Abstract
One of the promising direction of the development of interface cockpits of modern aircraft is the voice control of on-board equipment. The main element of the voice control is the automatic recognition of voice commands, the effectiveness of which is determined by many factors, first of all the different types of acoustic noises. Noise is one of the biggest problems in speech recognition area. It does not only create problems when finding a speech signal in condition of noise or another acoustic interference, but also can change the speaker`s voice manner. This article focuses on the results of the way acoustic noise influences speech characteristics. The authors have come up with the two methods to decrease this influence. Firstly, recognition patterns can be formed using words, which have been influenced in this way. Secondly, the special reverse, or feed-back, channel can be added in the speech recognition system, which enables a speaker to hear himself as well as usually. All results were obtained with the implementation of the speech recognition algorithm, based on parameterization of the speech signal, on method of forming a pattern and the method of recognizing the individual words by comparison with standards of words, on linear regression and application of an additional microphone. An additional microphone is positioned at a distance of 0.3–0.7 m from the speaker in order to fix predominantly acoustic noises in the cockpit.
Keywords: Acoustic noise influencespeech recognitionnoise resistant algorithmspeech characteristics
Introduction
Resistance to the acoustic noise is one of the most important requirements for the voice control system of on-board equipment in the cockpit, where the noise maximum level can amount 80–90 dB.
The main aim of this study is to carry out the experimental research of the way acoustic noise influences a speaker`s voice characteristics, which decreases the efficiency of the recognition algorithm developed (Korsun, 2017). The percentage of correctly recognized words for a small dictionary system has been chosen as a criterion.
Before the recognition all speech signals should be transformed into vectors of parameters, which are obtained by taking a Fourier transform of a short time window of speech (frame) and calculating the spectrum in one out of the three standard ways (Rabiner, 1993): spectrum, mel-spectrum, mel-cepstrum (Randall, 2016, Wisniewski, 2008). Only after such a transformation they can be further compared with the patterns from the dictionary. The pattern in this case is the average parametric vector of the series of the word implementations of one or more speakers.
Firstly, in case of silence our algorithm recognizes correctly 99% of words (Rabiner,1993). This level has been chosen as a required one. It is the highest level in known algorithms (Chia, 2012, Gong, 1995, Pal, 2015, Gonzalez Jose, 2016).
The next stage of the experimental research presupposes the program "mix" of the words records and acoustic noise record. This technique ensures a strict compliance with the assumptions of the noise additivity and excludes the noise influence on the speaker`s speech. The initial algorithm shows, as expected, the increased number of errors caused by the noise. The further application of the modified algorithm (Korsun, 2017), based on the additional microphone and multiple linear regression, once again enabled to correctly recognize words with the required 99% probability. However, the application of this algorithm in terms of real noise brought no positive results. That proves our hypothesis that the noise leads to changes in speech characteristics.
Problem Statement
The paper is aimed at carrying out the experimental research of the way acoustic noise influences a speaker`s voice characteristics.
Research Questions
The study had the following objectives: first, to examine the way acoustic noise influences a speaker`s voice characteristics. Secondly, to develop the method of decreasing such influence.
Purpose of the Study
The main goal of the present study is to find out the way we could reduce the influence of noise on the speech characteristics, which decreases the efficiency of the recognition algorithm developed.
Research Methods
The percentage of correctly recognized words for a small dictionary system has been chosen as a criterion in both methods. Methods are listed below.
The noise in the headphones
The experiment with the noise in the headphones (see Fig.
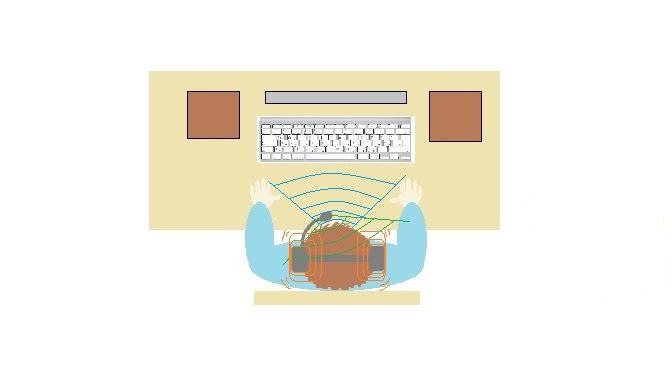
To recognize all the words from this experiment we used the last version of the algorithm with the multiple linear regression.
The pattern "N-v 0 dB" was formed on the words without noise in the headphones from the first stage using only the speaker`s N-v records. Also were formed serial of patterns for each of the speakers and for each of the two amplitudes of acoustic interference "80 dB" and "90 dB".
A reverse channel
In addition to the use of the right patterns to reduce this influence we recommend the use of a special reverse channel (Gong, 1995), or an acoustic feed-back. This reverse channel enables to play the signal through the headphones at real time from the headphones’ microphone. In this experiment, even under the influence of a strong acoustic noise produced by a stereo system, which was being played in the room while recording, the speech characteristics underwent minimal changes. This effect is achieved due to the fact that the speaker hears his own speech and does not distort his voice, as in the experiment before. The scheme of the experiment is presented in figure
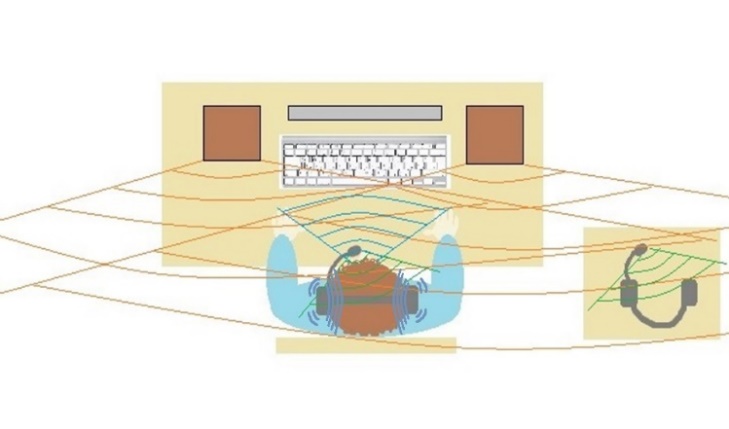
Findings
The results of the special experiments are described in 2 parts.
Experiment with the noise in the headphones.
Figure
It should be noted that patterns formed in the in conditions of the headphone noise with levels "80 dB" and "90 dB" ("N-v 80 dB" and "N-v 90 dB") did show some increase of errors but the maximum error level didn’t exceed 1%.
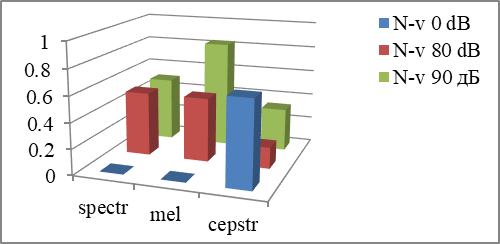
However, for words with the noise of 80 dB in the headphones, pattern "N-v 0 dB" ensures the recognition with the increase in the total number of errors for all 4 speakers to 4% in case of the spectrum method of parameterization, to 3.2% for the mel-spectrum method and to 1% for the mel-cepstrum method (Fig.
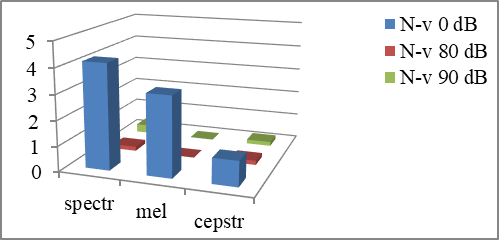
Figure
For the words with the noise of 90 dB the total number of errors for the pattern "N-v 0 dB" is respectively 4.2% for the spectrum, 3.7% for the mel-spectrum, 0.5% for the mel-cepstrum (Fig.
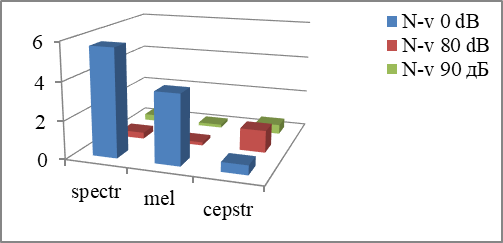
Pattern "N-v 80 dB" for the records with the noise of 90 dB shows the results– 0.4% for the spectrum, 0.2% for the mel-spectrum and 1.2% for the mel-cepstrum. The pattern "N-v 90 dB" in comparison with the "N-v 80 dB" reduced errors by 0.7% for the mel-cepstrum parameterization method, while the level of errors for other methods were not changed.
Figures
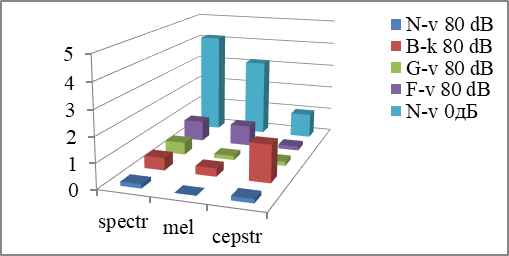
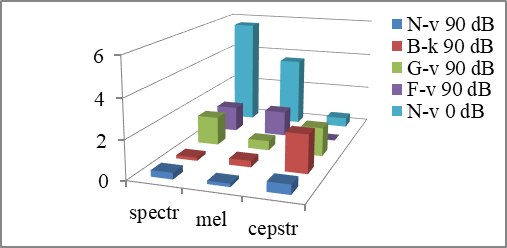
Thus, the right choice of a suitable pattern enables us to recognize words with high efficiency, even despite the influence of noise on the speech parameters of the speaker. The patterns with noise shows a good level of recognition not only in the recognition of words with noise, but also in the recognition without noise, for which there are no changes to voice characteristics of the speakers under the loud acoustic noise.
The use of a reverse channel.
Table
It is recommended to use the pattern "N-v 0 dB" for the noise "Boeing 80 dB", as it gives us the total number of errors equal to 0.5 % and 0.7% for spectrum (sp) and mel-spectrum (mel) types while using both microphones.
The efficiency of the reverse channel application is illustrated in figure
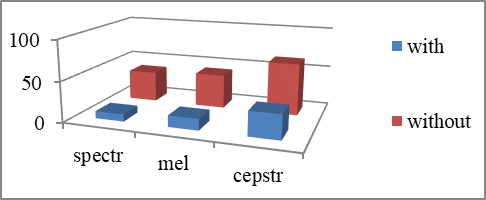
Thus, the use of the reverse channel reduces the number of errors for records with real noises. In addition to the reverse channel you must still take into account the correct choice of the patterns, the effectiveness of which depends on each specific casePlease replace this text with context of your paper.
Conclusion
The results of the special experiment (noise of 80-90 dB is supplied only to speaker`s headphones) showed that the speech characteristics of the speaker are changed significantly. However, the percentage of recognition errors using patterns, formed from the records without noise, is 3.2...4.2% for the spectrum and mel-spectrum variants of parameterization. For patterns, formed using words with noise of 80-90 dB, the percentage of recognition errors is reduced and is 0...0,4% for the spectrum and mel-spectrum variants of parameterization.
To reduce the influence of noise on the speech characteristics the reverse channel is proposed. The signal from the main microphone is led to the headphones, so that the speaker could hear himself in the conditions of strong noises as well as in normal conditions.
The experiment on speech recognition in real noise conditions and using the reverse channel were conducted for 4 speakers and absolute noise level of 80 dB for noise types "Boeing" and "Speech". The results of the experiment showed that using the patterns, generated on the data without noise, we can obtain the minimum error levels for the spectrum variant of the parameterization and the range 0.5...2.5%, that 1.5...3 times less than the same case without the use of the reverse channel.
Acknowledgments
This research is supported by Russian Fund for Basic Researches (RFBR), project 15-08-06946.
References
- Chia Ai O., Hariharan M., Yaacob S., Sin Chee L. (2012). Classification of speech dysfluencies with MFCC and LPCC features. Expert Systems with Applications, 39, 2157–2165.
- Gong, Y. (1995). Speech recognition in noisy environments: a survey. Speech Communication, 16(3), 261-291.
- Gonzalez Jose A., Gomez Angel M., Peinado, Antonio M. (2016). Spectral Reconstruction and Noise Model Estimation Based on a Masking Model for Noise Robust Speech Recognition. Circuits Systems And Signal Processing, 36(9), 3731-3760.
- Korsun O. N., Gabdrakhmanov A. Sh. (2017). Speech recognition based on relations with аll the patterns in the dictionary. Vestnik komp`iuternykh i informatsionnykh tekhnologii, 1, 10-15.
- Pal M., Saha G. (2015). On robustness of speech based biometric systems against voice conversion attack. Applied Soft Computing, 30, 214-228.
- Rabiner L., Juang B.H. (1993). Fundamentals of Speech Recognition. Prentice-Hall International, Inc., Englewood Cliffs, New Jersey, 507.
- Randall, Robert B. (2016). A history of cepstrum analysis and its application to mechanical problems. Mechanical Systems and Signal Processing, 97, 3-19.
- Wisniewski M., Kuniszyk-J´o´zkowiak W., Smolka E., Suszynski W. (2008). Automatic detection of disorders in a continuous speech with the hidden Markov models approach. Proceedings of Computer Recognition Systems 2, 45, 445–453.
Copyright information
This work is licensed under a Creative Commons Attribution-NonCommercial-NoDerivatives 4.0 International License.
About this article
Publication Date
13 December 2017
Article Doi
eBook ISBN
978-1-80296-032-7
Publisher
Future Academy
Volume
33
Print ISBN (optional)
-
Edition Number
1st Edition
Pages
1-481
Subjects
Cognitive theory, educational equipment, educational technology, computer-aided learning (CAL), psycholinguistics
Cite this article as:
Gabdrakhmanov, A. S., & Korsun, O. N. (2017). The Experimental Research Of The Way Acoustic Noise Influences Speech Characteristics. In S. B. Malykh, & E. V. Nikulchev (Eds.), Psychology and Education - ICPE 2017, vol 33. European Proceedings of Social and Behavioural Sciences (pp. 113-120). Future Academy. https://doi.org/10.15405/epsbs.2017.12.12