Abstract
Decision trees are an efficient data analysis tool. Ensembling methods have been developed on the basis of decision trees. These methods make it possible to obtain a data analysis tool in the form of a composition of trees. The paper proposes a new approach since the development of compositions based on decision trees is an urgent problem. The paper proposes a new hybrid approach to designing the composition of decision trees. The approach is based on the idea of the decision tree application built by a genetic programming algorithm as a technique to determine a machine learning method for object classification. Thus, with the help of the proposed approach the authors carry out a hybridization of a self-configuring genetic programming algorithm and a decision tree. The paper treats decision trees built by a modified algorithm with differential evolution considered as data analysis methods that make decisions concerning a sample objects classification. The proposed method is studied on some classification problems with different types of data and dimensions. The comparison with other methods for building compositions of decision trees is made.
Keywords: Decision trees, genetic programming algorithm, composition of algorithms, differential evolution
Introduction
Nowadays, decision trees are one of the most popular data analysis methods. The popularity is caused not only to its high efficiency, but the interpretability of the final result. Usually, a structure is selected and parameters of a separate technology are configured for solving problems of data analysis. After that, the problem solving is trusted to the best-found structure. However, a certain set of algorithms, when used simultaneously, according to the selected collective technology, often makes it possible to obtain a better solution. Such a set of algorithms is called an ensemble or composition (Mali et al., 2022; Ranzato, & Zanella, 2020).
It is proved that a combination of rather simple intelligent information technologies in their composition often led to an increase in the quality of problem solving. This trend can also be presented in ensembles built from decision trees. As an example, we can mention such methods for constructing compositions as random forest and gradient boosting (Hastie et al., 2009; Mason et al., 2000). They are undoubtedly very efficient. Moreover, the constant effort to improve results is quite normal. Therefore, the paper proposes an approach to constructing an ensemble of decision trees applying a decision tree constructed by a genetic programming algorithm for solving a classification problem.
Problem Statement
The classification problem is solved using a decision tree. The main disadvantage of decision tree learning algorithms is the complexity of choosing the splitting attribute of the original sample. This complexity lies in the fact that the classical algorithms for learning decision trees use exhaustive enumeration, which is obviously a resource-intensive procedure. The attribute selection procedure needs to be improved.
Research Questions
The present paper addresses the following Research Questions:
- The need to develop a procedure for combining decision trees into an ensemble based on evolutionary algorithms.
- Investigation of the efficiency of an evolutionary ensemble procedure for decision trees.
Purpose of the Study
The purpose of this work is to develop and study the decision tree ensemble procedure based on evolutionary algorithms. The article compares the efficiency of the procedure with modified decision tree encapsulation algorithms.
Research Methods
The paper proposes a new approach to constructing compositions of decision trees.
The authors of the paper propose the approach to select a decision tree for each individual classification object. First, the entire training set is divided into two subsamples. Then, the first subsample is divided into N samples applying the bootstrap method (Kozyrskiy et al., 2022). A decision tree is constructed with the help of the modified learning algorithm with differential evolution (CART+DE) on each of the N received samples (Mitrofanov & Semenkin, 2019). At each node the algorithm selects an attribute to separate a set of objects applying the Separation Measure method. Then, it optimizes a threshold value applying a differential evolution method. Figure 1 presents the main stages of this algorithm in the diagram.
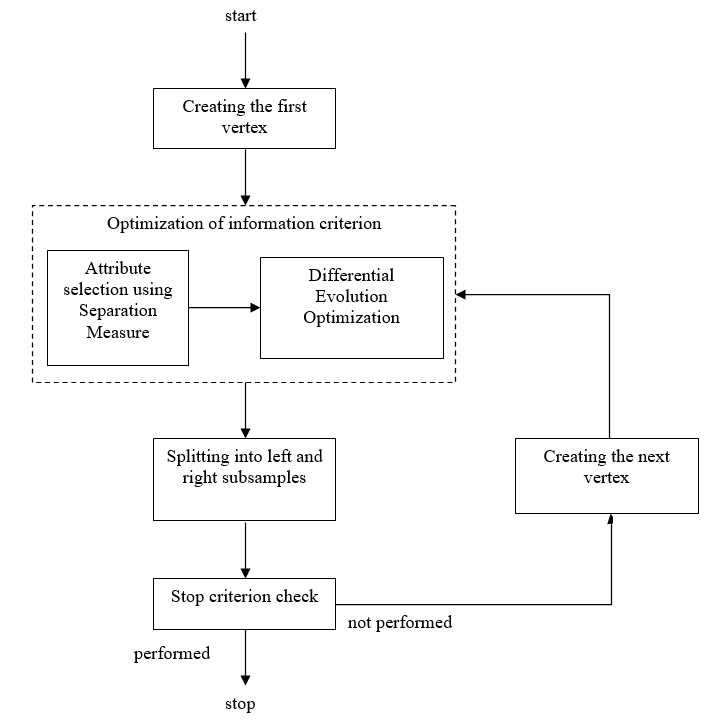
The resulting set of decision trees are passed to the genetic programming (GP) algorithm as a terminal set. A non-deep decision tree is designed with the help of genetic programming. However, in leaf vertices, i.e., as a terminal set, decision trees, but not class labels are applied. Thus, a training sample is divided into two subsamples. Decision trees are built on the first sample applying a modified algorithm for learning decision trees. The resulting set of decision trees is passed to the genetic programming algorithm as a terminal set. Then, the algorithm is trained on the second subsample. The result is a tree that, applying simple threshold rules, directs classification objects to various decision trees. Figure 2 presents design stages of the described composition.
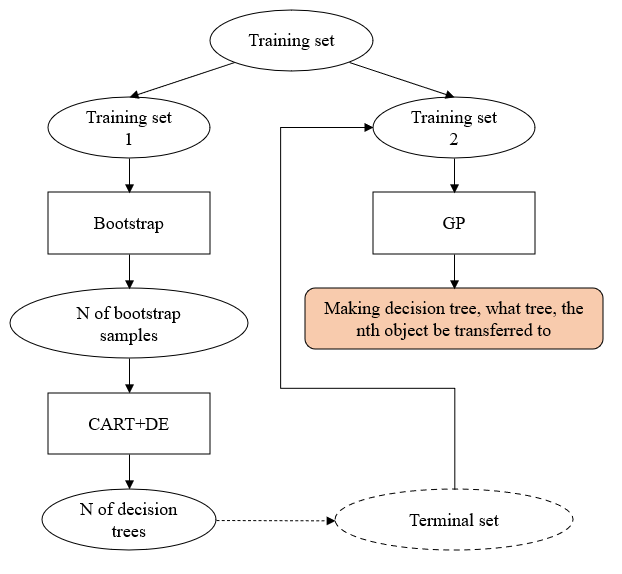
The authors built 50 trees applying a modified decision tree learning method with differential evolution in the proposed approach for each problem. They were transferred to genetic programming as a terminal set. In the genetic programming algorithm, 300 evolutionary cycles were performed, each of which included 50 individuals in the population.
Findings
Eight classification problems were applied to test the proposed approach to constructing an ensemble of decision trees applying a decision tree constructed by the genetic programming algorithm (Machine Learning Repository, 2022):
- Determining the type of car according to its technical characteristics.
- Recognition of the urban landscape.
- Determining the variety of rice.
- Diagnosis of Parkinson's disease.
- Recognition of the object type by its segment.
- Diagnosis of heart disease.
- Determining a type of soil from satellite images.
- Determining biodegradable chemicals.
The paper compares the best results of the standard compositions considered in (Mitrofanov & Semenkin, 2021) with the proposed approach (EGP).
The following approaches were applied for comparison:
- RF+DE: random forest modified by differential evolution.
In this approach, decision trees built applying the author's learning algorithm with differential evolution are combined into an ensemble according to the random forest principle:
- RF+GP: random forest modified by genetic programming algorithm.
In this approach, decision trees built applying a genetic algorithm are combined into an ensemble according to the random forest principle.
GBoost RapidMiner: classical gradient boosting implemented in the RapidMiner program (RapidMiner, 2022).
Table 1 presents results of classification problems solving by the listed methods.
Conclusion
The paper presents a new algorithm for constructing compositions from decision trees. The algorithmic basis of this approach is a self-configuring genetic programming algorithm.
According to the results obtained in the course of the work, we can conclude that the proposed approach to composition design works better than other methods. In the case when the modified random forest (RF + DE) also presents a high result, it is likely due to the fact that decision trees built according to a single algorithm are on their basis.
Acknowledgments
This work was supported by the Ministry of Science and Higher Education of the Russian Federation (Grant № 075-15-2022-1121).
References
Hastie, T., Tibshirani, R., & Friedman, J. (2009). Random Forests. The Elements of Statistical Learning: Data Mining, Inference, and Prediction (pp. 587-604). Springer-Verlag. DOI:
Kozyrskiy, B., Milios, D., & Filippone, M. (2022). Variational Bootstrap for Classification. Procedia Computer Science, 207, 1222-1231. DOI:
Machine Learning Repository. (2022). https://archive.ics.uci.edu/ml/index.php
Mali, R., Sipai, S., Mali, D., & Shakya, S. (2022). Parkinson’s disease data analysis and prediction using ensemble machine learning techniques. In S. Shakya, R. Bestak, R. Palanisamy, & K. A. Kamel (Eds.), Mobile Computing and Sustainable Informatics (pp. 327-339). Springer. DOI:
Mason, L., Baxter, J., Bartlett, P., & Frean, M. (2000). Boosting Algorithms as Gradient Descent. Advances in Neural Information Processing Systems, 12, 512-518.
Mitrofanov, S. A., & Semenkin, E. S. (2019). Differential evolution in the decision tree learning algorithm. Siberian Journal of Science and Technology, 20(3), 312-319. DOI:
Mitrofanov, S. A., & Semenkin, E. S. (2021). Tree retraining in the decision tree learning algorithm. IOP Conference Series: Materials Science and Engineering, 1047(1), 012082. DOI:
Ranzato, F., & Zanella, M. (2020). Abstract Interpretation of Decision Tree Ensemble Classifiers. Proceedings of the AAAI Conference on Artificial Intelligence, 34(04), 5478-5486. DOI:
RapidMiner. (2022). Fast. Forward. https://rapidminer.com
Copyright information
This work is licensed under a Creative Commons Attribution-NonCommercial-NoDerivatives 4.0 International License
About this article
Publication Date
27 February 2023
Article Doi
eBook ISBN
978-1-80296-960-3
Publisher
European Publisher
Volume
1
Print ISBN (optional)
-
Edition Number
1st Edition
Pages
1-403
Subjects
Hybrid methods, modeling and optimization, complex systems, mathematical models, data mining, computational intelligence
Cite this article as:
Mitrofanov, S. A., & Karaseva, T. S. (2023). Hybrid Evolutionary Approach to Decision Trees Ensembles Design. In P. Stanimorovic, A. A. Stupina, E. Semenkin, & I. V. Kovalev (Eds.), Hybrid Methods of Modeling and Optimization in Complex Systems, vol 1. European Proceedings of Computers and Technology (pp. 111-116). European Publisher. https://doi.org/10.15405/epct.23021.14