Abstract
Financial and economic evaluation of investment projects is central to the process of justification and selection of possible options for investing in transactions with real assets. It is largely based on the use of simulation methods. A common disadvantage of most performance indicators of investment projects is the requirement of certainty of input data. This results in significantly biased point estimates of project performance and risk. The presence of various types of uncertainties leads to the need to adapt the indicators of assessing the economic efficiency of the investment project through the use of mathematical methods that allow formalizing and simultaneously handling different types of uncertainty. The article analyzes the investment project by the Monte Carlo method, which is the most complex, but also the most powerful method of assessing and taking into account risks when making an investment decision. Due to the fact that in the process of implementation of this method is playing a fairly large set of options, it can be attributed to the further development of the scenario’s method. The Monte Carlo method provides the most accurate and reasonable probability estimates. The using of Bayesian intellectual technologies for the analysis of efficiency of the investment project allow to consider in detail the investment project from all possible parties in the conditions of considerable uncertainty, and also to estimate probability of occurrence of this or that event in the project, to show dispersion of the received sizes that further promotes more reasonable and considered investment decision.
Keywords: Investmentperformance evaluationriskinflationsimulation modeling
Introduction
Effectiveness evaluation of the investment project is one of the most important stages in solving a set of strategic tasks characteristic of the stage of implementation of the investment strategy. The validity of the investment decision depends on an objective and comprehensive assessment. The basis of the effectiveness evaluation of the investment project is a system of indicators that measures the effect of the implementation of IP (Individual Proprietor) with its investment costs.
Investors face some difficulties in the analysis of investment projects. These projects are driven by a system of specific factors that are fundamental.
These factors include: time, inflation, uncertainty, risk.
It should be noted that at present the theoretical approach to accounting for uncertainty and risk has not yet been fully formed. Most research on risk management is limited to identifying and classifying possible sources of risk. In the scientific literature there are different points of view on the topic of assessing the effectiveness of the project in conditions of uncertainty. In particular, it is necessary to single out significant works in this area published recently by the following scientists: Gatzert & Kosub (2016), Escribano, Marín-Quemada, & San Martín González (2013), Wing & Jin (2015), Burger, Graeber, & Schindlmayr (2014), Kolios, Read, & Ioannou (2016), Lee & Zhong (2015), Kitzing (2014), Thangavelu, Khambadkone, & Karimi (2015), Ji, Zhang, Huang, & Yin (2015), Spiecker & Weber (2014), Aragonés-Beltrán, Chaparro-González, Pastor-Ferrando, & Pla-Rubio (2014), Onar & Kılavuz (2015), Arnold & Yildiz (2015), Şengül et al. (2015) and Pereira, Pinho, Galhardo, & Macêdo (2014).
Problem Statement
In order to avoid problems in assessing the effectiveness of investment projects, it is necessary to take into account the time-factor. It is necessary to pay attention to the following aspects:
costs and results occur at different times;
the emergence of time lags, such as the time gap between production and realization and between the payment of resources and its consumption;
changes in the time of organization performance indicators;
the change over time in the prices of organization manufactured production and consumption of resources;
the influence of physical depreciation of the fixed assets. This is the reason for reduced productivity and increasing costs;
discrepancy between the volume of construction and installation works with the amount of payment, in particular the need for advance payment of contractors;
changes in time of the main economic indicators: key rate, tax rates and tax incentives.
The interaction between the investment process and the investment activity illustrated in Figure
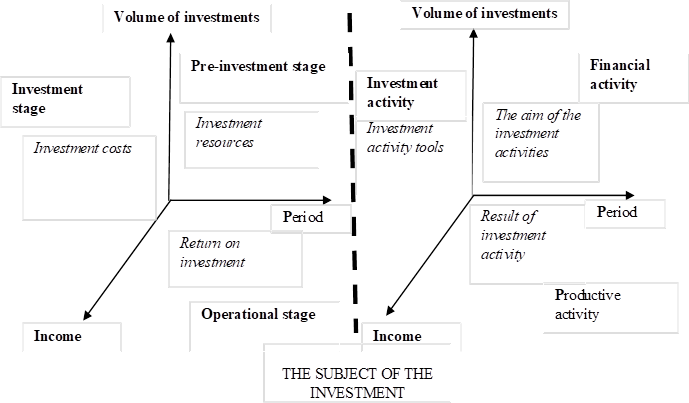
Research Questions
Accounting for the difference in costs and results is an integral part of assessing the effectiveness of an investment project, because over time, a financial resource has the ability to lose its value. There is a process of impairment over time. The basis of accounting is the discounting of cash flows, expressed in current and deflated prices, subject to the use of a single currency.
Discounting is the process of bringing different time values of cash flows to their value at a time that is necessary for the investor based on its goals and objectives.
The discount norm (discount rate) is the main economic indicator used in discounting. There are the following types of discount rates: commercial rate, rate for project participants, social rate, and budget rate.
The commercial discount rate is used to assess the commercial efficiency of the project. It is based on consideration of alternative capital efficiency.
Foreign practice in investment project management recommends using the weighted average cost of capital as a commercial discount rate (weighted average cost of capital – WACC). It represents the minimum rate of return that shareholders and creditors of the organization expect to receive from their investments. It follows that the weighted average cost of capital should not exceed the profitability of the investment project (Gizatullina, 2016).
Further, the weighted average cost of capital is dependent on two factors:
the cost of resources;
the structure of investment resources, that is, the share of each source of financing in their entirety.
The discount rate of the project participants reflects the effectiveness of participation in the investment project of organizations or other participants. The choice of this discount rate is up to the participants. If the participants have not formulated clear preferences, as a rule, a commercial norm is used as a discount rate (Gizatullina, Gerasimova, & Korenkova, 2015).
Inflation is a decrease in the purchasing power of money, which is manifested in the growth of the price level over time. Inflation significantly affects the efficiency of the investment project. First of all, inflation can have an impact on the financial feasibility of the project, as well as on the need for financing and efficiency of the use of equity.
Especially affected by inflation are investment projects, firstly, with a long investment cycle; secondly, requiring significant debt financing; thirdly, implemented with the simultaneous use of several currencies (Tarasova, 2015).
Purpose of the Study
Indicators of evaluation of economic efficiency of the investment project are the basis for making an informed investment decision. It is obvious that each of the indicators has its own distinctive advantages and disadvantages, which are also described in detail in the literature, so for making informed investment decisions it is necessary to share these indicators, as they allow decision makers to assess the effectiveness of the project from different sides. A common disadvantage of the above performance indicators of investment projects is the requirement of certainty of the input data, which leads to significantly biased point estimates of performance and risk of the project. The requirement of deterministic input data is an unjustified simplification of reality, as any investment project is characterized by a variety of uncertainty factors: the uncertainty of the source data, the uncertainty of the environment, uncertainty associated with the direction, options and model of the project, the uncertainty of the requirements for the effectiveness of the investment project. It is the uncertainties that determine the risk of the project, that is, the risk of loss of resources, loss of income or additional costs. It is necessary to predict in time the future state of a large number of uncertain parameters of market conditions in the analysis of long-term investment projects, including on the basis of the above indicators, so it is almost impossible to get an absolutely accurate forecast. The manifestation of uncertainty of numerical parameters of the planned project is the key in forecasting the economic efficiency and risk assessment of the investment project. Inherent uncertainty gives rise to equally fatal the risk of making investment decisions. Therefore, when carrying out forecasts, it is necessary to take into account the uncertainty factors that cause the risk for a certain performance indicator, so we inevitably face the problem of formal presentation of uncertain forecast parameters that determine the investment project, and carrying out appropriate calculations with it.
Thus, the presence of various types of uncertainties leads to the need to adapt the above indicators of assessing the economic efficiency of the investment project through the use of mathematical methods that allow formalizing and simultaneously handling different types of uncertainty.
Research Methods
Investors use the following indicators to assess the impact of inflation on the investment project:
the basic price index;
the chain price index;
the inflation rate.
The information base for the assessment is data collected on the basis of current information. It is obvious that changes in the basic variables in the future will lead to changes in the expected efficiency of the investment project. The focus on the average and the same for all costs price indices smoothes the existing differences between them and reduces the quality of the justification of investment decisions.
For a reliable and complete assessment of the impact of inflation on the efficiency of the project it is necessary to analyse and predict the price dynamics:
on manufactured products in the domestic and global market;
on fixed assets, raw materials, sales, wages, etc.;
on the consumer basket.
In addition, the exchange rate of the national currency and domestic inflation, the ratio and interest rates in the financial market are analysed and forecasted.
It is necessary to consistently perform certain actions in determining the current value of cash flows, taking into account inflation. At first, adjust the individual elements of cash flows that are directly dependent on inflation (sales, raw material costs, etc.). The adjustment should take into account the corresponding inflation rates. Secondly, determine the amount of profit from sales and the calculation of taxes and other mandatory payments. Thirdly, to estimate the nominal cash flows. In the fourth, calculate the nominal discount rate. In the fifth, to discount cash flows at a nominal rate.
If the investment project is evaluated by one way, investors need to adjust the calculations for the amount of risk. Method of correction of risk represents the adjustment of norms without the risk of the discount taking into account risk premiums.
The amount of the amendment should take into account three types of risks:
the country risk;
the risk of unreliability of the project participants;
the risk of non-receipt of the income provided by the project.
The need to take into account the country risk is due to the evaluation of the commercial efficiency of the project, as well as the effectiveness of the participation of organizations in the project and the effectiveness of investing in the shares of the organization.
The emergence of country risk is associated with changes in legislation, which can worsen the financial performance of the investment project.
Country risk assessment is based on the ratings of the world countries by the level of investment risk. Such a rating is published by the auditing companies (for example, “Fitch”, “Moody’s”), Association of Swiss banks, specialized rating firm BERI (Germany).
The size of the adjustments for country risk may be reduced, provided that the investment project of the Federal or regional support, as well as during implementation of the project on the terms of the agreement on production section.
The risk of unreliability of the project participants is the possibility of unforeseen termination of the investment project. The main reasons for the unexpected termination are:
the misuse of investment project funds;
the financial instability of the organization;
the insolvency, the negligence of other participants of the project.
The value of the premium for the risk of unreliability of the project participants is calculated by each participant of the investment project on the basis of expert evaluation. At the same time, a significant role is played by the elaboration of the project implementation mechanism.
The amount of the amendment for this type of risk may be reduced if property guarantees are provided for the fulfilment of obligations between the project participants or increased if the project participants do not have sufficient information about each other (for example, in the context of solvency and reliability).
The risk of non-receipt of income provided for in the implementation of the investment project arises primarily in connection with certain decisions of the project (technical, technological, organizational) and as a result of fluctuations in market conditions (changes in production volumes and prices for products and resources).
The risk adjustment shall be determined taking into account:
the technical feasibility of the project:
the degrees of elaboration of design solutions;
the availability of scientific and experimental design reserve of research;
the representativeness of marketing research.
The value of the risk premium is determined taking into account the goals of the investment project and the specifics of the industry (Table
The amount of risk adjustment may decrease as a result of:
the obtaining additional essential information concerning the indicators of feasibility and efficiency within the developed investment project;
the presence of representative marketing research, which confirms the moderately pessimistic forecast of the accepted volumes of demand and prices, taking into account the existing seasonal dynamics;
the availability in the project documentation of the organization of production at the stage of its development.
The Monte Carlo simulation is the most complex but also the most powerful method of risk assessment and accounting when making an investment decision. Due to the fact that in the process of implementation of this method is playing a sufficiently large number of options, it can be attributed to the further development of the method of scenarios. The Monte Carlo method provides the most accurate and reasonable probability estimates.
Further, the article analyses the investment project by the Monte Carlo method.
We have an investment project that will be implemented within 10 years.
The price for which we will sell our products is not exactly known. The exact quantity of production is unknown and the exact value of the variable cost of production is unknown. It will be random variables.
However, the expert way we have defined a range, which will be based on these values.
For example, the price will not be lower than 25 million rubles and not higher than 55 million rubles, the amount is not less than 11 and not more than 34 hectares, variable costs in the range of 57 to 297 rubles. The numbers can be quite different. The important thing is that we have an idea of the range of their probable values.
It is the values in these ranges that we will model to assess the overall attractiveness of the project.
To generate random values, we will use the Microsoft Excel function RANDBETWEEN, with the lower and upper range as arguments.
The resulting values will be used to calculate cash flows and net present value of the project (NPV).
A sufficiently large number of variants (experiments) are generated and all of them are processed by methods of statistical analysis. In our case, we use 5,000 experiments.
Next, specify the constant parameters of the project, and form a table of 5000 rows.
In each line we have a random value of output, variable cost and sales price. Also, for each line on the basis of these data are calculated such indicators as revenue, profit (minus fixed costs and tax), cash flow and net present value of the project for 10 years, taking into account the specified discount rate.
Initial data for the research of the effectiveness of the investment project by the Monte Carlo method are presented in Table
The results of the analysis are presented in Table
Thus, as a result of the research, it can be concluded that the project is highly risky.
Further, using the standard distribution, we estimate the probability of obtaining a particular NPV value. For example, a break-even project has NPV > 0.
By setting the value of X (this is our NPV) to zero, we get the probability of a loss of 98%.
To determine the probability, use the NORM.S.DIST function, which has the following syntax:
=NORM.S.DIST(z, integral)
Z - the value for which the distribution is built.
An integral is a logic value that defines the form of a function. If the integral argument is TRUE, the NORM.S.DIST function returns the integral distribution function; if the argument is FALSE, the weight distribution function is returned.
To determine the value of Z we use the NORMALIZATION function, which has the following syntax:
= NORMALIZATION (x, average, STDEVA)
x - normalized value. In our case, it is NPV.
Average - arithmetic mean of distribution.
STDEVA - standard deviation of the distribution.
However, despite the obvious attractiveness and advantages of the Monte Carlo method from a theoretical point of view, this method encounters serious obstacles in practical application due to the following main reasons:
High sensitivity of the result obtained by the Monte Carlo method to the laws of probability distribution and the types of dependencies of the input variables of the investment project;
Despite the fact that modern software allows to take into account the laws of probability distribution and the correlation of dozens of input variables, meanwhile, to assess their reliability in practical research is usually not possible, because, in most cases, analysts measure variations of the main variables of the macro- and microenvironment, select laws of probability distribution and statistical relationships between variables are subjective, since obtaining qualitative statistical information is not possible for various reasons (temporary, financial, etc.), especially for unique investment projects in the real sector.
The using the theory of fuzzy sets allows you to determine the permissible parameters of interrelated changes, pre-establishing verified rules for the formation of judgments about the integrated assessment of financial changes. An objective assessment is formed without interrupting non-financial information about the expansion of the sales market, the use of new key competencies, the emergence of emerging competitive advantages - the growing strategic development potential, and with a digital display of the interval values of the membership function representing the degree of completeness of organizational and technical measures that determine the growth of strategic potential, after the full implementation of development processes.
The effectiveness of decision making in information-analytical systems directly depends on the optimization power and adaptability to the decision-making conditions of the mathematical apparatus implemented here. Consider the scheme of formation of a mathematical model when making decisions. To do this, we define the main components of the decision-making model. Make the object of management or evaluation as a complex dynamically developing system. (CDDS) Got with a set of properties {Qoit} and relationships {Rojt,}, environment of functioning or vital activity of an object CDDS Get with a set of properties {Qeit} and relationships {Reit,}, as well as a management system or decision maker, CDDS Gat with a set of properties {Qait} and relationships {Rajt}.
Index t determines the time for the model CDDS. Indices i, j in reality tend to infinity, and in the corresponding models Gotm, GetmиGatm they are limited to values Iot, Iet, Iat, which at any given time t=tk determine the degree of completeness and adequacy of the models. The object of assessment or management can be understood as natural (abiotic and biotic) and anthropogenic (man-made, socio-economic objects and processes), under the environment of functioning, habitat or vital activity natural or man-made (production, social, landscape and other environments), under the control system (or management, management system) - technical, socio-political, natural environment or decision makers.
The control object is generally CDDS and can be active or passive with respect to the environment and the control system (CS). Thus, in complex problems, an adequate detailed mathematical model of the control object (CO) cannot be determined.
However, for practical problems, you can set the level of detail that allows you to create, within the specific constraints, requirements and criteria set, quasi-adequate mathematical models of CS, environment, and CO that are stable within the listed components of the decision-making process. At the same time, it is necessary to determine the metrological characteristics of models and solutions that establish the degree of adequacy, completeness and reliability of the models.
Under the conditions of the above uncertainty, the regularizing Bayesian approach (RBA) can serve as a methodological basis for creating CDDS models. Its advantages lie in its ability to provide stable estimates and models in small sample conditions, different types of information, significant inaccuracy of data and lack of clarity of knowledge about CO, CS and the environment. As a rule, in problems of mathematical modeling of complex systems of a priori knowledge is not enough, and the available experimental information and data are attracted.
The regularizing properties of RBA are provided by the introduction of a mathematical apparatus for creating, transforming and transmitting scales with dynamic constraints (SDC), where the acquisition, storage, transformation, transfer and interpretation of data and knowledge necessary for the formation of CS, CO and environment models takes place. Moreover, with each new piece of information there is a transformation and integration of it on the corresponding SDC, and the models are refined. Thus, as a result of such transformations on SDC, state assessments, decisions on compliance with criteria, model representations of processes and situations, conclusions, recommendations, tables and risk maps can be obtained as solutions.
The practical value of the Bayes theorem is to create Bayesian networks that allow, in graphic interpretation, to represent the probability distribution of features that have a causal relationship. It allowed to change the presentation and approaches to the assessment of expert opinions and statistical modeling.
To solve any problem of managing a complex object, to which, of course, the investment project under consideration also needs to clearly define the stages for obtaining a solution. A conceptual model for obtaining a solution based on BIT can be written as a formula:
Q = Q 1* Q 2* Q 3* Q 4* Q 5* Q 6* Q 7* Q 8* Q 9* Q 10* Q11. (1)
Q - complex problem solution, Q1 - definition of goals, restrictions, requirements for solving the problem, Q2 - creation of a conceptual model of an object with dynamic constraints (MDC), Q3 - choice of balanced scorecard, Q4 - determination of measurable indicators, Q5 - building scales with dynamic constraints (SDC), Q6 -inventory of initial information, Q7 - determination of dynamics and dynamic models, trends and trends in the development of situations and business processes at the facility and its environment, Q8 - business process risk assessment, Q9 - interpretation of the situation, Q10 - generation of recommendations for improving business processes, Q11 - verification of the effectiveness.
In real conditions, the specificity of the tasks of monitoring, auditing, making management decisions for complex objects is the lack of sufficiently complete, reliable data. Firstly, the information stored in electronic form, as a rule, has a statistical or experimental (measuring and / or expert) form, characterized by inaccuracy, vagueness and incompleteness, which determines the conditions of uncertainty and risk when choosing a rational decision. Secondly, uncertainty is also created due to the actions of subjects in the field of activity of the enterprise (suppliers, partners, competitors, banks, trade organizations, etc.), the purposeful impact of which on the situation cannot be predetermined. The first type of uncertainty can be considered fundamental and objective, the second - fundamental for the areas of active human activity and subjective. Thus, the problem of decision-making in a multi-criteria environment arises in conditions of risk and uncertainty.
When we are making appraisal and managerial decisions, a toolkit is needed that allows, on the basis of a formed request, to find the most complete, objective and reliable decisions in a reasonable time interval. The using of the BIT, designed to work in conditions of considerable uncertainty, allows solving the above problems. The BIT methodology is used in the object management concept as a basis for the generation of management decisions and technologies for their implementation. In this case, the tool with which it is possible to work with data of this nature becomes the software complex “Infoanalitik”. The content of the software package is presented schematically in Figure
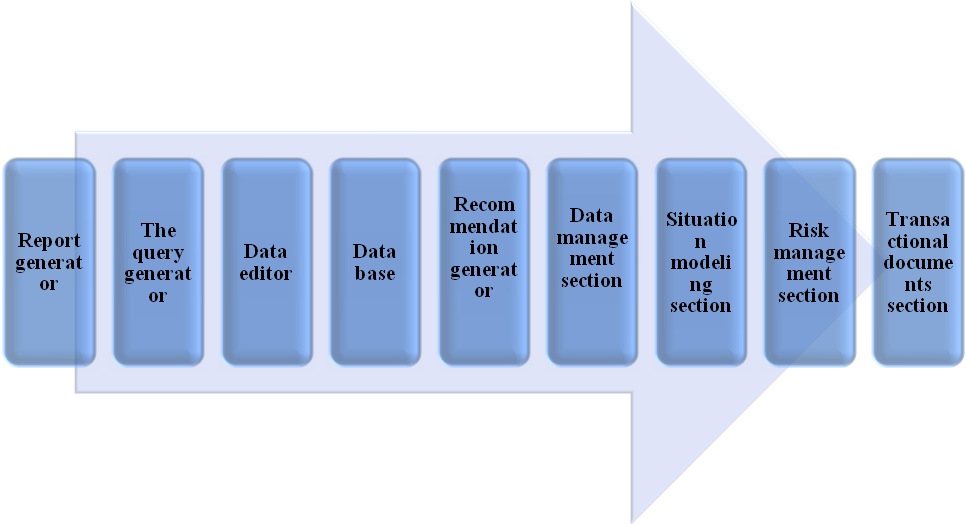
The concept of MDC and SDC Bayesian smart measurements is the key to the system «Infoanalitik». Scales with dynamic constraints are measurement scales, the frames of which can be different in terms of elements, values, estimates, and forms. They suggest the existence of alternative estimates with varying degrees of probability and are characterized by the variability of their characteristics, such as range, number of benchmarks, norms, controls.
To build MDC, controlled and measured properties of the object and the environment, as well as the relationship between them, are determined. The a priori uncertainty of the initial information can be considered as a measuring situation of recovering measured characteristics from incomplete information. The tasks of modeling an object from incomplete source information can be presented as incorrect inverse problems of restoring the model dependence (causes) from experimental data (consequences), and the solutions obtained are unstable. To ensure a sustainable solution, such problems require the use of regularizing schemes. Regularization is provided by replacing the likelihood function by the hypothesis distribution function and by introducing the mathematical apparatus for creating, transforming and transmitting scales with dynamic constraints on which the data is received, stored, transformed, transmitted and interpreted to form models.
Thus, the main idea of the regularizing approach is to use a scaling that metrising the solution space.
Findings
However, despite the obvious attractiveness and advantages of the Monte Carlo method from a theoretical point of view, this method encounters serious obstacles in practical application due to the following main reasons:
High sensitivity of the result obtained by the Monte Carlo method to the laws of probability distribution and the types of dependencies of the input variables of the investment project;
Despite the fact that modern software allows to take into account the laws of probability distribution and correlations of dozens of input variables, meanwhile, it is usually not possible to evaluate their reliability in practical research, since, in most cases, analysts measure variations of the main variables of the macro and micro environment the laws of probability distribution and statistical relationships between variables are subjective, since obtaining high-quality statistical information is not possible by amym various reasons (time, financial, etc.), especially for unique investment projects in the real sector of the economy;
Thus, the using of Bayesian intellectual technologies for the analysis of the investment project efficiency allows to consider the investment project in detail from all possible sides in conditions of considerable uncertainty. Also, the using of Bayesian intelligent technologies allows to estimate the probability of occurrence of any action in the project, to show the dispersion of the obtained values. Further it contributes to a more informed and deliberate investment decision. Evaluation of the investment project by this method is possible in the presence of a certain software package «Infoanalitik» (info Analytics).
Conclusion
The accuracy of the resulting by Monte Carlo estimates depends on the quality of the input assumptions and the relationships of the input variables. It can lead to significant errors in the results (for example, revaluation or underestimation of the risk of the investment project), and, consequently, it will lead to an erroneous investment decision.
The using of Bayesian intellectual technologies for the analysis of the investment project efficiency allows considering the investment project in detail from all possible sides in the conditions of considerable uncertainty. Also, the using of Bayesian intelligent technologies allows estimating the probability of occurrence of any action in the project, to show the range of values obtained. Further, it contributes to a more informed and deliberates investment decision.
References
- Aragonés-Beltrán, P., Chaparro-González, F., Pastor-Ferrando, J.-P., & Pla-Rubio, A. (2014). An AHP (analytic hierarchy process)/ANP (analytic network process)-based multi-criteria decision approach for the selection of solar-thermal power plant investment projects. Energy, 66, 222-238.
- Arnold, U., & Yildiz, Ö. (2015). Economic risk analysis of decentralized renewable energy infrastructures – A Monte Carlo Simulation approach. Renew Energy, 77, 227-239.
- Burger, M., Graeber, B., & Schindlmayr, G. (2014). Energy markets Manag Energy Risk. John Wiley Sons, Ltd, 1-54.
- Escribano, F., Marín-Quemada, J., & San Martín González, E. (2013). RES and risk: renewable energy's contribution to energy security. A portfolio-based approach Renew Sustain Energy Rev, 26, 549-559.
- Gatzert, N., & Kosub, T. (2016). Risks and risk management of renewable energy projects: the case of on shore and off shore wind parks. Renewable and Sustainable Energy Reviews, 60, 982-998.
- Gizatullina, О. (2016). Analysis and evaluation of logistics costs in the process of movement of goods, information and financial flows. Regional Economic Issues, 28(3), 83-87.
- Gizatullina, О., Gerasimova, Е., & Korenkova, J. (2015). Factors influencing the inflow of foreign direct investment in the Russian regions. Vestnik SSTU, 1, 82-87. [in Rus.].
- Ji, L., Zhang, X., Huang, G., & Yin, J. (2015). Development of an inexact risk-aversion optimization model for regional carbon constrained electricity system planning under uncertainty. Energy Conversion and Management, 94, 353-364.
- Kitzing, L. (2014). Risk implications of renewable support instruments: comparative analysis of feed-in tariffs and premiums using a mean-variance approach. Energy, 64, 495-505.
- Kolios, A., Read, G., & Ioannou, A. (2016). Application of multi-criteria decision-making to risk prioritization in tidal energy developments Int J Sustain Energy. International Journal of Sustainable Energy, 35, 59-74. DOI:
- Lee, C., & Zhong, J. (2015). Financing and risk management of renewable energy projects with a hybrid bond. Renewable Energy, 75, 779–787. DOI:
- Onar, S.Ç., & Kılavuz, T.N. (2015). Risk analysis of wind energy investments in Turkey.Hum. Ecol. Risk Assess Int J., 21, 1230-1245.
- Pereira, E., Pinho, J., Galhardo, M., & Macêdo, W. (2014). Methodology of risk analysis by Monte Carlo Method applied to power generation with renewable energy. Renewable Energy, 69, 347-355.
- Şengül, Ü., Eren, M., Eslamian, Shiraz S., Gezder, V., & Şengül, A. (2015). Fuzzy TOPSIS method for ranking renewable energy supply systems in Turkey. Renew Energy, 75, 617-625.
- Spiecker, S., & Weber, C. (2014). The future of the european electricity system and the impact of fluctuating renewable energy a scenario analysis. Energ Policy, 65, 185-197.
- Tarasova, О. (2015). Economic evaluation of transport market attractiveness in modern conditions. Shihobalovskie chteniya: Experience, problems and prospects of consumer market development, Plekhanov Russian University of Economics (Samara Institute, branch office),6, 74-79. [in Rus.].
- Thangavelu, S.R., Khambadkone, A.M., & Karimi, I.A. (2015). Long-term optimal energy mix planning towards high energy security and low GHG emission. Applied Energy, 154, 959-969,
- Wing, L., & Jin, Z. (2015). Risk management methods applied to renewable and sustainable energy: a review. Journal of Electrical and Electronic Engineering, 3, 1-12.
Copyright information
This work is licensed under a Creative Commons Attribution-NonCommercial-NoDerivatives 4.0 International License.
About this article
Publication Date
20 March 2019
Article Doi
eBook ISBN
978-1-80296-056-3
Publisher
Future Academy
Volume
57
Print ISBN (optional)
-
Edition Number
1st Edition
Pages
1-1887
Subjects
Business, business ethics, social responsibility, innovation, ethical issues, scientific developments, technological developments
Cite this article as:
Gizatullina, O., Piskunov, V., & Tarasova, T. (2019). Simulation Modeling For Effectiveness Evaluation Of Investment In Risk And Uncertainty Area. In V. Mantulenko (Ed.), Global Challenges and Prospects of the Modern Economic Development, vol 57. European Proceedings of Social and Behavioural Sciences (pp. 567-579). Future Academy. https://doi.org/10.15405/epsbs.2019.03.56