Abstract
Information and communication technologies are fundamentally changing the sphere of education in modern society. Online education is evolving and offering new opportunities for students’ data analysis. At the same time, there is a certain barrier in the interaction of specialists of different professions engaged in data analysis in online learning. The purpose of this article is to reduce this barrier by giving a brief analysis of the main directions of development and the possibility of using data in online learning and showing what results could be expected from the presented methods now and in the near future. This study presents the priority areas of learning analytics such as tracking students at risk, analysis of the most effective options for the course (face to face, blended, online), analysis of the relationship of student success from the key characteristics of the course and the behavior of students, the improvement of students’ assessment. The article also discusses the options for using data on the behavior of students in an individual and generalized form.
Keywords: E-learningstudent’s behaviorlearning analyticsonline course
Introduction
Information and communication technologies have changed dramatically human social life (Gashkova, Berezovskaya, & Shipunova, 2017; Kolomeyzev & Shipunova, 2017; Pokrovskaia, Ababkova, Leontieva, & Fedorov, 2018; Razinkina et al., 2018; Shipunova, & Berezovskaya, 2018; Spihunova, Rabosh, Soldatov, & Deniskov, 2017). Education is one of the areas where change is especially noticeable.
Online courses have firmly taken their place in the education system. Comprehension and evaluation of different forms of this phenomenon occurs by different methods, for example, with the help of sociological and expert surveys (Dziuban, Graham, Moskal, Norberg, & Sicilia, 2018; Göcks & Baier, 2003; Katz, 2002; Sezer, & Yilmaz, 2004; Xhaferi, 2018) or comparison of academic achievement (Han, & Ellis, 2019). There are studies on the impact of students’ demographic (Golband, Hosseini, Mojtahedzadeh, Mirhosseini, & Bigdeli, 2014; Kotsiantis, 2012) and psychological data such as positive self-concept, realistic self-appraisal, preference for long-term goals, leadership experience, community involvement, and knowledge acquired by individual’s preferred learning style (Sedlacek, 2004), higher level of independence in the learning process (Katz, 2002), attitudes of students towards technologies (Xhaferi, 2018), readiness to online learning (Akaslan & Law, 2011) and others on the success of online learning.
However, online education offers new opportunities to analyze the learning process, due to the use of the latest technological advances. Today, there are systems allowing to fully track the behavior of students during online education. Moreover the Method of biological feedback is used to evaluate student’s psychophysiological state and it can include body position and facial expression, sight direction (Eye Tribe), monitors of the level of physical activeness, different parameters of the electroencephalogram (EEG) of the brain (amplitude, power, coherence), measuring the values of the vegetative (sympathicparasympathetic) activation (skin conductivity, cardiogram, pulse frequency, electromyogram, temperature, photopletizmogram etc.), levels of mental stress, и others (Ababkova & Leontyeva, 2018; Ababkova, Pokrovskaia, & Trostinskaya, 2018; Sánchez Barragán, Solarte Moncayo, & Chanchí Golondrino, 2019).
Researchers have long pointed to the need for the expansion of the use of intelligence revealed by the analytics process from Learning Management Systems (LMSs) (Kalmykova, Pustylnik, & Razinkina, 2017; Macfadyen & Dawson, 2012; Ruipérez-Valiente, Muñoz-Merino, Leony, & Delgado Kloos, 2015). Furthermore, if attempts to organize the control of physiological and mental characteristics of behavior during offline training is quite controversial from an ethical point of view, so the online control does not bring any discomfort to students, although it also requires a consent to such control
Problem Statement
A huge amount of information about student learning behaviors and activities are retrieved from learning system logs or databases, creates the illusion of control. However, the question of how and for what purpose it can be used remains open.
Research Questions
The study considers the following key issues:
to find what data and for what purposes are used for online course data analytics;
to analyze and classify existing approaches to analytics;
to define the main ways to develop the use of data from learning analytics
Purpose of the Study
The aim of the study is to identify the main areas of application of analytical data on student behavior in the electronic course.
Research Methods
The study used a wide range of general scientific and special research methods, investigated the experience of 30 universities on the example of cases published in open sources. To form an idea of these online courses implementation in Russian universities the sociological methods were also used in the work (such as expert interviewing and participant observation). The results of the observation and interviewing are of scientific novelty, as in modern sociological practice there are no studies of Russian universities on the subject presented by these methods. When forming approaches to the analytics of online courses, the authors assumed the fact that this concept has a conditional character and is associated with the organization of educational activities and interaction of the teacher and IT-specialist based on a certain idea and principles of learning. The experience of application considered by authors allowed to define the main groups of data on students on online courses and to allocate a number of priority directions for the analysis of students’ behavior. In this study, we used an interdisciplinary approach, which allowed us to consider analytical tools in online education as a complex multidimensional process.
Findings
We can track the following major groups of data of students’ behavior:
number and success of all attempts to complete course tasks;
time/number of course visits, or specific different assignments;
viewing video lectures (e.g., how much time from which lecture was viewed)
the sequence of actions on the course (process mining);
all activities of each student in the course (in video form);
motion tracking (e.g., eye‐tracking);
a set of all student’s actions (keystrokes, mouse gestures and clicks) at the computer during the course (for example, using the keyboard shortcuts such as Ctrl+Copy and Ctrl+Paste while doing tasks figure
01 );analysis of social interaction on communication platforms
semantic analysis.
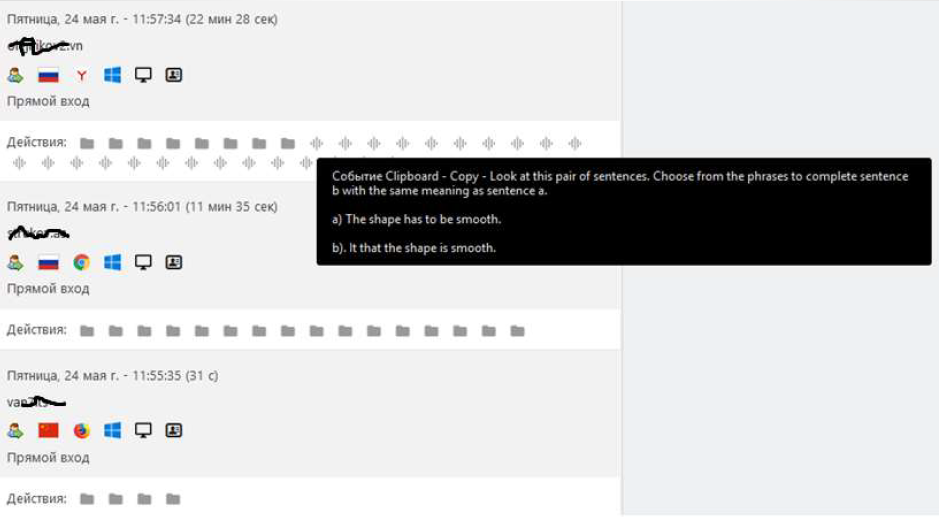
Today there are several priority areas of analysis of student behavior in the course.
tracking students at risk group (regarding the successful completion of the course)
The formation of the early warning system is an interesting task at the intersection of pedagogy and computer science. The construction of such a reliable predictive model can provide a new tool for a teacher in Moodle (Gaudioso, Montero, & Hernandez-Del-Olmo, 2012) or Massive Open Online Courses (Tabaa & Medouri, 2013). Hu, Lo and Shih (2014) use online course time-dependent variables for building automatic notification system for students instructor through the email and the user interface in LMS on probability ‘‘fail the course’analysis of the most effective course options (for example, the comparison of success rates face to face, blended, on-line)
The possibility of different combinations of online and offline elements of the course is at the center of studies for different courses from the point of view of time (Al-Qahtani & Higgins, 2013; Owston, & York, 2018) and the material being studied (Caron, Caron, Visentin, & Ermondi, 2011). Tomkins, Ramesh, & Getoor (2016) pay attention to the role of a teacher in a student’s online learning.
Analysis of the dependence of success on the key characteristics of the course or supporting resources (for example, various options for building communication schemes or the use of social networks)
Numerous studies point to the importance of social interaction during online learning (Al-Rahmi, Alias, Othman, Marin, & Tur, 2018; Davies & Graff, 2005; Gao & Lehman, 2003; Zhu, 2012). The role of connectedness is particularly emphasized, that can be supported by social networks (Alderson & Lowther, 2014), the features needed to build quality learning discussions in online learning are also highlighted (Han & Ellis, 2019).
Improving the assessment of the student
An appropriate assessment of the student's work on the electronic course can be complicated due to the distance between the teacher and the student. Special tools are required for individual assessment of student collaborative activities (Aouine, Mahdaoui, & Moccozet, 2019). A separate problem is online cheating in different forms. For example, at the Universitas Terbuka in Indonesia Farisi (2013) indicates the emergence of “examjockeys”, namely someone who paid to impersonate another student and look to replace their own exams (p. 181).
Analysis of the dependence of the success of the course on the behavior of students
The parameters that are most often extracted from the learning analytics system are: the time spent on the various elements of the course, the number of references to them, the click stream sequence in which students have accessed certain elements (in different combinations), and the data of communication (the length of the posts on the forum, the frequency of interactions between users, etc.) could be separately analyzed.
It would seem that the use of these data opens up tremendous predictive possibilities. But it turns out that despite the variety of data and their obvious relationship to learning outcomes, analyzing them becomes a challenge.
For instance, the time devoted to a course intuitively seems to be closely related to the level of engagement and likelihood of success. However, for example, a study by Macfadyen and Dawson (2010) shows that those students, who received the lowest grade, on average spent a little more time on the course than those, who received the highest grade (p.593). And a study by Kushwaha, Singhal, & Swain (2018) demonstrates that high achiever students take average time passing a quiz, while slow responder students take maximum and low achievers (p. 476).
Only the relationships of quite predictable indicators are statistically confirmed such as:
in terms of the number of accesses to elements of the course - the number of quizzes passed predicts the final mark (Romero, Espejo, Zafra, Romero, & Ventura, 2013) or that low level of contributions to the course learning activities leads to fail (Helal et al., 2019).in the area of time spent - with the help of Rip, the Ripper implementation in Weka it could be predicted that with a low initial level of knowledge and minimal time spent, the student will receive a low mark (Gaudioso, Montero, & Hernandez-del-Olmo, 2012, p. 624).when analyzing the sequence of activities on the course - failed students who began their interaction with the course with the test, and not with other activities (Bogarín, Cerezo, & Romero, 2018), students who watch videos regularly and in batch are more likely to perform better than those who skip videos or procrastinate in watching videos (Mukala, Buijs, Leemans, & van der Aalst, 2015, p. 32)
The search for new opportunities for data analysis in online learning continues. In particular, Coleman, Seaton, & Chuang (2015) offer to use modeling approach of latent Dirichlet allocation to predict the success of the course. Another approach is in modeling, based on the summation of LMS data- key types of behavior in the course (Gelman, Revelle, Domeniconi, Johri, & Veeramachaneni, 2016).
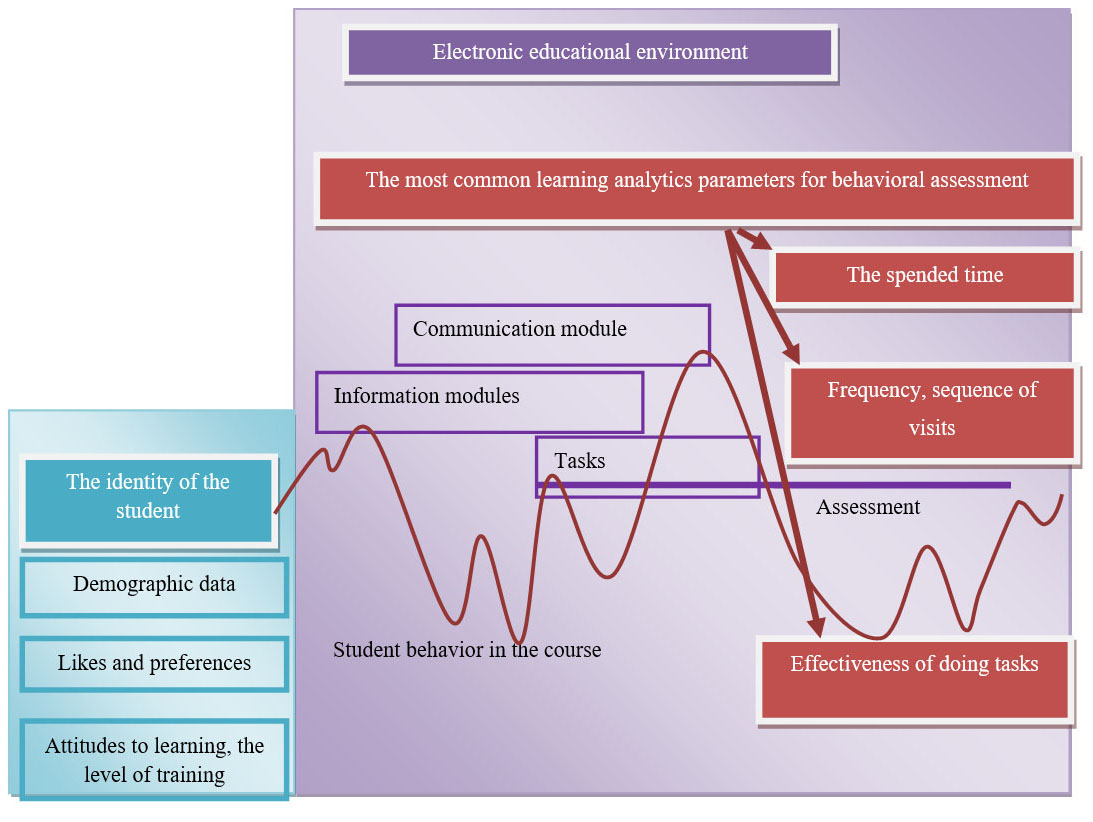
In Figure
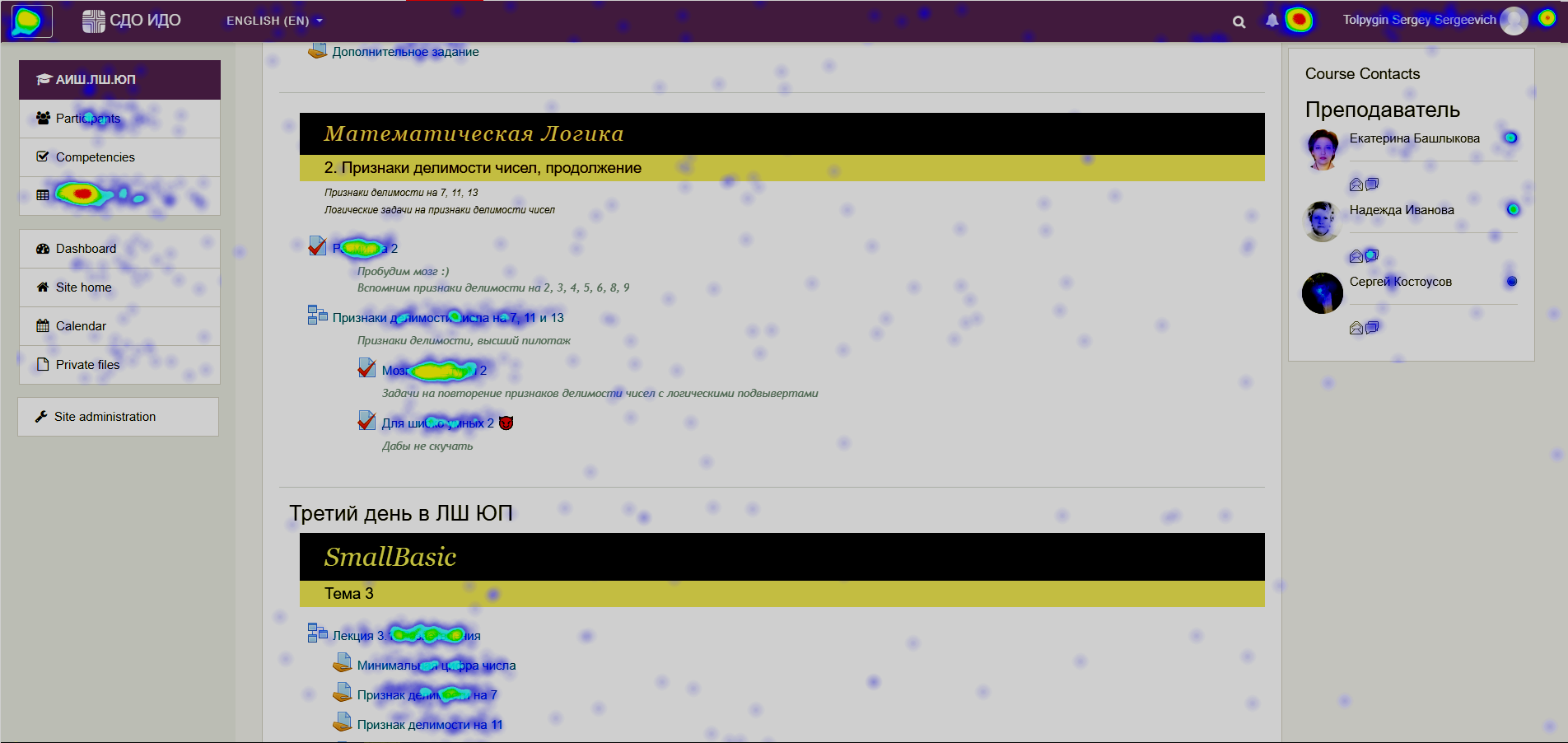
Conclusion
Online education continues to develop and improve, and along with increasing opportunities to analyze the behavior of students. It should be noted that there is a certain barrier in the interaction of specialists of different professions involved in data analysis in online learning. On the one hand, educators are not always ready to interpret the extensive data provided by the learning system. On the other hand, data analysts often form queries that do not fully meet the interests of teachers and course developers due to the lack of universal communication tools in the analysis of such data.
However, we can already identify the main ways to develop and use learning data:
In a generalized form, the data can be used:
-
to quickly change the content of the course;
-
to improve the design of the architecture of the teaching processes and content of the course;
-
to develop common approaches to the elaboration of online courses
In individual form:
-
for tutor assistance to students at risk;
-
to adapt the course materials to the peculiarities of student behavior
-
to track the most complex topics that require additional work
to track unethical behavior of students
References
- Ababkova, M., & Leontyeva, V. (2018). Neurobiological studies within the framework of highly technological teaching. IV International Scientific Conference “The Convergence of Digital and Physical Worlds: Technological, Economic and Social Challenges” (CC-TESC2018). SHS Web of Conferences. Vol. 44, 00002.
- Ababkova, M. Y., Pokrovskaia, N. N., & Trostinskaya, I. R. (2018). Neuro-technologies for knowledge transfer and experience communication. European Proceedings of Social & Behavioural Sciences, 35, 10-18.
- Akaslan, D., & Law, E. L. C. (2011). Measuring Student E-Learning Readiness: A Case about the Subject of Electricity in Higher Education Institutions in Turkey. In H. Leung, E. Popescu, Y. Cao, R. W. H. Lau, & W. Nejdl (Eds.), Advances in Web-Based Learning - ICWL 2011. ICWL 2011. Lecture Notes in Computer Science, vol 7048. (pp 209-218). Springer, Berlin, Heidelberg
- Alderson, L. L., & Lowther, D. L. (2014). Factors That May Influence Instructors’ Choices of Including Social Media When Designing Online Courses. In S. Michael (Ed.), 37th Annual Proceedings (pp. 205–211). North Miami Beach, USA: ERIC Retrieved from https://files.eric.ed.gov/fulltext/ED562048.pdf
- Al-Qahtani, A. A. Y., & Higgins, S. E. (2013). Effects of traditional, blended and e-learning on students’ achievement in higher education. Journal of Computer Assisted Learning, 29(3), 220–234.
- Al-Rahmi, W. M., Alias, N., Othman, M. S., Marin, V. I., & Tur, G. (2018). A model of factors affecting learning performance through the use of social media in Malaysian higher education. Computers & Education, 121, 59–72.
- Aouine, A., Mahdaoui, L., & Moccozet, L. (2019). A workflow-based solution to support the assessment of collaborative activities in e-learning. International Journal of Information and Learning Technology. https://doi.org/10.1108/ijilt-01-2018-0004
- Bogarín, A., Cerezo, R., & Romero, C. (2018). Discovering learning processes using inductive miner: A case study with learning management systems (LMSs). Psicothema, 30(3), 322–329.
- Caron, G., Caron, G., Visentin, S., & Ermondi, G. (2011). Blended-learning for courses in Pharmaceutical Analysis. Journal of E-Learning and Knowledge Society, 7(2), 93–102. Retrieved from https://www.learntechlib.org/p/43283/
- Coleman, C. A., Seaton, D. T., & Chuang, I. L. (2015). Probabilistic Use Cases: Discovering Behavioral Patterns for Predicting Certification. In G. Kiczales, D. M. Russell, & B. P. Woolf (Eds.), Proceedings of the Second ACM Conference on Learning, (pp. 141–148). Vancouver, BC: ACM.
- Davies, J., & Graff, M. (2005). Performance in e-learning: online participation and student grades. British Journal of Educational Technology, 36(4), 657–663.
- Dziuban, C., Graham, C. R., Moskal, P. D., Norberg, A., & Sicilia, N. (2018). Blended learning: the new normal and emerging technologies. International Journal of Educational Technology in Higher Education, 15(1), 3.
- Farisi, M. I. (2013). Academic dishonesty in distance higher education: Challenges and Models for Moral Education in the Digital Era. Turkish Online Journal of Distance Education-TOJDE, 14 (4), 176-195.
- Gao, T., & Lehman, J. D. (2003). The Effects of Different Levels of Interaction on the Achievement and Motivational Perceptions of College Students in a Web-Based Learning Environment. Journal of Interactive Learning Research, 14(4), 367–386. Retrieved from https://www.questia.com/library/journal/1G1-114743685/the-effects-of-different-levels-of-interaction-on
- Gashkova, E., Berezovskaya, I., & Shipunova, O. (2017). Models of self-identification in digital communication environments. The European Proceedings of Social & Behavioural Sciences, 35, 374-382.
- Gaudioso, E., Montero, M., & Hernandez-del-Olmo, F. (2012). Supporting teachers in adaptive educational systems through predictive models: A proof of concept. Expert Systems with Applications, 39(1), 621–625.
- Gelman, B., Revelle, M., Domeniconi, C., Johri, A., & Veeramachaneni, K. (2016). Acting the Same Differently: A Cross-Course Comparison of User Behavior in MOOCs. In T. Barnes, M. Chi, & F. Mingyu (Eds.), Proceedings of the 9th International Conference on Educational Data Mining (pp. 376–381). Raleigh, USA: IEDMS.
- Göcks, M., & Baier, D. (2003). Students’ Preferences Related to Web Based E-Learning: Results of a Survey. In M. Schader, W. Gaul, & M. Vichi (Eds), Between Data Science and Applied Data Analysis. Studies in Classification, Data Analysis, and Knowledge Organization (pp 430-437). Berlin, Heidelberg: Springer.
- Golband, F., Hosseini, A. F., Mojtahedzadeh, R., Mirhosseini, F., & Bigdeli, S. (2014). The correlation between effective factors of e-learning and demographic variables in a post-graduate program of virtual medical education in Tehran University of Medical Sciences. Acta Medica Iranica, 52(11), 860–864. Retrieved from https://www.semanticscholar.org/paper/The-correlation-between-effective-factors-of-and-in-Golband-Hosseini/345f1718b3bc73291646d616c68a83845a88a040
- Han, F., & Ellis, R. A. (2019). Identifying consistent patterns of quality learning discussions in blended learning. The Internet and Higher Education, 40, 12–19.
- Helal, S., Li, J., Liu, L., Ebrahimie, E., Dawson, S., & Murray, D. J. (2019). Identifying key factors of student academic performance by subgroup discovery. International Journal of Data Science and Analytics, 7(3), 227–245.
- Hu, Y. H., Lo, C. L., & Shih, S. P. (2014). Developing early warning systems to predict students’ online learning performance. Computers in Human Behavior, 36, 469–478.
- Kalmykova, S. V., Pustylnik, P. N, & Razinkina, E. M. (2017). Role Scientometric Researches’ Results in Management of Forming the Educational Trajectories in the Electronic Educational Environment. In M. E. Auer, D. Guralnick, & J. Uhomoibhi (Eds.), Interactive Collaborative Learning. Proceedings of the 19th ICL Conference. Vol. 2 (pp. 427–432). Belfast, UK: Springer.
- Katz, Y. J. (2002). Attitudes affecting college students’ preferences for distance learning. Journal of Computer Assisted Learning, 18, 2–9.
- Kolomeyzev, I., & Shipunova, O. (2017). Sociotechnical system in the communicative environment: management factors RPTSS 2017 International Conference on Research Paradigms Transformation in Social Sciences, The European Proceedings of Social & Behavioural Sciences, 35, 1233-1241.
- Kotsiantis, S. B. (2012). Use of machine learning techniques for educational proposes: A decision support system for forecasting students’ grades. Artificial Intelligence Review, 37(4), 331–344.
- Kushwaha, R. C., Singhal, A., & Swain, S. K. (2018). Learning Pattern Analysis: A Case Study of Moodle Learning Management System. Recent Trends in Communication, Computing, and Electronics, 524, 471–479.
- Macfadyen, L. P., & Dawson, S. (2010). Mining LMS data to develop an “early warning system” for educators: A proof of concept. Computers & Education, 54(2), 588–599.
- Macfadyen, L. P., & Dawson, S. (2012). Numbers Are Not Enough. Why e-Learning Analytics Failed to Inform an Institutional Strategic Plan. Journal of Educational Technology & Society, 15, 149–163.
- Mukala, P., Buijs, J., Leemans, M., & van der Aalst, W. (2015). Learning analytics on coursera event data: a process mining approach. In P. Caravolo & S. Rinderle-Ma (Eds.), Proceedings of the 5th International Symposium on Data-driven Process Discovery and Analysis (SIMPDA 2015) (pp. 18–32). Vienna: CEUR. Retrieved from https://research.tue.nl/en/publications/learning-analytics-on-coursera-event-data-a-process-mining-approa
- Owston, R., & York, D. N. (2018). The nagging question when designing blended courses: Does the proportion of time devoted to online activities matter? The Internet and Higher Education, 36, 22–32.
- Pokrovskaia, N. N., Ababkova, M. Y., Leontieva, V. L., & Fedorov, D. A. (2018). Semantics In E-Communication for Managing Innovation Resistance Within the Agile Approach. 18th PCSF 2018 - Professional Сulture of the Specialist of the Future. The European Proceedings of Social & Behavioural Sciences, 51, 18321842.
- Razinkina, E., Pankova, L., Trostinskaya, I., Pozdeeva, E., Evseeva, L., & Tanova, A. (2018). Student satisfaction as an element of education quality monitoring in innovative higher education institution. E3S Web of Conferences, 33, 03043 (2018).
- Romero, C., Espejo, P. G., Zafra, A., Romero, J. R., & Ventura, S. (2013). Web usage mining for predicting final marks of students that use Moodle courses. Computer Applications in Engineering Education, 21(1), 135–146.
- Ruipérez-Valiente, J. A., Muñoz-Merino, P. J., Leony, D., & Delgado Kloos, C. (2015). ALAS-KA: A learning analytics extension for better understanding the learning process in the Khan Academy platform. Computers in Human Behavior, 47, 139–148.
- Sánchez Barragán, M., Solarte Moncayo, L. A., Chanchí Golondrino, G. E. (2019). Proposal of an Open Hardware-Software System for the Recognition of Emotions from Physiological Variables. In V. Agredo-Delgado, & P. Ruiz P. (Eds), Human-Computer Interaction. HCI-COLLAB 2018. Communications in Computer and Information Science, vol 847 (pp. 199-213). Cham: Springer. DOI
- Sedlacek, W. E. (2004). Beyond the big test: Noncognitive assessment in higher education. San Francisco: Jossey-Bass.
- Sezer, B., & Yilmaz, R. (2004). Australasian journal of educational technology. Australasian Journal of Educational Technology, 35(3), 15–30. Retrieved from https://ajet.org.au/index.php/AJET/article/view/3959/1547
- Shipunova, O. D., & Berezovskaya, I. P. (2018). Formation of the specialist's intellectual culture in the network society. The European Proceedings of Social & Behavioural Sciences, 51, 447-455.
- Spihunova, O., Rabosh, V., Soldatov, A., & Deniskov, A. (2017). Interactions Design in Technogenic Information and Communication Environments. The European Proceedings of Social & Behavioural Sciences, 35, 1225-1232.
- Tabaa, Y., & Medouri, A. (2013). LASyM: A Learning Analytics System for MOOCs. International Journal of Advanced Computer Science and Applications, 4(5), 113-119.
- Tomkins, S., Ramesh, A., & Getoor, L. (2016). Predicting Post-Test Performance from Student Behavior: A High School MOOC Case Study. In T. Barnes, M. Chi, & M. Feng (Eds.), Proceedings of the 9th International Conference on Educational Data Mining, EDM 2016 (pp. 239–246). Raleigh, North Carolina: EDM. Retrieved from http://www.educationaldatamining.org/EDM2016/proceedings /paper_123.pdf
- Xhaferi, G. (2018). Analysis of Students’ Factors Influencing the Integration of E-Learning in Higher Education. Case Study: University of Tetovo. European Journal of Fomal Sciences and Engineering, 1(2), 33–38.
- Zhu, C. (2012). Student Satisfaction, Performance, and Knowledge Construction in Online Collaborative Learning. Journal of Educational Technology & Society, 15, 127–136.
Copyright information
This work is licensed under a Creative Commons Attribution-NonCommercial-NoDerivatives 4.0 International License.
About this article
Publication Date
02 December 2019
Article Doi
eBook ISBN
978-1-80296-072-3
Publisher
Future Academy
Volume
73
Print ISBN (optional)
-
Edition Number
1st Edition
Pages
1-986
Subjects
Communication, education, educational equipment, educational technology, computer-aided learning (CAL), Study skills, learning skills, ICT
Cite this article as:
Bylieva*, D., Lobatyuk, V., Nam, T., & Tolpygin, S. (2019). Possible Applications Of Analytics Data In Online Courses. In N. I. Almazova, A. V. Rubtsova, & D. S. Bylieva (Eds.), Professional Сulture of the Specialist of the Future, vol 73. European Proceedings of Social and Behavioural Sciences (pp. 630-639). Future Academy. https://doi.org/10.15405/epsbs.2019.12.67