Abstract
Asset Price movements indicate financial health of an economy because of the serious affects these have on financial indicators. This study is carried out to see the behaviour of asset price movements in different time horizons in the presence of leading economic indicators. The objective of this study is to track asset price movements and the impact of economic indicators on asset prices in different time horizons. This study intends to find out the appropriate time horizon for predicting asset price buildup that can lead to a potential financial crisis. Uni-variate and Multi-variate Logit Regression Analysis is performed in this study to be applied in four different time horizons. Results show that Real Gross Domestic Product, Credit to private sector, Interest rates of Long and Short term, Gross Domestic Product-deflator, the Consumer Price Index, Real effective exchange rates and nominal effective exchange rates are the significant early warning indicators for predicting the future financial crisis in the economy of Pakistan in longer time horizon as they affect asset price buildup in the long time periods. This study shows that asset prices contain more information in long run and can be effectively used as a tool to predict financial crises due to asset price misalignments;
Keywords: Price BubbleTime HorizonAsset PricesFinancial Crisis
Introduction
In the history of asset markets, no event has repeated itself more than the occurrence of price bubbles and their subsequent bust. It is seen that whatever policy or restrictions were followed to prevent asset price bust, it never ceased to stop. From time to time, price bubbles are formed. The simplest definition of a price bubble is the deviation of asset prices from their fundamental values. Again, to draw a conclusive opinion about the base value of an asset is quite difficult because asset markets continuously evolve and grow and are never (if it is the right term) peaceful or efficient as is hypothesized. There are always price deviations from their fundamentals and this is how asset markets function. An efficient asset market is just a hypothesis where every asset performs to its fullest incorporating all the information which happens to be correct.
Moving towards reality, asset markets face price build-up with subsequent price bust due to one very important factor as illustrated by (Queiroz, Medeiros, & Neto, 2011). They say that it is largely the investor behaviour which causes asset prices to deviate. Investors do not like unfavourable price fluctuations and rely on the market information and historic trends, thus causing price movements. This happens in asset markets all the time but it will not be correct to put all the blame on investors’ behaviour. There are other factors involved that lead to potential price build up which can become a financial crisis. According to (Loayza & Rancière, 2006)weak economic conditions create financial fragility which can be strengthened in the presence of volatile asset markets. Thus, a weak economy is also a major cause of price bubble formation. In this line, (Sarno & Taylora, 1999) found the evidence of asset price bubbles formation and burst because of presence of large amount of money in asset markets. (Gerdesmeier, Reimers, & Roffia, 2010) study asset prices with money and credit along with other macroeconomic variables and indicate that actually, asset prices are also affected by economic indicators. (Batool, 2016) also confirms the results saying that asset prices are affected by major macroeconomic indicators even in transition economies. This further confirms that asset prices are affected by many factors.
All the historical evidences point out to one important factor, “Time”. All the asset pricing models incorporate time horizon, the appropriate time period necessary for asset prices to take in information and perform. But the asset pricing theories always suggests that agents have full information. According to (Blanchet-Scalliet, El Karoui, & Martellini, 2005), asset markets face timing risk which induces uncertainty in the market. Uncertainty increases with time as in long time horizons, everything is variable while in short time horizons, some factors are fixed and some are not. So time horizon has its importance. It is important to include time horizon to predict the market conditions. If market condition is well known, it helps predict unfavourable price build up. Once a pattern is predicted, then it becomes easy to regulate the market in advance to prevent any financial crisis from occurring. Unfavourable price fluctuations can be predicted or not, is very important to know. In this regard (Komarek & Kubicová, 2011) state that to spot asset price rise and fall is not easy as it is very difficult to measure the base price of an asset in the complex web of asset markets and also because it is rather difficult to know how much prices have deviated because markets are not operating in ideal conditions. But something can still be done. A time horizon can be determined to know when bubbles start to form. (Henkel, Martin, & Nardari, 2011) put predictions in markets in the context of recession and expansion cycles and state that these cycles are involved in predicting upcoming market conditions. They argue that in the longer time horizons, market indicators contain more information as compared to short time horizons. Also, the recession cycle can provide with more predictability than expansion periods. It is because during expansion, more information circulates in the market which makes it difficult to predict future events as the indicators are less smooth.
This could mean that price bubbles prompt in a specific time and not always random or all of a sudden. What time period it is? How can it be specified and what can be done to prevent this cycle from re-emerging are important questions and need answers. Literature on time, as an important factor in price bubble formation, is scarcer and needs attention. This study focuses on the aspect of time in predicting price build-ups
Problem Statement
Asset markets are never stable, they always change. Sometimes, this change results in overpricing and causes crashes in markets, which then lead to financial crises. There have occurred many financial crises. The intensity of these crises has grown and it seems, just like climate change, changes in asset markets are also warming up. Since the history of financial crashes revealed involvement of asset markets, much research has been done to control and regulate asset prices. Many asset pricing models and theories have been presented to regulate the markets. Prediction of price bubbles has also been an important subject of research and much important findings have been generated in this regard. There are various assets and each has its own fundamentals and values to study. This study takes into account stocks and gold prices and strives to see what causes the bubble formation in these markets.
Stock and Gold Price Fluctuations
As stated earlier, the extreme price deviation of an asset from its fundamental value makes price bubbles. These bubbles burst and send ripples in the entire economy and make a financial crisis. Stock markets are one of the most busy asset markets across the globe. So much of stock volume is traded every day and this makes it one of the most volatile markets as well. (BATES, 1991) states that in stock markets, bubbles are most of the time rational. By rational bubbles, author means that development in stock markets is almost visible to the investors who know when stocks are overpriced. Overpricing means at some future point prices will have to come down and this is very easily spotted in share markets. Author also indicates that even though the bubble is rational, it bursts when prices come down. The same is discussed by (Chen, Hong, & Stein, 2001), who further this in their study and state that the volatility in stock markets is linked with the price fall. Authors say that in stock markets, it is the price melt-down rather than price build up that injects volatility in the market. It is also observed that any information in form of good news or bad news enters in the market, it increases risk premium which investors do not like and thus the price fall happens. Since stock markets are very busy and tons of trade happens daily, this means that stock markets are more volatile as more business is done there. Volatility means stock markets are not stable. (Eraker, 2004) also talks about volatility in share markets and states that this volatility increases over time. With a longer time horizon, volatility is observed to have increased with the change in share prices. Author also says that this happens due to increased uncertainty about share prices. This is a very interesting find because it incorporates factor of time along with price bubbles. With such bubbles present in stock markets, it can be said that many crashes must happen in share markets but this is not so. (Gilchrist, Himmelberg, & Huberman, 2005) share a very interesting trend in stock markets and state that actually the price hike or the bubble is normalized in share markets with the issuance of new shares. When the share prices rise, new issues are released causing the overall price rise to settle down a bit. Authors also say that dispersion in investors’ beliefs is also a reason why price bubbles are formed but do not end up as harmful. As for the Gold markets, (Lili & Chengmei, 2013) say that gold prices are negatively affected by macroeconomic factors as well as financial market indices. So, when an economy is facing hard times gold prices rise and vice versa. As for the prediction of gold prices, (Pierdzioch, Risse, & Rohloff, 2014) state that gold prices are linked to business cycles. According to the study, the ups and downs in the gold prices are predictable by understanding the trends in gold markets.
The factor of Time in Asset markets
In Asset markets, all the transactions are time bound yet the data on role of time in asset markets in least available. Even though multiple studies indirectly include time, but the direct effects of time are still not well known. (Friedman, Laibson, & Minsky, 1989) talk about markets crashes and price bubbles with respect to time and say that once a market crash happens, it indicates a larger price bubble over a longer time period. Such a bubble burst happens over long time horizon. Authors argue that this happens due to more information adds up in long run which can be used as a tool to predict price bubble formation and subsequent bust. It can be ad that time affects assets by affecting some market variables. This is presented in the study by (Ball, Cecchetti, & Gordon, 1990) who say that actually it is the uncertainty caused by inflation that affects markets. And this affect intensifies in the longer time periods than short time periods. Which means that in short time horizons, uncertainty does not unleash its severity to the fullest and it can only be observed in the long run. (Blanchet-Scalliet et al., 2005) also link uncertainty with market risk and timing risk and state that when a time horizon is unknown, i.e. the time of the occurrence of an event is unknown, market risk increases. Thus time horizon is important in understanding the behaviour of market to predict any negative financial happenings. In a very interesting study (Cecchetti, Lam, & Mark, 1990) state that asset prices revert to their mean values over time. But how much time does it take? They say that this cannot be seen in just five to ten years of time period as asset prices fluctuate and go up and down. So to go back to their mean, takes probably a longer time period. This study leaves with inference that there exists times, where asset price fluctuations can be captured or predicted beforehand. If such a time can be captured it can help avoid any potential crisis from happening.
Another interesting aspect in this regard is explored by (Gordon , 1998) and (Henkel et al., 2011), they talk about time in markets with respect to recession and expansion cycles. Author says that markets which experience fast business or more expansion cycles, the prices decline there. And in such markets, it becomes rather difficult to predict any future crises. Author also takes the research in the dimension of business cycles and state that among these is that time horizon which can lead to predicting and preventing financial crises in economy in general. An economy comprises of many variables an assets and each have their own effects on it. (Goodhart & Hofmann, 2000) relate assets with inflation and state that a shorter time horizon of two years in not enough to study the relationship of house prices with inflation. The full impact of these can be studied over a longer time horizons which can lead to other interesting insights.
Markets operate with information. This information can be of any sort and timing of this information and its impact is very important. This area is explored by (Ehrmann & Fratzscher, 2005) who say that an unexpected information in the market lasts longer. Normally, information is linked with short time horizon as it is incorporated in asset prices there and then. But an unexpected information remains in the market for longer and it remains there and can be detected over long time horizons.
Razen, Huber, & Kirchler, (2017) explain the working of asset markets with respect to the relative lives of the assets. They say that asset markets where trading horizons are longer and assets have longer lives like shares etc., these markets are more prone to price bubble formation. This study shows that not all assets cause financial cries, rather more tradable assets are more prone to such attacks and only in a longer time horizon is it possible to attacks and only in a longer time horizon is it possible to see a possibility of bubble formation. (Noussair, 2017) furthers the life of assets in his study and states that those assets with linger lives see a price fall over longer time horizons. This characteristic makes it possible to predict any dangerous price formation.
The research on importance of time in asset markets suggests the important things. First, information is the most important factor related to time. It is the degree of information seriousness that prevails over longer time horizons and changes investors’ beliefs. Second, assets have their lives too. The longer the life an asset has, the more chances are there to predict the future events in these markets.
Research Questions
Asset markets behave differently in different time spans. It is important to know which time period contains more information about the assets and how much that information can be used to predict financial crises. Keeping in mind this, the present study strives to answer the following question.
Purpose of the Study
The purpose of this study is to learn the importance of time horizon in predicting financial crises caused by extreme asset price fluctuations. This study is important in this regard that once an appropriate time horizon is known, the price patterns in asset markets can be mapped somewhat easily. But which time period should be taken as the benchmark is the real question as previously; all the research compares short and long time horizons in rather subjective terms. This study aims to find out that “appropriate” time horizon by undertaking all the available and necessary market information.
Research Methods
Asset prices have been studied under different macroeconomic indicators. Mostly, inflation and interest rates are taken as main incidents in this regard. The present study includes Real GDP, GDP Deflator, M2 (broad money aggregate), the interest rates of long and short time periods, the real and nominal effective exchange rates, credit to private sector and consumer price index to be tested along asset prices as independent variables. The dependent variable consists of changes at all in the variables
Asset price Bust Identification
The main focus of this study is to identify asset price busts in asset markets with respect to time; this study uses the asset price indicator developed by (Gerdesmeier et al., 2010). This indicator will serve as dependent variable in this study to check the cause and impact relationship of asset prices with macroeconomic variables. The composite indicator ΔC includes share prices and gold prices, presented mathematically as;
ΔC = ϕ1ΔShare Pricest + ϕ2Δ Gold Pricest
Where, ΔC indicates fluctuation in asset prices. Every time a bust occurs, ΔC falls below a pre-set value. This pre-set value is this case is the peak value of asset prices in the given time period. Asset price bust will occur when ΔC drops or becomes equal to a pre-set threshold in the said time period compared to its highest value in the same time period (Gerdesmeier et al., 2010), it is represented mathematically as;
D t=1 iff ΔCt+l ≤ (Mean of ΔC - λσ ΔC) Where t is from 1 to t+l
D will be equal to 1 whenever ΔC will drop from its mean minus standard deviation of ΔC from time 1 to t+l. where λ represents a constant, and for this study, it is normalized to 1. This indicates the current price bust. To predict an asset price bust ahead of time, this study follows (Berg & Pattillo, 1999), who created a dummy variable C to indicate a price bust head of time based on current market scenario. The dummy variable C is constructed as represented mathematically as;
C t= 1, Iff Σ Dt+g > 0; where g=1 to i
Note that, C depend on dummy variable D, i.e. if in a certain time period if summation of D is greater than zero, it indicates presence of a price bust in future. In case of present study, four months, six months, twenty four months and sixty months are used as the testing time horizons. All necessary calculations are done in these time horizons. Now, if Dummy variable C signals for a price bust in future, but dummy variable D does not indicate so, then this will be counted as false signal. But not necessarily a wrong signal due to the fact that asset prices are affected even by the investors’ behaviour. Therefore, the notion of wrong signals is not being used in the present study.
After the predictions based on asset prices are made, next come the affect the economy put on asset prices. To understand this cause and effect relationship, this study uses binary regression model, Logit (logritham + Unit) regression analysis. Logit regression is used to predictive analysis and generates results as probability of the occurrence of an event. The reason to use logit regression is to check out the possibility of occurrence of price bust in the presence of various independent variables in the tested time period. The general form of logit regression model is as follows;
Logit (Cik = 1) = βo + βit . Xik + εik
Where, Xit represent independent variables, βik represent respective coefficients, and εik represents the error term. Fundamental variables are used as growth rates. Logit regression is first applied to individual variables in all four time horizons to scoop out the significant variables. Then multi-variate logit regression is applied on the significant variables in all four time horizons to check the collective cause and effect of independent variables on the dependent variable.
Findings
This chapter contains results calculated based on the proposed methodology. The present study developed a composite indicator “C” comprised of two assets; Stock prices and Gold prices. The composite indicator serves as the dependent variables which are to be tested for different time horizons in the presence of leading economic indicators.
Occurrence of Bust
To indicate the bust occurrence of asset prices, present study developed a bust indicator which is shown as a dummy variable D. This dummy variable is then studied in different time horizons which in this study are 6 months, 1 year (12 months), 2 (24 months) years and 5 years (60 months). The dummy variable D shows the occurrence of asset price bust in the selected time horizons with respect to the maximum value of assets in the same time period. Table
Table
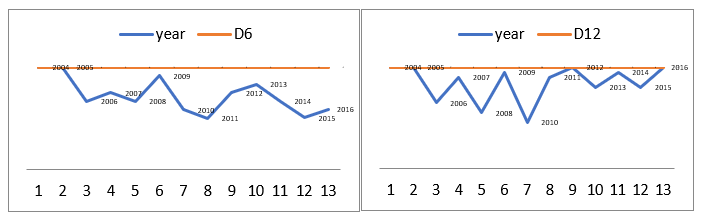
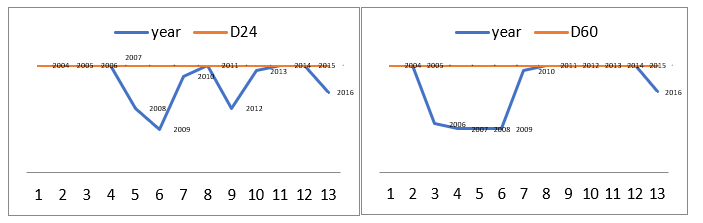
To predict the price busts ahead of time, i.e. in the future, the composite indicator C is tested in the proposed time horizons to check if any price busts can be predicted ahead of time effectively. Table given below presents the comparison.
When compared with Table
![[Bust Prediction in 6 months]](https://www.europeanproceedings.com/files/data/article/78/3072/AIMC2017F73.fig.003.jpg)
![[Bust Prediction in 12 months]](https://www.europeanproceedings.com/files/data/article/78/3072/AIMC2017F73.fig.004.jpg)
![[Bust Prediction in 24 months]](https://www.europeanproceedings.com/files/data/article/78/3072/AIMC2017F73.fig.005.jpg)
![[Bust Prediction in 60 months]](https://www.europeanproceedings.com/files/data/article/78/3072/AIMC2017F73.fig.006.jpg)
To know more, Logit regression analysis is applied to understand the behaviour of asset prices in the presence of macroeconomic variables.
Logit Regression analysis
In order to check how much are asset prices affected by the factors in the economy, univariate and multivatriate logit regression is applied to the data. Since this study’s main focus is on the effect of time horizon, therefore the logit regression test is performed on all four time periods. The results are shown in the comparative table
The tables represent the uni variate logit regression analysis of the selected variables. The tables contain z stat, and their corresponding p-values. The reason p values are included is to help out in the comparison of all four time horizons. The McFadden R-squared values tell the probability of a bust occurrence due to the said variables. In 6 months (Table C6), there are only two variables that affect asset prices significantly, Real-GDP (GDPR) and Monetary aggregate (M2). Even though, money does not show a strong effect on asset prices but it still leaves its impact compared to other variables in the same time period. In 6 months’ horizon, McFadden R-squared values for real-GDP and Money are greater than other variables and show greater likelihood of predicting crisis compared to other variables.
In 12 months’ time span (tables C12), the relatively significant variables are real-GDP, the Credit to the private-sector (CPS), the interest-rate for long term (LTIR) with a negative value, the interest rate for short term (STIR). The McFadden R-squared values for GDPR, CPS, LTIR and STIR are better than other variables and show greater fit and more likelihood of crisis prediction than other variables in the same time period.
In 24 months’ time span (Table C24), the variables that show significant behaviour are GDP-deflator (GDPD) and relatively significant variables are the stable interest rate (LTIR), M2 and the real exchange rate (REER) with negative values. McFadden R-squared values of GDP-deflator and long-term-interest-rate are more than other variables and show that their probability of predicting an upcoming crisis in the asset markets is more than other variables.
In the 60 months’ time span which is 5 years’ time period, the significant variable are the GDP-deflator, the consumer-price-index (CPI), the Credit to the private-sector (CPS), nominal-effective-exchange-rate (NEER) and Short interest rate (STIR). Whereas relatively considerable impact is exerted by the Real-GDP (GDPR), the stable rate of interest (LTIR) and the real exchange rate (REER). The McFadden R-squared values of GDP-deflator, Consumer-Price-Index, Credit-to-Private-Sector, REER, REER and STIR show that the odds of predicting the crisis in the long run of these variables are stronger.
The results show that Real-gross-domestic-product (GDPR) does not impact asset price movement much in the long run. But in longer time period, greater than five years, Real GDP might be an effective tool to adjust asset prices. GDP-deflator (GDPD) it grows stronger and leaves greater impact on asset price misalignments in the long run. Therefore, it can be said that inflation affects asset prices more in longer time horizon compared to shorter time horizons. Consumer-price-index (CPI) also leaves much impact on asset prices in the longer time periods as compared to shorter time periods. The other variables that grow stronger in affecting asset prices in the bigger time horizon are Credit-to-the-private-sector (CPS), Nominal-exchange-rate (REER) and the interest rate of the short term (STIR). The Stable interest rate (LTIR) and real-exchange-rate (REER) remain in the mild category and may cause asset price fluctuations to some extent. Money supply (M2) shows significant impact only in the shorter time horizon but remains ineffective in the long run. This can be due to strict monetary policy as increased money supply affects the economy adversely.
To understand the collective contribution of significant variables in all the time periods, multi-variate logit regression is applied. The results are shown in the tabular form below.
In 6 months’ time period, only two variables showed significance, Real gross domestic product (GDPR) and M2. When testes together, it is seen that money supply grows relatively less affective with GDPR but still leaves a considerable impact in bubble bust. The McFadden R-squared values rather small with almost 6 present probability of predicting an asset price bubble but this should not be neglected as in the very short run, with just two economic variables, a mere 6 present probability can be strengthened in the presence of other economic factors.
When the time horizon is extended to 12 months, the multivariate logit regression shows that with other variables, Real GDP and short term interest rate show more significant impact on asset price movements. The credit to the private sector and the stable interest rate also show somewhat significance. The McFadden R-squared value shows almost double the likelihood of predicting an upcoming price bubble bust in 12 months’ time span. This shows that as the time horizon widens, so are the economic variables ability to predict financial crisis caused by asset prices. In the 24 month time horizon, the multivariate logit regression analysis shows that the implicit price deflator for GDP (GDPD), the short term interest rate, the stable interest rate and real exchange rate show significance towards predicting a crisis caused by unfavourable asset price movement. The impact of short term interest rate, long term interest rate and real effective exchange rate increases on predicting crisis. The McFadden R-squared value indicates that possibility of crisis prediction with this set of variables increase in a two-year time period almost up to 20 present as compared to one year time span. Finally, the five year or sixty month multivariate logit regression results show that when tested as a group, credit to private sector becomes more significant followed by GDP deflator and short term interest rate. The McFadden R-squared increases to a hundred percent compared to 2 year time horizon.
Two interesting inferences can be drawn from these results. First, in short time horizon, the variables are not mature enough and individually or in a group, they might generate results inconsistent with the ongoing economic conditions. Also, short time horizon cannot be used as an appropriate time period to draw any conclusions. Secondly, as the time horizon expands, macroeconomic variables get mature and take in more information. Based on the overall market scenario and economic conditions, asset price fluctuations can show a pattern of bubble formation and bubble burst. This implies that the likelihood of predicting a financial crisis caused by asset price misalignments increases in the long run.
Conclusion
Time horizon is one of the most important factors in financial crisis prediction. In asset markets, price bubbles are formed due to many factors and these price bubbles sometimes transform into crises. In order to predict a potentially dangerous price bubbles, inclusion of time as an important factor is very necessary. Not much literature is found in this regard and that which is present usually takes into account the economic variables and their time-bound impact on economy and well as on asset markets. Previous research suggests that factor of time horizon can be found in two areas in asset markets. First, the information in the asset markets is not only a short time but also a long term phenomena. The degree of seriousness of the information determines if it’s going to prevail in the asset markets for how long. Second, the life of assets is also time bound. Those assets with longer trading horizon are prone to more price bubbles and in such markets it is easy to predict a future crisis. The assets with shorter lives are relatively hard to be used in predicting dangerous price fluctuations. The research strives to find out the importance of time horizon in predicting future financial crises caused by unfavorable asset price movements. Stock and Gold prices are combined to form a composite indicator to serve as asset price indicator to study the price changes while Nominal GDP, GDP deflator, Broad money Aggregate (M2), the Credit to the Private Sector, the stable interest rate, the Short Interest Rate, the Nominal Exchange Rate and the Real Exchange Rates are taken as the macroeconomic factors to study their impact on asset prices. The Cause and Effect relationship of these Variables is then checked in four different time horizons, to understand the turn of events over time. Uni-Variate and Multi-Variate Logit regression analysis is used as a tool to analyze the significance and role of these variables individually as well as in a group.
Results show that; Real GDP, credit in the economy, Interest Rates, inflation and, exchange rates are the significantly indicate and serve as early warning predictors for future financial crisis in the economy of Pakistan. The impact of these variables over asset prices is strong in the long time horizon as compared to shorter time horizons. The longest time horizon studied in the present study is 5 years or 60 months, and it shows the most accurate prediction period for unfavorable price buildups in share and Gold markets of Pakistan.
Since there is not much baseline literature available in this area of study, therefore much room is available for research here. The study can be further preceded by allowing more economic factors and inducing longer time horizons. It would be interesting to include Political Stability as a variable in different time horizons and to check ts impact on asset markets in unstable or leveraged economies.
References
- Ball, L., Cecchetti, S. G., & Gordon, R. J. (1990). Inflation and Uncertainty at Short and Long Horizons. Brookings Papers on Economic Activity, 1990(1), 215. https://doi.org/10.2307/2534528
- BATES, D. S. (1991). The Crash of ʼ87: Was It Expected? The Evidence from Options Markets. The Journal of Finance, 46(3), 1009–1044. https://doi.org/10.1111/j.1540-6261.1991.tb03775.x
- Batool, Z. (2016). ROLE OF MONEY AND CREDIT IN ASSET PRICE MOVEMENTS: A CASE STUDY OF PAKISTAN. International Journal of Innovative Knowledge Concepts, 4(7). Retrieved from http://www.ijikc.co.in/sites/ijikc/index.php/ijikc/article/view/225
- Berg, A., & Pattillo, C. (1999). Predicting Currency Crises: The Indicators Approach and an Alternative. Journal of International Money and Finance, 18(4), 561–586. https://doi.org/10.1016/S0261-5606(99)00024-8
- Blanchet-Scalliet, C., El Karoui, N., & Martellini, L. (2005). Dynamic asset pricing theory with uncertain time-horizon. Journal of Economic Dynamics and Control, 29(10), 1737–1764. https://doi.org/10.1016/j.jedc.2004.10.002
- Cecchetti, S. G., Lam, P.-S., & Mark, N. C. (1990). Mean Reversion in Equilibrium Asset Prices. The American Economic Review, 80(3), 398–418. Retrieved from http://links.jstor.org/sici?sici=0002-8282%28199006%2980%3A3%3C398%3AMRIEAP%3E2.0.CO%3B2-A
- Chen, J., Hong, H., & Stein, J. C. (2001). Forecasting crashes: trading volume, past returns, and conditional skewness in stock prices. Journal of Financial Economics, 61(3), 345–381. https://doi.org/10.1016/S0304-405X(01)00066-6
- Ehrmann, M., & Fratzscher, M. (2005). Exchange rates and fundamentals: new evidence from real-time data. Journal of International Money and Finance, 24(2), 317–341. https://doi.org/10.1016/j.jimonfin.2004.12.010
- Eraker, B. (2004). Do Stock Prices and Volatility Jump? Reconciling Evidence from Spot and Option Prices. The Journal of Finance, 59(3), 1367–1403. https://doi.org/10.1111/j.1540-6261.2004.00666.x
- Friedman, B. M., Laibson, D. I., & Minsky, H. P. (1989). Economic Implications of Extraordinary Movements in Stock Prices. Brookings Papers on Economic Activity, 1989(2), 137. https://doi.org/10.2307/2534463
- Gerdesmeier, D., Reimers, H.-E., & Roffia, B. (2010). Asset Price Misalignments and the Role of Money and Credit*. International Finance, 13(3), 377–407. https://doi.org/10.1111/j.1468-2362.2010.01272.x
- Gilchrist, S., Himmelberg, C. P., & Huberman, G. (2005). Do stock price bubbles influence corporate investment? Journal of Monetary Economics, 52(4 SPEC. ISS.), 805–827. https://doi.org/10.1016/j.jmoneco.2005.03.003
- Goodhart, C., & Hofmann, B. (2000). Do Asset Prices Help to Predict Consumer Price Inflation? The Manchester School, 68(s1), 122–140. https://doi.org/10.1111/1467-9957.68.s1.7
- Gordon, R. J., & Gordon, R. J. (1998). Foundations of the Goldilocks economy: supply shocks and the TimeVarying NAIRU. BROOKING PAPERS ON ECONOMIC ACTIVITY, 297--346. Retrieved from http://citeseerx.ist.psu.edu/viewdoc/summary?doi=10.1.1.604.9819
- Henkel, S. J., Martin, J. S., & Nardari, F. (2011). Time-varying short-horizon predictability☆. Journal of Financial Economics, 99(3), 560–580. https://doi.org/10.1016/j.jfineco.2010.09.008
- Komarek, L., & Kubicová, I. (2011). The Classification and Identification of Asset Price Bubbles. Czech Journal of Economics and Finance (Finance a Uver), 61(1), 34–48. Retrieved from https://ideas.repec.org/a/fau/fauart/v61y2011i1p34-48.html
- Lili, L., & Chengmei, D. (2013). Research of the Influence of Macro-Economic Factors on the Price of Gold. Procedia Computer Science, 17, 737–743. https://doi.org/10.1016/j.procs.2013.05.095
- Loayza, N. V., & Rancière, R. (2006). Financial Development, Financial Fragility, and Growth. Journal of Money, Credit, and Banking, 38(4), 1051–1076. https://doi.org/10.2307/3838993
- Noussair, C. N. (2017). The Discovery of Bubbles and Crashes in Experimental Asset Markets and the Contribution of Vernon Smith to Experimental Finance. Southern Economic Journal, 83(3), 644–648. https://doi.org/10.1002/soej.12195
- Pierdzioch, C., Risse, M., & Rohloff, S. (2014). The international business cycle and gold-price fluctuations. Quarterly Review of Economics and Finance, 54(2), 292–305. https://doi.org/10.1016/j.qref.2014.01.002
- Queiroz, T. B. de, Medeiros, O. R. de, & Neto, J. C. da C. O. (2011). Evidence of speculative bubbles on the BOVESPA: an application of the Kalman filter. Brazilian Review of Finance, 9(2), 257–275. Retrieved from https://ideas.repec.org/a/brf/journl/v9y2011i2p257-275.html
- Razen, M., Huber, J., & Kirchler, M. (2017). Cash inflow and trading horizon in asset markets. European Economic Review, 92, 359–384. https://doi.org/10.1016/j.euroecorev.2016.11.010
- Sarno, L., & Taylora, M. P. (1999). Moral hazard, asset price bubbles, capital flows, and the East Asian crisis: Journal of International Money and Finance, 18(4), 637–657. https://doi.org/10.1016/S0261-5606(99)00018-2l
Copyright information
This work is licensed under a Creative Commons Attribution-NonCommercial-NoDerivatives 4.0 International License.
About this article
Publication Date
01 May 2018
Article Doi
eBook ISBN
978-1-80296-039-6
Publisher
Future Academy
Volume
40
Print ISBN (optional)
-
Edition Number
1st Edition
Pages
1-1231
Subjects
Business, innovation, sustainability, environment, green business, environmental issues
Cite this article as:
Batool, Z. (2018). The Time Horizon Of Asset Price Movements To Predict Financial Crises. In M. Imran Qureshi (Ed.), Technology & Society: A Multidisciplinary Pathway for Sustainable Development, vol 40. European Proceedings of Social and Behavioural Sciences (pp. 895-909). Future Academy. https://doi.org/10.15405/epsbs.2018.05.73