Abstract
In recent years, big data analytics (BDA) have become an important subject for both researchers and business professionals. Especially, today’s managers and researchers put great emphasis on big data as a new source of innovation and competitive advantage. In the literature although there were some studies that examined BDA capability and firm performance relation, any studies have been conducted to directly investigate the effect of BDA capability on firm innovativeness. In this context we proposed a research model to investigate the effect of BDA capability on firm innovativeness moderated by the effect of data-driven culture. We created BDA capability dimensions as technical capability, managerial capability and talent capability depending on the previous studies in this field. Also we treated data-driven culture as moderator because without the acceptance and readiness of the whole organization for big data transformation, big data initiatives cannot be successful. With this model we created a roadmap for the following researchers to empirically test the expectations of managers and academicians. Therefore following researchers can fill the gap in the literature by showing how firm innovativeness will be affected by BDA capabilities.
Keywords: Big Databig data analytics capabilityfirm innovativeness
Introduction
In recent years, big data has become a major interest for companies and researchers. With the development of technology, a continuous data flow has emerged from different channels such as web sites, forums, social media applications, mobile phones and so on. The information stored in these data flows has a great importance for companies to make accurate strategies about their business fields and to take quick and correct decisions. That’s why companies utilizing from big data analytics perform better than their rivals and create a sustainable competitive advantage.
Some researchers have used different expressions for BDA such as "management revolution" (McAfee & Brynjolfsson, 2012, p.4), "the next frontier for innovation, competition and productivity" (Manyika et al., 2011, p.1), "the next blue ocean in nurturing business opportunities" (Kwon et al., 2014, p.387), and “the potential to revolutionize the art of management” (Wamba et al., 2015, p.234). In general, according to one of the most known definition, BDA is defined as “the collection of data and technology that accesses, integrates, and reports all available data by filtering, correlating, and reporting insights not attainable with past data technologies” (APICS, 2012).
BDA has become important for companies especially in decision making processes (Hagel, 2015). In addition to being an important distinction between high-performance organizations and low-performance organizations, BDA have made companies more proactive and visionary, reduced customer acquisition costs and contributed to firm revenue growth (Liu, 2014). Companies use BDA to study whole organizations, teams and workgroups to understand individual or group behaviors, social dynamics, coordination challenges and performance outcomes. On the customer side, they use BDA to get insights about purchasing intentions, consumption habits, communication channels, distribution channels, advertising, pricing, new product success and so on (Xu & Frankwick, 2016; George et al., 2014).
In the literature it is seen that firms need to develop BDA capabilities in order to be successful in big data investments. Ross et al. (2013) state that many companies fail in big data investments because these firms are not capable in making decisions on the basis of information that is filtered out of big data. Because these firms do not have required BDA capabilities to manage and utilize from big data. Depending on IT basis, researchers have created different BDA capabilities to utilize data sets in coordination with IT assets and human talents to gain sustainable competitive advantage (Garmaki, Boughzala & Wamba, 2016). From this perspective, in many studies researchers investigated the effect of BDA capability on firm performance (e.g. Gupta & George, 2016; Akter et al., 2016; Wamba et al., 2017).
In this study, with the role of BDA capability, we investigate the effect of BDA on firm innovativeness under the moderation of data-driven culture. In previous studies, although researchers emphasized big data as a driver for innovation and competitive advantage (Tan et al., 2015; Manyika et al., 2011; Erevelles et al., 2007) there were not any satisfactory empirical study to show the direct effect of BDA on firm innovativeness. With our research model and developed propositions we have created a road map for future studies.
This paper is organized as follows: the next section gives theoretical information about big data, BDA and BDA capabilities. The following section focuses on research model and proposition development. In the last section, expected results, research contributions and limitations are given.
Theoretical Framework
Big Data Definition
McAfee and Brynjolfsson (2012) point out that there are 3 main differences that distinguish big data from other data: volume, velocity and variety. Oracle (2012) added value dimension to these three dimensions and White (2012) added fifth dimension, veracity, and argued that big data has five distinguishing dimension: volume, velocity, variety, value and veracity.
Volume: The most distinguishing dimension of big data. It is used for to state that huge amount of data are collected from various resources. (O’Leary, 2013).
Velocity: It refers to the speed of collection and transmission of data (Russom, 2011). The data collected at high speed can be analyzed in real time, near real time, or in batches (Demchenko et al., 2013).
Variety: It points out heterogeneity of data types (Kaur & Sood, 2017). Big data can be collected from different resources and can be in different formats but in any relevant analysis common results can be derived using data from these different sources (Russom, 2011; O’Leary, 2013).
Value: It refers to the process of knowledge generation that creates value for companies (Kaur & Sood, 2017). Too much data is not enough alone. The important thing is to analyze data that creates value for companies to generate new knowledge from the hidden information in big data sets.
Veracity: It is related to reliability, consistency, accessibility and transparency of big data. In this context, providing data security becomes important in the process of obtaining, storing and analyzing the data (Demchenko et al., 2013).
Big data literature showed that big data is not only related to huge data volumes, it is also related to diversity of data types and delivery speed. The source of this huge amount of data can be internal or external and the structure of data can be structured or unstructured (Russom, 2011; Raguseo, 2018). In the literature there are many big data definitions. To understand big data more clearly some of the definitions are given in the table below.
BDA and BDA Capabilities
BDA is a term briefly used to describe advanced analysis techniques applied to big data. (Russom, 2011, p.8). When considering today's technological advances and people's dependence on the technology, as a result of our daily life we leave behind detailed digital records. Our twitter shares, social relationships, shopping habits, searches through web sites, photographs and videos, and even our physical movements and even the roots of cars create important usable digital records. For sciences that study human behavior this data pool has an irresistible appeal. Therefore private sector and public sector managers want to realize their activities and make decisions by analyzing these data. BDA has become important in this regard. Basically, BDA is the process of filtering data with terabytes of small values into the high-valued data, and in some cases even a high-valued single-bit data. The goal here is to see the bigger picture of our digital life (Fisher et al., 2012, p.50).
Big data is considered as data that exceeds processing capacity of traditional database systems. This data is too big, multiplying very rapidly and is not compatible for existing database systems. Therefore, firms need alternative analytical techniques to process data and to take the information in big data sets (Dumbill, 2013). In this context, BDA capabilities become important for companies to transform information in big data into strategic resources and creating new strategies to meet customer needs and expectations (Davenport & Patil, 2012).
In the literature there are many definitions for BDA capability. Generally it is seen as the firms’ ability to manage a huge volume of disparate data to allow users to implement data analysis and reaction (Hurwitz et al., 2013). Kwon et al. (2014, p.387) define BDA as “technologies (e.g., database and data mining tools) and techniques (e.g., analytical methods) that a company can employ to analyze large scale, complex data for various applications intended to augment firm performance in various dimensions”. Garmaki, Boughzala and Wamba, (2016) defined BDA capability as “firm’s ability to mobilize and deploy BDA resources effectively, utilize BDA resources and align BDA planning with firm strategy to gain competitive advantage and enhance firm performance”. In another study, Gupta and George (2016, p.1) defined BDA capability as “firm’s ability to assemble, integrate, and deploy its big data-specific resources”. Cosic et al., (2012, p.4) define BDA capability as ““the ability to utilize resources to perform a business analytics task, based on the interaction between IT assets and other firm resources.” Wamba et al. (2017, p.357) treats BDA capability as “an important organizational capability leading to sustainable competitive advantage in the big data environment”.
Theoretically researchers depend on IT capability literature to create BDA capabilities. BDA is seen as a new IT innovation for superior performance and fierce competition (Garmaki et al., 2016). In many studies, IT capabilities are theoretically based on a resource-based viewpoint. Because when creating IT capabilities it is assumed that resources can be easily imitated but capabilities are seen as unique and distinctive for every organization and cannot be easily imitated. Therefore, firms can achieve sustainable competitive advantage with these unique and distinctive capabilities (Santhanam & Hartono, 2003). From this point of view, depending on the resource based view, many researchers have argued that BDA capabilities are important organizational capabilities that provide sustainable competitive advantage to firms (Davenport 2006; Mcafee & Brynjolfsson, 2012; Gupta & George, 2016; Akter et al., 2016; Wamba et al., 2017).
In recent years, it is seen that researchers have given importance to experimental studies that generally investigated BDA capability and firm performance relationship (Garmaki et al. 2016; Akter et al., 2016; Wamba et al., 2017; Gunasekaran et al., 2017) but these researches are not sufficient to create a common understanding of BDA. In order to measure BDA capability researchers generally used information technology (IT) and information systems (IS) capabilities to create BDA capabilities (Garmaki et al., 2016; Gupta & George, 2016; Wang et al., 2016; Wamba et al., 2017; Akter et al., 2016). The table below gives a brief summary of dimensions used in previous BDA capability studies.
Research Model and Propositions
In this study we propose technical capability, managerial capability and talent capability dimensions to measure BDA capability. These three dimensions are mostly used ones in the literature. Briefly, technical capability refers to infrastructure elements such as applications, hardware, data, networks, etc. Managerial capability refers to the ability of BDA executives in performing their routines in line with business needs and priorities to manage BDA resources effectively. Talent capability refers to skills and knowledge of workers in achieving given tasks (Kim et al., 2012). Researchers in this field generally investigated the relationship between BDA capability and firm performance. For example; Gupta and George (2016), investigated the effect of BDA capability on market performance and operational performance. Chen et al. (2012) investigated the effect of BDA usage on asset productivity and business growth. Kwon et al. (2014) investigated the direct effect of data quality management on data usage experience and adoption intention of big data analytics. Akter et al. (2016) found a positive relationship between BDA capability and firm performance. Unlike other studies we propose a relationship between BDA capability and firm innovativeness with the moderation effect of data-driven culture.
BDA Capability and Firm Innovativeness
In the big data related studies, researchers generally investigated the relationship between BDA capability and firm performance (e.g. Gupta & George; Chen et al., 2012; Akter et al., 2016). Researchers do not investigated empirically how firm innovativeness will be affected by BDA capabilities. Firm innovativeness generally refers that to what extent organizations are open to new ideas and support innovative activities (Hurley & Hult, 1998; Calantone et al., 2002). In this study we propose a relationship between BDA capability and firm innovativeness.
LaValle et al. (2011) pointed out that top-performing organizations use analytics five times more than lower performers. That is why big data usage makes companies more competitive. They create new products and services according to the results of big data analyses. Manufacturing firms use data from actual product consumptions to create new innovative products, improve existing products and develop next generations of products. Also, firms can invent new business models or improve existing business processes depending on the big data analyses results. As a result big data usage becomes important for companies to outperform their rivals by creating sustainable competitive advantage in the long run. For this reason, forward thinking leaders have given importance to build big data capabilities in the entire organization (Manyika et al, 2011).
As it is mentioned above, when researchers create BDA capabilities in their studies they generally depend on IT literature and created their BDA capability measures on the basis of IT capability measures (e.g. Garmaki et al., 2016; Gupta and George, 2016; Wang et al., 2016). When we examine IT capability literature, it is understood that IT facilitates innovation processes by improving communication, knowledge sharing and organizational learning. Also, IT usage is expected to reduce development cost and shorten development process of new products and services (Carbonara, 2005; Banker et al., 2006). Similarly, today’s managers and researchers put great emphasis on big data as a driver for innovation and competitive advantage (Tan et al., 2015). In the big data literature it is assumed that firms can create new innovative products, improve existing products and develop next generations of products depending on the actual consumption data of existing products. This means that especially manufacturing firms use big data sets from different sources to create new products, new business models or processes (Manyika et al., 2011). Erevelles et al. (2007) states that in highly competitive marketplaces, to achieve competitive advantage firms must enhance idea generation speed. In this context, big data can be seen as a new competitive source of idea generation in new product development, distribution channels, dynamic pricing, customer service and so on (Erevelles et al., 2016). Based on this logic, we state the following proposition:
P1: BDA capabilities are positively related to firm innovativeness.
Moderating Role of Data-Driven Culture
Beyond the necessity of technical renewal to adapt big data usage, there exists a great difficulty in managerial and cultural adaption to big data environment. (Brynjolfsson et al., 2012; Wang et al., 2016). Therefore to be successful in big data usage, big data awareness should be settled throughout the firm and big data transformation should be experienced in the entire organization.
Organizational culture generally indicates shared values, norms, assumptions and understandings by members of a social group and this culture has a critical importance for managers to direct employees in the desired way (Schein, 1990; Daft, 2005). In the literature there are many studies takes culture as moderator variable to achieve better firm performance or innovative outcomes (e.g. Hynes, 2009; Prajogo & Ahmed, 2006). With this line, in this study we take data driven culture as a critical factor to enhance the relationship between BDA capability and firm innovativeness. Today, the biggest challenges of big data adoption in companies are managerial and cultural challenges. Companies generally ignore the need for human insight when they adopt big data analytical tools. However, without the acceptance and readiness of whole organization and workers for big data initiatives companies begin to fail (McAfee & Brynjolfsson, 2012). In the literature some researchers pointed out those firms are not always successful in their big data investments. Because these firms do not consider cultural dimension of big data initiatives (Ross et al., 2013; Lavelle et al., 2013). We can say that cultural readiness to big data initiatives is more important than big data applications alone. Data driven culture states a culture where decision makers base their decisions the insights extracted from data rather than their intuitions (Ross et al., 2013; McAfee & Brynjolfsson, 2012).
P2: Data driven culture moderates the relationship between BDA capabilities and firm innovativeness.
In this paper, depending on our literature review and propositions we settled proposed research model as below:
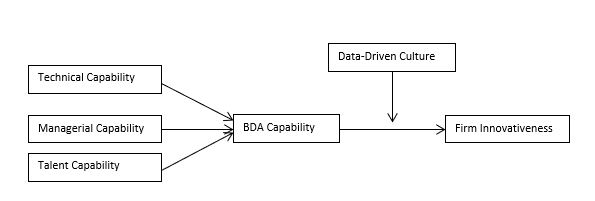
Conclusions
This study is expected to contribute BDA literature with the development of BDA capabilities and firm innovativeness relationship. Previous studies investigated the effect of BDA capability on firm performance from different perspectives but they haven’t examined such a relationship under the moderation of data-driven culture. In this model, we highlight that BDA capability is not sufficient alone to achieve higher innovation performance. Depending on previous literature, we argued that firms need an organization wide mindset to be successful in their big data initiatives and they can achieve this with data-driven culture. Therefore we emphasized the moderating role of data-driven culture in the proposed model. We believe that this model will be an important road map for the researchers and business people in this field. Depending on the given literature review, we expect that empirical researches in the future will support our propositions. When future researchers investigate the proposed model in this paper, they can also investigate the effect of other third variables (such as organizational learning, environmental dynamism, technological turbulence, strategic fit between BDA and business strategy, firm flexibility and so on) on the relationship between BDA capability and innovativeness.
The proposed model has some limitations for empirically testing. Although there are some theoretical contributions about BDA depending on IT and IS literature, empirical studies related to BDA capability are not sufficient enough to measure this variable accurately. The other limitation is related to data collection and sample. To test the proposed research model target sample should be selected from data analysts, business analysts or CIFs because BDA related variables requires technical knowledge from these areas of expertise. Also, accessing the information of this target sample is not easy but it is critical for the accurate measurement of the model.
Acknowledgment
Tugba Karabaoga acknowledges TUBITAK for PhD scholarship (TUBITAK- 2211/E programme)
References
- Akter, S., Wamba, S. F., Gunasekaran, A., Dubey, R., & Childe, S. J. (2016). How to improve firm performance using big data analytics capability and business strategy alignment?. International Journal of Production Economics, 182, 113-131.
- APICS. (2012). APICS 2012 Big Data Insights and Innovations Executive Summary.
- Banker, R. D., Bardhan, I., & Asdemir, O. (2006). Understanding the impact of collaboration software on product design and development. Information Systems Research, 17(4), 352-373.
- Barton, D., & Court, D. (2012). Making advanced analytics work for you. Harvard Business Review, 90, 78.
- Beyer, M.A. & Laney, D. (2012). The Importance of “Big Data”: A Definition. Gartner Publications, pp.1–9
- Boyd, D., & Crawford, K. (2012). Critical questions for big data: Provocations for a cultural, technological, and scholarly phenomenon. Information, communication & society, 15(5), 662-679.
- Brynjolfsson, E., & McAfee, A. (2012). Race against the machine: How the digital revolution is accelerating innovation, driving productivity, and irreversibly transforming employment and the economy.
- Calantone, R. J., Cavusgil, S. T., & Zhao, Y. (2002). Learning orientation, firm innovation capability, and firm performance. Industrial marketing management, 31(6), 515-524.
- Carbonara, N. (2005). Information and communication technology and geographical clusters: opportunities and spread. Technovation, 25(3), 213-222.
- Chen, H., Chiang, R. H., & Storey, V. C. (2012). Business intelligence and analytics: From big data to big impact. MIS quarterly, 36(4).
- Cosic, R., Shanks, G., & Maynard, S. (2012, January). Towards a business analytics capability maturity model. In ACIS 2012: Location, Location, Location: Proceedings of the 23rd Australasian Conference on Information Systems 2012 (pp. 1-11). ACIS.
- Daft, R. L., & Lane, P. (2005). The leadership experience (3rd). Mason, OH: Thomson-Southwestern.
- Davenport, T. H. (2006). Competing on Analytics. Harvard Business Review. 84: 98–107.
- Davenport, T. H., Barth, P., & Bean, R. (2012). How ‘big data’is different. MIT Sloan Management Review, 54, 43–46.
- Davenport, T. H., & Patil, D. J. (2012). Data scientist. Harvard business review, 90(5), 70-76.
- De Mauro, A., Greco, M., & Grimaldi, M. (2016). A formal definition of Big Data based on its essential features. Library Review, 65(3), 122-135.
- Demchenko, Y., Grosso, P., De Laat, C., & Membrey, P. (2013, May). Addressing big data issues in scientific data infrastructure. In Collaboration Technologies and Systems (CTS), 2013 International Conference on (pp. 48-55). IEEE.
- Dumbill, E. (2013). Making sense of big data.
- El-Kassar, A. N., & Singh, S. K. (2018). Green innovation and organizational performance: the influence of big data and the moderating role of management commitment and HR practices. Technological Forecasting and Social Change.
- Erevelles, S., Fukawa, N., & Swayne, L. (2016). Big Data consumer analytics and the transformation of marketing. Journal of Business Research, 69(2), 897-904.
- Erevelles, S., Horton, V., & Fukawa, N. (2007). Imagination in marketing. Marketing Management Journal, 17(2), 109-119.
- Fisher, D., DeLine, R., Czerwinski, M., & Drucker, S. (2012). Interactions with big data analytics. interactions, 19(3), 50-59.
- Garmaki, M., Boughzala, I., & Wamba, S. F. (2016, June). The effect of Big Data Analytics Capability on Firm Performance. In PACIS (p. 301).
- George, G., Haas, M. R., & Pentland, A. (2014). Big data and management. Academy of management Journal, 57(2), 321-326.
- Gunasekaran, A., Papadopoulos, T., Dubey, R., Wamba, S. F., Childe, S. J., Hazen, B., & Akter, S. (2017). Big data and predictive analytics for supply chain and organizational performance. Journal of Business Research, 70, 308-317.
- Gupta, M., & George, J. F. (2016). Toward the development of a big data analytics capability. Information & Management, 53(8), 1049-1064.
- Hagel, J. (2015). Bringing analytics to life. Journal of Accountancy, 219, 24–25.
- Havens, T. C., Bezdek, J. C., Leckie, C., Hall, L. O., & Palaniswami, M. (2012). Fuzzy c-means algorithms for very large data. IEEE Transactions on Fuzzy Systems, 20(6), 1130-1146.
- Hurley, R. F., & Hult, G. T. M. (1998). Innovation, market orientation, and organizational learning: an integration and empirical examination. The Journal of marketing, 42-54.
- Hurwitz, J., Nugent, A., Halper, F., & Kaufman, M. (2013). Big data for dummies. John Wiley & Sons.
- Hynes, N. (2009). Corporate culture, strategic orientation, and business performance: New approaches to modeling complex relationships. Technological Forecasting and Social Change, 76(5), 644-651.
- Intel, I. T. Center.(2012). Big Data analytics. Intel’s IT manager survey on how organizations are using big data.
- Ji-fan Ren, S., Fosso Wamba, S., Akter, S., Dubey, R., & Childe, S. J. (2017). Modelling quality dynamics, business value and firm performance in a big data analytics environment. International Journal of Production Research, 55(17), 5011-5026.
- Kaur, N., & Sood, S. K. (2017). Dynamic resource allocation for big data streams based on data characteristics (5Vs). International Journal of Network Management.
- Kim, G., Shin, B., & Kwon, O. (2012). Investigating the value of sociomaterialism in conceptualizing IT capability of a firm. Journal of Management Information Systems, 29, 327–362.
- Kiron D., Prentice P.K., Ferguson R.B. (2012). Innovating with analytics. MIT Sloan Management. Review. 54(1), 47–52.
- Kwon, O., Lee, N., & Shin, B. (2014). Data quality management, data usage experience and acquisition intention of big data analytics. International Journal of Information Management, 34(3), 387-394.
- LaValle, S., Lesser, E., Shockley, R., Hopkins, M. S., & Kruschwitz, N. (2011). Big data, analytics and the path from insights to value. MIT sloan management review, 52(2), 21.
- Manyika, J., Chui, M., Brown, B., Bughin, J., Dobbs, R., Roxburgh, C., & Byers, A. H. (2011). Big data: The next frontier for innovation, competition, and productivity.
- McAfee, A., Brynjolfsson, E., Davenport, T. H., Patil, D. J., & Barton, D. (2012). Big data: the management revolution. Harvard business review, 90(10), 60-68.
- Microsoft, (2013). The Big Bang: How the Big Data Explosion Is Changing the World.
- Morabito, V. (2015). Big data and analytics: strategic and organizational impacts. Springer.
- O'Leary, D. E. (2013). Artificial intelligence and big data. IEEE Intelligent Systems, 28(2), 96-99.
- Oracle, (2012). Big Data for the Enterprise. Redwood Shores, CA: Oracle
- Prajogo, D. I., & Ahmed, P. K. (2006). Relationships between innovation stimulus, innovation capacity, and innovation performance. R&D Management, 36(5), 499-515.
- Raguseo, E., & Vitari, C. (2018). Investments in big data analytics and firm performance: an empirical investigation of direct and mediating effects. International Journal of Production Research, 1-16.
- Ross, J. W., Beath, C. M., & Quaadgras, A. (2013). You may not need big data after all. Harvard Business Review, 91(12), 90
- Russom, P. (2011). Big data analytics. TDWI best practices report, fourth quarter, 19, 40.
- Santhanam, R., & Hartono, E. (2003). Issues in linking information technology capability to firm performance. MIS quarterly, 125-153.
- Schein, E. H. (1990). Organizational Culture: What it is and How to Change it. In Human resource management in international firms (pp. 56-82). Palgrave Macmillan, London.
- Tan, K. H., Zhan, Y., Ji, G., Ye, F., & Chang, C. (2015). Harvesting big data to enhance supply chain innovation capabilities: An analytic infrastructure based on deduction graph. International Journal of Production Economics, 165, 223-233.
- Wamba, S. F., Akter, S., Edwards, A., Chopin, G., & Gnanzou, D. (2015). How ‘big data’can make big impact: Findings from a systematic review and a longitudinal case study. International Journal of Production Economics, 165, 234-246.
- Wamba, S. F., Gunasekaran, A., Akter, S., Ren, S. J. F., Dubey, R., & Childe, S. J. (2017). Big data analytics and firm performance: Effects of dynamic capabilities. Journal of Business Research, 70, 356-365.
- Wang, Y., Kung, L., & Byrd, T. A. (2016). Big data analytics: Understanding its capabilities and potential benefits for healthcare organizations. Technological Forecasting and Social Change.
- White, M. (2012). Digital workplaces: Vision and reality. Business information review, 29(4), 205-214.
- Xu, Z., Frankwick, G. L., & Ramirez, E. (2016). Effects of big data analytics and traditional marketing analytics on new product success: A knowledge fusion perspective. Journal of Business Research, 69(5), 1562-1566.
Copyright information
This work is licensed under a Creative Commons Attribution-NonCommercial-NoDerivatives 4.0 International License.
About this article
Publication Date
28 January 2019
Article Doi
eBook ISBN
978-1-80296-053-2
Publisher
Future Academy
Volume
54
Print ISBN (optional)
-
Edition Number
1st Edition
Pages
1-884
Subjects
Business, Innovation, Strategic management, Leadership, Technology, Sustainability
Cite this article as:
Karaboga, T., Zehir, C., & Karaboga, H. A. (2019). Big Data Analytics And Firm Innovativeness: The Moderating Effect Of Data-Driven Culture. In M. Özşahin, & T. Hıdırlar (Eds.), New Challenges in Leadership and Technology Management, vol 54. European Proceedings of Social and Behavioural Sciences (pp. 526-535). Future Academy. https://doi.org/10.15405/epsbs.2019.01.02.44