Self-Configuring Evolutionary Algorithms Based Design of Hybrid Interpretable Machine Learning Models
Abstract
The paper describes an approach in which the decision-making process of an artificial neural network is interpreted by a fuzzy logic system. A neural network and a fuzzy system are automatically designed with the use of the self-configuring evolutionary algorithms. Experiments are carried out on classification tasks. As a result, it is shown that the building of a fuzzy system on the inputs and outputs of a neural network allows one to build an interpreted rule base of a smaller size, as if this rule base were built on the data of the original problem. In addition, the accuracy of such a system is comparable to the accuracy of a fuzzy system trained on the original task. As a result, the researcher has a neural network with high accuracy of solving the problem, as well as a fuzzy system explaining the neural network’s decision-making process. The article presents some constructed rule bases and neural networks for interpretation of which they were built.
Keywords: Fuzzy logic classifier, neural network interpretability, self-configuring evolutionary algorithms
Introduction
In last years a lot of attention to the theme of neural networks was paid. However, despite their effectiveness, neural networks have a disadvantage, which is the complexity of interpreting the resulting model. Trained neural network models are often referred to as a «black box». This means that information about how exactly the model makes a decision is not available. But there are also approaches results of which are a «white box» model. In such approaches, information about the internal relations of the model is available to the user. One of such approaches is systems based on fuzzy logic, since such models have a base of products rules that explain the decision-making process. However, models based on fuzzy logic are not always interpretable, because they often contain too many rules which can be very difficult to understand. In this regard, the idea of combining the two approaches to get advantage of each of them is prospective. The resulting system has the possibility of explaining how the neural network converts inputs into outputs, and itself also performs classification with acceptable accuracy.
Problem Statement
The use of non-interpreted machine learning methods is associated with great risks. These risks are primarily associated with a lack of understanding of how exactly the non-interpreted model makes a decision. An analysis of recent publications makes it clear that interest in the problem of interpretability and trust in machine learning is increasing.
Research Questions
In course of the study the following questions were raised:
- How can a fuzzy system be trained to describe the decision-making process of a neural network;
- With what accuracy can a fuzzy system describe a neural network;
- What accuracy on the initial problem will have a fuzzy system trained on the inputs and outputs of a neural network;
- Are the constructed rule bases different if one builds a fuzzy system on the original problem and build it on the inputs and outputs of the neural network.
Purpose of the Study
Supposedly, the answers to the questions above will help achieve this purpose and contribute to the development of interpreted machine learning systems.
Research Methods
Evolutionary neural network
The approach described in (Lipinsky & Semenkin, 2006) is considered below, in which the ANN structure is optimized using the self-configuring genetic programming method (Koza, 1990). ANN is encoded as a binary tree. The functional set consists of two operations: "+" – the operation of combining neurons into layers; ">" – the operation of ordering two structures one after the other. The terminal set contains inputs and hidden neurons with various activation functions. So, for a problem with 4 input variables, the terminal set may look like this: T = {in1, in2, in3, in4, 2rl, 2gs, 2sg, 2tg}. Where the number means the number of hidden neurons in the block, and the two symbols are the name of the activation function. So, "2sg" is a block with two hidden neurons with a logistic (sigma) activation function. Elements in1, in2, etc., are blocks of input neurons.
Using this method, it is possible to encode the network structure in the form of a process of constructing this structure from elementary parts of the network (neurons or groups of neurons). Figure 1 shows an example of encoding the ANN structure in a binary tree.
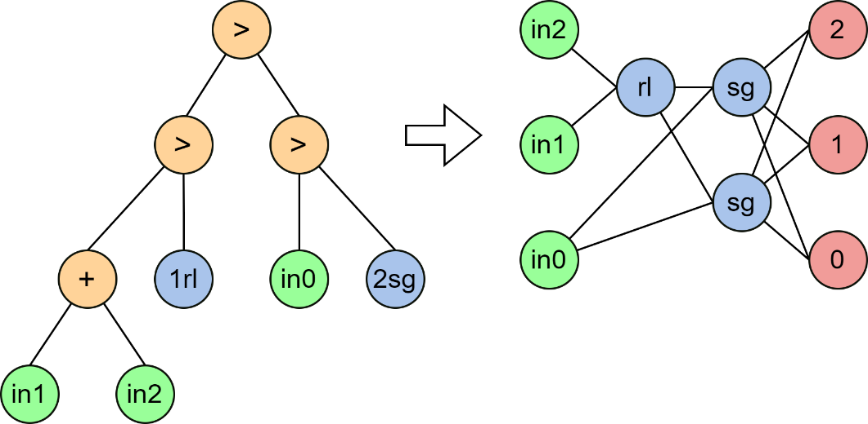
It can be noted that with the help of such coding, structures of varying complexity can be encoded: connections can pass not only from layer to layer; various activation functions in the same network; incompletely connected connections, etc.
The fitness function of the genetic programming algorithm forming the ANN is equal to the efficiency of the network on the problem being solved after learning its weighting coefficients. The tuning of the weight coefficients of such ANNs can be carried out by an evolutionary optimization algorithm (EA). In this paper, a self-configured genetic algorithm is used (Semenkina, 2013).
Fuzzy logic classifier
Fuzzy Logic Classifier (FLC) is a classification method based on the concept of a fuzzy set and the rules of fuzzy logic (Ishibuchi et al., 1999). The formation of FLC is reduced to the task of choosing the best rule base from all possible combinations of rules, in solving which EA can also be applied. The use of EA, however, is complicated by the need to choose the settings and parameters of evolutionary optimization algorithms (types of selection operators, mutation, crossover, etc.). To solve this problem, this paper uses the self-configuration method from (Semenkina, 2013), essence of which lies in the fact that when generating each offspring solution, the type of operator used is chosen randomly, and the probability of its choice increases for the type of operator that delivered the greatest average fitness for this generation by reducing the probabilities of less effective types of operators. Let be the number of different – type operators. The initial probability of choosing each of the operators is defined as. Further, the probability of the operator delivering the maximum average value of the fitness function is increased by the formula:
,
where is the number of generations of the algorithm, is a constant that regulates the rate of change in probability. The probabilities of using the remaining operators are recalculated so that the total sum of probabilities is equal to 1.
To use EA when building a rule base, it is necessary to encode the solution. There are two ways of such coding (Cordon et al., 2001): Michigan type, when an individual in a population is a rule, and Pittsburgh type, when an individual is a whole base of rules. In this paper, the second coding method is used, because it avoids the conflict between the fitness of individuals and the effectiveness of the rule base, although this leads to extra computational costs.
Description of datasets
Classification tasks are taken from open sources and were selected from essentially different areas, but in such a way that the resulting rule bases could be interpreted, and fuzzy variables would have an understandable physical meaning. The data sets:
- Blood transfusion service center data set (BTS) – the task of predicting a donor's decision to donate blood again (Yeh et al., 2009);
- Breast cancer Wisconsin (BCW) – the task of diagnosing cancer cells using the characteristics of cell nuclei (Street et al., 1993);
- Credit risk (CR) – the task of making a decision on granting a loan to a client based on information about the age, income and amount of the requested loan (Credit risk. Kaggle, 2022);
- Banknote authentication Data Set (BA) – a data set for solving the authentication problem, i.e. determining the authenticity of banknotes (Dua & Graff, 2019);
- User knowledge modelling data set (UKM) – the task of assessing the student's level of knowledge (Kahraman et al., 2013).
The applied approach
The resulting rule base should explain how the ANN converts inputs into outputs. To do this, it is necessary that the outputs of the fuzzy system correspond to the outputs of the neural network on the same inputs. Since classification problems are being solved, the approach used can be described as follows.
Let the objects be described by numerical features The space of feature descriptions is. Let be a finite set of class numbers (labels). Next, let the constructed ANN takes XNN at the inputs and returns YNN labels at the output. The algorithm of decisions interpretation of ANN using FLC can be described by the following steps:
At the output of the entire procedure, a base of FLC rules should be produced that interprets the rules for converting the ANN inputs into outputs.
To start the procedures for designing and forming ANN and FLC using self-configuration evolutionary algorithms, it is necessary to determine their hyperparameters. The following parameters were selected for the GP algorithm that adjusts the ANN structure: number of generations: 50; population size: 15; For FLC design, the following are selected: the number of terms for each linguistic variable: 3 (Small, Medium, Large); type of membership function: triangular; maximum number of rules: 10. The boundaries of the terms are distributed uniformly through the entire range of values of the variable. The parameters were selected in such a way that the resulting rule base was capacious and easy to interpret. Parameters of a self-configurable genetic algorithm solving the problem of building a rule base for FLC: number of generations: 300; population size: 100; penalty coefficient for the size of the rule base: 0.1. The parameters were selected considering the recommendations of the author of the method (Semenkina, 2013).
Findings
Since evolutionary optimization algorithms are stochastic procedures, the results obtained with their help are random. Therefore, the models were tested according to the scheme of multiple runs with 5 block cross-validation. As an estimation of the accuracy of the generated models (ANN and FLC), the percentage of correctly classified objects was used.
At the first stage, the ANN is automatically designed using self-configuring EA. Further, the ANN inputs and the obtained outputs are passed for automatic FLC construction. The accuracy of the FLC is defined as the number of matching outputs of the ANN and FLC. Having an FLC model trained on the ANN inputs and outputs, it is possible to evaluate its accuracy for the classification problem being solved. To do this, the FLC model gets inputs from the original dataset and the accuracy is estimated as the relative number of matching class labels from the dataset and FLC outputs.
Below, Table 1 shows the average accuracy of the obtained models for each classification task. For simplicity of perception, we introduce the following enumeration: 1 – the accuracy of the ANN on the classification problem; 2 – the accuracy of the FLC on the classification problem; 3 – the accuracy of the FLC on the inputs and outputs of the ANN; 4 – The accuracy of the FLC on the original classification problem.
Table 1 shows that FLC solves the classification problems under consideration worse (columns 1 and 2). The accuracy of ANN modeling using FLC is relatively high for all tasks except the last one (column 3). It can be noted that the accuracy of FLCs trained on the inputs and outputs of ANN is comparable to the accuracy trained by FLC on the original classification problem.
During the analysis of the results, it was noticed that the average number of prerequisites for approach 4 is much lower than for approach 2. For comparison, on the BCW task, the average number of prerequisites decreased from 15.98 to 8.15. As an example, consider the rule base for the BCW task:
if (mean radius is) and (mean texture is) and (mean perimeter is) and (mean area is) and (mean compactness is) and (mean concavity is) and (mean concave points is) and (mean symmetry is) and (radius error is) and (texture error is) and (compactness error is) and (concavity error is) and (concave points error is) and (symmetry error is) and (worst radius is) and (worst smoothness is) and (worst compactness is) and (worst concave points is) and (worst symmetry is) then Malignant
if (mean radius is) and (mean perimeter is) and (mean area is) and (mean compactness is) and (mean concavity is) and (mean symmetry is) and (mean fractal dimension is) and (radius error is) and (area error is) and (compactness error is) and (concave points error is) and (fractal dimension error is) and (worst texture is) and (worst area is) and (worst concavity is) and (worst concave points is) then Benign.
That is, the rule base includes only two rules, but the number of prerequisites in each of them is more than 15. Thus, despite the fact that the FLC algorithm returns rules that are understandable, in principle, to a person, the size of these rules does not allow to be easily interpreted.
At the same time, the rules based on the ANN inputs and outputs have a much smaller size, which contributes to their interpretation. To demonstrate this, table 2 presents the rule based obtained for the models whose effectiveness is presented in Table 1.
Additionally, let's consider the ANN structure constructed for the BCW problem. In figure 2, input neurons are marked in green, hidden neurons are shown in blue, and network outputs are shown in red. Hidden layers that have no input connections work as bias neurons and return 1.
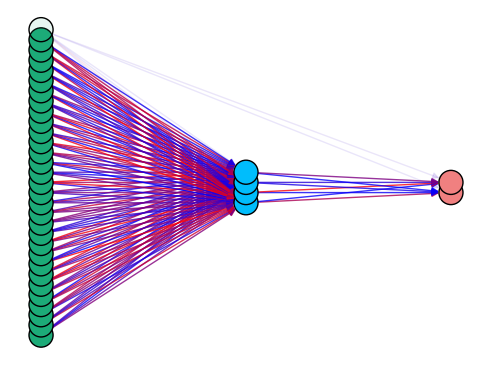
Although this ANN has a relatively small structure, it is difficult to determine how ANN converts input data into output. However, using FLC, it is possible to describe the ANN decision-making process. So, table 2 shows the rule base for the BCW task, consisting of only 2 rules, according to which it is easy to understand how does ANN from Figure 1 work.
table 2 shows that for most tasks the number of rules does not exceed the number of classes and the number of prerequisites in the rules varies from 1 to 4. Moreover, not all input variables are used to build rules. Thus, the rule base built on the inputs and outputs of the network has a much smaller size compared to the base built on the original data set with comparable classification accuracy.
Conclusion
This paper demonstrates the effectiveness of an approach that allows automatic designing simple and interpretable data mining models for classification problems using self-configuring evolutionary optimization algorithms. This approach consists in the fact that with the help of a self- configuring EA, an ANN is automatically designed that solves the classification problem with the required accuracy, then also with the help of EA, an FLC is automatically designed that interprets the results given by this ANN. At the same time, this FLC has comparable effectiveness, i.e. also sufficient accuracy and is based on a small number of simple rules. This distinguishes it from FLC, designed using the same approach based on self-configurable EA, which (FLC) turns out to be non-interpretable.
Acknowledgments
This work was supported by the Ministry of Science and Higher Education of the Russian Federation (Grant № 075-15-2022-1121).
References
Cordon, O., Herrera, F., Gomide, F., Hoffmann, F., & Magdalena, L. (2001). Ten years of genetic fuzzy systems: current framework and new trends. Proceedings Joint 9th IFSA World Congress and 20th NAFIPS International Conference, 3, 1242-1246.
Credit risk. Kaggle. (2022). Credit risk. Will the Customer pay the financing or not? https://www.kaggle.com/upadorprofzs/credit-risk
Dua, D., & Graff, C. (2019). UCI Machine Learning Repository. https://archive.ics.uci.edu/ml/datasets/banknote+authentication
Ishibuchi, H., Nakashima, T., & Murata, T. (1999). Performance evaluation of fuzzy classifier systems for multidimensional pattern classification problems. IEEE Trans. on Systems, Man, and Cybernetics, 29, 601-618.
Kahraman, H. T., Sagiroglu, S., & Colak, I. (2013). Developing intuitive knowledge classifier and modeling of users' domain dependent data in web. Knowledge Based Systems, 37, 283-295.
Koza, J. (1990). Genetic programming: a paradigm for genetically breeding populations of computer programs to solve problems. Stanford University.
Lipinsky, L., & Semenkin, E. (2006). Primenenie algoritma geneticheskogo programmirovaniya v zadachah avtomatizacii proektirovaniya intellektual'nyh informacionnyh tekhnologij [Application of the genetic programming algorithm in the tasks of design automation of intelligent information technologies]. Bulletin of the Siberian State Aerospace University, 3(10), 22-26.
Semenkina, M. E. (2013). Samoadaptivnye evolyucionnye algoritmy proektirovaniya informacionnyh tekhnologij intellektual'nogo analiza dannyh [Self-adaptive evolutionary algorithms for designing information technologies for data mining]. Artificial intelligence and decision-making, 1, 12-24. [in Rus.].
Street, W. N., Wolberg, W. H., & Mangasarian, O. L. (1993). Nuclear feature extraction for breast tumor diagnosis. IS&T/SPIE 1993 International Symposium on Electronic Imaging: Science and Technology, 1905, 861-870.
Yeh, I.-C., Yang, K.-J., & Ting, T.-M. (2009). Knowledge discovery on RFM model using Bernoulli sequence. Expert Systems with Applications, 36(3), 5866-5871.
Copyright information
This work is licensed under a Creative Commons Attribution-NonCommercial-NoDerivatives 4.0 International License
About this article
Publication Date
27 February 2023
Article Doi
eBook ISBN
978-1-80296-960-3
Publisher
European Publisher
Volume
1
Print ISBN (optional)
-
Edition Number
1st Edition
Pages
1-403
Subjects
Hybrid methods, modeling and optimization, complex systems, mathematical models, data mining, computational intelligence
Cite this article as:
Sherstnev, P. A. (2023). Self-Configuring Evolutionary Algorithms Based Design of Hybrid Interpretable Machine Learning Models. In P. Stanimorovic, A. A. Stupina, E. Semenkin, & I. V. Kovalev (Eds.), Hybrid Methods of Modeling and Optimization in Complex Systems, vol 1. European Proceedings of Computers and Technology (pp. 313-320). European Publisher. https://doi.org/10.15405/epct.23021.38