Abstract
Osteitis pubis is a constantly increasing syndrome among soccer players and not only, affecting their sports performances. Simulation and comparison of functional parameters of osteitis of the pubis use new computational techniques, neural networks, in the establishment and optimization of kinetic treatment in pubalgia. In this direction, the present study is conducted on a total of 35 patients (healthy and affected by osteitis of the pubis). Both healthy and affected patients benefited from the initial testing, which contained different parameters that were recorded at the level of the plantar region, and those affected by osteitis of the pubis benefited from kinetic treatment. The kinetic treatment was performed over a four-week period and consisted of various kinetic techniques, such as physical exercises, proprioceptive techniques etc. At the end of the treatment period, we performed the final test, and the obtained data were compared with the original ones using modern techniques of interpretation of the experimental results, which supported the idea of creating techniques for optimization of the kinetic treatment and implicitly the reduction of the convalescence period. This paper aims to introduce a modern computational concept, neural networks, in sports medicine, but also to find and implement an optimal treatment plan to recover from osteitis pubis and to reintegrate as quickly as possible into sports activity.
Keywords: Osteitis pubisneural networkkinetic treatmentsports medicine
Introduction
In recent years, soccer has had tremendous growth, in qualitative terms, by increasing the intensity and frequency of the game, as well as by the increased number of competitions a soccer player has to play in a competitive year/season. All this development has increased the intensity of both matches and training, increasing the number of training sessions, training camps, matches played in domestic and international championships etc. All these factors have led to the overuse of the mio-arthro-kinetic chain, thus favoring the emergence of new pathologies such as osteitis pubis.
Osteitis pubis is a noninfectious inflammation of the pubic bone that causes pain in the muscles with insertion at this level (Fricker, Taunton, & Ammann, 1991; Major & Helms, 1997; Albers, Spritzer, Garrett, & Meyers, 2001; Zaoui et al., 2012; Elattar, Choi, Dills, & Busconi, 2016; Turnea, Rotariu, Ionite, Arotaritei, & Ilea, 2017). In 2011, this pathology was present in the soccer game in a percentage of 10-13% of the total accidents within a year (Litwin, Sneider, McEnaney, & Busconi, 2011). The main causes of this pathology are stress overload or training errors and biomechanical features (McIntyre, Johson, & Schroeder, 2006; Ilea, Turnea, Arotaritei, Rotariu, & Popescu, 2015).
Problem Statement
The literature does not have many studies to address osteitis pubis in terms of kinetic treatment. The vast majority of studies address pharmacology, surgical interventions and diagnostic methods, thus identifying a lack of information on the approach of osteitis pubis in terms of kinetic treatment, as confirmed by Choi, McCartney and Best in 2011. Two studies, one in 2001 and the other in 2012, addressed the problem from a rehabilitation point of view, by using manual therapy and proprioceptive neuromuscular facilitation (PNF) techniques (Rodriguez, Miguel, Loma, & Heinrichs, 2001; Vijayakumar, Nagarajan, & Ramli, 2012). But the two studies were not enough to convince Cheatham,
Research Questions
-
By applying a kinetotherapeutic treatment, can we alleviate the symptoms of osteitis pubis?
-
Can multi-layer neural networks help improve the quality of the recovery program?
Purpose of the Study
The aim of the study is to optimize the kinetotherapeutic treatment and to highlight its effects in the recovery of osteitis pubis. Using mathematical simulations in vivo, we can achieve a multitude of scenarios, thus leading to a personalised treatment for each patient.
Research Methods
The study was conducted on 5 soccer players affected by osteitis pubis. All 5 subjects are 20 to 30 years of age, male, body weight between 60-90 kilograms, size between 170-190 cm, size of the sole between 22.5 and 28.5 cm and a minimum of 8 years in sports activity in this branch. They benefited from initial and final evaluations.
Subject evaluation was performed with a postural test, “Pedana OEM/DF, CLASS I MED. DEVICE”, along with the “Dr Foot Analysis 4.0” (Figure
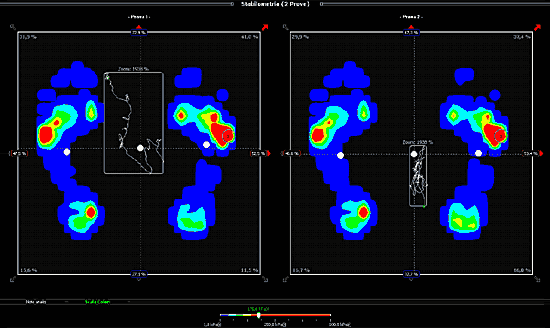
Between the two initial and final evaluations, the subjects underwent kinetotherapeutic treatment. The treatment protocol was performed over a period of four weeks (twenty sessions), with each treatment session lasting between 50 and 70 minutes. The treatment protocol was the same for each subject and was structured as follows: massage techniques (10 minutes), stretching (5-10 minutes), massage techniques (5-10 minutes), PNF techniques (5-10 minutes), massage techniques (5-10 minutes), a combination of stretching with PNF techniques (5-10 minutes), massage (5 minutes) and therapeutic physical exercises (5-10 minutes).
After the initial and final evaluations, the data obtained were quantified. Through the multi-layer neural networks, the data collected were compared with data from the study of Ionite, Rotariu and Gheorghita (2017).
Multi-layer neural networks are known as universal approximators (Hornik, Stinchcombe, & White, 1989). After local processing of the input signal, depending on the information stored in the synaptic weights (multiplying it with the stored information values), a global summary of the obtained results is produced – a process similar to that taking place in the cellular body of a real biological neuron. If the global response obtained exceeds a certain threshold of significance imposed by the user, the information is forwarded (Haykin, 1998; Lippmann, 1987; Arotaritei & Negoita, 2003).
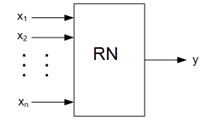
Artificial neural networks (ANNs) are structures that attempt to mimic the way the human brain functions and are constructed from multiple processing elements (EPs) or artificial neurons grouped into layers, each layer having a variable number of elements (Figure
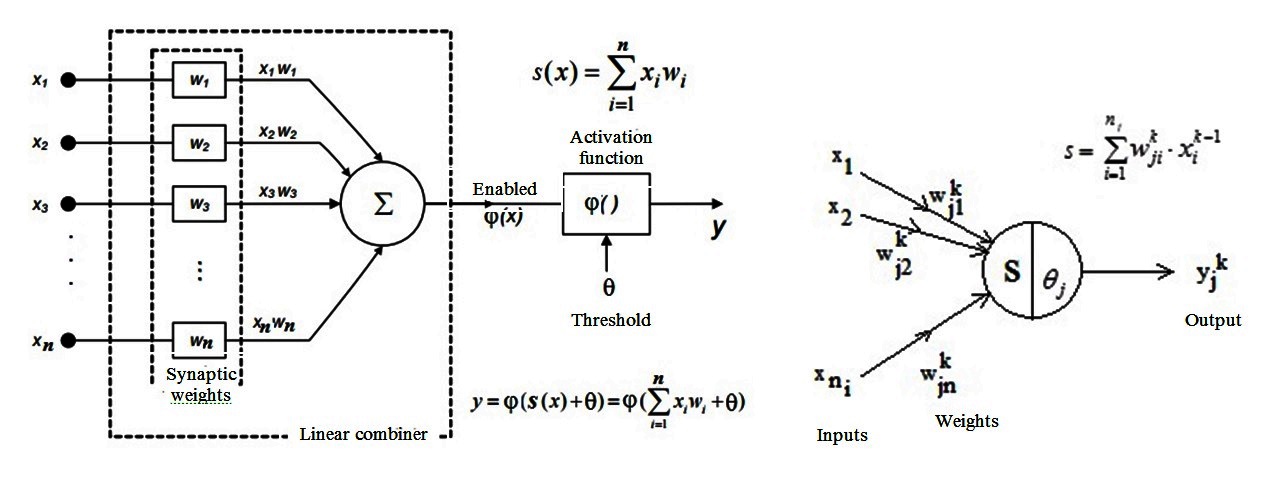
Signal propagation is made from input to output according to the equations in Figure
(3.1)
(3.2)
After assimilation/storage, the network fulfils its role as a classifier or predictor. Applying a vector to the input, we get, at the output, the desired class or the prescribed value (or even a prediction sequence) (Arotaritei, 2011; Arotaritei & Negoita, 2002).
Findings
Following the initial (I) and final (F) evaluations, the data on right plantar footprint (rPF), left plantar footprint (lPF), right plantar pressure (rPP), left plantar pressure (lPP) and the surface of the gravity centre (sGC) were collected and analysed. Synthetically, we present them in Table
We can see, in Table
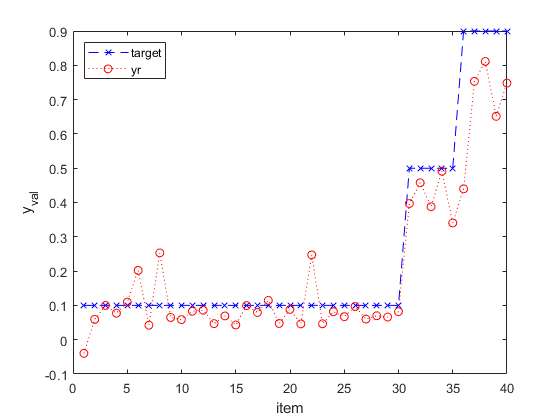
The value 0.1 is marked with class A (reference value), value 0.5 with class B (final assessment), and value 0.9 with class C (initial assessment).
There is a change in parameters ranging from class C to class B. This passage shows a tendency to normalise the parameters to class A, which is also visible in Figure
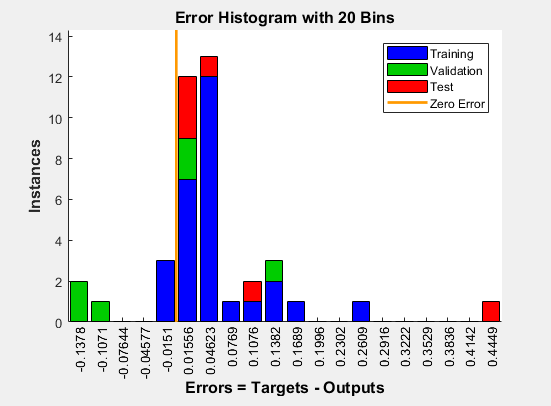
The built-in histogram gives us the training errors after building the classifier. A good classifier should give small errors for both sets of data, as described in Figure
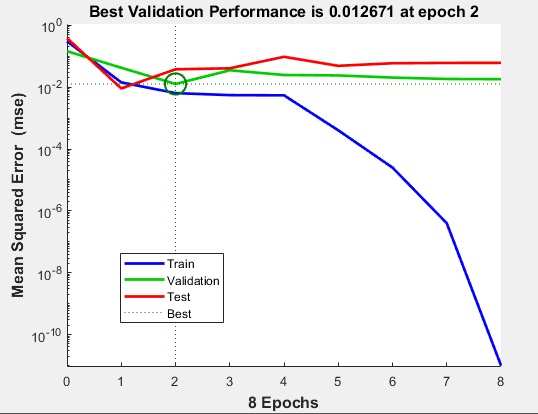
According to Figure
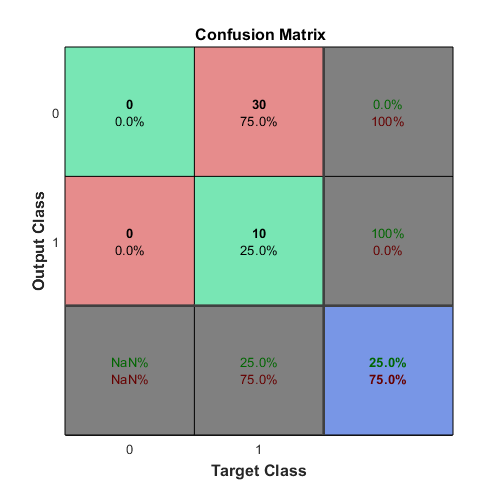
The results obtained in testing a two-tier form recognition system are synthesised in the confusion matrix in Figure
we determine how many shapes we have in each of the two classes according to the reference distribution (the test data set);
we determine how many forms have been recognized by the recognition system in each of the two classes;
we interpret the values in all cells on row 2 of the matrix of confusion.
We can see that 25% of in vivo tests meet the required acceptance threshold. Thus, the parameter values to be considered are on these layers (level 2) in the description of the optimal kinetic treatment. This “learning” process leads to the personalisation of treatment and the creation of optimal treatment.
Conclusion
Once osteitis pubis is established, it produces changes in the lower limbs, changes that can be recorded at the plantar level.
The kinetotherapeutic treatment plan used in this study has had positive effects, improving the symptom of osteitis pubis, which leads to a positive answer to the first question.
Multi-layer neural networks have helped to better understand the results obtained in this study and compare them with the results of other studies in the specialised literature, which means a positive answer to the second question. Through neural networks, we have demonstrated how, after applying the recovery treatment, class C changed and became class B, with a tendency towards normalisation, class A.
In addition to the above mentioned, the multi-layer neural networks helped us to classify small errors for datasets, adjust data and regulate the required acceptance level, 0.012671, obtained by testing the binary assumptions reaching level 2, and, through the matrix of confusion, we succeeded in statistical data validation. All this information provided by the multi-layer neural network helped us optimize the kinetotherapeutic treatment and automatically increase the quality of the therapeutic program
References
- Albers, S. L., Spritzer, C. E., Garrett, W. E. Jr, & Meyers, W. C. (2001). MR findings in athletes with pubalgia. Skeletal Radiology, 30(5), 270-277.
- Arotaritei, D. (2011). Genetic algorithm for fuzzy neural networks using locally crossover. International Journal of Computers Communications & Control, 6(1), 8-20.
- Arotaritei, D., & Negoita, M. G. (2003). An optimization of data mining algorithms used in fuzzy association rules. In V. Palade, R. J. Howlett & L. Jain (Eds.), Knowledge-based intelligent information and engineering systems (Part 2, Vol. 2774, pp. 980-985). Berlin, Heidelberg: Springer.
- Arotaritei, D., & Negoita, M. G. (2002). Optimization of recurrent NN by GA with variable length genotype. In B. McKay & J. Slaney (Eds.), AI 2002: Advances in artificial intelligence. Lecture notes in computer science (Vol. 2557, pp. 681-692). Berlin, Heidelberg: Springer.
- Cheatham, S. W., Kolber, M. J., & Shimamura, K. K. (2016). The effectiveness of nonoperative rehabilitation programs for athletes diagnosed with osteitis pubis. Journal of Sport Rehabilitation, 25(4), 399-403.
- Choi, H., McCartney, M., & Best, T. M. (2011). Treatment of osteitis pubis and osteomyelitis of the pubic symphysis in athletes: A systematic review. British Journal of Sports Medicine, 45(1), 57-64.
- Elattar, O., Choi, H. R., Dills, V. D., & Busconi, B. (2016). Groin injuries (athletic pubalgia) and return to play. Sports Health: A Multidisciplinary Approach, 8(4), 313-323.
- Fricker, P. A., Taunton, J. E., & Ammann, W. (1991). Osteitis pubis in athletes. Infection, inflammation or injury? Sports Medicine, 12(4), 266-279.
- Haykin, S. (1998). Neural networks: A comprehensive foundation (2nd ed.). Prentice Hall.
- Hornik, K., Stinchcombe, M., & White, H. (1989). Multilayer feedforward networks are universal approximators. Neural Networks, 2(5), 359-366.
- Ilea, M., Turnea, M., Arotaritei, D., Rotariu, M., & Popescu, M. (2015). Graphical user interface with applications in susceptible-infectious-susceptible models. The Medical-Surgical Journal, 119(2), 610-614.
- Ionite, A. C., Rotariu, M., & Gheorghita, A. (2017). Assessment of the pubalgic walking with the help of podiatry platform at football players. In I. Roceanu (Ed.), Proceedings of the 13th International Scientific Conference “eLearning and Software for Education”, April 27-28, 2017 (Vol. 3, pp. 537-546). Bucharest: Carol I National Defence University. DOI: 10.12753/2066-026X-17-254
- Lippmann, R. (1987). An introduction to computing with neural nets. IEEE ASSP Magazine, 4(2), 4-22.
- Litwin, D. E. M., Sneider, E. B., McEnaney, P. M., & Busconi, B. D. (2011). Athletic pubalgia (sports hernia). Clinics in Sports Medicine, 30(2), 417-434.
- Major, N. M., & Helms, C. A. (1997). Pelvic stress injuries: The relationship between osteitis pubis (symphysis pubis stress injury) and sacroiliac abnormalities in athletes. Skeletal Radiology, 26(12), 711-717.
- McIntyre, J., Johson, C., & Schroeder, E. L. (2006). Groin pain in athletes. Current Sports Medicine Reports, 5(6), 293-299.
- Rodriguez, C., Miguel, A., Loma, G., & Heinrichs, K. (2001). Osteitis pubis syndrome in the professional soccer athlete: A case report. Journal of Athletic Training, 36(4), 437-440.
- Rotariu, M., Turnea, M., Ilea, M., Arotaritei, D., Ionite, A. C., & Gheorghita, A. (2017). Statistical analysis and simulation of orthostatic position by means of the pedometer in patients with hyperkyphosis. Revista de Cercetare și Intervenție Socială, 59, 209-221.
- Turnea, M., Rotariu, M., Ionite, A.-C., Arotaritei, D., & Ilea, M. (2017). Fractional derivatives in mathematical model of the acute inflammatory response. World Wide Journal of Multidisciplinary Reasearch and Development, 3(11), 91-91.
- Vijayakumar, P., Nagarajan, M. & Ramli, A. (2012). Multimodal physiotherapeutic management for stage-IV osteitis pubis in a 15-year old soccer athlete: A case report. Journal of Back and Musculoskeletal Rehabilitation, 25(4), 225-230.
- Zaoui, A., Bacha, O., Boughammoura, H., El Frigui, S., Bouaziz, M. A., Mseddi, M., Rejeb, N. (2012). Osteitis pubis in the young professional soccer player. Science & Sports, 27(5), 305-307.
Copyright information
This work is licensed under a Creative Commons Attribution-NonCommercial-NoDerivatives 4.0 International License.
About this article
Publication Date
16 February 2019
Article Doi
eBook ISBN
978-1-80296-054-9
Publisher
Future Academy
Volume
55
Print ISBN (optional)
-
Edition Number
1st Edition
Pages
1-752
Subjects
Sports, sport science, physical education
Cite this article as:
Ionițe, A., Rață, G., Arotăriței, D., & Rotariu, M. (2019). Optimization Of The Kinetic Treatment Of Osteitis Pubis In Soccer Players. In V. Grigore, M. Stănescu, M. Stoicescu, & L. Popescu (Eds.), Education and Sports Science in the 21st Century, vol 55. European Proceedings of Social and Behavioural Sciences (pp. 640-647). Future Academy. https://doi.org/10.15405/epsbs.2019.02.79