Abstract
In today’s digitalised era, the adoption of Industry 4.0 technology pillars can boost businesses by enhancing organizational performance. As one of the crucial pillars, big data potential has been receiving heightened interests from academic and practitioners. Small Medium Enterprise (SMEs) played a vital role in Malaysia and Big Data Analytics (BDA) is seen as a new strategic business tool that enables organizations to gain valuable insights for decision-making towards better performance. Most multinationals and larger companies may already be implementing BDA aiming at enhancing organizational performance, but many SMEs are still at the exploratory stage. Malaysia is heavily invested in accelerating the BDA adoption and innovation for SMEs. In 2020, Malaysia is encouraged by the government to become a BDA hub in ASEAN. The present study, drawing upon Technological, Organizational and Environment (TOE) model, proposes and investigates the influence of TOE factors on BDA adoption among Malaysian SMEs. The result will contribute to the understanding of the determinants affecting BDA adoption.
Keywords: Technology Organizational Environment (TOE)Big Data Analytics AdoptionInnovation diffusionSmall Medium Enterprises
Introduction
Since 2011, the term Industry 4.0 has been introduced at Hannover fair one of the world largest trade fair. Data has a major impact on the revolution of networks; digital technology, people and platforms changed the business. Small, Medium and Enterprises (SMEs) play an importance role in Malaysia in the late 1990’s. SMEs are the backbone for of business establishments in Malaysia. SMEs are driver’s economy growths; it represented 98.5% of all business establishments in the country and contribution total 66% of employment in 2017 (DOSM, 2017). SMEs were at the core of economic transition in Malaysia into a middle-income country and are a vital drive of growth in job. A study found that 69% of Malaysia SMEs have incorporated Industry 4.0 technologies into their operations such as automation software, BDA and mobile payments. Mahalingam’s (2018) study also shows a significantly high adoption rate of BDA among Malaysian SMEs at 77%. Developing world is rapidly created the amount of data; BDA is a revolution within the field of data technology. BDA from the manufacturing is concerning analytic of assorted operational knowledge generated in a typical producing method like equipment, maintenance systems, internal control, instrument. BDA done systematically effective actions will achieve smart manufacturing and operational excellence.
SMEs are a vital contribution to economic development, political stability and social ascent of each county. SME can be started for any kind of business activities in a rural and urban area. Many studies have been conducted regarding SME challenge, with broad views that included; SME unskillfulness, restricted banking service, access to credit and survival rates. Real GDP growth in Malaysia’s SMEs has persistently exceeded the overall economy, averaging 6.6 percent per annum compared to 5.1 GDP growth over the past 14 years (2004-2017) (DOSM, 2017). SMEs are considered as the foundation of industrial growth and Report from Department of Statistic Malaysia, Malaysia Gross Domestic Product (GDP) in 2017 was RM1.353 trillion grew 9.9% compared to 2016. Today, SME Company occupies a position of strategic vital in the Malaysia economic structure due the term of import, export and employment contributions are significant. The SMEs sector have a chance to take advantage from BDA technology to make fast and right decisions to develop their business (Polkowski, Khajuria, & Rohadia, 2017). Having less employee and lower revenue does not mean SMEs will not need the big data. SMEs embrace the big data to create new market opportunity, understand customer needs better, reduce operational costs and focus on the targeted market
Big Data Analytics
The ‘V’ definition is regarded to highlight data related dimensions in order to define big data. Some scientists present 3 mains ‘Vs’ as ‘Volume, Velocity, Variety’ (Arockia, Varnekha, & Veneshia, 2017; McAfee & Brynjolfsson, 2012). The ‘Volume’ is the main big data dimension that makes big data differ from traditional information concept. Currently, Big data sizes is expressed in petabytes exabytes or zettabytes (Garmaki, Boughzala, Wamba, & Fosso, 2016). The enormous amount of information is produced by distinct formats from big sources, includes multidimensional information areas including the structured and unstructured type and current big data ‘Variety’. Data generation speed or information distribution frequency is focused on big data ‘Velocity’. By considering the prospective financial advantage of big data, Oracle (2013), Gantz and Reinsel (2011) and present big data with 4 ‘Vs’ they are volume, value, variety and velocity. Oracle generally describes the information obtained in the initial form with a small value relate to its quantity. However, by evaluating big quantities of such information, a high value can be acquired. To emphasize the importance of data quality and reliability of big data resource, Russom (2011) indicated ‘Veracity’ as the fifth dimension and defines big data like Volume, Value, Variety and Velocity and Veracity’ which is also considered as date related aspects in this paper as well.
In the globalized and technology changing environment, organizations are facing great challenges, especially when facing big data issues. Big data is useless on its own but can lead to powerful results when it is effectively mined. Businesses that can leverage big data in a meaningful manner will have a competitive advantage over others. Delay in processing big data to extract business insight could possibly cost a million of lost revenue and resulted in a competitive disadvantage in businesses. Apparently, traditional solutions such as Excel spreadsheets and conventional relational databases are incapable of analysing disparate big data sets. Relational databases require structured data for final analysis and there is insufficient time or resources to place massive amounts of data being generated each second into a structured format. Likewise, business intelligence (BI) tools typically require technology to create pre-configured dashboards and static reports and are not interactive in nature.
Despite the sophistication of traditional BI solutions, when comes to big data sets, traditional BI tools are incapable of managing big data's basic characteristics such as high velocity, volume and variety. They are unable to collect and store information from online sources, such as blogs or social media, connected devices, and application data. They are unable to combine and correlate a variety of data sets and incapable in analysing all data simultaneously. Thus, the attributes of traditional solutions make it best at analysing structured datasets to provide answers to predetermined questions only.
BDA technologies allow companies to improve the existing application by offering business focused practices and methods that offer competitive pressure (Chen, Chiang, & Storey, 2018; Davenport, 2006). BDA is examining the varied and large amount of data sets to identify similarities, market trends, hidden pattern and consumer expectations that can help firm make a business decision. Driven systems for analytics like BDA will help various business advantages, such as more effective customer service, marketing, better operating performance, new revenue opportunities and competitive pressure on rivals. The study on BDA has mainly concentrated on the role of BDA capability and identify it direct effect on organizational performance. However, researchers have criticized the fact that IT resources and capabilities alone cannot directly define organizational performance.
Some IT productivity paradox research indicated that big data could not produce substantial productivity gains in SMEs. Wang, Kung, Wang, and Cegielski (2018) argue adopting practice base view would build more comprehensive picture of how BDA can maximize efficiency to generate business value. Kwon, Lee, and Shin (2014) define the BDA as methods that can improve organizational performance by analysing complex. Frost and Sullivan (2015) describe BDA as the entire process from the acquisition and aggregation of structured and unstructured largescale data from different sources to economically, timely storage, management, analysis and access. Wamba, Akter, Edwards, Chopin, and Gnanzou (2015) explain BDA is an integrated system involving the analysis, compilation, interpretation and handling of numerous various divisions in order to achieve insights that can be implemented, produce business value and create competitive pressure.
SMEs Big Data Analytics Challenges
Technology and innovations are considered one of its key drivers as strategic goals for SME development and big data (Sen, Ozturk, & Vayvay, 2016). The ability to analyse and forecast business and consumer behaviour with big data is a new paradigm change for SMEs. Through making better decisions, it can offer efficiency, flexibility, responsiveness and the ability to meet client needs. Thompson, Williams, and Thomas (2013) the growth of SMEs could potentially increase is due to the common belief of the innovation orientation. Ventures across the spectrum, from multinationals to SMEs investigate avenues to burden and exploit the data.
Using BDA technologies adjust the way business work across enterprises. To address their voluminous data challenges, SMEs are desperately required to genuinely consider the adoption of big data. BDA provides different open doors for SMEs to make an aggressive key impact on basic leadership. Advanced data management and analytics drive business and business development. SMEs are well placed to take advantage of the long-lasting benefits of huge information. Because of their size and adaptability, even a minor change can effectively encourage a substantial scale. All SMEs are unable to afford advanced new technology, whenever a company is considering investing new technology, cost and value for money also play a critical role. Equally important may be that SMEs business also lack the capacity to selecting, configuring, installing and maintain sophisticated IT systems. Such consideration pose a potential challenge to BDA in SMEs (Schmidt & Möhring, 2013).
Problem Statement
Terziovski (2010) study found that the high failure rate was primarily due to a lack of frameworks of informal strategic planning process which keep track of the success of SMEs. Base on the United State small business administration, almost 50 percent of SMEs failed in the first year and 95 percent tended to fail by the 5 years period. SMEs with less than 20 workers have 5 years survival rate of only a 37 percent and 10 years survival rate 9 percent (Kannan, 2016). Although there are not reliable sources of publishing failure rates for SMEs in Malaysia, there are serious issues facing sustainable SMEs performance. Since Industry 4.0 has slowly lead SMEs in the digital technology innovation development, SMEs can optimize the performance and efficiency in technologies. For the year 2012-2013 at declined trends in BDA is reported by (Kiron, Prentice, & Ferguson, 2014).
Progressively organizations adopted BDA to gain competitive pressure; the steep growth curve of BDA based organizations is diminishing. Coleman et al. (2016) have shown that SMEs are in danger of being left behind for slow adoption new BDA technology. BDA play a key role as the organization start their digital transforms. International Data Corporation (IDC) mentions by 2021, BDA software marketing Malaysia forecasted to reach RM595million. In 2017, Malaysia BDA software expenditure will total RM433.7million, an increase of 10.9% over 2016 (IDC, 2017) Malaysia organizations have started to prioritize analytical solutions. Early adopters are both SMEs and traditional manufacturing companies (Raju, 2018). Big data is seen to be as vital to society and businesses as the technology keep improving. Some information will lead to more reliable analyses and make decisions more comfortable. A good business decision will mean lower risk, higher operational efficiencies and cost reduction to business. Big data brings developing countries a lot of challenges, but it also brings tremendous benefit to improve the rural communities and transformation in economic and social life (Kshetri, 2016). In order to determine the local and regional future of the industry, Malaysia must enhance big data investment. The growths of the Internet, the extent of smart mobile devices and social networking have contributed to the ever-increasing amounts of data that can be accessed by companies. (Coleman et al., 2016) identify SMEs has various factor condition adoption of BDA in business.
Research Questions
The purpose of the study is to investigate the impact of technological, organizational and environmental (TOE) factors on BDA adoption among Malaysian SMEs. This study will examine three main research questions in particular:
Does technological factor have a positive impact on BDA adoption among the Malaysian SMEs?
Does organizational factor have a positive impact on BDA adoption among the Malaysian SMEs?
Does environmental factor have a positive impact on BDA adoption among the Malaysian SMEs?
Purpose of the Study
SMEs are the largest segment of international market segment and their contribution to any economy’s growth and survival requires constant study. Malaysia’s SMEs are the biggest contributor to economic growth (Moorthy et al., 2012). SMEs accounted for approximately 99.2 percent of all Malaysian business entities as of 2010. They contributed for 42 percent of the GDP, 19 percent of total exports and up to 56 percent of the country’s overall employment level (DOSM, 2017). SMEs continue to perform as they contribute significantly to the GDP of their national economies (Moorthy et al., 2012). While contribution levels are high, success of SMEs and innovative technology was found to receive insufficient research focus, which was the primary impetus for this study. BDA has been reported to be increasingly adopted by both middle market organization and large enterprises. Mid-market organizations were defined as organizations with 500 - 5, 000 employees, while large companies were defined as organizations with 5, 000 or more employees.
The role that analytics and big data play in these organizations and enterprises is expanding at an impressive pace, particularly in business processes, operational and decision-making (IIA, 2015). IDC (2017) reported that large companies, with a size of 1,000 employees and more, would be responsible for more than 60% of all BDA expenditure (BDA revenue forecast to reach US$150.8 billion in 2017) and IDC forecasts big business spending on BDA in 2018 will be more than US$ 100 billion. SMEs GDP in manufacturing sector recorded a marginal increase of 34.65% compared to the year 2016 34.4% (DOSM, 2017). Tightening measures and adverse external factors, such as a thorough trade war, ensure the strengthening of the SME sector is essential to strengthen the economy. According to the 11th Malaysian Plan 2016-2020 study was mid-term updated: The government of Malaysia has retained its target of 41 percent of national GDP for Malaysian SMEs by 2020, compared to 37.1 percent by 2017. In order to obtain a significant and representable result, the target respondents of this study are 650 companies SMEs manufacturing sector in Malaysia.
Significance of The Study
Many recent studies have highlighted that BDA as a research discipline are still evolving and not yet established, particularly in the research field of management (Frizzo-Barker, Chow-White, Mozafari, & Ha, 2016). The lack happened especially on theoretical constructs and academic rigour. Gupta and George (2016) observed that most of the current big data literature has been written by technology consultants and therefore lack of theoretical insight. This study combines diffusion of innovation theory and TOE framework, expect to discover and develop a BDA adoption model and investigate factors influencing adoption among Malaysian SMEs. With the recognized importance of data-driven organizations, and the potential business value from BDA, more and more organizations invested or planning to invest in BDA. However, the adoption trend is at a decreasing rate (Kiron et al., 2014).
There are gaps in BDA investment and expectations, business value from BDA investment was not demonstrated clearly (Sharma, Mithas, & Kankanhalli, 2014; Mithas, Lee, Earley, Murugesan, & Djavanshir, 2013). Malaysia is heavily invested in accelerating the BDA adoption and innovation within the country. The government is pushing for Malaysia to become a BDA hub in ASEAN by 2020. The study findings have immense practical effects in Malaysia on different industries, such as manufacturing, retail, public sector administration, and healthcare. Government bodies and policymakers involved such as MDEC, MAMPU and the rest can make use of the findings to assist in crafting their strategy in promoting BDA adoption in Malaysia.
Conceptual Model
Many researchers have suggested combining more than one theoretical perspective to better explain the adoption of technology at the organizational level (Salahshour Rad, Nilashi, & Mohamed Dahlan, 2018; Oliveira & Martins, 2011; Wang & Wang, 2016). In order to better understanding the technology adoption organizational decisions, the background study should be details, and the variables should be adapted to the complexity of change (Oliveira, Thomas, & Espadanal, 2014; Salahshour Rad et al., 2018). Diffusion of innovation is consistent with TOE framework (Oliveira et al., 2014; Salahshour Rad et al., 2018; Wang & Wang, 2016).
Studies have found that TOE framework particularly well when integrated with DOI theory in explaining organization level innovation. DOI shares some similarities with TOE framework (Oliveira et al., 2014). Firstly, technological contexts of TOE are essentially the same view with Rogers (1983) DOI innovation characteristics. Secondly, the external and internal organizational features of DOI include the same steps as the organizational framework of TOE. On the other hand, there are differences between DOI and TOE framework, which compliments each other (Oliveira et al., 2014). TOE does not describe the role of individual characteristics of the DOI within its organizational context (e.g. top management support). Likewise, DOI does not take environmental context into account where technological context is one of the important contexts under TOE. Therefore, the combination of these two theories and framework complements each other and is capable of providing a comprehensive understanding of technological adoption at the organizational level. Based on the above, this research adapted the TOE model and DOI theory to explore BDA adoption determinants among Malaysian SMEs.
Figure
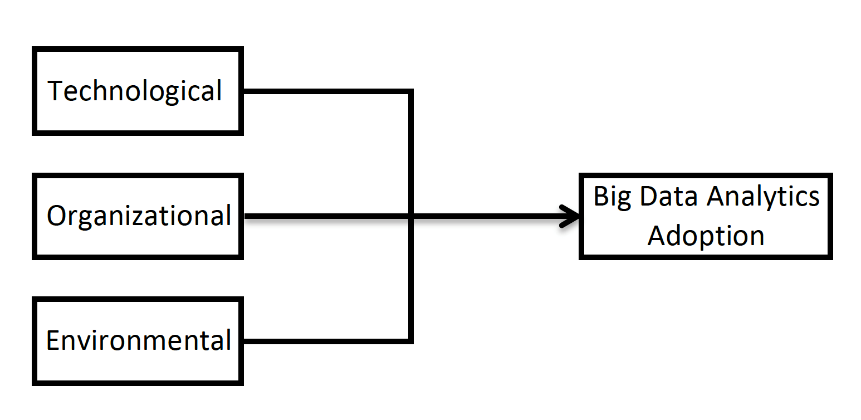
Research Methods
This is a conceptual paper. As such, no research methods were used.
Findings
This is a conceptual paper. As such, there aren’t any findings.
Conclusion
As SMEs adapts to the era of the 4th Industrial revolution, BDA adoption has become a critical issue. Based on the literature synthesized, this study proposed a conceptual model highlighting the factors influencing BDA adoption based on Technology-Organization-Environment framework. In conclusion, this paper put forward three key factors namely technological, organizational and environmental factors that are posited to influence BDA adoption among Malaysian SMEs.
References
- Arockia, P. S., Varnekha, S. S., & Veneshia, K. A. (2017). The 17 V’s Of Big Data. International Research Journal of Engineering and Technology (IRJET), 4(9), 3–6. Retrieved from https://irjet.net/archives/V4/i9/IRJET-V4I957.pdf
- Chen, H., Chiang, R. H., & Storey, V. C. (2018). Business Intelligence And Analytics: From Big Data to Big Impact. MIS Quarterly. https://doi.org/10.2307/41703503
- Coleman, S., Göb, R., Manco, G., Pievatolo, A., Tort-Martorell, X., & Reis, M. S. (2016). How Can SMEs Benefit From Big Data? Challenges And A Path Forward. Quality and Reliability Engineering International, 32(6), 2151-2164. https://doi.org/10.1002/qre.2008
- Davenport, T. H. (2006). Competing On Analytics. Harvard Business Review. Retrieved from http://www.impactline.net/%C0%DA%B7%E1%C3%B7%BA%CE%B9%B0/OLAPDW/AnalyticsHBR.pdf
- DOSM (2017). Department of Statistics Malaysia Press Release Small and Medium Enterprises. Department of Statistics Malaysia. Retrieved from https://www.dosm.gov.my/v1/index.php?r=column/pdfPrev&id=cEI0bklpZHJaTlhRNDB3d2ozbnFIUT09
- Frizzo-Barker, J., Chow-White, P. A., Mozafari, M., & Ha, D. (2016). An Empirical Study Of The Rise Of Big Data In Business Scholarship. International Journal of Information Management, 36(3), 403–413. https://doi.org/10.1016/j.ijinfomgt.2016.01.006
- Frost, W., & Sullivan, P. (2015). National Big Data Analytics Initiative : Assessing The Opportunity & Impact Of Big Data Analytics In Malaysia. Retrieved from frost.com
- Gantz, J., & Reinsel, D. (2011). Extracting Value from Chaos State of the Universe. IDC iView. Retrieved from https://uk.emc.com/collateral/analyst-reports/idc-extracting-value-from-chaos-ar.pdf
- Garmaki, M., Boughzala, I., Wamba, S. F., & Fosso, S. (2016). The Effect of Big Data Analytics Capability On Firm Performance. In Pacific Asia Conference on Information Systems Proceedings. Retrieved from https://pdfs.semanticscholar.org/7ea1/cdf8d22c9f28b53b48a16212d585fe7a6242.pdf
- Gupta, M., & George, J. F. (2016). Toward The Development Of A Big Data Analytics Capability. Information and Management, 53(8), 1049-1064. https://doi.org/10.1016/j.im.2016.07.004
- IDC (2017). Malaysia Big Data Analytics Software Market To Hit RM595 million by 2021, Says IDC. Retrieved November 10, 2018, from https://www.idc.com/getdoc.jsp?containerId=prAP43367017
- IIA (2015). Advanced Analytics & Big Data Adoption Report. Retrieved from http://datastorageasean.com/sites/default/files/IIA_dell-big-data-external.pdf
- Kannan, G. (2016). What’s Holding You Back ? 11 Factors That Derail SME’s Track To Success. Retrieved October 21, 2018, from https://leaderonomics.com/pdf/LDR-161210-04.pdf
- Kiron, D., Prentice, P. K., & Ferguson, R. B. (2014). The Analytics Mandate. MIT Sloan Management Review, 55(4), 1-25.
- Kshetri, N. (2016). Big Data’s Big Potential In Developing Economies: Impact On Agriculture, Health And Environmental Security. https://doi.org/10.1079/9781780648682.0000
- Kwon, O., Lee, N., & Shin, B. (2014). Data Quality Management, Data Usage Experience And Acquisition Intention Of Big Data Analytics. International Journal of Information Management, 34(3), 387-394. https://doi.org/10.1016/j.ijinfomgt.2014.02.002
- Mahalingam, E. (2018). How Retail SMEs Can Stay Competitive. Retrieved November 10, 2018, from https://www.thestar.com.my/business/smebiz/2018/09/03/how-retail-smes-can-stay-competitive/
- McAfee, A., & Brynjolfsson, E. (2012). Big Data: The Management Revolution. Harvard Business Review. Retrieved from https://wiki.uib.no/info310/images/4/4c/McAfeeBrynjolfsson2012-BigData-TheManagementRevolution-HBR.pdf
- Mithas, S., Lee, M. R., Earley, S., Murugesan, S., & Djavanshir, R. (2013). Leveraging Big Data And Business Analytics. IT Professional, 15(6), 18-20. https://doi.org/10.1109/MITP.2013.95
- Moorthy, M. K., Tan, A., Choo, C., Wei, C. S., Tan, J., Ping, Y., & Leong, T. K. (2012). A Study on Factors Affecting the Performance of SMEs in Malaysia. Academic Research in Business and Social Sciences, 2(4), 224–239.
- Oliveira, T., & Martins, M. F. (2011). Literature Review Of Information Technology Adoption Models At Firm Level. Electronic Journal Information Systems Evaluation, 14(1), 110–121.
- Oliveira, T., Thomas, M., & Espadanal, M. (2014). Assessing The Determinants Of Cloud Computing Adoption: An Analysis Of The Manufacturing And Services Sectors. Information and Management, 51(5), 497-510. https://doi.org/10.1016/j.im.2014.03.006
- Oracle. (2013). Information Management And Big Data A Reference Architecture. Oracle White Paper, 28. Retrieved from https://www.oracle.com/technetwork/topics/entarch/articles/info-mgmt-big-data-ref-arch-1902853.pdf
- Polkowski, Z., Khajuria, R., & Rohadia, S. (2017). Big Data Implementation In Small And Medium Enterprises In India And Poland. Scientific Bulletin - Economic Sciences / Buletin Stiintific - Seria Stiinte Economice, 16(3), 149–161. Retrieved from http://proxy.lib.sfu.ca/login?url=https://search.ebscohost.com/login.aspx?direct=true&db=aph&AN=130869411&site=ehost-live
- Raju, C. (2018). Econ 4.0: How Big Is Big Data? Retrieved November 10, 2018, from http://www.theedgemarkets.com/article/econ-40-how-big-big-data
- Rogers, E. M. (1983). Diffusion Of Innovations (3th ed.). Macmillian Publishing Co. https://doi.org/citeulike-article-id:126680
- Russom, P. (2011). Big Data Analytics. Tdwi Research, 1, 3–5. Retrieved from https://vivomente.com/wp-content/uploads/2016/04/big-data-analytics-white-paper.pdf
- Salahshour Rad, M., Nilashi, M., & Mohamed Dahlan, H. (2018). Information Technology Adoption: A Review Of The Literature And Classification. Universal Access in the Information Society, 17, 361–390. https://doi.org/10.1007/s10209-017-0534-z
- Schmidt, R., & Möhring, M. (2013). Strategic Alignment Of Cloud-Based Architectures For Big Data. In Proceedings - IEEE International Enterprise Distributed Object Computing Workshop, EDOC. https://doi.org/10.1109/EDOCW.2013.22
- Sen, D., Ozturk, M., & Vayvay, O. (2016). An Overview Of Big Data For Growth In SMEs. Procedia - Social and Behavioral Sciences, 235, 159–167. https://doi.org/10.1016/j.sbspro.2016.11.011
- Sharma, R., Mithas, S., & Kankanhalli, A. (2014). Transforming Decision-Making Processes: A Research Agenda For Understanding The Impact Of Business Analytics On Organisations. European Journal of Information Systems, 23, 433-441. https://doi.org/10.1057/ejis.2014.17
- Terziovski, M. (2010). Innovation Practice And Its Performance Implications In Small To Medium Enterprises (SMEs) In The Manufacturing Sector: A Resource-Based View. Strategic Management Journal, 31(8), 892–902. https://doi.org/10.1002/smj.841
- Thompson, P., Williams, R., & Thomas, B. (2013). Are UK Smes With Active Web Sites More Likely To Achieve Both Innovation And Growth? Journal of Small Business and Enterprise Development, 20(4), 934–965. https://doi.org/10.1108/JSBED-05-2012-0067
- Wamba, S. F., Akter, S., Edwards, A., Chopin, G., & Gnanzou, D. (2015). How “Big Data” Can Make Big Bmpact: Findings From A Systematic Review And A Longitudinal Case Study. International Journal of Production Economics, 165, 234-246. https://doi.org/10.1016/j.ijpe.2014.12.031
- Wang, Y-M., & Wang, Y-C. (2016). Determinants Of Firms’ Knowledge Management System Implementation: An Empirical Study. Computers in Human Behavior, 64, 829-842. https://doi.org/10.1016/j.chb.2016.07.055
- Wang, Y., Kung, L. A., Wang, W. Y. C., & Cegielski, C. G. (2018). An integrated big data analytics-enabled transformation model: Application to health care. Information and Management, 55(1), 64-79. https://doi.org/10.1016/j.im.2017.04.001
Copyright information
This work is licensed under a Creative Commons Attribution-NonCommercial-NoDerivatives 4.0 International License.
About this article
Publication Date
30 March 2020
Article Doi
eBook ISBN
978-1-80296-080-8
Publisher
European Publisher
Volume
81
Print ISBN (optional)
-
Edition Number
1st Edition
Pages
1-839
Subjects
Business, innovation, sustainability, development studies
Cite this article as:
Hong, L. C., & Ping, T. A. (2020). Investigating Determinants Of Big Data Analytics Adoption In Malaysian Smes. In N. Baba Rahim (Ed.), Multidisciplinary Research as Agent of Change for Industrial Revolution 4.0, vol 81. European Proceedings of Social and Behavioural Sciences (pp. 255-263). European Publisher. https://doi.org/10.15405/epsbs.2020.03.03.32