Abstract
The infamous CXCR2-CXCL8 axis, now trending for high tissue expression during tumorigenesis and chemo-resistance, led to the discovery of various antagonist compounds with varying efficacies. Undeniably, they are challenging to manipulate and pose toxic effects due to non-specificities. Therefore, the antibody is chosen for its specificity, demonstrable minimum off-target effects. Albeit various antibodies available commercially, almost none disclosed variable region for verification. Here, we visualised the interaction of receptor-antibody/ligand by generating in silico models. HY29-1 IgG (VH&VL) sequences were sourced from a patent while the CXCR2 receptor sequence was taken from UniProt web. For comparison, the structure of monomeric IL8 obtained from the RCSB PDB web was included. All modelling of complexes was done using Schrödinger software suite: molecular docking was done on ‘Glide’ while ‘Bioluminate’ was used to perform visualisation and prediction of the interacted residues. From the models, we identified the interacted residues between HY29-1/CXCR2 and CXCL8/CXCR2 complexes. Although both HY29-1 and IL8 mostly bind on N-terminal CXCR2, differing residues may result in antagonism/ agonism effect on CXCR2 receptor. This finding will guide us towards the development of targeted therapy against various cancers.
Keywords: CXCR2scFvIL8molecular modellingin silico
Introduction
Physiologically, CXCR2 receptor is mainly expressed on the surface of leukocytes and lymphocytes which played immense role in mediating the process of cells migration across endothelium and within tissues from the circulation in response to inflammation due to infection (Dyer et al., 2019) and wound healing (Sharma, Nannuru, Saxena, Varney, & Singh, 2019). More importantly, its chemokines particularly interleukin-8 (CXCL8) are shown to be strong neutrophil chemo-attractant (Liao et al., 2019) and able to perpetuate angiogenesis, proliferation and aid in the development of chemotherapy resistance in various cancer types (Sharma et al., 2019).
Tumorigenesis and antitumor immune responses suppression phenotypes due to enhanced CXCL8 expression are associated with the presence of cancer-associated fibroblasts in advanced primary gastric cancer (Zhai et al., 2019), recruitment of pro-tumorigenic tumour-associated neutrophils (TAN) and tumour-associated macrophages (TAM) in colorectal cancer (CRC) (Ogawa et al., 2019) and cancer stem-like cells in chemo- and radio-therapeutic resistant cancer cells in triple negative breast cancer (Ruffini, 2019; Wang et al., 2018). Blocking of CXCR2/CXCL8 signalling increases the sensitivities of resistant cancer cells towards immune checkpoint inhibitor (anti-PD-1 therapy) (Liao et al., 2019) and irinotecan therapy in CPT-resistant CRC (Chen et al., 2019), platinum-based therapy in cisplatin-resistant gastric cancer (Zhai et al., 2019), gemcitabine-resistant pancreatic cancer (Imafuji et al., 2019) and targeting myeloid-derived suppressor cells against cisplatin-resistant bladder cancer (Takeyama et al., 2019).
Despite being hailed as highly specific, toxicities have been reported in antibody therapy (Peterson et al., 2018). Although personalised strategy based on expression levels of relevant biomarkers may guide the choice of combined (individual) antibody, dosing schedules and duration of therapy to ensure optimised therapeutic potential with minimum side effects (Peterson et al., 2018), improvement onto the target specificities and affinities should play an immense role in reducing toxicities too. Therefore, precise determination and improvements to the target site should be looked at to achieve high target specificity while minimising the potential off-target toxicities. One most profound modification that sets apart the true quality of antibody from the rest of therapeutic molecules is the ability for directed (in vivo and in vitro) antigen-binding affinity maturation (Tiller & Tessier, 2015). The objective of this process is to improve the antibody’s biological activity and ultimately enhance treatment efficacy, apart from reducing toxicities and cost of manufacturing (Sefid, Payandeh, Azamirad, Abdolhamidi, & Rasooli, 2019).
Mouse anti-human CXCR2 antibody, ZY05, was developed by VelocImmune mice immunisation & hybridoma generation and fused with human CH and CL chains into humanised HY29-1 anti-CXCR2 antibody (Rossant et al., 2014). HY29-1 showed specific binding to N-terminal of CXCR2 and exhibited functional blockade towards IL8 and other ligands at the orthosteric site, therefore possess the possibility of therapeutic potential. In this study, we visualise the binding of HY29-1 antibody & CXCR2 receptor and compare the interacted residues of HY29-1/CXCR2 with CXCL8/CXCR2 complexes.
Problem Statement
The revelation of the importance of CXCL8-CXCR2 pathway in driving invasiveness, tumorigenesis and resistance towards several chemotherapeutic agents in colorectal cancer has perpetuated the discovery of various compounds with varying antagonistic properties. However, these compounds are difficult to manipulate and tend to have high off-target effects. For this reason, the antibody is the best solution for its ease of modifications and improvement of binding affinity through molecular engineering to reduce its off-target effects.
In order to design a modified antibody for improved binding, more in-depth understanding of the binding kinetics of each receptor-ligand and receptor-antibody complexes via computer-aided modelling and prediction is needed. This study is done to predict the structure of each biomolecule (homology modelling), identify the interacted residues (docked complexes) and calculate the binding energies, by using a patent-disclosed mouse anti-human CXCR2 HY29-1 single-chain fragment variable antibody.
Research Questions
In order to visualise the complexes, each of the interacted structures (CXCL8, CXCR2 receptor and HY29-1 antibody) must be correctly 3D modelled in silico, whereby nuclear magnetic resonance (NMR) crystal structure is essential in modelling these structures. However, only CXCL8 ligands crystal structure is available but neither antibody nor receptor at the moment. So, de novo 3D structures must be correctly modelled based on their amino acid sequences and compared with experimental 3D structure of a related homologous protein.
Complexes will be made by performing in silico docking of the biomolecules to identify the residues interacted. Since there is no crystal structure of the complexes available, predicted protein-protein docking will be done and the docked poses with the highest ligand/antibody-receptor binding affinity scoring will be taken into consideration. Each residue and epitope-paratope interactions will be identified.
Purpose of the Study
The purpose of this study is to (a) compare the predicted interacted residues between complexes CXCL8-CXCR2 and HY29-1-CXCR2. In order to achieve this, we generate correctly modelled in silico 3D biomolecules, based on the crystal structure (CXCL8 ligands), and homology modelling (CXCR2 receptor & HY29-1 antibody), and (b) To identify the interacted residues within the complexes. Each biomolecule is docked with the CXCR2 receptor. A docked pose with the highest affinity scoring is selected, and each amino acid residues of the components are identified. Then, the interacted residues between complexes are compared.
Research Methods
For the generation of a CXCR2 3D model, we employed GPCR-I-TASSER web server (Yang Zhang Research Group, Department Computational Medicine and Bioinformatics, University of Michigan) using sequence obtained from Universal Protein Resource (UniProt), a publicly available resource database for protein sequence and annotation data. We used Schrödinger Release 2018-4 Bioluminate Suite (Schrödinger, LLC) for further modelling, docking and protein-protein interaction analysis of each biomolecule and complexes. CXCL8 ligand species were sourced from The Protein Data Bank (PDB), an open-access digital data resource in all of biology and medicine. All in silico analysis and rendering was made on a DELL OptiPlex 7010 desktop computer equipped with Intel® Core™ i7-3770 CPU @ 3.40GHz ×8 with 15GB RAM and a 480GB solid-state hard disk. The operating system used is 64-bit Zorin OS 15 LTS Core.
Proteins
Human CXCR2 receptor, its ligands CXCL8 species and mouse anti-human CXCR2 HY29-1 single-chain fragment variable (scFv) antibody (designed by MedImmune, AstraZeneca) are chosen for their demonstrable agonist/antagonistic effects, reagent and test assay availability. The ligands, CXCL8 existed in two species: monomer (PDB #5WDZ) and dimer (PDB #1IL8) were included in the experiment. Only CXCL8 ligands crystal structure is available. Thus, 3D modelling of both human CXCR2 and HY29-1 antibody must be rendered using homology modelling.
Structure preparation
CXCR2 sequence obtained from UniProt (UniProtKB #P25025) was uploaded into GPCR-I-TASSER web server (Yang Zhang Research Group, Department Computational Medicine and Bioinformatics, University of Michigan) for modelling. Resultant model file was imported into Schrödinger Bioluminate Suite (Schrödinger, LLC) using Import Structure function. The HY29-1 scFv sequence was obtained from a patent file (WIPO #WO2015/169811A2) and imported into Schrödinger Bioluminate Suite using Antibody Modelling – Prediction toolkit as described in Schrödinger Antibody Visualization and Modeling in Bioluminate Suite tutorial. CXCL8 ligand species were modelled by importing its monomer (PDB #5WDZ) and dimer (PDB #1IL8) into Schrödinger Bioluminate Suite using Import Structure function. The antibody complementarity-determining region (CDR) was determined by using Martin (Enhanced Chothia) numbering scheme on Multiple Sequence Viewer toolkit. Essentially, under the Annotation tool on the toolbar, Antibody CDRs was checked, and Antibody Numbering Scheme was selected with Enhanced Chothia checked. The application identified the CDR sequence automatically. For ease of viewing, the sequences and CDR annotations were uploaded onto Benchling.com web application.
Receptor-Ligand/ Antibody Docking
Before docking, all biomolecules were prepared by using Protein Preparation Wizard toolkit. This process consists of Preprocess and Refine steps. Under the Preprocess step, the following keys are included: Bond order assigned, Conserved Domain Database (CCD, NCBI) used, hydrogen atoms added, zero-order bonds to metal added, disulfide bonds created. Water more than 5.00 Å from het groups was removed, and Het states were generated using Epik toolkit at pH 7.0 ± 2.0. Under the Refine step, we optimised the hydrogen bond assignment with the following keys included: sample water orientation and use of PROPKA toolkit with pH 7.0. Finally, Restrained Minimization was done by converging heavy atoms to 0.30 Å using repeat searcher and motif detector (RSMD) toolkit. The resultant protein was pre-processed, hydrogen-bond optimised and minimised.
Receptor-Ligand/Antibody Docking was done using Protein-Protein Docking toolkit. The possible poses of docked protein were set at maximum 30 (system default). An exception for CXCR2-CXCL8 species docking, an additional Repulsion Constraint rule was added before the generation of docked poses as follows: Residue number (between 1-360) 54-100,126-178,214-268,300-360. Each pose generated was numbered 1-30 for identification. The resultant poses consisted of docked protein as one complex.
Ligand-Receptor Binding Affinity Scoring
Each chain of protein was split from a single pose of docked complex molecule. Particularly for HY29-1-CXCR2 complex, the resultant VH and VL chains were merged into a single fragment variable molecule and labelled as ‘Fv’, while its docked receptor was labelled as ‘receptor’. Both molecules were selected for estimation of ligand-receptor binding affinity scoring by using Prime Visualize Energy toolkit. The molecular mechanics energies combined with generalised Born and surface area continuum solvation (MM-GBSA) by the binding of free energy (ΔGbind) or MM-GBSA dG(bind) were chosen for estimation of binding affinity scoring. Solvation model of VSGB setting was selected.
Protein Interaction Analysis
Similar to ligand-receptor binding affinity scoring, both molecules (ligand/antibody and receptor) were selected and submitted for Protein Interaction Analysis into separate groups: ligand/antibody in Group 1 while receptor in Group 2. The interactions (hydrogen bonds, salt bridges, π-π stacking interactions and van der Waals clashes) between residues of each group were taken into consideration.
Findings
CXCR2 receptor and HY29-1 antibody were chosen for this study for their demonstrated (1) in vivo submicromolar-range of efficacy in mediating antagonistic profile against CXCL8 and CXCL1 at varying concentration (Carroll, Rossant, & Barry, 2015; Rossant et al., 2014), and (2) availability of sequence and its assay performance data (Carroll et al., 2015). Both species of human CXCL8 were included because both can reversibly exist in vivo, and each has distinct binding kinetics to CXCR2 (Das et al., 2010). Except for CXCL8 species, since there was no available crystal structure for the receptor, antibody, nor complexes currently, this opportunity was taken to study the biomolecules’ potential binding kinetics using de novo homology modelling.
CXCR2 is a seven trans-membrane, G protein-coupled receptor which belongs to the CXCR family and primary receptor for ELR-CXC chemokines that mediate angiogenesis (Liu et al., 2016). It is generally expressed in most circulating innate immune and endothelial cells and its signalling is implicated in the innate response to infection, inflammation and wound healing. It is also found to mediate tumour angiogenesis, growth, metastasis and chemo-resistance in majority of solid cancer cells (Cheng, Ma, Wei, & Wei, 2019). CXCL8, or known as interleukin 8, belongs to the elastin-like re-combinamer (ELR)+ CXC chemokine family (Liu et al., 2016). Despite having a moderate affinity towards CXCR2, it is the most abundant chemokine in chronic inflammation and almost absent in healthy individuals (Cheng et al., 2019). In the present study, a 3D model of CXCR2, CXCL8 (monomer & dimer), and HY29-1 antibody was successfully generated (Figure
![T3D modelling of biomolecules (A. CXCR2 receptor, B. CXCL8 monomer
[5WDZ], C. dimer [1IL8] and D. HY29-1 scFv antibody)](https://www.europeanproceedings.com/files/data/article/1025/10342/ICMR2019F15.fig.001.jpg)
Before rendering and analysis, each biomolecule must be optimised and minimised to fix any structural errors and to ensure structural correctness (Schrödinger Release 2019-2, 2019b). Docking of biomolecules was done to predict the structural conformation of a given complex by focusing on steric and physicochemical complementarity between protein-protein interface (Schrödinger Release 2019-2, 2019a). Since CXCL8 species are extracellular chemokines, a specific repulsion constraint rule was included to prevent any potential unwanted docking of CXCL8 away from N-terminal or extracellular loops. In this study, a maximum of 30 potential docking poses were computed and a single pose with the highest affinity scoring was chosen. The resultant docking poses of each complex is as represented (Figure
A distinct difference in binding affinity scores was seen between monomer and dimer of CXCL8 ligand towards the CXCR2 receptor. A comparable binding affinity score is also seen in HY29-1 antibody towards the CXCR2 receptor. Further, the CDR residues of HY29 (Figure
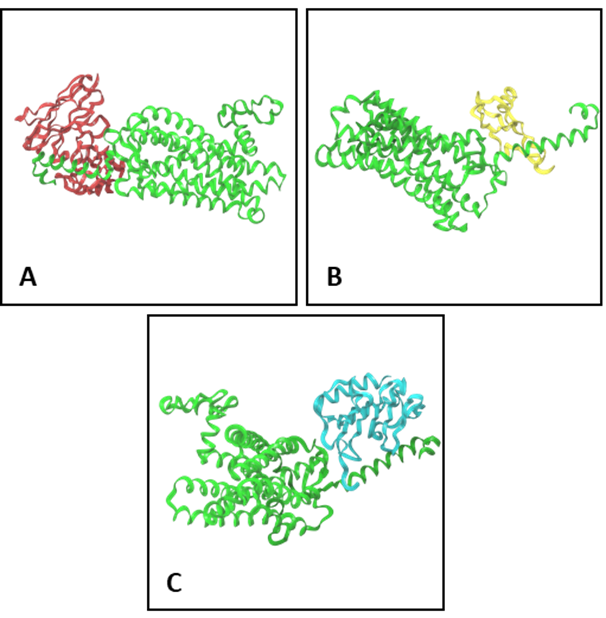
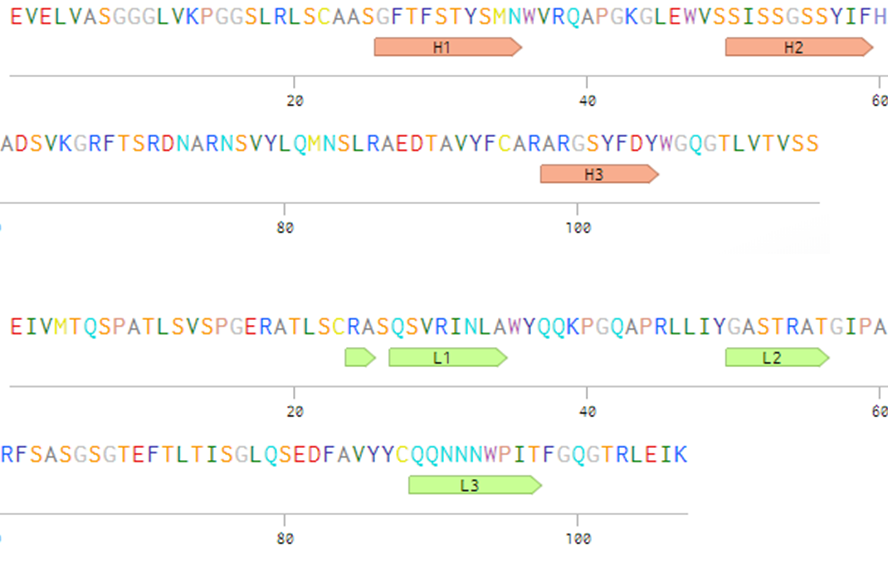
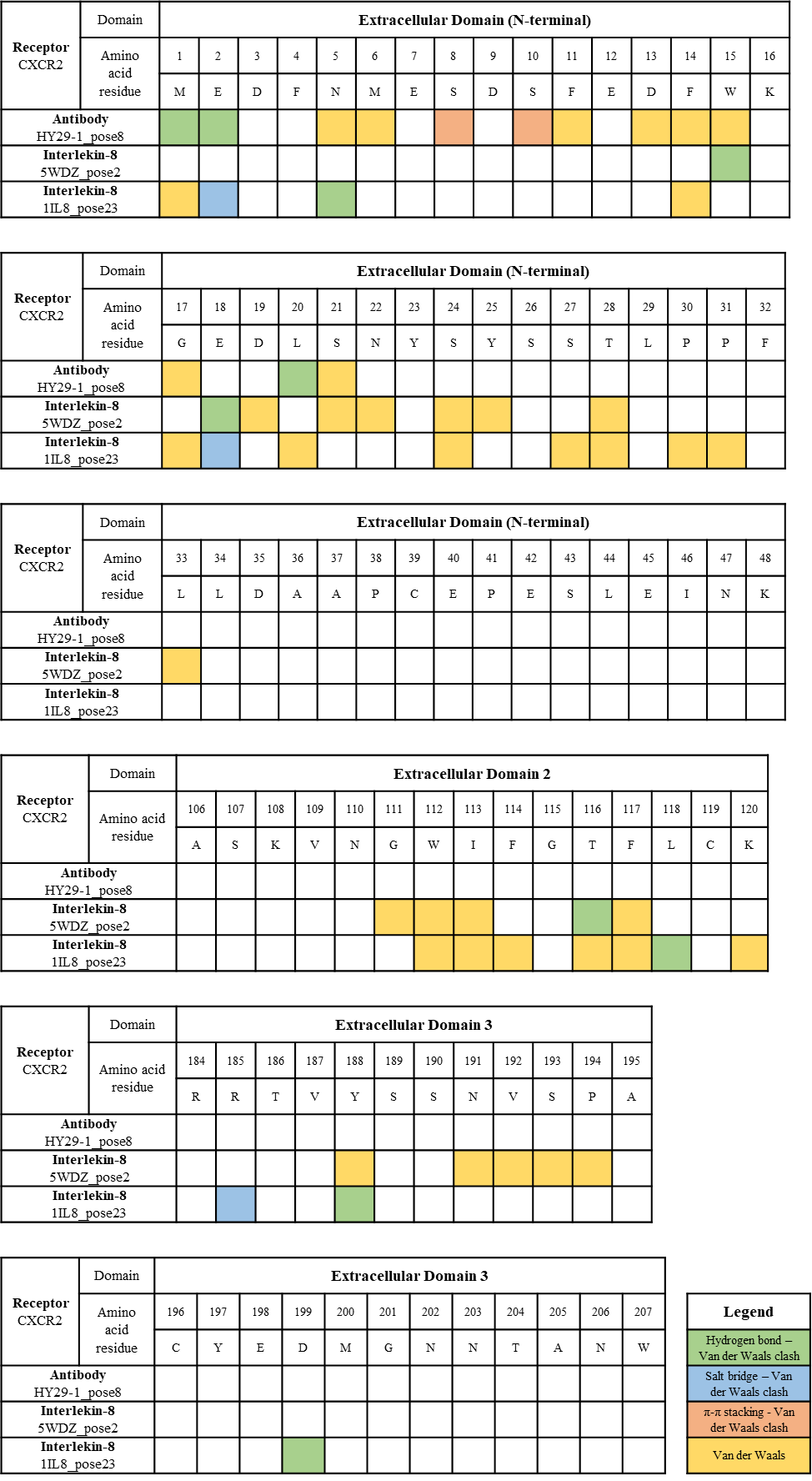
Our data showed all three biomolecules (monomer & dimer of CXCL8 and HY29-1 antibody) were mostly bound to N-terminal of the CXCR2 receptor. HY29-1 antibody interacted residues were confined between amino acid 1-20 of N-terminal. 5WDZ interacted residues spanned partly on N-terminal (amino acid 15-33), Extracellular Domain 2 (amino acid 111-117), and Extracellular Domain 3 (amino acid 188-194) while 1IL8 spanned between N-terminal (amino acid 1-31), Extracellular Domain 2 (amino acid 112-120) and Extracellular Domain 3 (185-188).
Although all three biomolecules were bound to specific residues on CXCR2, shared residues between these three biomolecules may suggest competitive binding kinetics between CXCL8 and HY29-1. In addition, the distinct pattern of interacted residues may define the antagonistic/agonistic effects, and their specific bonds may suggest the intensity or duration of the antagonistic/agonistic effects onto the CXCR2 receptor. Our data warrants for further study in understanding the relationship between the interaction among critical residues and their pharmacodynamics effects.
Conclusion
Predicted interacted residues between CXCR2 receptor and CXCL8 ligands & HY29-1 single-chain antibody were dentified. Distinct differences in binding affinity scoring and interacted residues may be implicated in defining the extent of antagonistic or agonistic effects of the CXCL8 ligands and HY29-1 antibody towards CXCR2 receptor.
Acknowledgments
This study was supported by Universiti Sains Malaysia Short Term Grant (304/PJJAUH/6315185), and also sponsored by “Tabung Kumpulan Wang Siswazah Pusat Pengajian Pendidikan Jarak Jauh USM”.
References
- Carroll, D., Rossant, C., & Barry, S. T. (2015). Patent No. WO 2015/169811 A2. World Intellectual Property Organization. Retrieved from https://patentscope.wipo.int/search/en/-detail.jsf?docId=WO2015169811
- Chen, M. C., Baskaran, R., Lee, N. H., Hsu, H. H., Ho, T. J., Tu, C. C., …& Huang, C. Y. (2019). CXCL2/CXCR2 axis induces cancer stem cell characteristics in CPT-11-resistant LoVo colon cancer cells via Gαi-2 and Gαq/11. Journal of Cellular Physiology, 234(7), 11822–11834. https://doi.org/10.1002/jcp.27891
- Cheng, Y., Ma, X., Wei, Y., & Wei, X. W. (2019). Potential roles and targeted therapy of the CXCLs/CXCR2 axis in cancer and inflammatory diseases. Biochimica et Biophysica Acta - Reviews on Cancer, 1871(2), 289–312. https://doi.org/10.1016/j.bbcan.2019.01.005
- Das, S. T., Rajagopalan, L., Guerrero-Plata, A., Sai, J., Richmond, A., Garofalo, R. P., & Rajarathnam, K. (2010). Monomeric and dimeric CXCL8 are both essential for in vivo neutrophil recruitment. PLoS ONE, 5(7), e11754. https://doi.org/10.1371/journal.pone.0011754
- Dyer, D. P., Nebot, J. B., Kelly, C. J., Medina-Ruiz, L., Schuette, F., & Graham, G. J. (2019). The chemokine receptor CXCR2 contributes to murine adipocyte development. Journal of Leukocyte Biology, 105(3), 497–506. https://doi.org/10.1002/JLB.1A0618-216RR
- Imafuji, H., Matsuo, Y., Ueda, G., Omi, K., Hayashi, Y., Saito, K., …& Takiguchi, S. (2019). Acquisition of gemcitabine resistance enhances angiogenesis via upregulation of IL‑8 production in pancreatic cancer. Oncology Reports, 41(6), 3508–3516. https://doi.org/10.3892/or.2019.7105
- Liao, W., Overman, M. J., Boutin, A. T., Shang, X., Zhao, D., Dey, P., … & DePinho, R. A. (2019). KRAS-IRF2 Axis Drives Immune Suppression and Immune Therapy Resistance in Colorectal Cancer. Cancer Cell, 35(4), 559-572.e7. https://doi.org/10.1016/j.ccell.2019.02.008
- Liu, Q., Li, A., Tian, Y., Wu, J. D., Liu, Y., Li, T., …& Wu, K. (2016). The CXCL8-CXCR1/2 pathways in cancer. Cytokine and Growth Factor Reviews, 31, 61–71. https://doi.org/10.1016/j.cytogfr.2016.08.002
- Ogawa, R., Yamamoto, T., Hirai, H., Hanada, K., Kiyasu, Y., Nishikawa, G., … & Kawada, K. (2019). Loss of SMAD4 promotes colorectal cancer progression by recruiting tumor-associated neutrophils via the CXCL1/8–CXCR2 axis. Clinical Cancer Research, 25(9), 2887–2899. https://doi.org/10.1158/1078-0432.CCR-18-3684
- Peterson, G. M., Thomas, J., Yee, K. C., Kosari, S., Naunton, M., & Olesen, I. H. (2018). Monoclonal antibody therapy in cancer: When two is better (and considerably more expensive) than one. Journal of Clinical Pharmacy and Therapeutics, 43(6), 925–930. https://doi.org/10.1111/jcpt.12750
- Rossant, C. J., Carroll, D., Huang, L., Elvin, J., Neal, F., Walker, E., … & Vaughan, T. J. (2014). Phage display and hybridoma generation of antibodies to human CXCR2 yields antibodies with distinct mechanisms and epitopes. MAbs, 6(6), 1425–1438. https://doi.org/10.4161/mabs.34376
- Ruffini, P. A. (2019). The CXCL8-CXCR1/2 Axis as a Therapeutic Target in Breast Cancer Stem-Like Cells. Frontiers in Oncology, 9, 40. https://doi.org/10.3389/fonc.2019.00040
- Schrödinger Release 2019-2. (2019a). BioLuminate. Retrieved July 1, 2019, from Schrödinger, LLC websit: https://www.schrodinger.com/products/bioluminate
- Schrödinger Release 2019-2. (2019b). Protein Preparation Wizard. Retrieved July 1, 2019, from Schrödinger, LLC website: https://www.schrodinger.com/protein-preparation-wizard
- Sefid, F., Payandeh, Z., Azamirad, G., Abdolhamidi, R., & Rasooli, I. (2019). In Silico Engineering Towards Enhancement of Bap–VHH Monoclonal Antibody Binding Affinity. International Journal of Peptide Research and Therapeutics, 25(1), 273–287. https://doi.org/10.1007/s10989-017-9670-9
- Sharma, B., Nannuru, K. C., Saxena, S., Varney, M. L., & Singh, R. K. (2019). CXCR2: A novel mediator of mammary tumor bone metastasis. International Journal of Molecular Sciences, 20(5), 1237. https://doi.org/10.3390/ijms20051237
- Takeyama, Y., Kato, M., Shimizu, Y., Hamada, K., Iguchi, T., Tamada, S., & Nakatani, T. (2019). Development of myeloid-derived suppressor cells (MDSC) targeted therapy for the treatment of cisplatin-resistant bladder cancer. Journal of Clinical Oncology, 37(7_suppl), 415–415. https://doi.org/10.1200/jco.2019.37.7_suppl.415
- Tiller, K. E., & Tessier, P. M. (2015). Advances in Antibody Design. Annual Review of Biomedical Engineering, 17(1), 191–216. https://doi.org/10.1146/annurev-bioeng-071114-040733
- Wang, Y., Tu, L., Du, C., Xie, X., Liu, Y., Wang, J., … & Luo, F. (2018). CXCR2 is a novel cancer stem-like cell marker for triple-negative breast cancer. OncoTargets and Therapy, 11, 5559–5567. https://doi.org/10.2147/OTT.S174329
- Zhai, J., Shen, J., Xie, G., Wu, J., He, M., Gao, L., …& Shen, L. (2019). Cancer-associated fibroblasts-derived IL-8 mediates resistance to cisplatin in human gastric cancer. Cancer Letters, 454, 37–43. https://doi.org/10.1016/j.canlet.2019.04.002
Copyright information
This work is licensed under a Creative Commons Attribution-NonCommercial-NoDerivatives 4.0 International License.
About this article
Publication Date
30 March 2020
Article Doi
eBook ISBN
978-1-80296-080-8
Publisher
European Publisher
Volume
81
Print ISBN (optional)
-
Edition Number
1st Edition
Pages
1-839
Subjects
Business, innovation, sustainability, development studies
Cite this article as:
Nordin, M. A. C., Hamid, S. B. S., Wajidi, M. F. F., & Omar, M. T. C. (2020). In Silico Visualization And Modelling Of Cxcr 2Recombinant Antibody (Anti-Cxcr2 Fv) Complex. In N. Baba Rahim (Ed.), Multidisciplinary Research as Agent of Change for Industrial Revolution 4.0, vol 81. European Proceedings of Social and Behavioural Sciences (pp. 115-124). European Publisher. https://doi.org/10.15405/epsbs.2020.03.03.15