Abstract
The development of the online space into a sphere where sociopolitical practices can develop resulted in the accumulation of massive linguistic data—Big Data—thus, traditional methods of discourse analysis and text analysis are irrelevant. To analyze this data, the development of innovative approaches and methods of research, one of which is network analysis, is highly warranted. Not only does network analysis allow us to identify and analyze quantitative markers (like in traditional content analysis), but also to conduct efficient qualitative research into the bulk of linguistic data (posts, commentaries, subscripts to video and pictures), which comprises the area of overlapping online discursive fields. Besides, innovative hybrid methodology enables analysis of linguistic and extralinguistic context of a particular network discourse and makes it possible to retrieve connotations, to conduct sentiment analysis, to cluster linguistic data based on semantic similarity, to estimate and predict the development of the pragmatic potential of a given discourse regarding its potential for the sociopolitical action both in the online and the offline spheres. Network approach allowed the authors of the article to provide a description of the linguistic model of the sociopolitical communication exemplified with Twitter and characterize the linguistic pattern of communication and the discursive field as the constituent parts of this model.
Keywords: Network discourseBigDatanetwork analysisonline-spacediscursive fieldssocial graph
Introduction
The network society is facing a progressive digitalization of all the spheres of human interaction. The current trend of gradual integration of the online space and the offline space causes principally new character of interactions within the sociopolitical space. Communication is inherently a system of multimodal, multicultural and multilingual discursive interactions. Being the platform for these interactions and the medium to obtain and spread information, the Internet becomes the principal communication technology. Thus, the role of ordinary Internet and social media users has changed. Their capacity to generate valuable sociopolitical content and network communicative interactions, i.e. online discourse fields various in scale, has increased. Therefore, there increased their ability to generate triggers whose consumption can affect users’ decisions and induce the sociopolitical action in the offline space ( Masías, Hecking, & Hoppe, 2018).
Discursive fields operate within multiple social networks having a different degree of transparency, which determines an implicit formation and emergence of linguistic patterns of social action. Thus, the development of innovative methodology and research toolset is highly warranted. It will allow us to monitor the formation and the development of network discourses in the online space, to predict constructive and destructive vectors of their development and manage these processes.
In the framework of the network society, the online space continues to transform media and the character of communication, information sharing and social interactions. As of 2019, there were an estimated 4,479 million active internet users and 3,725 million active social media users worldwide. This accounted for 58% of the global population ( Clement, 2019). Due to the boost in the development of social networks and the possibility of any user to create content, social networks are currently viewed as ‘new media’ or ‘social media’. They have a unique interface and operate via a particular set of technologies which make them a preferred means of communication. Social media operate using an ‘equal participation’ principle, which means that the process of communication is equally maintained by all the participants and the social media platform itself ( Pleshakova, 2017).
Twitter is one of the most popular services of sharing instant messages worldwide ( Kallas, 2019). As of 2019, the number of active users in the USA, Japan and Russia accounted for 48.5, 35.69, 13.9 million people correspondingly. Notably, Twitter is a popular social media platform used by the most famous and influential people in the world. The peculiarity of Twitter is the format of personalized messages—tweets—which makes it possible to have an online dialogue with online users as if it was a chat of likeminded people.
Problem Statement
Operation of online discursive fields results in a colossal amount of network linguistic data whose analysis is possible only if we enhance traditional methods of analysis with network methodology, mathematic analysis, and Big Data methodology.
Network linguistics is a modern prospective direction in linguistics, whose main ideas were conceptualized by Crystal ( 2003) in his work “Language and the Internet”. One of its main research questions is how to study peculiarities of the language functioning in the online space. Thus, the methodological foundation of the analysis of language phenomena in the online space is a multi-paradigm and interdisciplinary approach which is determined by the complexity and the scale of sociotechnical features of the online communicative space ( Montgomery, 2017). Network data, therefore, can be regarded as a mediated, yet objective, reflection of interests and needs of the participants of the communication encounter ( Aggarwal, 2011).
We define network data as a block of textual, graphical, audial, visual and multimedia information (content) and metadata cross-linked on a network principle. This data is generated and spread in the online space, and reflects relations between sociopolitical actors in the online space.
Network data exists irrespective of its creator, due to the fluent character of the sphere where it operates. Thus, the principal idea of online interaction is that content generates content ( Calacanis, 2007). Such data seems to have a mind of its own, which causes complexity of its analysis, susceptibility to transformation and alteration with constructive or destructive purposes. Network data can be deleted, excluded from the context, updated or distorted. Thus, its verification is highly warranted.
As a hybrid construct, network linguistic data has a number of distinctive features characteristic of both network and linguistic data. Therefore, it is possible to perform a number of manipulations to collect and analyze network and linguistic data. It is possible to find a particular entry in the selection of entries the same type; to trace the links between the blocks of information; create, modify or delete a particular entry; include or exclude it from context; to conduct sentiment analysis, or to identify intentions of the user; to determine the popularity of a post or a user; to identify a set of linguo-discursive strategies and tactics used by online users and construct their psycholinguistic portrait; to estimate the pragmatic potential of certain content to induce social action.
Network linguistic data differs from properly linguistic data (language data) in terms of its lifespan, non-structural character, non-linear or asynchronous nature, and explicit hypertextuality (apart from its intertextuality). Not only does it enhance the informational capacity of the creator of a media product, rather, it empowers the consumers of the information product.
We define network linguistic data as asynchronous, non-structured verbal and non-verbal language data which forms form asynchronous discursive fields in the online space and can be structured on a network principle, i.e. visualized as a social graph. Its lifespan is not managed by its creator or its source. It is susceptible to any transformation or alteration either with constructive or destructive purposes.
Network linguistic data operating in the online space forms a continuous field—the asynchronous discursive field. The asynchronous discursive field comprises a network of asynchronous discourses made up of discrete messages which are a means of interaction for the actors of the public sphere. The development vector of the discourses is determined by social internet users who contribute to the development of a particular discourse. The degree of such influence is determined by the role of users in the online communication encounter.
Discourses which operate within a particular discursive field through planned or spontaneous linguo-discursive strategies and tactics generate linguistic patterns of social action ( Ryabchenko & Malysheva, 2017). These patterns have high pragmatic potential which generates the ‘power field’ of a particular discursive field whose participants are inevitably affected by its influence. As such influence involves the cognitive aspect of decision making, discursive fields exercise a powerful influence on the consumption patterns of its participants ( Il'in, 2008).
We define the linguistic pattern as a multidimensional socio-cognitive construct maintaining high pragmatic potential which is formed in the mind of an individual due to the consumption of a particular trigger. The types of triggers range from an individual visual, audial, graphical symbol to their combination. Being a symbolic representation of a particular phenomenon or an event, such triggers can induce constructive or destructive social action in the offline space. Notably, the mind of an actor is predetermined by a particular discursive power field. In the online space, discursive fields accumulate certain potential of social action. When an occasional user consumes a linguistic pattern from another discursive field, he/she interprets it according to the background knowledge, conceptual cognitive schemes and a set of decision-making strategies they already have. This can lead to misinterpretation and unpredictable destructive consequences.
A distinctive feature of asynchronous discursive fields which operate in the online space is that linguistic content (network linguistic data) generated by discourses in enhanced by non-verbal means of communication— emoji, symbols, graphics, sound or moving visual images—which can be regarded as network linguistic data as they represent a system of symbols ensuring the transmittance of ideas, attitudes, opinions. This enhances the pragmatic potential of discourses and increases the probability of the fields to accumulate and realize the potential for social action in the offline space.
Research Questions
The main research objective is to answer the following questions.
How are asynchronous discursive fields created and how do they operate in the online space?
What causes the emergence of linguistic patterns in asynchronous discursive fields?
How does the consumption of linguistic patterns lead to constructive or destructive social action?
How can network analysis be used in order to collect network linguistic data and generate datasets and to analyze asynchronous discursive fields in the online space?
The authors of the research aim to describe the stages of the original methodology, which enables the analysis of network linguistic data, and exemplify it with the “PizzaGate” case.
Purpose of the Study
The aim of the research is to develop and test a hybrid methodology enabling analysis of network linguistic data, which generate discursive fields and linguistic patterns of social action that proves to be the result of the directed operation of such data in the online space.
The sociopolitical online space is a global discursive field as it is formed with multiple discursive fields and ensures links between the online users. Notably, discursive fields maintain high potential for social action in the offline space, which causes transformations of the sociopolitical systems in the offline space as it produces complex, stable constructs—linguistic patterns. Linguistic patterns maintain a recurrent emotive component, which has a drastic effect on figurative thinking of recipients, on their cognitive schemes (including decision making), thus, ensuring high pragmatic potential and sustainable emotional background of the discursive field which generates this linguistic pattern.
Network discourse emerges when a particular phenomenon is being discussed, quoted, shared or modified as it is enriched with subjective estimations, attitudes or opinions. Discursive fields in the online space emerge as a response to a particular piece of reality. Once a number of the participants of the discursive interaction account for the ‘critical number’ of users, there forms a ‘power field’ of the discourse. It affects the participants of discursive interaction—their reactions, opinions and, eventually, their decisions. Discursive fields can have a drastic effect on the consumption patterns which can serve as a tool for robust manipulation. Given a particular discursive field is formed and starts operating, political, ideological, psychological, cognitive schemes are implanted into collective consciousness. Power character of online discursive fields is ensured by the ‘filter bubble’ in which they operate and through complex linguo-cognitive constructs generated by the discursive field—through innovative linguistic patterns.
Recurrent use (repetition) of some content ensures sustainable consumption of the linguistic pattern as long as the user is either included in the discursive field from the moment of its formation or acquires all the necessary background knowledge about the reasons and conditions of the discursive field development. The consumption of the linguistic pattern is also determined by the linguo-cognitive and the linguo-cultural aspects of the field in which the pattern operates.
A colossal block of network data which is generated by discursive fields in the online space makes it impossible to instantly and timely identify destructive changes in the socio-political sphere. It results in ex post facto registration of the destructive effect on the development of socio-political systems caused by the discursive space.
The development and testing of a hybrid methodology enabling analysis of network linguistic data, which generate discursive fields and linguistic patterns of social action—which are the results of a task-oriented operation of this data in the online space—is highly warranted, as it will allow us to: identify and develop a typology of linguistic patterns according to the constructive or destructive potential of social action; define the structure of the linguistic pattern and identify the main components which cause its high pragmatic potential; identify linguistic patterns which bear destructive potential and neutralize their effect in the online space of modern states; identify linguistic patterns which bear constructive potential and enhance their effectiveness to reinforce the development of the sociopolitical systems in the post-truth era.
Research Methods
Innovation approach in linguistic studies is a complex methodology of network linguistic data which includes linguo-discursive analysis, network analysis, and folksonomy analysis.
Linguo-discursive analysis is a complex linguistic research method that comprises content analysis and discursive analysis.
Quantitative analysis of content or content analysis is a specific research tool, which uses particular formalized procedures in order to trace the regularities, general trends in the structure of discursive fields and their operation. The peculiarity of content analysis used to analyze network data is that it presupposes counting the frequency and the scope of mentions of some notional units in the analyzed datasets. The identified quantitative characteristics of the network data enable conclusions on the qualitative aspects of meaning of online discourses, including implicit ideas. Thus, the aim of content analysis is to provide an insight into a deeper intertextual reality. Therefore, we can make conclusions on the potential of discursive fields to influence the development of the sociopolitical sphere.
Discourse analysis is a specific research method applied to analyze linguistic data and its relations with social context. Discourse analysis is premised on different interdisciplinary theories: ethnography, social and cultural anthropology, and linguistics. Community is a key notion in most theoretic approaches to discourse analysis, as it determines the ways social actors speak and think and, consequently, determine their role in implementing social practices. Discourse analysis theoretical constructs are less speculative and extend our outlook about the content, structural components, and functions of the analyzed phenomena. Discourse analysis is premised on dialectal recursive interaction between linguistic and the sociopolitical reality and regards a particular text or a communication encounter in the framework of more global sociopolitical entities—text corpora, discursive information, historical context.
Linguo-discursive analysis was used to analyze discursive fields formed by network linguistic data and to identify the patterns of social action. Network analysis was used to form the selection of network linguistic data.
Network analysis is a specific research method and an operational tool based on the methods of mathematical modelling, graph theory and relational algebra ( Gao, Barzel, & Barabási, 2016). It is used to describe network communities and the processes in a network community as scale-free networks. This approach enables analysis and description of social structures which arise due to recurrent nature of the relations within a network ( Scott, 2017). The reason to apply such approach is the efficiency of explanation and possibility of prediction of social phenomena which interpretation of network data can provide. This data is obtained through the analysis of interactions between the entities—network actors. Network actors are visualized as vertices (users) and their relations as edges (links). It allows us to construct a graph which can visualize the degree of proximity and convergence of users within a particular network.
Network analysis includes folksonomy analysis. Folksonomy analysis, or the analysis of tags and hashtags, is a technique used to analyze a practice of collective categorization of linguistic patterns – produced, spread and consumed by internet users in different online discursive fields, through arbitrarily chosen tags, called tags and hashtags ( Lee, 2018; Zappavigna, 2018).
Folksonomy analysis in the given research was used to identify the markers of network linguistic data in online discursive fields.
Findings
The study of network linguistic data is exemplified with the analysis of the asynchronous discursive field “PizzaGate”.
During the US election cycle 2016 ( Enli, 2017), an asynchronous multimodal discursive field “Pizzagate” was generated the online space. This asynchronous multimodal discursive field contained some content which claimed that influential supporters of H. Clinton were allegedly related to a secret organization engaged in violent actions against children. This asynchronous multimodal discursive field had penetrated into such platforms as 4chan, Twitter and Reddit and was eventually picked up by mainstream news sites such as Godlike Productions, YourNewsWire, SubjectPolitics, Conservative Daily Post, The New York Times, which have a rather high trust rate among internet users.
This asynchronous multimodal discursive field “Pizzagate” was intentionally created to discredit H. Clinton, who was running for President, by the linguistic pattern of sociopolitical action ‘Do not vote for Clinton! Vote for Trump, as H. Clinton’s supporters are involved in some discrediting issues’.
Nevertheless, the generated linguistic patterns appeared to be multilayered in terms of implications and sentiments. Its “side effect” was that the pattern also encouraged internet users to—‘rescue the children from the abusers’. The consumption of the pattern resulted in the fact that on 4 December 2016 Edgar Maddison Welch, a 28-year-old North Carolina citizen, discharged a large-caliber rifle inside the “Comet Ping Pong” pizzeria ( Ryabchenko & Malysheva, 2017) as he was convinced that he was liberating the children, who had been harbored in the pizzeria cellar. The cause was the asynchronous multimodal discourse field “Pizzagate”. The consumption of the linguistic pattern—‘rescue the children from the abusers’ resulted in ‘self-investigation’ and triggered armed vigilantism. In the post-truth era—which is, in the modern world has become a synonym to ‘disinformation’—the phrase ‘self-investigation’ reveals the essence of ultimately new format of relationships between people, the truth and sociopolitical action. Millions of people like Welsh, being circumscribed with the so-called ‘filter bubble’, apply a ‘do-it-yourself’ approach when searching and verifying information and facts, which results in uncontrollable and unpredictable cascades of sociopolitical actions.
The sampling of network linguistic data can be formed through a continuous sampling of a bulk of network linguistic data accumulated in the online space ( Bonzanini, 2016) or through the entry point which allows narrowing the scope of the sampled data. Continuous sampling of network linguistic data inevitably causes difficulty with Big Data analysis. Identification of the entry point enables targeted mining of network linguistic data, whose scope can be broadened once the analysis of the first datasets has been completed.
The type of the entry point or the mining criteria falls into technological (quantitative) or notional (qualitative). Technological criteria include a choice of a particular social network or a particular type of data (text, video, commentary or posts), while notional criteria include keywords, hashtags, named entities or dates.
To analyze the lifespan of the discursive field “PizzaGate” we mined relevant network linguistic data using a notional criterion ‘#pizzagate’ and a technological criterion ‘social networking service Twitter’. Using a notional criterion ‘#pizzagate’ and a technological criterion ‘social networking service Twitter’, we formed a block of network linguistic data—dataset ‘Tweet’—comprising the messages containing #pizzagate which were posted on Twitter platform in January 2020. Using network analysis methodology, we subsequently formed the subsets of network linguistic data: ‘Emoji’, ‘Hashtag’, ‘Mention’, ‘Wordcloud’.
Dataset ‘Emoji’ allows analyzing emoticons used in the posts from the ‘Tweet’ dataset and evaluate non-verbal communication in the discursive field ‘Pizzagate’ and its emotional background.
One distinctive feature of the online space is the high speed of content generation, which is why network linguistic data is inevitably represented with massive blocks of data. For example, the number of tweets in D. Trump’s Twitter accounts for 48 300 items. If we include commentaries, whose minimal number for each tweet equals to 1000, the selection would contain 48 mln messages. The analysis of such blocks of network linguistic data makes it possible to single out principal conceptual blocks and to identify their interrelation and intersection. Frequency analysis—which means identification of the most frequently used words and phrases—is therefore conducted, as the most frequently used words and phrases are the foundations of the discursive fields which generate ideas, norms, and values of the users who have produced network linguistic data.
We used a network analysis toolset to analyze the data from the ‘Tweet’ dataset. Thus, we obtained the ‘Wordcloud’ dataset which consists of the most frequently used words and phrases from the ‘Pizzagate’ discursive field. Visualization of the ‘Wordcloud’ dataset in the form of a social graph allows us to conclude that the ‘Pizzagate’ discursive field is not homogeneous in terms of the discourses which operate within the field, and it comprises several discursive field—discursive subfields (see Figure
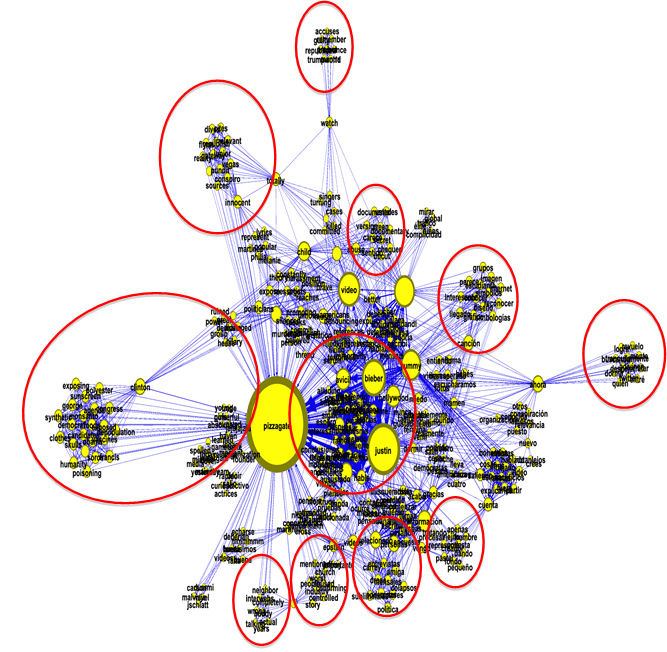
As the graph shows, there is the discursive subfield ‘Clinton’. The analysis of this subfield proves that regardless of the fact that it has been four years since the incident with Maddison Welsh, the linguistic pattern ‘Do not vote for Clinton!...’ is still relevant (see Figure
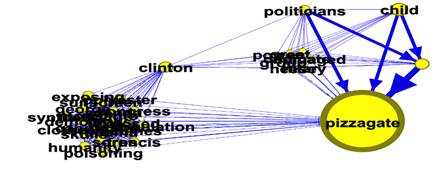
Notably, the findings of the analysis of the ‘Mention’ dataset prove that the official Twitter account of the US President D. Trump—@realdonaldtrump—is an active participant of the discursive field ‘Pizzagate’.
The analysis of the ‘Hashtag’ dataset, which consists of the hashtags used in the messages comprising the ‘Tweet’ dataset, allows exploring the user-generated classification of the content generated within the studied discursive field. As the ‘Tophashtag’ dataset was formed due to the analysis of the network linguistic data comprising the ‘Tweet’ dataset, the hashtags which comprise this dataset can be visualized as a social graph. This enables the analysis of the hashtags themselves, but rather their combinations and links. Notably, despite the fact that H. Clinton is listed in the keywords selection and we have singled out the entire subfield ‘Clinton’, the discursive field ‘Pizzagate’ does not include #clinton. This proves that the discursive subfield ‘Clinton’ and its linguistic pattern ‘Do not vote for Clinton! Vote for Trump, as H. Clinton’s supporters are involved into some discrediting issues’ are in hibernation and can be brought to the political arena. This also shows that multidimensional analysis of network linguistic data is highly warranted due to non-linear and multilayer nature of such data.
Conclusion
In the framework of the network society, complex analysis of network linguistic data is based on the integrated methodology and theory from several scientific spheres: relational sociology, mathematics, network theory and linguistics, Big Data methodology, network analysis, folksonomy analysis, and linguo-discursive analysis.
Nowadays, problems in the sociopolitical sphere arise due to the development of particular online discourses. Convergence of several discourse is the foundations for the development of a particular discursive field, whose distinctive feature is its power field, i.e. the ability to determine users’ behavioral patterns in terms of information consumption and generate constructive or destructive linguistic patterns, which determine internet users’ decision-making behavior.
The linguo-discursive methodology enables analysis of discursive fields and linguistic patterns of social action and is applied to evaluate network linguistic data, which operates within discursive fields and results in generating particular linguistic patterns.
Insights into the main principles of the discursive field operation will allow identification of the triggers and the point where the critical number of users is exceeded, which means the formation of a particular discursive field. Besides, it will allow us to determine and describe a complex set of linguistic means which ensure the targeted formation of particular linguistic patterns within a particular discursive field. It will be possible to develop a typology of discursive fields regarding their constructive or destructive potential for the development of sociopolitical systems and modify constructive or destructive development vectors of online discursive fields.
The analysis of ‘Pizzagate’ case allowed us to conclude that asynchronous discursive fields have a particular lifespan and are capable of retaining and accumulating certain linguistic patterns. Occurrence of a trigger in a discursive field can induce either constructive or destructive social action both in the online and in the offline space.
Acknowledgments
The research is given a financial support by The Russian Foundation for Basic Research (Department of Humanitarian and Social Science), the research project no. 20-012-00033 entitled “Linguistic models of sociopolitical communication in online space: discursive fields, patterns and hybrid methodology of network data analysis.” (2020-2022).
References
- Aggarwal, C. C. (2011). Social Network Data Analytics. Springer. https://doi.org/10.1007/978-1-4419-8462-3
- Bonzanini, M. (2016). Mastering Social Media Mining with Python. Birmingham-Mumbai. PACKT Publishing.
- Calacanis, J. (2007, October 3). Web 3.0, the “official” definition. Retrieved from https://calacanis.com/2007/10/03/web-3-0-the-official-definition/
- Clement, J. (2019, November 20). Worldwide digital population as of October 2019. Retrieved from https://www.statista.com/statistics/617136/digital-population-worldwide/
- Crystal, D. (2003). Language and the Internet. Cambridge. Cambridge University Press.
- Enli, G. (2017). Twitter as arena for the authentic outsider: exploring the social media campaigns of Trump and Clinton in the 2016 US presidential election. European Journal of Communication, 32(1), 50-61. https://doi.org/10.1177/0267323116682802
- Gao, J., Barzel, B., & Barabási, A.-L. (2016). Universal resilience patterns in complex networks. Nature 530, 307-312.
- Il'in, V. I. (2008). Потребление как дискурс [Consumption as Discourse]. SPb.: Intersocis.
- Kallas, P. (2019, September 2). Top 15 Most Popular Social Networking Sites and Apps. Retrieved from https://www.dreamgrow.com/top-15-most-popular-social-networking-sites
- Lee, C. (2018). Discourse of Social Tagging. Discourse, Context & Media, 22, 1-3. https://doi.org/10.1016/j.dcm.2018.03.001
- Masías, V. H., Hecking, T., & Hoppe, H. U. (2018). Exploring the Relationship Between Social Networking Site Usage and Participation in Protest Activities. Frontiers in Applied Mathematics and Statistics, 4(56). https://doi.org/10.3389/fams.2018.00056
- Montgomery, M. (2017). Mediation, technological change, and discourse: the case of television talk. In C. Cotter, D. Perrin (Eds.) The Routledge Handbook of Language and Media, 113-135. https://doi.org/10.4324/9781315673134-10
- Pleshakova, A. (2017). Mediation, technological change, and discourse: the case of television talk. In C. Cotter, D. Perrin (Eds.) The Routledge Handbook of Language and Media, 77-92. https://doi.org/10.4324/9781315673134-7
- Ryabchenko, N., & Malysheva, O. (2017). How to Become a President: Election Technologies in the Post-Truth and Fake News Era. Man In India, 97(26), 507-527.
- Scott, J. (2017). Social Network Analysis. SAGE Publications Ltd.
- Zappavigna, M. (2018). Searchable Talk: Hashtags and Social Media Metadiscourse. Sydney: Bloomsbury Publishing Plc.
Copyright information
This work is licensed under a Creative Commons Attribution-NonCommercial-NoDerivatives 4.0 International License.
About this article
Publication Date
03 August 2020
Article Doi
eBook ISBN
978-1-80296-085-3
Publisher
European Publisher
Volume
86
Print ISBN (optional)
-
Edition Number
1st Edition
Pages
1-1623
Subjects
Sociolinguistics, linguistics, semantics, discourse analysis, translation, interpretation
Cite this article as:
Ryabchenko, N. A., & Malysheva, O. P. (2020). Innovative Approaches In Linguistics: Network Analysis Of Linguistic Data. In N. L. Amiryanovna (Ed.), Word, Utterance, Text: Cognitive, Pragmatic and Cultural Aspects, vol 86. European Proceedings of Social and Behavioural Sciences (pp. 1199-1209). European Publisher. https://doi.org/10.15405/epsbs.2020.08.138