Abstract
In this study we investigate the relationship between the DJIA and the KLCI. Extreme movements in the DJIA and KLCI are compared and we examine the extent and magnitude of extreme movements in the KLCI as triggered by extreme movement in the Dow Jones. The data consists of historical indices for DJIA and KLCI. Due to the difference in the ranges of the KLCI and DJIA indices, percentage return is used for comparison. Pearson’s correlation coefficient was used to determine the strength of the relationship between the two variables, followed by fitting a linear equation. Using hypothesis testing, extreme movements in returns of KLCI and DJIA are also compared Result shows there exist a moderate relationship between KLCI and DJIA. In particular, we found that regardless of the magnitude of change in both the DJIA and KLCI, the two indices move in the same direction more often than in opposite directions. Thus, KLCI traders should consider this finding when trying to predict directional changes in the KLCI with respect to DJIA movements.
Keywords: Percentage returnDow Jones industrial averageKuala Lumpur composite index
Introduction
The Kuala Lumpur Composite Index (KLCI), now known as the FTSE Bursa Malaysia KLCI, is a stock market index generally accepted as the barometer of the Malaysian stock market. It was introduced in 1986 to satisfy the need for a stock market index which would serve as an accurate performance indicator of the Malaysian stock market as well as the economy. It contains companies from the Main Board such as Malayan Banking, CIMB Group Holdings, Public Bank, Sime Darby, Axiata Group, Genting, Tenaga Nasional, IOI Corporation, PETRONAS Chemical Group and Digi.Com analyze the impact of interest rates and exchange rate on volatility of different sectors and composite indices in Istanbul stock exchange (Vardar, Aksoy & Can, 2008). Results show strong power of prediction of the two variables on the volatility of the composite index.
The Dow Jones Industrial Average was created on May 26, 1986 and named after Charles Dow, nineteenth century Wall Street Journal editor and co-founder of Dow Jones & Company. At that time, there were only twelve stocks in the index, representing the industrial component of America’s stocks markets. Furthermore, some alternative explanations for spill over phenomenon between markets are developed based upon behavioural financial theory, which assumes that the information and the characteristics of market participants systematically affect individual investment decisions and market outcomes (Fung & Lam, 2009). The Dow Jones Industrial Average (DJIA) is one of the most closely-watched benchmark indices. It shows how 30 American-based large and publicly owned companies have traded during a standard trading session in the stock market. The companies are Alcoa, Allied Signal, American Express, Boeing, Caterpillar, Chevron, DuPont, Disney, Kodak, General Electric, General Electric, General Motors, Goodyear, Hewlett-Packard, IBM, International Paper Co. , J.P. Morgan, Johnson & Johnson, Coca Cola, McDonalds, 3M, Philip Morris, Merck, Procter and Gamble, Sears, AT&T, Travlers, Union Carbide, United Technologies, Exxon and WalMart. However, due to the unique characteristics of China’s stock market, Huang and Bacon (2009) found difficulties in ascertaining that the nine-percent fall in the SSE Composite Index was indeed the sole trigger for the subsequent $1.5 trillion global market shake out. It is a price-weighted average and gives more emphasis on higher-priced stocks without regard for market capitalization and size of the industry, unlike the KLCI.
In August 2017,
The formula to calculate return for this study is:
This study focuses on the daily data of historical indexes for DJIA and KLCI from year 2013 to 2016. We use percentage return to find the comparison between DJIA and KLCI indexes in order to get the relationship between the Malaysia and the U.S stock market. The result from the percentage return of DJIA and KLCI is used to predict the relationship between DJIA and KLCI when there are extreme movements in the Dow Jones.
The finding from this study can provide information to the investor to predict what happen to local stock market in details. Besides that, the study of the extreme movement on the DJIA also can give ideas to other researcher on the relationship between DJIA and KLCI and mathematical concept.
Problem statement
In this project, we examine the relationship between the Kuala Lumpur Composite Index (KLCI) and the Dow Jones Industrial Average (DJIA). Dow Jones is a weighted average of the stock prices of 30 important companies that trade on the New York Stock Exchange. It is common knowledge that the performance of the Dow Jones affects many indices worldwide, including indices in Malaysia. Mathur and Subrahmanyam (1990) found that the Denmark market was affected by the U.S. market, as demonstrated by Granger's causality concept. Hence, the Dow Jones is the stock exchange that traders worldwide usually analyse in detail, in anticipation of trends.
The Dow Jones is widely tracked also because it is an indicator of the health of the global stock market. The Dow includes large-capitalization companies representative of the U.S. industry. Hamao, Masulis and Ng (1990) investigated how price changes in one market influenced the opening prices in the next market to trade and whether changes in price volatility in one market were positively. When US subprime loan crisis worsened in mid-2007, Dow Jones took a deep plunge and so did Asian markets including the KLCI. However, it is noted that the subsequent recovery of the Dow Jones helped to rally other stock markets worldwide including the KLCI, thus aiding with economic recovery both on a macroeconomic and microeconomic scale.
However, the KLCI is still under-performing if we compare it with other Asian stock indices like the Hang Seng Index, the Straits Times Index and the Nikkei 225 index. If the Dow Jones continues performing well, we expect the performance of the KLCI to improve as well. A demand forecasting and production planning for high seasonal demand situations had been explored by Yenradee, Pinnoi, and Charoenthavornying (2001). Three forecasting techniques, Holt-Winter’s, decomposition ARIMA are applied to this study.
Hence, our research is concerned with the relationship between the Dow Jones Industrial Average and the KLCI, so as to assist traders in predicting changes in the KLCI based on extreme movements in the Dow Jones. This will help KLCI traders manage their shares when confronted with extreme movements in the Dow Jones, so as to be well-prepared to face a likely extreme movement in the same direction in the KLCI. Our research will also examine the extent and magnitude of extreme movements in the KLCI triggered by similar movements in the Dow Jones.
Research Questions
The purpose research questions are as follows:
What are the relationship between the Dow Jones Industrial Average and the Kuala Lumpur Composite Index, in particular when there are extreme movements in the Dow Jones.
What are the extension and magnitude of extreme movements in the KLCI as triggered by extreme movements in the Dow Jones.
Purpose of the Study
Since both of the countries are members of Trans Pacific Partnership Agreement (TPPA) country. It will show that the movement stock market for both countries. The objective of this study is to examine the relationship between the DJIA and the KLCI. Furthermore, extreme movements in the DJIA and KLCI are compared and we investigate the extent and magnitude of extreme movements in the KLCI as triggered by extreme movement in the Dow Jones.
Thus, with unstable economic situation nowadays, this can affect to the stock market. When the stock price is decreasing, it can give bad assumption to the government. Indirectly, it can shrink the economy.
This research will provide valuable information to the investors to predict what happen to local stock market. Therefore, they can improve their company strategy. At the same time, they can increase profit for their company.
Research Methods
Obtaining the data:
The data obtained from Blomberg website for KLCI and DJIA. Both indices were secondary data and were exported into Microsoft Excel. There is time difference between USA and Malaysia. New York is 12 hours behind Kuala Lumpur. Therefore to see how KLCI is influenced by changes in DJIA, we compared KLCI with DJIA a day earlier.
Calculate the percentage return.
Due to the difference in the ranges of the KLCI and DJIA indices, percentage return is a more appropriate comparison. Below is the formula to calculate rate of return where is final value for the indices and is initial value for the indices:
We divide the percentage return into six group based on values.
Find the Pearson’s correlation (R2).
Using the percentage returns of both indices we find . It is important to measure the relationship between two variables. According to Boslaugh (2016), the Pearson correlation coefficient is a measure of linear association between two interval- or ratio-level variable. Values closer to +1 indicate a positive linear relationship. Values closer to -1 indicate a negative linear relationship.
Linear Equation Method.
Linear equation is used to fit data to obtain a mathematical relationship between two variables
Hypothesis testing
Hypothesis testing is used here to compare extreme movements in returns of KLCI and DJIA. The test procedure is as below:
State the hypotheses.
Null hypothesis;
Alternative hypothesis;
Where;
: First proportion of sample to be tested
: Second proportion of sample to be tested
Formulate an analysis plan.
Using sample data earlier we complete the following computations to find the test statistic and its associated
Where;
: First proportion of sample to be tested
: Second proportion of sample to be tested
: Sample size of first percentage return
: Sample size of opposite percentage return
Next we need to find the standard deviation. Compute the standard deviation of the sampling distribution for difference between two proportions.
Then we can make a test statistic. The test statistic is a
When the
5.6. Test the null hypothesis against the alternative hypothesis (at ) for various filter sizes of .
State the hypotheses.
Null hypothesis;
Alternative hypothesis;
where is the proportion of sample with the same direction for both DJIA and KLCI
Formulate an analysis plan.
where
: The proportion of sample with the same direction for both DJIA and KLCI
: The proportion of sample with the opposite direction for both DJIA and KLCI
: Observed sample proportion (number of success divided sample size)
: The proportion of sample with opposite direction for both DJIA and KLCI
N: sample size where both DJIA and KLCI are in the same filter size of percentage return
After all the calculation complete, we can make a test statistic. When the z-value is greater than 5% significance level, reject the null hypothesis.
Findings
Table below describe the percentage return for each group and all the value.
Table
We only choose top two highest to do fitting of linear equation, i.e. and all .
Table
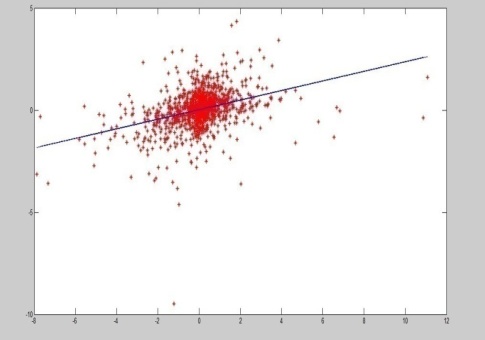
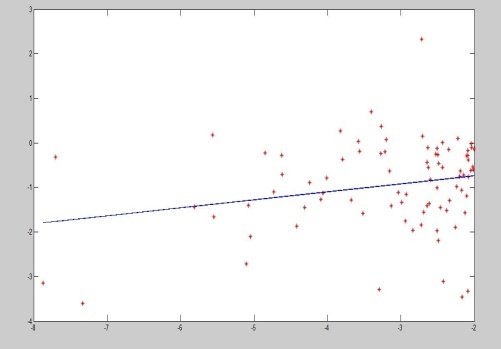
Figure
Result from testing of null hypothesis against the alternatives hypothesis (at ) for various filter sizes of .
From the earlier calculations involving two-tailed tests comparing the two indices, we saw that for the cases of positive return and opposite direction return, we did not reject any of the null hypotheses. This means that if it is known that both the DJIA and the KLCI have positive returns or returns in opposite directions, we have no statistical evidence to predict a particular KLCI return magnitude as being more likely, at 5% significance level. But, when there is negative return, we can make the deductions listed below, meaning that the following proportions differ significantly at α=0.05 between the Dow Jones Industrial Average and the Kuala Lumpur Composite Index.
versus with both DJIA and KLCI having negatives rates of returns.
versus with both DJIA and KLCI having negatives rates of returns.
versus with both DJIA and KLCI having negative rates of returns.
In particular, we can further deduce that when both DJIA and KLCI have negative returns, it is far less likely that KLCI will have a change of magnitude of less than 1%.
Result from testing null hypothesis against the alternatives hypothesis (at ) for various filter sizes of .
From the calculations involving upper-tailed tests investigating the relative frequency at which the two indices moved in the same direction as compared to movement in opposite directions, with respect to different filter sizes based on the rate of return, we found that the null hypothesis was rejected for all of 4 cases. This means that regardless of the magnitude of change, the two indices move in the same direction more frequently than in opposite directions. This conclusion is useful for KLCI traders trying to predict directional changes in the KLCI with respect to changes in the DJIA.
Conclusion
This research was undertaken to see the comparison between the DJIA and KLCI. The research findings provide important information for KLCI stock holders to manage their stock.
From the finding of study, this data will help to investors in order to know the relationship between KLCI and DJIA. This is very important because since Malaysia government with US government agreed in the Trans Pacific Partnership Agreement (TPPA). TPPA is a trade agreement between Australia, Brunei Darussalam, Canada, Chile, Japan, Malaysia, Mexico, New Zealand, Peru, Singapore, the United States (until 23 January 2017) and Vietnam.
References
- Boslaugh, P. A. (2016). Statistics In A Nutshell: A Desktop Quick Reference,. S.L.: Shroff & Dist.
- Christodoulos, C., Michalakelis, C., & Varoutas, D. (2010). Forecasting with limited data: Combining ARIMA and diffusion models. Technological Forecasting and Social Change, 77(4), 558-565.
- DJIA. (n.d.). In Bloomberg. Retrieved April 8, 2017, from https://www.bloomberg.com/quote/INDU:IND
- Drakos, K., & Kutan, A. M. (2005). Why do financial move together? An investigation of Greek and Turkish markets. Eastern European Economics Journal, 5-26.
- Fung, A. K. W., & Lam K. M. (2009). Overreaction of index futures in Asia to U.S market performance-evidence of behavioral biases? Journal of Empirical Finance.
- Hamao, Y., Masulis, R. W., & Ng, V. (1990). Correlations in price changes and volatility across International Stock Markets. The Review of Financial Studies, 281-307.
- Huang, Y. J. and Bacon, F. W. (2009). Can the US stock market be Shanghaied? Management Research News, 469-476.
- KLCI. (n.d.). In Bloomberg. Retrieved April 8, 2017, from https://www.bloomberg.com/quote/FBMKLCI:IND
- Mathur, L., & Subrahmanyam, V. (1990), Interdependencies among the Nordic and U.S stock markets. The Scandinavian Journal of Economics, 587-597.
- Yenradee, P., Pinnoi, A. & Charoenthavornying, A. (2001). Demand forecasting and production planning for highly seasonal demand situations: Case study of a pressure container factory. Journal of the Science Society of Thailand. 27, 271-278.
- Vardar, G., Aksoy, G., & Can, E. (2008). Effects of interest and exchange rate on volatility and return of sector price indices at Istanbul stock exchange. European Journal of Economics. 11, 126-35.
Copyright information
This work is licensed under a Creative Commons Attribution-NonCommercial-NoDerivatives 4.0 International License.
About this article
Publication Date
31 July 2018
Article Doi
eBook ISBN
978-1-80296-043-3
Publisher
Future Academy
Volume
44
Print ISBN (optional)
-
Edition Number
1st Edition
Pages
1-989
Subjects
Business, innovation, sustainability, environment, green business, environmental issues, industry, industrial studies
Cite this article as:
Muainuddin, M. M. A. M., Hasan, M. N., & Kadir, M. H. A. (2018). Foreign Bilateral Relations Between Usa And Malaysia, From Djia And Klci Perspectives. In N. Nadiah Ahmad, N. Raida Abd Rahman, E. Esa, F. Hanim Abdul Rauf, & W. Farhah (Eds.), Interdisciplinary Sustainability Perspectives: Engaging Enviromental, Cultural, Economic and Social Concerns, vol 44. European Proceedings of Social and Behavioural Sciences (pp. 692-701). Future Academy. https://doi.org/10.15405/epsbs.2018.07.02.74