Abstract
Strategic decision-making processes necessitate a significant amount of experience and the consideration of a wide range of factors associated with the decision problem. Determination of applicant credit score and loan decisions are such decisions that subjective judgement of loan officers can produce poor results with substantial losses. This study is triggered as a result of an industrial consultancy project with a partner providing technological solutions for financial services. Anticipated requirement for an e-payment system that connects financial lenders, credit applicants and retailers digitally through a system is the main motivation of the study. Another focus of the study is on providing immediate loan access to retail customers which has necessitated automated processes and a decision-support system to assist the analysis of loan applications. The proposed system automatically evaluates a credit application as soon as the relevant information is inputted to the system at the end of the shopping process. The decision-making process should be supported with appropriate systems performing computerized credit assessment processes for quick and accurate decisions. The main complementary tools for the proposed system include a fraud detection module and credit risk assessment module which runs credit scoring algorithms. The proposed system will make loan or credit line decisions faster by eliminating paper work, easier by eliminating bureaucracy, and more accurate by newly integrated algorithms and approaches.
Keywords: Credit scoringfraud managementconsumer loansretailers’ credit assessment
Introduction
Along with the worldwide picture depicting increase in loan amounts the volume of consumer loans and debt of credit cards has been rising significantly in Turkey as well for the last few years. The reason behind the continuously increasing demand for consumer loans is the substantial rise in consumption levels and decrease in savings (Akcayir, 2016). Banks and lender institutions are searching for new strategies to answer this demand more profitably and expand their client base. In the way of accomplishment efficiency in decision-making processes is critical in terms of matching the right client with right amount of credit on time while lowering the risk of credit portfolio and enhancing the loan volume at the same time. It has been a topic of debate as to whether to grant credit to clients since the early 1960s. Credit scoring, which offers the benefits of enhancing cash flow, eliminating risk and increasing performance in decision-making, has become an important issue in the credit industry. As the attention allocated to credit scoring has grown, credit scoring models using various techniques and approaches have emerged (Lee et al., 2002).
Leonard (1996) stated that in the credit field, the first step is to identify the strategic objectives which include decreasing losses from loans and delinquency, increasing client base, and increasing market share and profitability. The accomplishment of these objectives can take place through the implementation of credit scoring mechanisms to a credit portfolio. Credit scores have been in use for a long time in consumer credit markets and low-cost lines of credits have impacted the availability of consumer loans positively (Leonard, 1992). Credit scoring models vary according to type of credit, size and type of information (application data, financial data etc.). From the lenders’ point of view, it is crucial to find the most appropriate model that enhances their lending by yielding a profitable credit management strategy (Berger & Frame, 2006). In the sense of consumer credit, the dynamic nature of consumers slows down the lending process because of difficulties in scoring. The usage of historical data in most traditional scoring models does not work well, especially when there is a new client or there is not enough financial data. Nevertheless, the integration of social media data into scoring process has been gaining popularity as it provides to support clients that have limited financial history (Ntwiga & Weke, 2016).
People encounter great financial constraints when financial institutions have difficulties in evaluating their risk because of insufficient information regarding the financial situation of the borrower. The effect of information sharing and third parties such as credit bureaus in order to enhance credit information has been thoroughly discussed by researchers (Arraiz, Bruhn & Stucchi, 2015). Peria, Soledad & Singh (2014) discussed the role of information sharing in finance and a considerable obstacle in accessing financing was found to be the asymmetric information. Information asymmetry is the situation in which the borrower has better information than the lender regarding his/her financial status and ability to repay. Credit bureaus or publicly regulated credit registries are institutions that lower information asymmetry by means of sharing information about clients of different lenders. The presented business model intends to overcome problems related to customers’ credit approval processes and satisfy the need for an automated credit decision and approval system for micro-lenders. This study is based on the need of an automated system for decision support in credit lending processes and its major aim is to provide the interconnection of parties during the shopping processes of customers electronically. Quickening the information and document exchange between parties in a digital environment has the potential of enabling lenders to assess credit applications immediately and provide loans for customers online or when they are at the store. Although in Turkey banks offer customers short term loans with repayment periods of up to 48 months, the process necessitates being a current customer of that bank and credit is transferred directly to borrowers’ accounts. Thus there is a need for a business model that integrates money transfer, information and document exchange processes of lenders, customers and retailers. The systems’ main modules are fraud detection algorithms and credit scoring mechanisms which consider a wide range of factors including credit bureau data, psychometric data and social media integration. Usage of psychometric data supports the fraud detection module when fraudulent behaviour is detected or the initial risk assessment score demonstrates high risk levels. Self-reported data of customer is used in the evaluation process and this application data is primarily based on subjectivity and bias. There are many automated credit evaluation mechanisms developed for credit evaluation purposes that use different methodologies and various types and sources of data. This proposed system focuses specifically on retailers’ lending processes by combining various types of data to perform scoring and to define credibility. In order to increase the accuracy and produce realistic score reports for customers, it is essential to hold up the structure of the data by using various data sources.
In this context, the first step of this study is a comprehensive literature review of credit scoring and fraud management regarding the loan application processes. The study goes on with a description of our case study, which focuses on a company dealing with Fintech business and financial services. This company is specifically well suited for analysing problems that lenders encounter and evaluating important tasks that need special attention in the marketplace. The need for an adoption of an integrated mechanism that synchronizes credit lending processes of lenders, retailers and borrowers for both e-commerce and in-store credit decisions has stimulated the development of a new business model for consumer lending.
Literature Review and Theoretical Framework
Credit Scoring
Credit scoring is defined as the process of a model that evaluates if someone is creditworthy or not (Hand & Jacka (1998) as cited in Abdou & Pointon, 2011). Credit scoring is a discipline which was developed and implemented since the beginning of 1960s. Since the decision-making in a wide range of businesses and industries is supported by credit assessment tools and automated credit decision processes (Anderson, 2007), credit scoring has been an essential tool for various institutions. For this reason, various scoring techniques with different classification and prediction methods have been developed (Abdou & Pointon, 2011). Greenspan (2002) (as cited in Anderson, 2007) stated that as the initial purpose of credit scoring was to evaluate the credit related risks. Recently defining credit limits for borrowers, facilitating fraud detection, delinquency intervention, loss mitigation and other loan servicing activities have been increasingly supported by credit delivery systems The current economic landscape is highly dependent on credit and borrowing money is considered as a right for people (Anderson, 2007). There have been dramatic increases in lending and loan applications worldwide which contributed to development of statistics and operations research based credit scoring. Depending on the fact that individuals’ ability and willingness to pay back change, offering credit to borrowers is a highly risky business for credit lenders. Automation, which has shifted to the decision-making field, significantly affected the credit decision processes (Anderson, 2007). Automated credit scoring mechanisms offer lenders the benefits of expanding their loans in an effective manner and providing risk assessment properly (Thomas et al. (2002) as cited in Abdou & Pointon, 2011). Entire banks and most financial lenders manage their loan processing activities by means of robust software tools provided by professional consultants (Crook, Edelman & Thomas, 2007). Judgemental methods of the past for credit giving and consumer credit decisions have been replaced by credit scoring. Judgemental decisions were based on the subjective evaluation of credit officers and assessments were based on characteristics of the credit, historical credit behaviour of the applicant, income data, occupation and residential status. Bias and inconsistencies among different evaluators, lack of education and training, cost of credit reports and mistakes all influenced the seeking of new ways for credit decisions. Hence, quantitative methods based on numerical scoring of a number of a given applicant’s characteristics were developed. However, the use of these methods was not observed until the early 1960s which represented the development period of relevant computer technologies (Capon, 1982).
Laha (2007) defined credit scoring as a way of anticipating potential risk belonging to a credit portfolio which can be split into two as application scoring and behavioural scoring. Demographic data of borrowers is used for computing the application score whereas historical payment behaviour of the applicant is used for the behavioural score. Capon (1982) stated that zip code, bank reference, type of housing, occupation type, time at the present address, time with employer and finance companies’ references were among indicator that retailers had been using for computing a final score in those times. It has been a topic of investigation as to whether to solely use the financial history of consumers for credit scoring is sufficient or not. Popular financial credit scores such as Fair Isaac Corporation (FICO) credit score mostly depends on debt levels of consumers and the length of and payment behaviour (regular or irregular payments) in their credit histories. However, significant changes in the variety of data sources encouraged companies to look for different types of data to integrate into their decision-making processes regarding credit assessment. For instance, recently there is an increasing trend to use network-based data and network-based indicators which have great potential to decrease financial risks of lenders (Wei, Bulte & Dellacoras, 2015).
Thomas (2000) pointed out that in terms of use of techniques, statistical methods including parametric and non-parametric techniques and artificial intelligence approaches have been widely used in credit decision models as cited in Lee et al. (2002). Discriminant analysis and logistic regression were reported to be the most common methods used in scoring models by Lee et al. (2002). Beyond these classical statistical approaches neural networks by performing better in the case of existence of complex non-linear relationships among variables can help with better decision making. In addition to neural networks, expert systems, fuzzy systems and genetic algorithms that depend on artificial intelligence can be used in scoring models as well (Šušteršič, Mramor and Zupan, 2009). Nosratabadi, Nadali and Pourdarab (2012) proposed a credit scoring model based on a fuzzy expert system approach. They classified credit borrowers by association rules and rules extracted by data mining which were supported by experts’ knowledge. Iç & Yurdakul (2010) developed a decision support system for immediate credit scoring for determining credibility of manufacturing firms in Turkey. The model considered financial ratios and used a fuzzy TOPSIS approach. In order to reduce time in the credit assessment process a two-stage approach was used to indicate whether a further analysis is necessary for an applicant or not. Thus, inappropriate applicants could be rejected quickly. Among developed classifiers, one common method for classifying “good” and “bad” credits is logistic regression which enables the comparison of the logit value with a cut off. In addition to logistic regression, “support vector machines” is another method which has widely been found to be precise in literature (Crook, Edelman & Thomas, 2007).
In the case of micro-lender financing Dellien & Schereiner (2005) stated that scoring is a tool that is mostly used by banks instead of micro-lenders. They discussed the adaption of scoring to micro-lenders which offers accurate forecasts and quantitative risk assessment. In this sense, banks’ high-tech automated scoring should be adapted to micro-lenders which traditionally use personal characteristics and financial data for credit risk assessment. Successful scoring is associated with the quality of data and automated tools which are usually supported with credit bureau data that captures applicants’ details of income and financial history. Credit bureau data supported by other application data such as occupation, education, age etc. offers the advantage of more powerful predictions regarding the future payment performance of borrowers. Credit bureaus supply profile for the payment performance of applicants through data gathered from various sources (Dellien & Schereiner, 2005). Abdou & Pointon (2011) reviewed the literature on credit scoring applications for decision-making and discovered that the methodologies were highly varied based on different areas and circumstances. Thus, there is no methodology fitting all circumstances and key determinants of a specific scoring model is dependent on the nature of the case. According to Anderson (2007) scoring models can be classified under four categories comprised of models based on pure judgement, expert systems, hybrid models and statistical models. Expert systems are suitable for conditions where there is not enough data but experience is enough to build a set of rules. In contrast, statistical models work well with a large amount of structured data and they are highly dependent on data availability. If statistical models and expert systems are used together, these hybrid models can combine data from different models into one model. Moreover, Anderson (2007) pointed out that for small business and retailer credit, statistical models are more appropriate because of the high transaction volume. J.P. Morgan (no date) summarizes some key data sources used in scoring applications as follows; socio-demographic data, behavioural data, credit bureau data, psychometric data and big data. There has been an increasing trend in the use of psychometric data which is captured by means of questionnaires to depict people’s personality, capabilities and mental outlook. Together with psychometrics, big data compromising a wide range of external sources like social media and phone companies can be integrated to credit assessment mechanisms. Rusli (2013) indicated that education, employment history, contacts, followers, quality of contacts obtained from social networks have been increasingly used for credit scoring as cited in Wei, Bulte & Dellacoras (2015).
Klinger et al. (2013) investigated the benefits of an innovative psychometric tool for identifying the credit risks of a particular bank’s clients. Psychometrics based credit scores were evaluated in terms of their ability to streamline the credit processes and it was discovered that scorecards involving psychometrics-based data were helpful in improving performance of process. Impact Matters (2015) stated that Entrepreneurial Finance Lab (EFL) tests, which rely on psychometrics, can be used as a secondary screening tool in credit risk assessments especially when the data sources are scarce. Credit bureaus in various countries are not always well-developed and it takes time to gather profound information on potential clients. However, psychometric tools such as EFL, which is a low cost credit evaluation mechanism based on psychometrics, reduces the information asymmetry encountered by creditors. Arráiz, Bruhn & Stucchi (2015) investigated the use of EFL’s psychometric testto filter high credit risk and enhance the availability of credit for small business owners in Peru. Findings of researchers revealed that EFL tool had remarkable contribution in decreasing the risk of loan portfolios. Moreover, borrowers previously rejected under traditional scoring could also be revaluated through the instrument of psychometrics and potentially be offered previously denied loans. Since psychometrics provides additional information, this prevents loss stemmed from misclassification. Thus, applicants under rejection initially based on financial indicators and classical scoring models can be offered a loan after psychometrics check.
Fraud Management
Fraud is not a new concept unique to the age in which we are living. However, globalization, widespread usage of technology and the speed of technological developments have reinforced the need to control and monitor transactions with the help of data analysis algorithms and programmes. Developments in IT have changed the ways in which people deal with fraud and the way fraudsters act. Thus, it is important to divert significant attention to fraud management activities for organizations and financial institutions (Anderson, 2007). Technology is the most influential factor in preventing and detecting fraudulent behaviour. Organizations using proactive data monitoring/analysis tools had almost 59.7% elimination in loss associated with fraud (Banarescu, 2015). Credit fraud, which is more likely to be executed by professional fraudsters, requires the attentive monitoring of identities and transactions. Suspicion scores, rules or anomalies should be determined in enormous data sets that are generated from transactions between external parties. Fraud detection which automates controlling and monitoring activities aids in dealing with fraud in a cost-effective way. Thus, fraud management is a strategic tool for organizations to operate in a competitive environment (Phua et al., 2010). In order to get a macro-view of fraud, strategic analysis is required. Analytical tools based on statistics and data mining contribute to this analysis by visualising trends and patterns (Bresfelean et al. (2008) as cited in Banarescu, 2015). Text analytics and geospatial analysis are among analytical tools that identify and explore patterns in fraudulent behaviour. Nevertheless, the adoption of an antifraud analytical tool is a big obstacle that should be overcome, since it necessitates retrieving different types of data from a wide range of sources (Banarescu, 2015). Financial fraud has remarkable negative effects on organizations, markets and economies. Existing resources dedicated for financial fraud detection (FFD) are not always adequate in capturing entire fraud occurrences on time. Regarding the automating of FFD, although the usage of financial statistics is the most prevalent way, recently vocal and linguistic predictors can be used as well (Throckmorton et al., 2015).
E-commerce systems which have proliferated with technology’s diffusiveness, advancements in IT and internet provide companies to trade their products and services in an effective and productive manner. Nevertheless, the prevalence and characteristics of e-commerce systems cause these systems’ to be vulnerable to large scale systematic fraud. Hence, in addition to Fraud Prevention Systems (FPSs) integrating Fraud Detection Systems (FDSs) is considerably important. FDSs can be subdivided into as “Anomaly based fraud detection”, “Misuse based fraud detection” and “Hybrid of anomaly and misuse detection” as stated by (Abdallah, Maarof & Zainal, 2016). Fraud detection techniques can be classified as supervised or unsupervised techniques. Supervised techniques use a set of known fraudulent cases for identifying scores for new data whereas unsupervised ones do not deal with previously identified sets of observations (Bolton & Hand, 2002). Intelligent systems comprising neural networks, fuzzy systems, GA, data mining, rule-based, supervised/unsupervised methods and statistical techniques are among techniques used in FDSs (Abdallah, Maarof & Zainal, 2016). Because of the limitations of expert systems or systems’ dependence on pre-defined rules, FDSs based on data mining and integrating various statistical, artificial intelligence and machine learning techniques are more preferable. Although rule based systems have limited capabilities in terms of detecting unusual and multifarious cases, they easily identify familiar fraudulent behaviour in a short time. Thus, it has been a topic for researchers to investigate usage of various techniques in a combined manner. West & Bhattacharya (2016) also investigated literature associated with fraud detection. Hybrid usage of text mining, support vector machines and ANNs, utilization of self-organizing maps which provide visualisation of fraud, game theory, decision trees and hybrid usage of text mining and Bayes networks were discovered in the fraud detection literature by researchers. Some other studies reveal a similar outcome by drawing attention to usage of neural networks, artificial immune systems, decision trees, GA and Bayes networks (Arif & Dar, 2015; Lata, Koushika & Hasan, 2015). A system integrating GA and Scatter search (SS) algorithm was developed by Duman & Ozcelik (2011) in order to overcome the problem of misclassification of some commercial fraud detection tools used in a bank in Turkey. After the implementation, fraud detection performance increased by approximately 200%. Wheeler & Aitken (2000) studied case-based reasoning and its combined usage with various algorithms. Previous research discussed the disadvantages of defining rules demonstrating fraudulent behaviour and detecting fraud accordingly. The integration of case-based reasoning with different algorithms demonstrated high performance. Richhariya & Singh (2012) and Major & Riedinger (2002) focused on expert systems’ implementation for fraud management. Major & Riedinger (2002) also argued that statistical evaluation should be integrated with expert knowledge. The method they developed used machine learning to determine new rules and improve detection performance.
Research Method
This study is a qualitative research, and a case study strategy which is appropriate for catching the experiences and knowledge of practitioners (Benbasat, Goldstein & Mead, 1987) has guided the research processes. Benbasat, Goldstein & Mead (1987) stated that case study research is appropriate as an information systems research strategy due to the fact that it contributes to anticipate the characteristics of processes, to capture rapid changes in the information systems field and explore the state of the art. The use of the case study approach has aided in understanding the nature of the industry, the role of the laws and regulations dominating the retail and finance sectors. Theoretical fraud management and credit scoring algorithms were analyzed in order to evaluate model components based on the characteristics of loan applications through literature review. After capturing prior knowledge through investigation into different systems used by banks or financial lenders by means of online secondary sources, open ended interviews with practitioners were performed. These interviews then formed the basis for the further investigation of data resources and guided the development of the conceptual model. In this study, the implementation of an innovative business model for the purpose of improving lending practices of retailers is investigated. For this purpose, credit scoring and risk management systems which can be integrated to other important systems of all parties will be developed. Information streaming from different channels and transactions are represented through graphical user interfaces of the system. At the center of the business model, there is a decision support system for credit risk assessment that evaluates credibility of clients by running a scoring algorithm. As this study is motivated from an industrial consultancy project, our partner which provides financial technology to its customers required a new business model for acceleration in decision-making in the credit lending processes of lenders. Based on the fact that consumer lending has experienced a transition from traditional interview based processes to data-driven models for credit risk assessment, the size and the magnitude of channels influenced by the implementation of credit scoring should be considered (Einav, Jenkins & Levin, 2013).
Company Background
TRA Bilişim is a professional service company operating in Turkey’s finance sector since 1990. The company has lines of services composed of providing financial needs of enterprises and developing new technology/innovation. The company develops end-to-end payment solutions for banks, retailers, education and livestock etc. and implements technology for advancement in financial activities by supplying financial backing from its own micro-finance institution.
Case Description/Problem
The company wants to extend its business to a new marketplace by offering a new end-to-end payment system that involves decision-making regarding the credit lending decisions in retailers. Customers’ demanded credit amounts should be supplied immediately at the end of shopping which requires finding the appropriate financier on time. From the retailer side, customer base should be improved by offering short term loans to customers that are unable to pay cash or incapable of providing documents necessary for the credit application processes of banks. Thus, retailers’ decision should be supported by a complementary set of tools that automate processes, and data about creditworthiness should be retrieved from various sources and integrated. Thus the company’s main focus is to integrate financial service providers, retailers and customers into one comprehensive system to provide small loans for customers effectively at the time of shopping in-store or online. This goal is concerned with determination of how a lender should allocate a loan to a particular customer which necessitates a new business model including new technological implementations and processes. A graphical user interface is needed for in-store transactions of retailers to input appropriate data to calculate customers’ credibility. The credit assessment process of the system should not take more than 5 minutes. Another important aspect that should be addressed is the prevention and detection of fraud through a fraud management approach.
Proposed Model and Solution
The main goal of the model is to increase turnaround time for credit delivery and increase client base by providing short term loans to clients immediately during their shopping activities. Thus, clients should be screened quickly and decisions regarding the credit risk should be made. A good solution should utilize a fraud management tool as well in order to lower the risk of the loan portfolio. Profitability of parties involved is associated with defining risks better, which is highly correlated with assigning scores to loan applicants. The following steps were considered in producing a model for this decision support system: Investigation of credit scoring and fraud management models studied in the literature, investigation of substitute services offered, determination of appropriate data and data sources for the system and construction of the conceptual model. Among various inputs for credit scoring mentioned in literature, appropriate ones for this case were found to be application data, behavioural data, credit reference bureau data (Findeks score), psychometric data and social media data. Cooperation between The Banks Association of Turkey, Turkish Union of Chambers and Credit Reference Bureau has developed an initiative for securing payments and prevent fraud. Hence, Findeks score which assesses credibility of individuals/organizations has been developed and served via a digital platform. This score largely depends on behavioural data demonstrating past payment behaviour and financial performance (http://www.cglawoffice.net). However, in order to make the application process more simple and efficient, a minimum number of documents should be demanded from customers. As a result of interviews with practitioners, application data was determined to be the national identity number and mobile phone number. The system is integrated to MERNIS (Central Registration Administration System) and credit registration bureau for getting supportive data. Psychometrics is used as a secondary screening mechanism for assessing customers that demonstrate high levels of risk as a result of initial assessment. In order to improve decision-making, social media analytics and text mining are integrated to the system to provide supportive data and real-time scoring. Due to the fact that this is a new system, historical data representing previous payment behaviour of clients is scarce, which makes the credit bureau checking process more noteworthy. The system both integrates statistical and expert scoring models for providing a shift from expert score to statistical score after enough historical information is gathered. The model has the advantage of pooled consortium data which supports retailer specific data.
Analyses and Findings
To assess state of the art technology theoretical models developed by researchers were investigated thorough literature review. Decision assessment models comprised of credit risk evaluation tools and fraud management literature were deeply analyzed to acquire relevant information. As industrial practitioners express the need for a national payment system that assists in loan access for customers of retailers, more generally this study is on a business model related to an innovative payment system. Thus, potential substitute systems and their real world applications were analyzed in terms of a set of features that they possess. The gap analysis’ results demonstrate the findings by contrasting the proposed model and developed models in the area of short term loans. Table
The proposed model involves a decision support system in general, and specifically, a method of automatically checking fraud and performing credit risk assessment for borrowers. After processing the applicants’ loan applications, a decision of approve/reject regarding the application is performed automatically. There is no need for applicants to fill a form. Submission of ID and mobile phone number for verification through MERNIS is the only required information for the application process. As the system is capable of searching associated data in publicly available databases, MERNIS (Central Registration Administration System) is used to determine whether the ID corresponds to the user. Entire processes following the input of loan application data are fully automated with no need for human intervention. The ID information is entered manually to the system before a verification code is sent to the borrower’s phone. After the information associated with requested loan amount is inputted to the system, the fraud detection module screens for fraud risk by communicating with different channels (credit card information, social security number, national fraud information sources etc.). The fraud detection tool uses a hybrid modelling approach comprised of analytical and rule-based techniques and allowing users to define rules in addition to rules of the system. This mechanism defines whether the application needs further assessment involving psychometrics. If high risk is determined after the execution of fraud detection algorithms, a secondary screening mechanism is taken into account which constitutes of a psychometrics test. The aim of this test is to eliminate loss from misclassification of borrowers and to provide supportive information before taking action. Decisions based on computerized analysis of tests, and if restriction is removed, credit scoring, are made. The system has the ability to communicate with the credit registration bureau. When the loan application data is approved after fraud checking, the credit registration bureau data and Findeks score are retrieved to compute the credit score. Assessment models based on expert and statistical models are executed to assign risk-based credit score. The system retrieves and transmits social media data automatically to integrate into decision assessment models. The system uses information obtained from various channels in determining the loan application result of the potential borrower, and generates a real-time response ideally in 3-4 minutes. After information about lenders’ loan options with different payment periods and interest rates are presented to the applicant, information regarding the preference is transmitted to the associated particular lender. All applications’ information and scanned IDs are stored in the system database. Figure
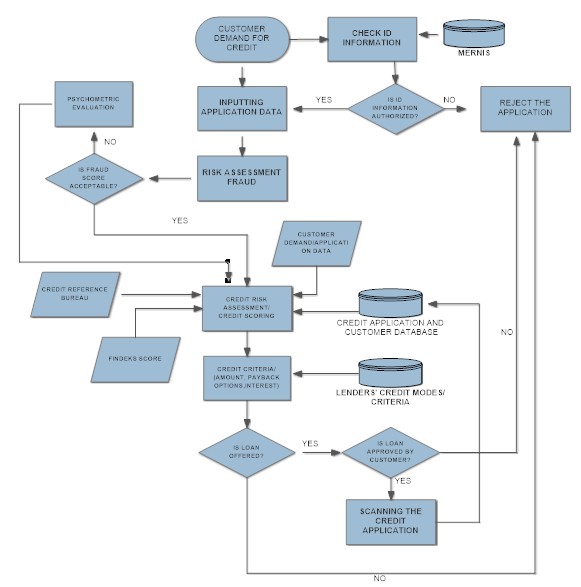
Conclusion and Discussions
This study is about the adoption of a business model associated with a payment system integrating data flow and processes among financial lenders, customers and retailers electronically. The core of the model depends on a decision support system that facilitates credit lending processes by means of credibility evaluation mechanism. Instant credit applications are also screened by a fraud detection tool and psychometric evaluation is performed when a high level of risk appears. In this way, there is a potential for increased profitability by targeting to offer loans to previously rejected customers. As credit scoring’s success is highly dependent on data availability data sources considered for the model are analyzed elaborately. From a literary point of view data taken account in credit evaluation processes can be classified as behavioural data, application data, social media data, credit bureau data and psychometrics. Applications use different combinations of this data so as to integrate into an assessment model. Social media data and psychometrics, which are recently new trends in the field, are considered in this model for the purpose of increasing prediction performance of models. Due to the fact that this is a new payment model that will be implemented, data regarding the past payment habits and performance of customers is not sufficient enough for model construction. However, social media data can offer the advantage of providing supportive information by means of analytical tools. As Findeks score of customers involves information regarding the financial behavioural data to some extent, retrieving this score to integrate is an innovative aspect of the model. The credit scoring module uses both statistical and expert models in order the get the benefits of both and complement each other until sufficient amount of statistical data is gathered as a result of transactions. This research presents a snapshot of a payment system depending on a decision support system. The magnitude of data, channels of data sources and flow of process required for credit scoring are identified for the conceptual model. The result of a gap analysis shows that there are relatively good indications on exactly how the proposed system differs from any other systems in the market. Potential value for the market is a rise in the volume of trade, increased availability of credit, elimination of loan portfolio risk of lenders and a uniform system among retailers.
References
- Abdallah, A., Maarof, M. A. & Zainal, A. (2016). Fraud detection system: A survey. Journal of Network and Computer Applications, 68, pp. 90-113.
- Abdou, H. A. & Pointon, J. (2011). Credit scoring, statistical techniques and evaluation criteria: A review of the literature. Intelligent Systems in Accounting, Finance and Management, 18(2-3), 59-88.
- Akcayir, O. (2016). The impact of consumer loans and credit cards on current account balance, Finans Politik ve Ekonomik Yorumlar, pp. 47-59.
- Anderson, R. (2007), The Credit Scoring Toolkit, Oxford University Press Inc., New York.
- Arif, M. & Dar, A.R. (2015). Survey on fraud detection techniques using data mining, International Journal of u- and e- Service, Service and Technology, 8(3), pp. 163-170.
- Arráiz, I., Bruhn, M. & Stucchi, R. (2015). Psychometric tests as a tool to improve screening and access to credit: Evidence from Peru. Retrieved from http://caed2015.sabanciuniv.edu/sites/caed2015.sabanciuniv.edu/files/Irani_Arr%C3%A1iz_Psychometric9thDraft.pdf
- Banarescu, A. (2015). Detecting and preventing fraud with data analytics, Procedia Economics and Finance, 32, pp.1827-1836.
- Benbasat, I., Goldstein, D.K. & Mead, M. (1987). The case research strategy in studies of information systems, MIS quarterly, pp. 369-386.
- Berger, A.N. & Frame, W.S. (2006). Small business credit scoring and credit availability, Journal of Small Business Management, 46.
- Bolton, RJ. & Hand, D.J. (2002). Statistical fraud detection: A review, Statistical Science, 17(3), pp. 235-249.
- Bresfelean, V.P., Bresfelean, M., Ghisoiu, N., & Comes, C.A. (2008). Determining students’ academic failure profile founded on data mining methods, In Information Technology Interfaces, IEEE, pp. 317-322.
- Capon, N. (1982). Credit Scoring Systems: A Critical Analysis, Journal of Marketing, 46(2).
- Crook, J. N., Edelman, D. B., & Thomas, L. C. (2007). Recent developments in consumer credit risk assessment, European Journal of Operational Research, 183(3), 1447-1465.
- Dellien, H. & Schereiner, M. (2005). Credit Scoring, Banks, and Microfinance: Balancing High-Tech with High-Touch. Retrieved from http://www.microfinance.com/English/Papers/Scoring_High_Tech_High_Touch.pdf
- Duman, E. & Ozcelik, M. H. (2011). Detecting credit card fraud by genetic algorithm and scatter search. Expert Systems with Applications, 38(10), pp. 13057-13063.
- Einav, L., Jenkins, M. & Levin, J. (2013). The impact of credit scoring on consumer lending, The RAND Journal of Economics, 44(2), pp. 249-274.
- Hand, D.J & Jacka, S.D. (1998). Statistics in Finance. Arnold: London.
- Iç, Y. T. & Yurdakul, M. (2010). Development of a quick credibility scoring decision support system using fuzzy TOPSIS, Expert Systems with Applications, 37(1), 567-574.
- Impact Matters (2015). Psychometrics: Putting Creditworthiness to the Test. Retrieved from
- https://www.fomin.org/Portals/0/impact%20evaluation/Brief_psychometrics.pdf
- J. P.Morgan (no date), Microfinance credit risk management tool guides credit scorin. Retrieved from
- http://www.accion.org/sites/default/files/credit-scoring.pdf
- Klinger, B., Khwaja, A. & LaMonte, J. (2013). Improving credit risk analysis with psychometrics in Peru. Inter-American Development Bank.
- Laha, A. (2007). Building contextual classifiers by integrating fuzzy rule based classification technique and k-nn method for credit scoring, Advanced Engineering Informatics, 21(3). 281-291.
- Lata, L.N., Koushika, I.A. and Hasan, S.S. (2015). A comprehensive survey of fraud detection techniques, International Journal of Applied Information Systems, 10(2).
- Lee, T. S., Chiu, C. C., Lu, C. J., & Chen, I. F. (2002). Credit scoring using the hybrid neural discriminant technique. Expert Systems with applications, 23(3), pp.245-254.
- Leonard, K. J. (1996). Information systems and benchmarking in the credit scoring industry. Benchmarking for Quality Management & Technology, 3(1), pp.38-44.
- Leonard, K. J. (1992). Credit-scoring models for the evaluation of small-business loan applications. IMA Journal of Management Mathematics, 4(1), pp.89-95.
- Major, J.A. & Riedinger, D.R. (2002). EFD: A Hybrid Knowledge/Statistical-Based System for the Detection of Fraud, International Journal of Intelligent Systems, 69(3), pp. 309-324.
- Peria, M, Soledad, M. & Singh, S. (2014). The Impact of Credit Information Sharing Reforms on Firm Financing. World Bank Policy Research Working Paper 7013
- Nosratabadi, H. E., Nadali, A., & Pourdarab, S. (2012). Credit assessment of bank customers by a fuzzy expert system based on rules extracted from association rules, International Journal of Machine Learning and Computing, 2(5), 662.
- Ntwiga, D. B. & Weke, P. (2016). Consumer lending using social media data, International Journal of Scientific Research and Innovative Technology 3.2 , pp. 1-8.
- Phua, C., Lee, V., Smith, K. & Gayler, R. (2010). A comprehensive survey of data mining-based fraud detection research. arXivpreprint arXiv:1009.6119.
- QR Code Check Sistem in Turkey. Retrieved from http://www.cglawoffice.net/tag/findeks/
- Richhariya, P. & Singh, P.K. (2012). A Survey on Financial Fraud Detection Methodologies. International Journal of Computer Applications 45(22), pp.15-2
- Šušteršič, M., Mramor, D. & Zupan, J. (2009). Consumer credit scoring models with limited data, Expert Systems with Applications, 36(3), pp. 4736-4744.
- Throckmorton, C. S., Mayew, W. J., Venkatachalam, M., & Collins, L. M. (2015). Financial fraud detection using vocal, linguistic and financial cues. Decision Support Systems, 74, 78-87.
- Wei, Y., Yildirim, P., Van den Bulte, C., & Dellarocas, C. (2015). Credit scoring with social network data, Marketing Science, 35(2), 234-258.
- West, J. & Bhattacharya, M. (2016). Intelligent financial fraud detection: a comprehensive review, Computers & Security, 57, pp. 47-66.
- Wheeler, R. & Aitken, S. (2000). Multiple algorithms for fraud detection, Knowledge-Based Systems 13(2/3), pp. 93-99.
Copyright information
This work is licensed under a Creative Commons Attribution-NonCommercial-NoDerivatives 4.0 International License.
About this article
Publication Date
20 December 2017
Article Doi
eBook ISBN
978-1-80296-033-4
Publisher
Future Academy
Volume
34
Print ISBN (optional)
--
Edition Number
1st Edition
Pages
1-442
Subjects
Business, business studies, innovation
Cite this article as:
Alma, B., & Coskun, E. (2017). Strategic Decision Making Of Micro-Lenders: An Innovative Business Model For Risk Management. In M. Özşahin (Ed.), Strategic Management of Corporate Sustainability, Social Responsibility and Innovativeness, vol 34. European Proceedings of Social and Behavioural Sciences (pp. 308-321). Future Academy. https://doi.org/10.15405/epsbs.2017.12.02.26