 2019; Arteaga-Falconi et al., 2018; Berkaya et al., 2018; Bhurane et al., 2019; Challa et al., 2018; Chukwunonyerem et al., 2016; Deng et al., 2018; Dodangeh, & Jahangir, 2018; Ehatisham-ul-Haq et al., 2018; Ellouze et al., 2018; Hu et al., 2018; Kang et al., 2018; Koya & Deepthi, 2018; Krishnan, Lokesh, & Devi, 2019; Kumar, Singhal, Saini, Roy, & Dogra, 2018; Lozoya-Santos et al., 2019; Michael, 2018; Michel-Macarty, Murillo-Escobar, López-Gutiérrez, Cruz-Hernández, & Cardoza-Avendaño, 2018; Modak & Jha, 2019; Moosavi et al., 2018; Nellyzeth, Roberto, Marco, & Conrado, 2018; Peris-Lopez et al., 2018; Pirbhulal et al., 2018; Pirbhulal et al., 2019; Wang et al., 2018; Wazid, Das, & Vasilakos, 2018, Yildirim, 2018).
The possibility of continuous biometric identification of a person can be useful in cases when a person performs a demanding job, for example, an atomic power station operator or an air traffic controller.
The IoMTs (Internet of Medical Things) is a set of different intellectual nodes that are able to interact and work in mutual cooperation to collect information without human assistance. IoMT can be a part of such medical devices as magnetic resonance imaging scanners, ultrasound imaging units; it might also be represented by wearable devices able to record patients' medical parameters and diagnose diseases.
The IoMT structure includes three layers: data registration, network and application. The layer of data registration is responsible for collecting medical information by means of certain devices. The network layer provides transmission of data collected by the data registration layer. The application layer is responsible for making decisions in the field of medical diagnostics. Data collected by the Internet of Medical Things is available both to pharmacists and insurance companies (Yin et al., 2019, p. 3077).
Since the Internet of Medical Things uses wireless media to exchange data between devices, there exists a risk of unauthorized access with the data transfer process.
Hacker attacks can lead to the following negative consequences:
- Hackers can impose unnecessary expensive medical services on a patient.
- Hackers can, on behalf of the patient, order some expensive drugs, prescribed to the patient, for subsequent sale on the black market.
- Hackers can create a fictitious clinic and on its behalf issue and invoice to the insurance company for provision of non-existent medical services.
It is necessary to improve information protection mechanisms, as criminals can use different ways for source data compromise. One of such approaches is as follows. Medical device, for example, designed to record electrocardiograms, collects data. Then encryption of obtained results is carried out. It is advisable to achieve some compromise between cryptographic strength, energy efficiency and system performance. Several encryption options are available. One of them involves application of elliptic curves. The second algorithm allows generating biometric keys obtained on the basis of electrocardiograms. The stream of encrypted data via BlueTooth is transmitted to the smartphone, then it is sent to the cloud server for further decryption and analysis. Figure
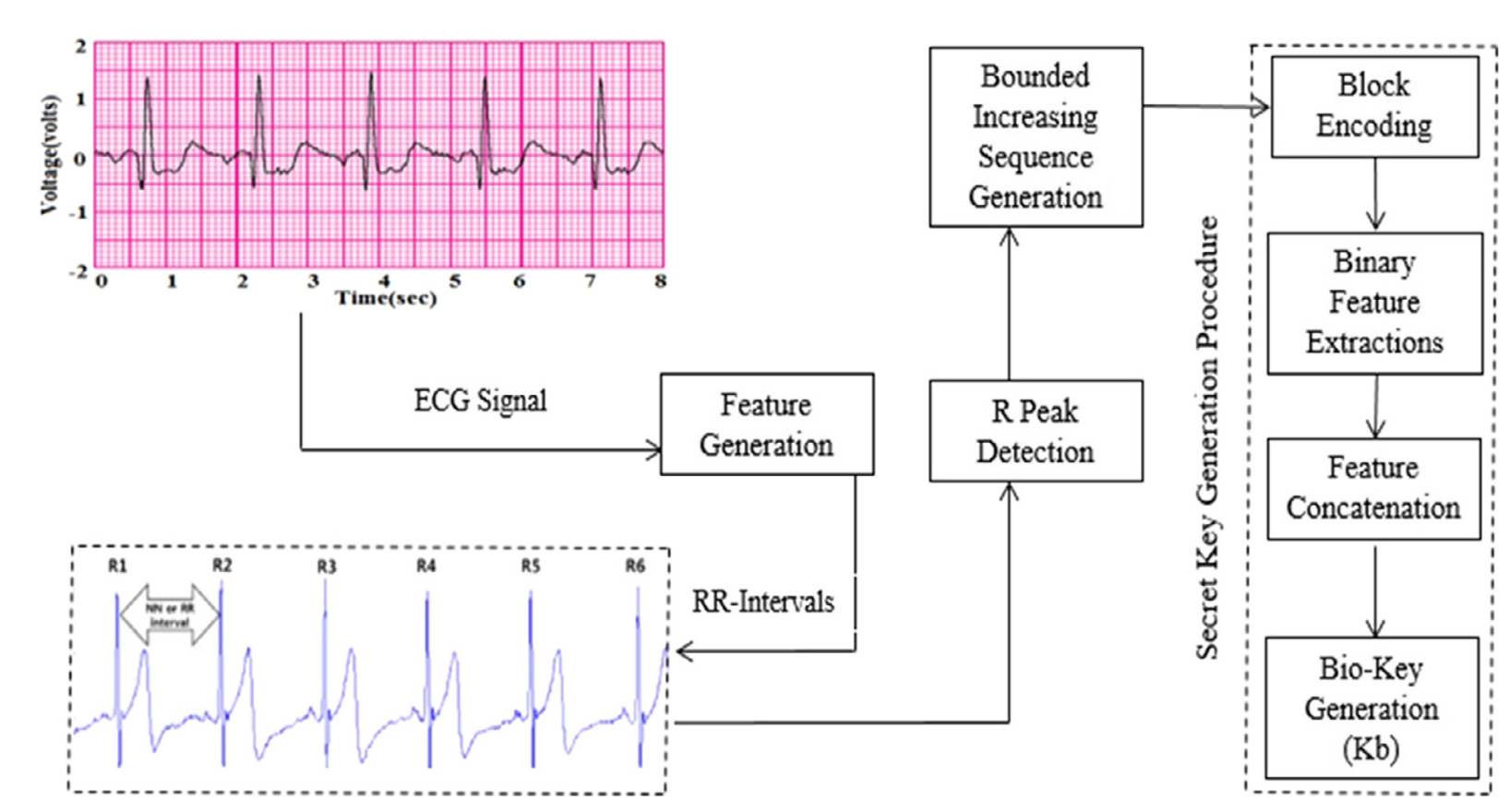
Paper (Krishnan et al., 2019) considers mechanism for generating random 128-bit keys based on cardiocycles intervals.
As we have already mentioned above, there are implantable devices in addition to the wearable Internet of things. These include various cardiostimulators, neuroimplants, etc. Life and health of patients depend on normal operation of such devices. Neurostimulators allow, for example, preventing epilepsy attacks. Despite the extreme importance of implantable devices, serious threats are being gradually detected (Challa et al., 2018, p. 535).
Threat 1. Nowadays, implantable devices are serviced by the doctor who installed them. It is performed by means of the BlueTooth protocol. In course of time this procedure can be carried out remotely via Wi-Fi and hackers attacks can lead to rapid death of the patient. Therefore, reliable protection is required, for example, with biometric assignment to the doctor. However, if the doctor quits, all his patients will be at great risk (Challa et al., 2018, p. 537).
Threat 2. If the patient gets into accident and is helpless, emergency doctors will need to have access to the implanted devices by themselves, without assistance of the attending physician, bypassing the existing safety protocol. In such case using special vibration signal is proposed (Sun, Lo, & Lo, 2019; Yin et al., 2018; Yin et al., 2019).
Findings
The population of developed countries is gradually ageing. The problem of chronic diseases is aggravated with age. Medical care for the elderly people becomes an onerous burden on the state. The success of postoperative and follow-up treatment largely depends on timely diagnosis of patients' medical parameters. One of the ways to improve the situation is to implement health monitoring programs. To implement such measures, wearable and implantable medical devices referring to the IoMT type are used. This type of devices records physiological parameters of patients and sends them to the “cloud” services for the attending doctor to analyze. Medical parameters of patients in western countries are used by pharmacists to make out prescriptions and by representatives of insurance companies to make recommendations for further treatment. Nowadays new medical technologies have some vulnerabilities, that could allow hackers to perform various fraudulent schemes.
Conclusion
One of the ways to protect data is to encrypt medical parameters before sending them to the cloud, as well as to conduct continuous biometric identification of patients. Implantable medical modules allow you to provide assistance to patients with severe ailments. Advances in technologies of remote monitoring and control of these devices require development of new telecommunication protocols.
Acknowledgments
The study was held with the financial support of RFBR (the research project № 19-07-00780).
References
- Aloui, K., Nait-Ali, A., & Naceur, M. S. (2018). Using brain prints as new biometric feature for human recognition. Pattern Recognition Letters, 113, 38-45.
- Altop, D. K., Seymen, B., & Levi, A. (2019). SKA-PS: Secure key agreement protocol using physiological signals. Ad Hoc Networks, 83, 111-124.
- Arteaga-Falconi, J. S., Al Osman, H., & El Saddik, A. (2018). ECG and fingerprint bimodal authentication. Sustainable cities and society, 40, 274-283.
- Berkaya, S. K., Uysal, A. K., Gunal, E. S., Ergin, S., Gunal, S., & Gulmezoglu, M. B. (2018). A survey on ECG analysis. Biomedical Signal Processing and Control, 43, 216-235.
- Bhurane, A. A., Sharma, M., San-Tan, R., & Acharya, U. R. (2019). An efficient detection of congestive heart failure using frequency localized filter banks for the diagnosis with ECG signals. Cognitive Systems Research, 55, 82-94.
- Challa, S., Das, A. K., Odelu, V., Kumar, N., Kumari, S., Khan, M. K…, & Vasilakos, A. V. (2018). An efficient ECC-based provably secure three-factor user authentication and key agreement protocol for wireless healthcare sensor networks. Computers & Electrical Engineering, 69, 534-554.
- Chukwunonyerem, J. A. M., Aibinu, A. J., Onumanyi, O. C., Ugweje, E. N., Onwuka, C., & Alenogbena, N. E. (2016). Development of key generation algorithm using ECG biometrics for node security in wireless body area sensor network. European Research in Telemedicine. La Recherche Européenne en Télémédecine 5, 137-144.
- Deng, M., Wang, C., Tang, M., & Zheng, T. (2018). Extracting cardiac dynamics within ECG signal for human identification and cardiovascular diseases classification. Neural Networks, 100, 70-83.
- Dodangeh, P., & Jahangir, A. H. (2018). A biometric security scheme for wireless body area networks. Journal of Information Security and Applications, 41, 62-74.
- Ehatisham-ul-Haq, M., Azam, M. A., Naeem, U., Amin, Y., & Loo, J. (2018). Continuous authentication of smartphone users based on activity pattern recognition using passive mobile sensing. Journal of Network and Computer Applications, 109, 24-35.
- Ellouze, N., Rekhis, S., Boudriga, N., & Allouche, M. (2018). Powerless security for cardiac implantable medical devices: Use of wireless identification and sensing platform. Journal of Network and Computer Applications, 107, 1-21.
- Hu, L., Yang, J., Chen, M., Qian, Y., & Rodrigues, J. J. (2018). SCAI-SVSC: Smart clothing for effective interaction with a sustainable vital sign collection. Future Generation Computer Systems, 86, 329-338.
- Kang, J. H., Jo, Y. C., & Kim, S. P. (2018). Electroencephalographic feature evaluation for improving personal authentication performance. Neurocomputing, 287, 93-101.
- Koya, A. M., & Deepthi, P. P. (2018). Anonymous hybrid mutual authentication and key agreement scheme for wireless body area network. Computer Networks, 140, 138-151.
- Krishnan, S., Lokesh, S., & Devi, M. R. (2019). An efficient Elman neural network classifier with cloud supported internet of things structure for health monitoring system. Computer Networks, 151, 201-210.
- Kumar, P., Singhal, A., Saini, R., Roy, P. P., & Dogra, D. P. (2018). A pervasive electroencephalography-based person authentication system for cloud environment. Displays, 55, 64-70.
- Lozoya-Santos, J. D. J., Sepúlveda-Arróniz, V., Tudon-Martinez, J. C., & Ramirez-Mendoza, R. A. (2019). Survey on biometry for cognitive automotive systems. Cognitive Systems Research, 55, 175-191.
- Michael, H. M. (2018). Postoperative Remote Automated Monitoring: Need for and State of the Science. Canadian Journal of Cardiology, 34, 850–862.
- Michel-Macarty, J. A., Murillo-Escobar, M. A., López-Gutiérrez, R. M., Cruz-Hernández, C., & Cardoza-Avendaño, L. (2018). Multiuser communication scheme based on binary phase-shift keying and chaos for telemedicine. Computer Methods and Programs in Biomedicine, 162, 165–175.
- Modak, S. K. S., & Jha, V. K. (2019). Multibiometric fusion strategy and its applications: A review. Information Fusion, 49, 174-204.
- Moosavi, S. R., Nigussie, E., Levorato, M., Virtanen, S., & Isoaho, J. (2018). Performance analysis of end-to-end security schemes in healthcare IoT. Procedia computer science, 130, 432-439.
- Nellyzeth, F., Roberto, L. A., & Marco, A. R. (2018). Conrado, G. Readily available ECG databases. Journal of Electrocardiology, 51, 1095–1097.
- Peris-Lopez, P., González-Manzano, L., Camara, C., & de Fuentes, J. M. (2018). Effect of attacker characterization in ECG-based continuous authentication mechanisms for Internet of Things. Future Generation Computer Systems, 81, 67-77.
- Pirbhulal, S., Samuel, O. W., Wu, W., Sangaiah, A. K., & Li, G. (2019). A joint resource-aware and medical data security framework for wearable healthcare systems. Future Generation Computer Systems, 95, 382-391.
- Pirbhulal, S., Shang, P., Wu, W., Sangaiah, A. K., Samuel, O. W., & Li, G. (2018). Fuzzy vault-based biometric security method for tele-health monitoring systems. Computers & Electrical Engineering, 71, 546-557.
- Sun, Y., Lo, F. P. W., & Lo, B. (2019). EEG-based user identification system using 1D-convolutional long short-term memory neural networks. Expert Systems with Applications, 125, 259-267.
- Wang, J., Han, K., Alexandridis, A., Zilic, Z., Pang, Y., Wu, W., ... & Jeon, G. (2018). A novel security scheme for body area networks compatible with smart vehicles. Computer Networks, 143, 74-81.
- Wazid, M., Das, A. K., & Vasilakos, A. V. (2018). Authenticated key management protocol for cloud-assisted body area sensor networks. Journal of Network and Computer Applications, 123, 112-126.
- Yildirim, Ö. (2018). A novel wavelet sequence based on deep bidirectional LSTM network model for ECG signal classification. Computers in biology and medicine, 96, 189-202.
- Yin, W., Yang, X., Li, L., Zhang, L., Kitsuwan, N., & Oki, E. (2018). Hear: Approach for heartbeat monitoring with body movement compensation by ir-uwb radar. Sensors, 18(9), 3077. https://doi.org/10.3390/s18093077
- Yin, W., Yang, X., Li, L., Zhang, L., Kitsuwan, N., Shinkuma, R., & Oki, E. (2019). Self-adjustable domain adaptation in personalized ECG monitoring integrated with IR-UWB radar. Biomedical Signal Processing and Control, 47, 75-87.
Copyright information
This work is licensed under a Creative Commons Attribution-NonCommercial-NoDerivatives 4.0 International License.
About this article
Publication Date
15 November 2020
Article Doi
eBook ISBN
978-1-80296-092-1
Publisher
European Publisher
Volume
93
Print ISBN (optional)
-
Edition Number
1st Edition
Pages
1-1195
Subjects
Teacher, teacher training, teaching skills, teaching techniques, special education, children with special needs, computer-aided learning (CAL)
Cite this article as:
Bogdanov, M., Gorbunov, V., Zabikhullin, F., & Titova, L. (2020). Modern Medicine: Issues And Prospects. In I. Murzina (Ed.), Humanistic Practice in Education in a Postmodern Age, vol 93. European Proceedings of Social and Behavioural Sciences (pp. 212-220). European Publisher. https://doi.org/10.15405/epsbs.2020.11.23