Abstract
Cyber-Physical Systems (CPS) is the next generation of advance technology which combines major aspects of control, systems, electronics, and computer science. Currently, this system is considered as the most prominent technologies in the fourth industrial revolution (Industry 4.0); however, it has not been widely adopted especially in Malaysia. Based on report by Ministry of International Trade and Industry (MITI), the manufacturing sector in Malaysia is implementing industrial revolution between 2.0 and 3.0 to date. This is a clear indication that the existing industry is not fully ready for the new and current technology adoption. Realising this, a conceptual model is proposed focusing on the roles played by technological, organizational, and environmental characteristics of manufacturing firms (following the Technology-Organization-Environment (TOE) framework) in influencing their intention to adopt CPS technology. The TOE framework is extended in this model with two additional variables, namely, government policy and government incentive that are thought to play a moderating roles in the relationship between organizational characteristic (financial and technology readiness) of the manufacturing firms and the firms’ intention to adopt CPS technology.
Keywords: Cyber-Physical Systems (CPS)Manufacturing firmsGovernment policyIncentiveIndustry 40Technology-Organization-Environment (TOE) framework
Introduction
The Fourth Industrial Revolution or better known as Industry 4.0 has become a global buzzword since it was first introduced by the Germans in 2011. It was subsequently spreading to other countries as the technology advances and becomes complex (Götz & Jankowska, 2017). According to Schwab (2017), the term Industry 4.0 is the extension of previous industrial revolutions which started in the second half of the eighteenth century with the introduction of mechanical production. The second revolution followed in the 1870s after the electrification and the division of labour was introduced. The industry further evolved with the third phase of revolution in the 1970s with the adoption of information and communication technologies (ICT) and advanced electronics. To date, the industry is undergoing the latest stage of revolution, namely, Industry 4.0 which is built based on the operation of Cyber-Physical Systems (CPS) (Kagermann, Wahlster, & Helbig, 2013).
In brief, Cyber-Physical Systems (CPS) represents the next generation of advanced technology whereby computations and physical world are tightly integrated. Samples of CPS include wireless sensor networks, industrial automation systems and mobile robots (Chaari, Ellouze, Koubaa, Qureshi, Pereira, Youssef, & Tovar, 2016). The major roles of CPS are to increase efficiency of the entire industry, improving the effectiveness of operational process and fulfilling the dynamic requirements of production through integrated systems (Shafiq, Sanin, Szczerbicki, & Toro, 2015). These are achieved by increasing the flexibility and quality, reducing time to market, as well as developing new skills and talent globally Monostori, Kádár, Bauernhansl, Kondoh, Kumara, Reinhart, Sauer, Schuh, Sihn, & Ueda, 2016). Currently, CPS application has been observed in aerospace, automotive engineering, civil infrastructure and construction industries (Akanmu & Anumba, 2015); in energy, healthcare, and additive manufacturing industries (Cooper & Wachter, 2014); as well as in transportation, entertainment, consumer devices and smart city (Elshenawy, Abdulhai, & El-Darieby, 2018). There are few challenges facing adopters of CPS like storage and virtuality. CPS devices features are typically resource-constrained; with limited storage capacities, CPS requires abundant storage resources to generate and integrate large amount of data. As CPS is heterogeneous in nature, the need to virtualize its resources becomes a major requirement to ensure interoperability between devices and facilitate the integration (Chaari et al., 2016).
To date, Malaysia seems to be lagging behind other countries in adopting Industry 4.0 and CPS. As per reported by Malaysia’s Ministry of International Trade and Industry (MITI, n.d.), our manufacturing sector is currently still in phases 2.0 and 3.0 of the industrial revolution. Results from a survey conducted in the second half of 2016 by Federation of Malaysian Manufacturers (FMM) and Malaysian Institute of Economic Research (MIER) show very low awareness of Industry 4.0 amongst respondents; only 12 per cent of the respondents reported that they were very aware of what Industry 4.0 is. The majority (41 per cent) were only somewhat aware of Industry 4.0, the other 28 per cent admitted that they need more information while the other 19 per cent were not aware at all. Although this is so, MITI also identified efforts made by few sectors, namely, the electrical and electronic (E&E), aerospace and automotive sectors to be Industry 4.0 compliant. The dynamic nature of these sectors in terms of technology enhancement may be the main reason for why they are more advanced and thus, have moved forward to adopt Industry 4.0 on their own effort compared to other sectors.
The importance of Industry 4.0 has been the key reason for government departments and agencies to motivate industrial players in the country to adopt it. The departments including Ministry of International Trade and Industry (MITI), Ministry of Higher Education (MOHE), and Ministry of Energy, Science, Technology, Environment and Climate Change (MESTECC) have been tasked to lead the initiative in formulating a national policy or framework on Industry 4.0. The current economic plans such as Industrial Master Plan (IMP) 3 and Eleventh Malaysia Plan (2016-2020), have been guiding Malaysia’s mid-term policy until 2020. For instance, under the Eleventh Malaysia Plan (2016-2020), five sectors, namely, electrical and electronic (E&E), machinery and equipment (M&E), chemical, aerospace and medical devices have been identified as the game changers for manufacturing industry to grow further with the other sectors in Malaysia.
Problem Statement
The importance of Industry 4.0 adoption by various industries in Malaysia is critical; mainly because Malaysia’s vision is to re-emerge as Asian Tiger by improving governance, accelerating innovation, boosting productivity, moving industries up the value chain and enhancing the wellbeing of the people. (Ministry of Economic Affairs, 2018). Industry 4.0 would help Malaysia to not only transform its manufacturing sector towards advance technology, but it also would drive the country towards innovativeness that is among critical success factors of other developed countries. The importance of Industry 4.0 has led the government to initiate formulations of new policy and regulation to encourage manufacturing companies to adopt new technology through National Policy on Industry 4.0 (Industry4
The new industrial revolution encompasses the adoption of sophisticated technology which integrates the manufacturing process by using embedded system such as CPS. Through CPS, employers can monitor their daily manufacturing process and activities, thus making them more efficient and effective. CPS adoption is increasing rapidly particularly in developed nations to improve production process. A report by Ministry of International Trade and Industry (MITI, n.d.) identified several factors that contributed to the non-adoption of CPS phenomenon by manufacturers in Malaysia: lack of awareness on the importance of Industry 4.0 including its advantages to the firm, insufficient infrastructure developed by relevant parties to support the ecosystem, unclear policy and lack of special incentives to promote more companies to adopt Industry 4.0 in Malaysia, lack of standards which affect the integration process of different systems, and mismatch skillsets and lack of right talent to support the execution.
These findings indicate on the important role of manufacturing firms. Manufacturers need to understand that decisions to adopt CPS depend on their firms’ readiness of TOE framework (technology, organization and environment). For instance, CPS adoption requires new functional areas such as storage, real-time processing, and accessibility (Chaari et al., 2016). CPS also requires firms to adopt sophisticated system to support the adoption, namely, cloud computing and the Internet- of-Things (IoT) to extend its capabilities (Bordel, Alcarria, Robles, & Martín, 2017; Wang, Holm, & Moore, 2017).
This means that firms need to prepare for additional capital investment and sophisticated arrangement of sensor network architectures to ensure the abundant storage capabilities and the seamless access of computation-physical devices for smooth integration (Ashibani, & Mahmoud, 2017). It certainly requires manufacturing firms to invest more in new technologies and equipment and hire skilled workers to fit the job. The findings also indicate the important role to be played by the government. It is crucial for ministries and relevant government bodies to address the non-adoption factors of Industry 4.0 (through CPS) identified immediately to expedite its application so that our manufacturing industry will be more competitive and can keep abreast with emerging economies.
Since the adoption of CPS requires full commitment from companies to address issues like readiness, skills shortage and equipment upgrades (Leitao, Colombo, & Karnouskos, 2016), it is imperative for an operational model to be developed which can identify factors that may influence manufacturing firms’ intention to adopt CPS. In view of this need, the literature has acknowledged the use of the TOE framework, which focused on three factors, namely, technological, organizational and environmental characteristic of firms as well as the role of government policy and incentive to encourage CPS adoption. In addition, the roles played by relevant government policy and attractive incentives for instance may be important as those that moderate the relationship between Industry 4.0 determinants and its adoption by the firms. In view of this need, an operational model for the adoption of CPS is thereby proposed.
Research Questions
The proposed model would be answering these research questions:
Does manufacturing firms’ intention to adopt CPS technology depends on TOE characteristics (Technological, Organisational (financial, technology readiness), Environmental)?
Does the relationship between organizational characteristic (financial, technology readiness) and manufacturing firms’ intention to adopt CPS technology is moderated by government’s policy and incentives?
Purpose of the Study
-
To examine whether TOE characteristics (Technological, Organisational (financial, technology readiness), Environmental) influence manufacturing firms’ intention to adopt CPS technology.
-
To investigate the role of government policy and incentive as a moderator to moderate the relationship between organizational characteristic (financial and technology readiness) and manufacturing firms’ intention to adopt CPS technology.
TOE framework
The TOE framework developed by Tornatzky and Fleischer (1990) consists of three separate contexts (technology, organization, and environment) as influencer of firm’s operation process. This framework is used in past studies for investigation of technology adoption factors.
According to Tornatzky & Fleischer (1990), technological context refers to internal and external technologies that are relevant to the firm. Technologies which have been used by the firm are considered internal technologies while the set of technologies available in the market is the external technologies. Perceived relative advantage of technology, its compatibility and complexity are three elements characterising the technological context and proposed influencers for firms to adopt innovation technology. In brief, relative advantage (or perceived benefits) is defined as a set of a new technology perceived to be superior to the incumbent in building competitive advantage; and it has consistently been the main predictor for adoption intention in previous innovation research (Wamba, Gunasekaran, Bhattacharya, & Dubey, 2016; Awa, Ukoha, & Igwe, 2017). Compatibility describes the high chance of a firm gaining an advantage when it managed to incorporate new technology with its current and existing systems (Tornatzky and Klein, 1982). Lack of adaptability of the new technology with the organizational processes and the existing technologies were the reason for the most failure cases in technology adoption (Cooper & Zmud, 1990), that compatibility is investigated as a main predictor of technology adoption in previous studies (Brown & Russell, 2007; Wang, Wang, & Yang, 2010; Ramdani, Chevers, & Williams, 2013; Leung, Lo, Fong, & Law, 2015; Wamba et al., 2016; Awa et al., 2017). As for complexity, it describes the degree to which an innovation is perceived as relatively difficult to understand and use; thus, it is usually associated negatively with adoption since it can be a deterrent to successful implementation (Ramdani et al. 2013; Oliveira, Thomas, & Espadanal, 2014; Gangwar, Date, & Ramaswamy, 2015; Wang, Li, Li, & Zhang, 2016). Complexity has been investigated as main predictor of technology adoption in past studies (Brown & Russell, 2007; Ramdani et al., 2013; Leung et al., 2015; Wamba et al., 2016).
Tornatzky & Fleischer (1990) describes organizational context as characteristics and resources available to the firm that would support the adoption and operationalization of the new technology successfully (e.g. firm’s size, degree of centralization, degree of formalization, managerial structure, human resources, amount of slack resources, and linkages among employees). In other words, organization must have sufficient resources to support any adoption or else, the adoption becomes meaningless although it provides huge benefits to the firm. Past studies found organizational readiness as the main factor that affects the adoption of innovative technologies (Grandon & Pearson, 2004; Ramdani et al. 2013). Financial readiness and technological readiness have been tested as predictors of technology adoption (Lai, Lin, & Tseng, 2014; Leung et al. 2015). Financial readiness refers to the financial resources available by the firm to invest and implement the technology, while technological readiness refers to the level of sophisticated technologies are being used by organization (Iacovou, Benbasat, & Dexter, 1995). In brief, organizational readiness highlights the importance of sufficient IT sophistication (level of technological expertise) to execute the adoption of technology innovation as well as on the importance of management’s level of understanding and support to adopt the technology in question (Chwelos, Benbasat, & Dexter, 2001). In short, it is imperative for a firm to have the minimum office automation equipment, IT usage and IT management to be classified as “technologically ready” (Naicker & Pillay, 2009).
Environmental context refers to the external factors that would affect the technology adoption like opportunities or constraints that influence the technological innovations (Tornatzky & Fleischer, 1990). Various environmental factors that affect the technology adoption have been proposed (Salwani, Marthandan, Norzaidi, & Chong, 2009; Tornatzky & Fleischer, 1990; Awa et al., 2017) with some identified most important external factors for adoption (Fuchs, Höpken, Föger, & Kunz, 2010; Ramdani et al., 2013; Wang et al., 2010). Examples include perceived pressure from industry to upgrade the level of technological capability by the firm, perceived pressure from trade partners and customers, and regulation by government. Pressure by industry, competitors, and trading partners in influencing the adoption of innovation technologies have been found significant in past studies (Fuchs et al., 2010; Grandon & Pearson, 2004; Ramdani et al., 2013; Wang et al., 2010; Oliveira et al., 2014; Leung et al., 2015; Wamba, Gunasekaran, Akter, Ji-fan Ren, Dubey, & Childe, 2017; Ngah, Zainuddin, & Thurasamy, 2017).
Since the TOE framework is more robust and comprehensive covering both internal and external factors, it has been widely used to analyse the adoption of innovation technology such as an open system (Chau & Tam, 1997), Electronic Data Interchange system (EDI) (Kuan & Chau, 2001), Service-oriented architecture (SOA) (Maclennan & Belle, 2014), radio frequency identification technology (RFID) (Wang, et al., 2010), cloud computing (Alshamaila, Papagiannidis, & Li, 2013; Oliveira et al., 2014; Borgman, Bahli, Heier, & Schewski, 2014; Gangwar et. al, 2015), E-business (Yeh, 2014); Social Customer Relationship management (Ahani, Rahim, & Nilashi, 2017), enterprise applications (Ramdani et al., 2013), Enterprise Resource Planning (ERP) (Ruivo, Oliveira, & Neto, 2014), mobile marketing (Maduku, Mpinganjira, & Duh, 2016), Building information modelling (BIM) (Ahuja, Jain, Sawhney, & Arif, 2016) internet standard adoption (Hovav, Hemmert, & Kim, 2011) and electronic supply chain system (Lin, 2014).
Drawing upon the empirical evidences of past studies, it seems that TOE framework is a suitable underlying theory and explain factors that affect manufacturing firms’ intention to adopt CPS technology in Malaysia. Therefore, it is proposed in this model that:
Proposition 1: Technological, Organizational, and Environmental (TOE) factors would be positively related to manufacturing firms’ intention to adopt CPS.
The Role of Government Policy and Incentive for Technology Innovation
Government policy and incentive refer to the degree of pressure induced by government through policy and regulation which play a pivotal role to promote technology innovation (Lai et al., 2014; Oliveira et al., 2014). According to Coe, Helpman and Hoffmaister (2009), the characteristic of country in providing support and assistance will influence a firm’s decision to invest in innovation technologies. For instance, the study by Wonglimpiyarat (2016) has shown that the strong policy by Israeli government to create excellent infrastructure such as Silicon Wadi which is a similar to Silicon Valley in the United States was significant to ensure the success of industrial growth and economic activities. On top of that, several researches have shown that the firms are strongly encouraged to adopt new technology if they are not vulnerable to the financial constraints and this situation particularly happened in advanced economies such as United States (Amore, Schneider, & Zaldokas, 2013). In other words, if the firms face lack of access to liquidity, there is high possibility to constraints firms’ innovation activities (Aghion, Askenazy, Berman, Cette, & Eymard, 2012; Brown, Martinsson, & Peterson, 2012). Nevertheless, emerging economy also faces the same issues. According to Howell (2016), the financial constraints in China would affect Chinese firms to invest in innovation technology. Besides, in addition to financial constraints, several innovation barriers such as poor intellectual property rights and low talent pool would also affect innovation technology adoption. In view of this, it shows the importance of government policy and incentive to wipe out innovation barriers and encourage firms to adopt innovation technologies. Therefore, it is proposed that:
Proposition 2: Government policy and incentive moderates the relationship between organizational characteristic and manufacturing firms’ intention to adopt CPS.
Findings
A model conceptualising is proposed based on the arguments posed in the introductory and problem statement as well as literature support (Figure
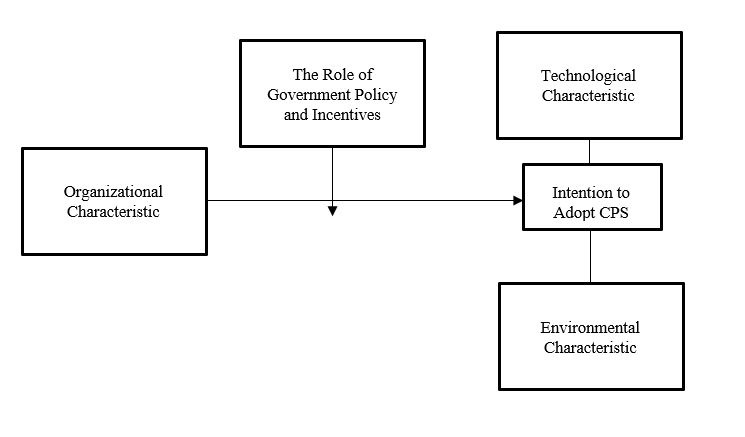
Conclusion
In conclusion, the issue of manufacturing firms in Malaysia taking a step to adopt CPS is critical to be tackled in order for the country to be competitive and be at par with other developed countries who are now implementing Industry 4.0. Having neighbouring countries like Singapore and Thailand ahead of us is not helping our case to apply an innovation-driven economy as a way to be a high-income nation by 2020. Thus, it is proposed that a model explaining reasons for adoption of CPS be developed and investigated. The findings from investigation of applicability of the adoption model would shed a new light on the effect of Technological, Organizational and Environmental (TOE) characteristic of manufacturing firms on their intention to adopt Cyber-Physical Systems (CPS). The findings would further identify the impact of government policy and incentive as moderator to enhance the relationship between organizational characteristic and intention to adopt CPS. In sum, the proposed model should be investigated as the findings would provide significant contribution to various stakeholders particularly the government and industrial players to understand the factors that influence CPS adoption among manufacturing firms in Malaysia.
References
- Aghion, P., Askenazy, P., Berman, N., Cette, G., & Eymard, L. (2012). Credit constraints and the cyclicality of R&D investment: Evidence from France. J. Eur. Econ. Assoc, 10(5), 1001-1024.
- Ahani, A., Rahim, N. A., & Nilashi, M. (2017). Forecasting social CRM adoption in SMEs: A combined SEM-neural network method. Computers in Human Behavior, 75, 560-578.
- Ahuja, R., Jain, M., Sawhney, A., & Arif, M. (2016). Adoption of BIM by architectural firms in India: Technology–organization–environment perspective. Architectural Engineering and Design Management, 12(4), 311–330.
- Akanmu, A., & Anumba, C. J. (2015). Cyber-physical systems integration of building information models and the physical construction. Engineering, Construction and Architectural Management, 22(5), 516-535.
- Alshamaila, Y., Papagiannidis, S., & Li, F. (2013). Cloud computing adoption by SMEs in the north east of England: A multi‐perspective framework. Journal of Enterprise Information Management, 26(3), 250-275.
- Amore, M. D., Schneider, C., & Zaldokas, A. (2013). Credit supply and corporate innovation. J. Finance. Econ. 109(3), 835-855.
- Ashibani, Y., & Mahmoud, Q. H. (2017). Cyber physical systems security: Analysis, challenges and solutions. Computers & Security, 68, 81-97.
- Awa, H. O., Ukoha, O., & Igwe, S. R. (2017). Revisiting technology-organization-environment (T-O-E) theory for enriched applicability. The Bottom Line, 30(1), 2-22.
- Bordel, B., Alcarria, R., Robles, T., & Martín, D. (2017). Cyber–physical systems: Extending pervasive sensing from control theory to the Internet of Things. Pervasive and Mobile Computing, 40, 156–184.
- Borgman, H. P., Bahli, B., Heier, H., & Schewski, F. (2014). Cloudrise: Exploring cloud computing adoption and governance. IEEE Computer Society, 12, 4425–4435.
- Brown, I., & Russell, J. (2007). Radio frequency identification technology: An exploratory study on adoption in the South African retail sector. International Journal of Information Management, 27(4), 250-265.
- Brown, J., Martinsson, G., & Peterson, B. (2012). Do financing constraints matter for R&D? Eur. Econ. Rev, 56(8), 673-692.
- Chaari, R., Ellouze, F., Koubaa, A., Qureshi, B., Pereira, N., Youssef, H., & Tovar, E. (2016). Cyber-physical systems clouds: A survey. Computer Networks, 108(24), 260-278.
- Chau, P. Y., & Tam, K. Y. (1997). Factors affecting the adoption of open systems: An exploratory study. MIS Quarterly, 21(1), 1–24.
- Chwelos, P., Benbasat, I., & Dexter, A. S. (2001). Research report: Empirical test of an EDI adoption model. Information Systems Research, 12(3), 304–321.
- Coe, D. T., Helpman, E., & Hoffmaister, A. W. (2009). International R&D spill overs and institutions. European Economic Review, 53(7), 723-741.
- Cooper, K. P., & Wachter, R. F. (2014). Cyber-enabled manufacturing systems for additive manufacturing. Rapid Prototyping Journal, 20(5), 355-359.
- Cooper, R. B., & Zmud, R. W. (1990). Information technology implementation research: A technological diffusion approach. Management Science, 36(2), 123–139.
- Elshenawy, M., Abdulhai, B., & El-Darieby, M. (2018). Towards a service-oriented cyber–physical systems of systems for smart city mobility applications. Future Generation Computer Systems, 79(2), 575-587.
- Fuchs, M., Höpken, W., Föger, A., & Kunz, M. (2010). E-business readiness, intensity, and impact: An Austrian destination management organization study. Journal of Travel Research, 49(2), 165–178.
- Gangwar, H., Date, H., & Ramaswamy, R. (2015). Understanding determinants of cloud computing adoption using an integrated TAM-TOE model. Journal of Enterprise Information Management, 28(1), 107-130.
- Götz, M., & Jankowska, B. (2017). Clusters and industry 4.0 – do they fit together? European Planning Studies, 25(9), 1633-1653.
- Grandon, E. E., & Pearson, J. M. (2004). Electronic commerce adoption: an empirical study of small and medium US businesses. Information & Management, 42(1), 197-216.
- Hovav, A., Hemmert, M., & Kim, Y. J. (2011). Determinants of internet standards adoption: The case of South Korea. Research Policy, 40(2), 253-262.
- Howell, A. (2016). Firm R&D, innovation and easing financial constraints in China: Does corporate tax reform matter? Research Policy, 45(10), 1996-2007.
- Iacovou, C. L., Benbasat, I., & Dexter, A. S. (1995). Electronic data interchange and small organizations: Adoption and impact of technology. MIS Quarterly, 19(4), 465–485.
- Kagermann, H., Wahlster, W., & Helbig, J. (2013). Recommendations for Implementing the Strategic Initiative. Industrie 4.0 Working Group of Acatech. Berlin.
- Kuan, K. K., & Chau, P. Y. (2001). A perception-based model for EDI adoption in small businesses using a technology–organization–environment framework. Information & Management, 38(8), 507-521.
- Lai, H. M., Lin, I. C., & Tseng, L. T. (2014). High-level managers’ considerations for RFID adoption in hospitals: An empirical study in Taiwan. Journal of Medical Systems, 38(2), 1–17.
- Leung, D., Lo, A., Fong, L. H., & Law, R. (2015). Applying the technology-organization-environment framework to explore ICT initial and continued adoption: An exploratory study of an independent hotel in Hong Kong. Tourism Recreation Research, 40(3), 391-406.
- Lin, H.-F. (2014). Understanding the determinants of electronic supply chain management system adoption: Using the technology–organization–environment framework. Technological Forecasting & Social Change, 86, 80–92.
- Maclennan, E., & Belle, J.-P. (2014). Factors affecting the organizational adoption of service-oriented architecture (SOA). Information Systems and e-Business Management, 12(1), 71-100.
- Maduku, D. K., Mpinganjira, M., & Duh, H. (2016). Understanding mobile marketing adoption intention by South African SMEs: A multi-perspective framework. International Journal of Information Management, 36(5), 711–723.
- Ministry of Economic Affairs. (2018). Mid-term Review of the Eleventh Malaysia Plan 2016-2020. Retrieved from https://www.talentcorp.com.my/clients/TalentCorp_2016_7A6571AE-D9D0-4175-B35D99EC514F2D24/contentms/img/publication/MidTerm%20Review%20of%2011th%20Malaysia%20Plan.pdf
- Ministry of International Trade and Industry (MITI). (n.d). The current status of our industry. Retrieved from https://www.miti.gov.my/index.php/pages/view/industry4.0?mid=559
- Ministry of International Trade and Industry (MITI). (2018). Industry 4.0. Retrieved from http://www.miti.gov.my/index.php/pages/view/industry4.0?mid=559
- Ministry of International Trade and Industry (MITI). (2018). National Policy on Industry 4.0 (Industry4WRD). Retrieved from https://www.miti.gov.my/miti/resources/National%20Policy%20
- on%20Industry%204.0/Industry4WRD_Final.pdf
- Monostori, L., Kádár, B., Bauernhansl, T., Kondoh, S., Kumara, S., Reinhart, G., Sauer, O., Schuh, G.,
- Sihn, W., & Ueda, K. (2016). Cyber-physical systems in manufacturing. CIRP Annals, 65(2), 621-641.
- Naicker, V., & Pillay, R. (2009). Electronic data interchange in developing countries: Lessons from South Africa. International Journal of Management and Marketing Research, 2(1), 89-101.
- Ngah, A. H., Zainuddin, Y., & Thurasamy, R. (2017). Applying the TOE framework in the halal warehouse adoption study. Journal of Islamic Accounting and Business Research, 8(2), 161-181.
- Oliveira, T., Thomas, M., & Espadanal, M. (2014). Assessing the determinants of cloud computing adoption: An analysis of the manufacturing and services sectors. Information & Management, 51(5), 497–510.
- Ooi, K. B., Lee, V. H., Tan, G. W. H., Hew, T. S., & Hew, J. J. (2018). Cloud computing in manufacturing: The next industrial revolution in Malaysia? Expert Systems with Applications, 93, 376-394.
- Ramdani, B., Chevers, D., & Williams, D. A. (2013). SMEs' adoption of enterprise applications: A technology-organisation-environment model. Journal of Small Business and Enterprise Development, 20(4), 735–753.
- Ruivo, P., Oliveira, T., & Neto, M. (2014). Examine ERP post-implementation stages of use and value: empirical evidence from Portuguese SMEs. International Journal of Accounting Information Systems, 15(2), 166-184.
- Salwani, M. I., Marthandan, G., Norzaidi, M. D., & Chong, S. C. (2009). E‐commerce usage and business performance in the Malaysian tourism sector: Empirical analysis. Information Management & Computer Security, 17(2), 166-185.
- Schwab, K. (2017). The Fourth Industrial Revolution. Geneva: Crown Business.
- Shafiq, S. I., Sanin, C., Szczerbicki, E., & Toro, C. (2015). Virtual engineering object (VEO): Toward experience-based design and manufacturing for industry 4.0. Cybernetics and Systems, 46(1-2), 35-50.
- Tornatzky, L. G., & Klein, K. J. (1982). Innovation characteristics and innovation adoption-implementation: A meta-analysis of findings. IEEE Transactions on Engineering Management, 29(11), 28-45.
- Tornatzky, L., & Fleischer, M. (1990). The processes of technological innovation. Lexington: Lexington Books.
- Wamba, S. F., Gunasekaran, A., Akter, S., Ji-fan Ren, S., Dubey, R., & J. Childe, S. (2017). Big data analytics and firm performance: Effects of dynamic capabilities. Journal of Business Research, 70, 356–365. https://doi.org/1016/j.jbusres.2016.08.009
- Wamba, S. F., Gunasekaran, A., Bhattacharya, M., & Dubey, R. (2016). Determinants of RFID adoption intention by SMEs: An empirical investigation. Production Planning and Control, 27(12), 979-990.
- Wang, L., Holm, M., & Moore, P. (2017). Cloud manufacturing – a critical review of recent development and future trends. International Journal of Computer Integrated Manufacturing, 30(4-5), 347-380.
- Wang, Y. S., Li, H. T., Li, C. R., & Zhang, D. Z. (2016). Factors affecting hotels' adoption of mobile reservation systems: A technology-organization-environment framework. Tourism Management, 53, 163-172.
- Wang, Y. M., Wang, Y. S., & Yang, Y. F. (2010). Understanding the determinants of RFID adoption in the manufacturing industry. Technological Forecasting and Social Change, 77(5), 803-815.
- Wonglimpiyarat, J. (2016). Government policies towards Israel’s high-tech powerhouse. Technovation, 52-53, 18-27.
- Yeh, R. (2014). E-HR Adoption in Taiwan: An Exploration of Potential Multilevel Antecedents and Consequences. International Conference on Knowledge Management in Organizations (pp. 126-135). Santiago, Chile: Springer International doi:
Copyright information
This work is licensed under a Creative Commons Attribution-NonCommercial-NoDerivatives 4.0 International License.
About this article
Publication Date
02 August 2019
Article Doi
eBook ISBN
978-1-80296-064-8
Publisher
Future Academy
Volume
65
Print ISBN (optional)
-
Edition Number
1st Edition
Pages
1-749
Subjects
Business, innovation, sustainability, environment, green business, environmental issues
Cite this article as:
Aziz, A. S. A., Wahid, N. A., & Iskandar, Y. H. P. (2019). Nurturing Business Sustainability and Innovation. In C. Tze Haw, C. Richardson, & F. Johara (Eds.), Business Sustainability and Innovation, vol 65. European Proceedings of Social and Behavioural Sciences (pp. 683-692). Future Academy. https://doi.org/10.15405/epsbs.2019.08.69