Abstract
People are thought to lie somewhere along the spectrum between two opposing theories of intelligence, the incremental theory and the entity theory. Individuals with a growth mindset tend to believe that intelligence and ability can grow with effort and practice, while individuals with a fixed mindset tend to believe that peoples’ level of skill and intelligence is predetermined and innate. Individual differences in beliefs and perceptions about intelligence and ability manifest themselves through differences in motivation and goal-seeking behavior. Typically, individuals who hold a growth mindset have been linked to be more mastery goal oriented, as opposed to fixed mindset individuals, who are associated with more performance orientation. Research has shown that individuals exposed to a growth mindset significantly perform better on mathematics tasks than individuals with a fixed mindset (
Keywords: Growth mindsettheories of intelligenceentity theoryincremental theorygoal orientationimplicit theoriesnumber lineestimation
Introduction
Implicit Theories of Intelligence
The mindset of an individual plays a critical role in delegating all areas of a person’s life, including how one perceives circumstances, makes decisions, approaches or avoids challenges, and it has profound control over behaviors an individual exhibits or tends to shy away from. The beliefs about intelligence and ability in particular, are the focal mindsets of the current study. This is best known in the literature as an individual’s implicit theory of intelligence. There are two theories; an incremental theory and a pure entity theory. The incremental theory of intelligence holds the belief that intelligence is malleable and can be developed through effort and practice. Individuals who hold an incremental theory are characterized as having a growth mindset of intelligence. The pure entity theory of intelligence believes that intelligence is an innate trait, and that the amount of intelligence one has is static. Individuals who hold an entity theory are characterized as having a fixed mindset of intelligence. People are thought to lie somewhere along the spectrum between the two opposing theories (Dweck, 1986; Dweck & Leggett, 1988).
Implicit Theories and Goal Orientation
Individual differences in beliefs and perceptions about intelligence and ability manifest themselves through differences in motivation and behavior. Dweck & Leggett (1988) constructed a model of how individuals’ theory of intelligence orients them towards pursuing particular goals. They outline two types of goal orientations: learning, or mastery goal orientation, and performance goal orientation. The motivation that fuels behavior for those with a mastery goal orientation is that they perform a task for the purpose of developing their skill and increasing competence. In contrast, behavior for those with performance goal orientation is propelled by the desire to gain positive judgments or avoid negative judgments from others about their competence. Both of the goal orientations, however, produce mastery-oriented behavior of some sort. Performance goal with the intention of gaining positive judgments is mastery oriented because it exhibits challenge seeking and high persistence, often to prove their ability. In this paper, I refer to this type of performance goal orientation as performance-approach goal orientation. Learning goal orientation is identical to this category of performance goal with regards to its mastery-oriented nature, with the exception that the intention is to foster learning. The one category that is not inline with this behavior pattern is performance goal orientation with the intention to avoid negative judgments. In this paper, I refer to this type of performance goal orientation as performance-avoid goal orientation. Typical behavior pertaining to this subcategory of performance goal orientation is characterized as helplessness, displaying low persistence and avoiding challenges.
Though there is a fairly unanimous agreement on the general definitions of the implicit theories, there are a number of terms that are often used interchangeably in the literature that all refer to the same concept. Incremental theory and growth mindset, as well as entity theory and fixed mindset are used interchangeably, and are often used in association with learning goal orientation and performance goal orientation, respectively. It is also common for learning goal orientation to be referred to as mastery goal orientation, because of its mastery and self-improvement focused behavior. Pintrich (1999) offers definitions for the remaining two performance goal orientations that slightly deviate from the definitions given above. He labels them as extrinsic orientation and relative ability orientation. The extrinsic orientation is defined as having a focus on getting good grades and pleasing others as the main criterion, while the relative ability orientation is concerned with comparing one’s ability to others.
Regardless of the definition, consensus in the literature support that theory of intelligence is a consistent predictor of goal orientation and behavior (Dweck & Leggett, 1988; Elliott & Dweck, 1988; Blackwell et. al., 2007; Hong et. al., 1999.) A study conducted by Blackwell and colleagues (2007) found that students with a learning goal orientation held stronger beliefs about the power of effort in promoting ability, and that mindsets predicted mathematics achievement. They found that those who held growth mindset beliefs were significantly more oriented toward learning goals, and displayed more mastery-oriented behavior to setbacks compared to fixed mindset individuals, who displayed more performance orientation behavior and were more oriented towards validating their intelligence.
A study that focused on the relationship between implicit theories and remedial actions found that incremental theorists were more inclined improve their performance when they found their skills to be unsatisfactory, in comparison to entity theorists, who were less inclined to take a remedial course, even knowing the skills they lacked were essential for future success (Hong et. al., 1999). In an attempt to test the causal relationship between implicit theories and goal orientation behavior, Hong and colleagues manipulated the participants’ implicit theories of intelligence and analyzed their likelihood of taking remedial action in response to setbacks. They found that participants who were prompted to believe an incremental article were more likely to take the tutorial and attribute effort after receiving negative feedback compared to participants who were prompted with an entity article. There is considerable evidence in the research suggesting that implicit theories of intelligence and goal orientations are determinants of goal seeking behavior.
Goal Orientation, Motivation, and Achievement
Research supports that goal orientation predicts motivation and achievement (Eppler & Harju, 1997; Grant & Dweck, 2003) and is often facilitated by self-regulatory strategies aimed toward goal attainment (Ames, 1992; Pintrich, 1999). Individuals invested in learning goals put forth more effort into monitoring their individual progression. Research had shown a pattern of individuals with learning goal orientation as having higher levels of intrinsic motivation, effort attributions, planning, and desire for positive growth (Grant & Dweck, 2003). According to Pintrich (1999), there are consistent relations between goal orientation and self-regulated learning; the goal orientation that students adopt sets the standard for the self-regulatory strategies they execute. He found a strong relation between mastery goals and self-regulatory strategies, whereas, negative relations were found between extrinsic goals and self-regulatory strategies.
Present Study
The present study attempts to take a closer look into the individual differences in goal directed behavior between those who hold differing implicit theories of intelligence and goal orientations. The researcher is interested in how goal-seeking behavior is exhibited in a mathematical context. In a study that compared the performance of college students who were either prompted with a fixed mindset explanation for gender differences in math achievement or a growth mindset explanation for differences in math achievement, individuals who were exposed to the growth mindset explanation performed significantly better on the following mathematics task than those given a fixed mindset prompt (Dar-Nimrod & Heine, 2006). The present research is interested in examining whether similar findings will result on a number line estimation task between individuals who already hold a growth or fixed mindset disposition. The primary goal of this study is to analyze how participants’ implicit theories, goal orientations and goal seeking behaviors differ with respect to how they approach number line estimation problems presented in a digital game environment.
A number line estimation task provides a good environment to investigate this relationship because repetition using the digital software enables participants to improve their estimation skills over time, and additionally, offers the opportunity for participants to take strategic measures to enhance their performance.
People in general are poor estimators, and even adults, who consistently demonstrate greater accuracy relative to children on number line estimation tasks, show patterns of error and estimation bias (Sullivan et. al., 2011). Research has shown that children can improve their estimation performance, and that a predictor of performance was their numerical categorization abilities (Laski & Siegler, 2007). Our research presents an opportunity to examine if adults can improve their number line estimation skills in a similar fashion, and enables them to utilize their own goal seeking strategies in the process if they please.
It is theorized that sorting numbers into categories will help with numerical estimation along a number line. The present study incorporated a numerical categorization task, similar to that used in the Laski & Siegler study, which required the participants to categorize a given number into one of five groups: really small, small, medium, big, and really big. The rationale behind implementing this exercise prior to each number line estimation task is to provide the most optimal opportunity for participants to improve and demonstrate their estimation skills within the short 30-minute intervention.
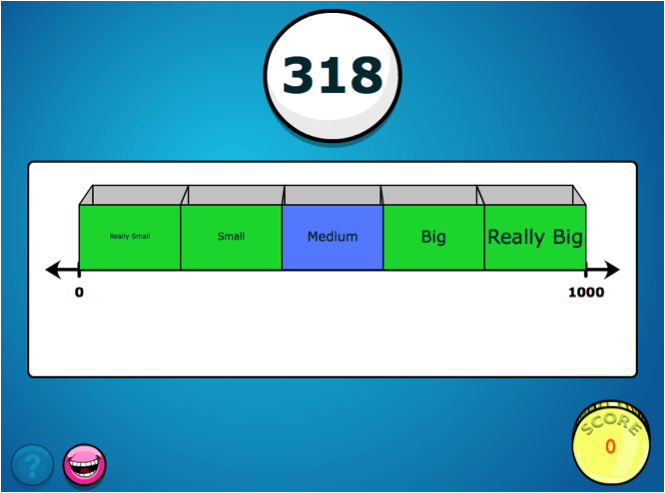
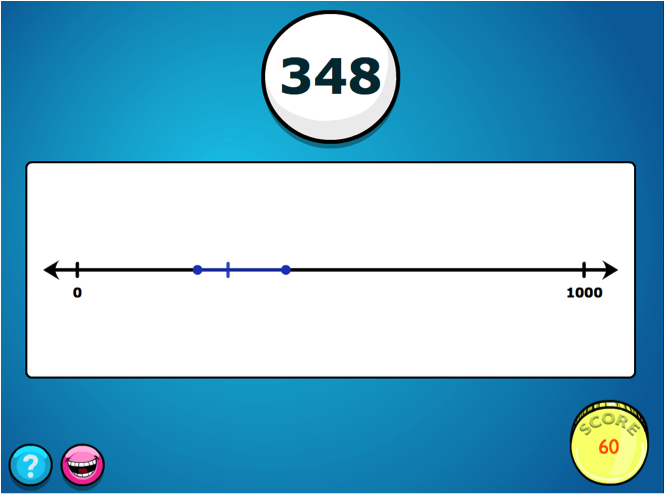
The numerical categorization (Figure
The participants interacted with the software by using the mouse or arrow keys to specify the category and the location of a number on a digital number line. The number line estimation exercise supports a maximum of two attempts per trial and delivers scaffolded feedback that guides the subject in the correct direction and enables them to employ goal directed strategies after an initial failed attempt. Opfer & Siegler (2007) found that feedback produced beneficial changes in children’s estimates along a 0-1000 number line.
The number line estimation task also allows the user to not only define the location of a specific number on the number line, but also the confidence interval, or range in which they perceive it falls between. This feature allows the user to define the level of risk. An important aspect of using games as a learning system is to allow players feel comfortable to take risks without fear (Gee, 2010). The ability of the participants to manipulate the arms of the confidence interval long and short, to make the range as wide or as narrow as they please until they are confident that the interval likely encapsulates the target number enables the participants to control their own cost of failure. This user defined range feature of the software provides a nuanced way to gauge participants’ assurance for each of their individual estimates. It also helps to assess risk-taking behavior. The less certain the individual is about their estimate, the wider the confidence interval, the less risk associated with that estimate. Alternatively, greater degrees of perceived certainty in the estimate are associated with narrower confidence intervals and higher risk taking behavior on the number line estimation exercise.
The researchers hypothesize that individuals who are learning goal oriented will be looking for ways to enhance their numerical categorization skills, and the effects of such an improvement will carry over into number line estimation performance. We predict that those with a growth mindset will be more likely than fixed mindset individuals to attribute more precision on their second estimation attempt when initially faced with failure. Similarly, those with a performance goal orientation are predicted to employ more strategies that ensure the attainment of extrinsic rewards within the game compared to mastery oriented individuals, who are expected to demonstrate more challenge seeking strategies.
Research Questions
The present research study attempts to address the following research questions:
Is there association present between growth mindedness and mastery goal orientation, and between a fixed mindset and performance goal orientation?
Is there a difference in performance, as measured by percent absolute error (PAE), between growth and fixed mindset individuals?
Is there a difference in performance, as measured by percent absolute error (PAE), between mastery, performance-approach and performance-avoid goal orientations?
Is there a difference in behavior with respect to user defined range (UDR) manipulation of the confidence interval, between growth and fixed mindset individuals?
Is there a difference in behavior with respect to user defined range (UDR) manipulation of the confidence interval, between mastery, performance-approach, and performance-avoid goal orientations?
Is there a difference in performance, as measured by PAE, between individuals of high and low mastery, performance-approach, and performance- avoid goal orientations?’
Is there a difference in behavior, as measured by UDR between individuals of high and low mastery, performance-approach, and performance- avoid goal orientations?
Method
Participants
There was a total of 26 adults who participated in the present study, 7 of who were excluded because there were no record of their MASE log files. The results of this study focuses on the 19 remaining participants who completed all portions of the present study. Participants were recruited by word-of-mouth and email announcements. Participation was voluntary. Participants were informed of the purpose of the present study, and were given a detailed overview of the procedure and time duration of the study activities before consent was obtained. A majority of participants were female (17) and 2 male, and they ranged in age from 21 to 32 years, with a mean of 26.11 years (M=26.11, SD=2.787). The sample consisted of 47% Asian and 53% Caucasian participants. The participants’ highest level of education ranges from undergraduate to graduate level, with 3 and 14 participants, respectively, with highest-level math course ranging from the high school to undergraduate level. Five participants have taught a mathematics course at the elementary and/or middle school level for less than a five-year period.
Procedure
Participants were provided a link to a web-based survey which contains all four study activities, the first of which being the Motivation and Learning Questionnaire, a set of items in which they disclosed their self-report responses. The participants were informed that the purpose of the questionnaire was to seek the participants’ personal opinions on motivation and learning.
The second activity was the MathemAntics Sort and Estimate (MASE) activity. The activity description in the online survey described that the activity required participants to sort specified numbers into a size category and to pinpoint a specific number on the number line provided. Participants were informed that the software would display a number, and their task was to locate that number on a number line that ranged from 0-1000. They were notified that they will see that the location they select would also signify a confidence interval and that they had the ability to manipulate the arms of the confidence interval long and short, to make the range as wide or as narrow as they pleased to encapsulate the target number. Participants used their own personal computer to complete the MASE activity and were required to download the software and settings file to their computer. This study required that the settings for the software were preset to accommodate the research objectives.
The MASE activity consisted of 30 sort trials and 30 estimate trials, resulting in a total of 60 scored trials. Instructions were given for the first three trials for both the numerical categorization and number line estimation activities. Instructions for the numerical categorization activity were: “Here are boxes labeled by size, click the box where [number appears at the top of the screen] belongs.” For the estimation activity, the program said, “The number line is still from zero to [pause], where should we place [number appears at the top of the page] on the number line? Use the mouse or arrow keys and then press the spacebar to submit your answer.” When a participant clicked a location on the number line, the program announced, “Show how sure you are and then press the spacebar,” as the error range for the chosen location is highlighted in blue and blinked on and off. If the participant submitted an incorrect estimate, the software responded by guiding the direction of the correct estimate saying, “Try again, your estimate is too [high/low]. Immediately following, the midpoint of the number line (500) appeared as did an arrow pointing to the first attempt estimate for scaffolding purposes. During number line estimation exercises, adults typically fixate on the midpoint as a point of reference (Sullivan et. al. 2011), so the researcher chose to display the midpoint for the second attempt. When a correct answer was submitted, chimes sound and the software responds with the following: “good estimate!”, “great estimate!”, or “awesome estimate”. Then, the coins appeared above the estimate paired with a cash register sound, signifying that the participant had just been awarded points. The participant then pressed the “next” button to proceed to the next trial. Players received two possible attempts to answer correctly for every number line estimation trial.
The target numbers on both the numerical categorization and number line estimation tasks were 17, 42, 86, 121, 155, 168, 203, 264, 291, 318, 329, 348, 412, 457, 470, 533, 561, 596, 609, 650, 674, 732, 780, 795, 824, 845, 889, 907, 936, and 973. This specific group of 30 numbers were selected to avoid any chance of number biases because (a) across the entire target number list as a whole, digits 0-9 are incorporated an even total of three times in each decimal place (hundreds place, tens place, ones place), (b) there are three target numbers present for every interval of 100, (c) there is an equal number of thirteen 100s place-large,10s place-small digits (ex. 203) and 100s place-small, 10s place-large digits (ex.121), with 1 even 100s and 10s place digits (ex. 889), excluding the three double digit numbers, (d) there is an equal number of fourteen 10s place-large,1s place-small digits (ex.42) and 10s place-small,1s place-large (ex.17), with 2 even 10s and 1s place digits (ex.155), and (e) there is an almost equal number of 100s place-Large, 1s place-Small digits (ex. 291), and 100s place-Small,1s place-Large (ex.168). The ratio is 15:14, respectively, with 1 even 100s and 1s place digits (ex.121). The target numbers were pseudo-randomly ordered only to ensure that the target number for numerical categorization did not match the target number in the number line estimation task that immediately followed. The target number pairs were presented to the participants at random.
Players received 10 points for every correct response, and plus a bonus 10 points if they answered correctly on the first attempt. Zero points were awarded for incorrect responses. It was important that the system offered the same number of points for every correct response regardless of the user defined confidence interval range size. Implementing an advantage in reward points for more restricted user defined ranges would add an additional extrinsic motivator to the system that would confound our research findings. Because this study aimed to compare the behavior of individuals with varying goal orientations on one particular task, setting a fixed amount of reward points allows distinction between goal orientation behavior since the only motivation for making the confidence interval smaller is mastery of estimation accuracy. Thus, performance and mastery individuals received the exact same score rewards for any user defined range (UDR).
After completing the Sort and Estimate activity, students returned to online survey to complete two additional number line estimation exercises for target numbers (372 and 768 and were asked to describe how they had arrived at their estimation. This was step three of four total for this study. The fourth and final step of this study asked students to disclose their demographic information in a final set of survey questions. All data was collected individually through electronic administration of the online questionnaire and email submission of log data files to the researcher.
Measures - Motivation and Learning Questionnaire
The present study relied on the numerical values derived from the participants’ responses to the self-report measures containing items rated on a 6-point Likert-type scale from 1 (Strongly Disagree) to 6 (Strongly Agree). Two sets of scales designed to measure implicit theories of intelligence and goal orientation were used to determine the participants’ motivational disposition.
The theory of intelligence subscale (𝝰=.92,
The fourteen-item goal orientation subscale was taken directly from the Patterns of Adaptive Learning Survey [PALS], Personal Achievement Goal Orientation subscale, which measured the motivation behind learning and achievement (Midgley et al., 1998). Five items of the goal orientation subscale measured Mastery Goal Orientation (𝝰=.77,
Measures - Session Data
The MASE software produced game play logs that archived the participant ID, configuration settings, the number of trials, number of attempts per trial, number of correctly answered questions, amount of time it took to answer a question, percent absolute error (PAE) per trial, the user defined range, the extent to which the range was altered in each direction. Percent absolute error is defined as: PAE = (Actual Value - Observed Value) / Scale.
Measures - Demographic Survey
A demographic survey was administered at the culmination of the study to gather background information on the subject. The survey consisted of questions regarding age, gender, ethnicity, highest level of education, highest-level math course, and whether or not the participant has taught a math course and in what context.
Results
Question 1: Is there association present between an incremental theory of intelligence and mastery goal orientation, and between entity theory and performance goal orientation?
To determine whether theories of intelligence were associated with goal orientation, the researcher first calculated each participant's’ average score on each of the four subscales: mindset, mastery goal orientation, performance-approach goal orientation, and performance-avoid goal orientation. These measures were then used to calculate the correlations between mindset and each of the three goal orientations (Table
A Chi-Squared analysis was conducted comparing the mindset, mastery, performance-approach, and performance-avoid groups to determine whether there was a difference between expected and observed frequencies in each group (Table
Question 2: Is there a difference in performance, as measured by percent absolute error (PAE), between growth and fixed mindset individuals?
Because PAE was used as a measure of performance, the computer log files were used to calculate participants’ mean PAE across the 30 trials, and then this mean was correlated against their mean mindset score. These calculations would indicate whether or not there was a significant association between the two variables, and the direction of the association. No significant correlation was found between mindset and PAE, r=.14, p=ns, and thus there was no evidence to suggest that the two variables are strongly associated.
Then, to test the difference in performance between individuals with a growth mindset as opposed to a fixed mindset, we split the sample into the two subsequent groups, using the mean as the point of division. The mean score and median score for the mindset subscale were equal for this sample. 58% of the participants were in the high (growth mindset) group and 42% were in the low (fixed mindset) group. Results of an independent samples t-test comparing growth and fixed mindset groups’ mean PAE scores revealed no significant difference between the two groups, t(17)=1.25, p=ns. Our findings suggest that there are no observable differences between growth and fixed mindset individuals’ PAE scores.
Question 3: Is there a difference in performance, as measured by percent absolute error (PAE), between mastery, performance-approach and performance-avoid goal orientations?
*p<0.5
A similar analysis was conducted to determine whether there was a difference in performance between the three goal orientations. The participants’ average scores for mastery, performance-approach, and performance-avoid goal orientations were calculated and correlated against their mean PAE across the 30 trials (Table
Question 4: Is there a difference in behavior with respect to user defined range (UDR) manipulation of the confidence interval, between growth and fixed mindset individuals?
Using the computer log files, the mean UDR was calculated for each participant and correlated against the mean growth mindset scores. No significant correlation was observed between the two variables, r(17)=-.11, p=ns, suggesting that mindset and UDR are not closely associated with one another. An independent t-test was analyzed to determine whether there was a difference in UDR between growth and fixed mindset groups. The results of this calculation suggest that there is no difference in UDR manipulation between growth and fixed mindset groups, t(17)=-.79, p=ns. These findings suggest that there is no perceivable difference between how growth and fixed mindset individuals defined their confidence interval in during the MASE activity.
Question 5: Is there a difference in behavior with respect to user-defined range (UDR) manipulation of the confidence interval, between mastery, performance-approach, and performance-avoid goal orientations?
The participants’ mean UDR scores were correlated against their mean scores for the mastery, performance-approach, and performance-avoid goal orientation scales scores (Table
Question 6: Is there a difference in performance, as measured by PAE, between individuals of high and low mastery, performance-approach, and performance- avoid goal orientations?
To test the difference between individuals who are categorized as having high mastery orientation versus individuals who have low mastery orientation, the sample was split into two groups, high and low mastery, using the mean score as the divider. The mean score and median score were equal for this sample. 58% of participants were categorized as having high mastery orientation, and the remaining 42% of participants were categorized as having low mastery orientation. Results of an independent samples t-test comparing the high and low mastery groups’ mean PAE scores revealed no significant difference between the two groups, t(17)=-1.078, p=ns, indicating no observable difference between the high and low groups.
A similar procedure was conducted to compare high and low performance-approach, and performance-avoid groups. The participants were split into 58% high, 42% low performance-approach groups, and the 63% high, 37% low performance-avoid groups. The mean was used in both cases as the divider, since the means and medians were also equal. An independent t-test was performed comparing the mean PAE scores of the high and low groups for each performance goal orientation. The calculations suggest there is no significant difference in mean PAE scores between the high and low groups for the performance-approach goal orientation, t(17)=.67, p=ns. A non statistically significant difference was observed between the high and low performance-avoid groups, t(17)=1.98, p=0.65. These findings suggest that there is a marginal difference in performance as measured by PAE between the high and low performance-avoid groups. This trend characterizes the high performance-avoid group’ mean PAE scores has being marginally higher than the low performance-avoid groups’ mean PAE scores.
Question 7: Is there a difference in behavior, as measured by UDR between individuals of high and low mastery, performance-approach, and performance- avoid goal orientations?
A series of independent sample t-tests were performed to examine whether there is a difference in mean UDR scores between individuals of high and low mastery, performance-approach, and performance-avoid goal orientations. Results indicated no significant difference in mean UDR scores between high and low mastery goal orientation groups (t(17)=-1.3, p=ns), high and low performance-approach groups (t(17)=1.65, p=ns), and high and low performance-avoid groups (t(17)=.23, p=ns). These findings suggest there is no observable difference in manipulation of the confidence interval during the MASE activity between the high and low goal orientation groups.
Discussion
Overview of findings
No significant association found between incremental theory of intelligence and goal orientation
Contrary to what was expected, only a marginal positive trend between mindset and mastery goal orientation was found. A lack of significant findings in the present study conflicts with most of the literature in this field that suggests that implicit theories of intelligence are consistent predictors of goal orientation (Dweck & Leggett, 1988; Elliott & Dweck, 1988; Blackwell et. al., 2007). However, this association trend is positively directed, which is reflected in previous studies. The association is in a positive trend toward significance, meaning that there is an association between growth mindset and high mastery goal orientation, and conversely, fixed mindset and low mastery goal orientation, however, it is not yet statistically significant. It is likely that a significant correlation between growth mindedness and mastery goal orientation does exist, but our sample data is not strong enough to illustrate such a relation. These unanticipated findings may be due to the study’s small sample size and may not be truly representative of this relationship.
Higher Performance-Avoid Goal Orientation is related to less accurate estimates on the MASE activity
Although a strong association was anticipated between in PAE and mastery, performance-approach, and performance-avoid goal orientations, only a significant positive correlation between performance-avoid goal orientation and PAE was observed. This means that the performance-avoid goal orientation compared to other goal orientations, are more strongly associated with their PAE scores. A non-significant difference was also observed between the high and low performance-avoid groups, indicating that high performance-avoid group’ estimation performance has being marginally higher, and less accurate, than the low performance-avoid groups’ mean PAE scores. Combined, these findings suggest that the more inclined an individual is toward a performance-avoid orientation, the greater the PAE score and the less accurate the estimation performance. Because performance is often facilitated by self-regulatory strategies, one possible explanation for these results is that individuals who have high performance-avoid orientation are executing less self-regulatory strategies after initial failure of MASE task, leading to less accurate performance (Grant & Dweck, 2003; Pintrich, 1999). It is possible that individuals who were more mastery and performance-approach goal oriented were more inclined than those who were more performance-avoid oriented to employ self-regulatory strategies and during the MASE activity and used the numerical categorization task as a way to enhance their number line estimation performance. As you can recall, mastery and performance-approach goal orientations both have the desire for increased competence, and so it is plausible that individuals who are higher in these orientations adopted goal seeking behavior to enhance their estimation ability. However, results show that mastery and performance-approach goal orientations scores were less associated with their estimation performance.
No difference in performance between high and low mindset, mastery, or performance-approach goal orientations was observed
It was hypothesized that growth mindset individuals would outperform their fixed mindset counterparts, and likewise, the high mastery goal oriented individuals would outperform their low mastery goal oriented counterparts. No significant differences in performance were found comparing growth and fixed mindset groups, or high and low groups for mastery or performance-approach groups. These results are surprising because research has shown that individuals with a growth mindset, or incremental theory of intelligence, typically perform better than those with a fixed mindset, or entity theory of intelligence (Blackwell et. al., 2007; Hong et. al., 1999), and that mastery goal orientation is positively related to achievement (Eppler & Harju, 1997). It is possible that there is a difference between growth and fixed mindset, and high and low mastery and performance goal orientation, but our sample data is not strong enough to detect the difference. A possible explanation for these findings is that there is unequal distribution between the high and low groups. The mean score for the mindset subscale was 4.26, and mastery subscale was 5.13, meaning the distributions were negatively skewed in the more growth and mastery direction. The means needed to be used in these instances, since if they were to be split by using the median possible score from 1-6, dividing by high and low response options (3.5), this would only leave 2, and 1 participants for low mindset, and low mastery groups, respectively, and the comparison would be extremely uneven. The performance-approach high and low groups were also split using the mean (3.22), with a skewness of -.486. Future research should include an even distribution of high and low mindset, mastery, and performance-goal orientations for a better and more representative comparison.
No difference in behavior between high and low mindset and goal orientations was observed
It was anticipated that there would be a difference in the way growth and fixed mindset individuals manipulated their user-defined confidence interval. The researcher hypothesized that individuals who are more growth mindset inclined would produce more narrow UDRs compared to fixed mindset inclined individuals, given that they would have the desire to challenge themselves with precision, compared to fixed mindset folks who would have no incentive to make their UDR more narrow. It was also hypothesized that high mastery oriented individuals versus low mastery oriented individuals would display behavior parallel to mindset folks. There was no significant difference in behavior with respect to UDR manipulation between either groups in the MASE activity. This is surprising because research suggests that those with an incremental theory of intelligence and mastery goal orientation typically exhibit more goal seeking strategies and behavior, and was expected to be manifested in UDR behavior for the MASE activity. It can be argued that the UDR feature of the MASE activity provides a poor context for participants’ to express goal-seeking behavior and that the behavior illustrated in UDR data has nothing to do with their personal motivational goals.
The researcher also anticipated that there would be a prominent difference in UDR manipulation between mastery, performance-approach, and performance-avoid goal orientations, yet there was no observable difference in the way individuals manipulated their confidence interval in the MASE activity. Going even further, there was no significant difference between UDR manipulation of the confidence interval between high and low groups within the mastery, performance-approach, and performance-avoid goal orientations. These findings were even more surprising, since it was expected that performance-oriented individuals would extend their confidence intervals more than high mastery individuals, to ensure attainment of the external reward, with performance-avoid displaying this behavior exponentially. Again, the researcher attributes these lack of significant findings to unequal distribution between high and low groups. The researcher is not convinced that there is absolutely no difference in UDR behavior between the groups. It is possible that there does lie a difference in UDR manipulation between groups, however, our sample data is not strong or representative enough to detect a difference.
Implications
The findings from the present study have implications for researchers and educators. The present study found very little significant relationships between implicit theories of intelligence and goal orientations. Although research has shown that mindset is a predictor of goal orientation, these results imply that there may not be a definite trend from mindset to goal orientation. Next steps for researchers is to investigate what factors play a part into whether a person follows or deviates from the expected mindset to goal orientation trajectory.
Additionally, because no difference was found in performance between high and low mindset, mastery, and performance-goal orientation groups, and in behavior between all high and low groups, these results imply that there also may not be a definitive difference in achievement and goal seeking behavior, and that certain goal seeking behaviors can be employed by all goal orientations. It is important to note that there is no clear distinction of goal seeking strategies particular to one specific goal orientation. Future studies could examine the utilization of one particular strategy by each difference goal orientation and how they may differ.
This study also demonstrated how individuals who were high in performance-avoid goal orientation produced less accurate estimates on the MASE activity. It is important for educators to distinguish individuals who hold this disposition so they are able to provide individualize scaffolding of mathematical material for these students who may be struggling.
Limitations and Future Directions
The present study has several limitations. First, the sample size was small and had little diversity in terms of age, gender, ethnicity, education, and because recruitment was through email announcements and word-of-mouth, a majority of the sample attended the same education graduate school, with five having taught math at some point in their career. Additionally, there was very little variation in mindset and mastery scores for the sample. Future studies should be conducted across schools and locations for an even distribution of age, gender, ethnicity, education level and career fields. It would be ideal to have an even distribution of growth and fixed mindset individuals, as well as equal number of participants for each of the three goal orientations for comparison.
Second, though the study enables participants to complete the study at their own pace, giving them the luxury of setting their own time to participate and using their own computer device, this manner allows for inconsistency. Conducting future studies in a standardized fashion, where participants meet with the researcher one on one and use the same computer device for the activities ensures that each participant performs the study activities with the exact same screen size. This procedure will also allow the researcher to thoroughly explain the study activities and instructions directly to the participants, rather than having the participants read the description, which can result in different personal interpretations. The present study assumes that the participants’ have read and understood the description and instructions during the study, however, the fact that a number of the participants failed to complete one critical step that then led to them being excluded, suggests that there is major room for improvement in regards to the format of this study.
Finally, though the goal orientation scales used in the present study have been reliably validated, the scoring mechanism made it possible for the participants to score high in multiple goal orientations. Future studies should utilize an improved method of scoring the items in a way that organizes these goal orientations along a spectrum, or at the very least, has a way of accurately categorizing a participant into one distinct goal orientation group to provide a more accurate comparison of performance and behavior between the orientations.
Conclusion
The present study helps to shed some light onto the relations between implicit theories of intelligence, goal orientation, and goal seeking behavior with regards to a number line estimation activity. While there were little significant findings to be reported, the lack thereof also reveals a lot about the interactions between mindsets and goal orientations. What is certain is that a person’s theory of intelligence does not automatically dictate their personal goal orientation and goal seeking behavior.
References
- Ames, C. (1992). Classrooms: Goals, structures, and student motivation. Journal of Educational Psychology, 84, 261-271.
- Blackwell, L.S., Trzesniewski, K.H., Dweck, C.S. (2007) Implicit theories of intelligence predict achievement across adolescent transition: A longitudinal study and an intervention. Child Development, 78, 246-263.
- Dar-Nimrod, I., & Heine, S. J. (2006). Exposure to scientific theories affects women's math performance. Science, 314(5798), 435-435.
- Dweck, C. S. (1986). Motivational processes affecting learning. American Psychologist, 41(10), 1040-1048.
- Dweck, C. S., & Leggett, E. L. (1988). A social-cognitive approach to motivation and personality. Psychological Review, 95, 256 – 273.
- Elliott, E. S., & Dweck, C. S. (1988). Goals: an approach to motivation and achievement. Journal of personality and social psychology, 54(1), 5.
- Eppler, M.A., Harju, B.L. (1997). Achievement motivation goals in relation to academic performance in traditional and nontraditional college students. Research in Higher Education, 38, 557-573.
- Gee, J. P., & Hayes, E. R. (2010). Women and gaming: The Sims and 21st century learning. Palgrave Macmillan.
- Grant, H., & Dweck, C. S. (2003). Clarifying achievement goals and their impact. Journal of Personality and Social Psychology, 85, 541 – 553.
- Hong, Y. Y., Chiu, C. Y., Dweck, C. S., Lin, D. M. S., & Wan, W. (1999). Implicit theories, attributions, and coping: A meaning system approach. Journal of Personality and Social Psychology, 77(3), 588.
- Laski, E. V., & Siegler, R. S. (2007). Is 27 a big number? Correlational and causal connections among numerical categorization, number line estimation, and numerical magnitude comparison. Child Development, 78(6), 1723-1743.
- Opfer, J. E., & Siegler, R. S. (2007). Representational change and children’s numerical estimation. Cognitive Psychology, 55(3), 169-195.
- Pintrich, P.R. (1999). The role of motivation in promoting and sustaining self- regulated learning. International Journal of Educational Research, 31, 459-470.
Copyright information
This work is licensed under a Creative Commons Attribution-NonCommercial-NoDerivatives 4.0 International License.
About this article
Publication Date
22 November 2016
Article Doi
eBook ISBN
978-1-80296-015-0
Publisher
Future Academy
Volume
16
Print ISBN (optional)
-
Edition Number
1st Edition
Pages
1-919
Subjects
Education, educational psychology, counselling psychology
Cite this article as:
Villanuevaa, K. (2016). A Comparison of Mindsets and Goal Orientations Using Number Line Estimation Software. In Z. Bekirogullari, M. Y. Minas, & R. X. Thambusamy (Eds.), ICEEPSY 2016: Education and Educational Psychology, vol 16. European Proceedings of Social and Behavioural Sciences (pp. 96-112). Future Academy. https://doi.org/10.15405/epsbs.2016.11.11